- 1Department of Biomedical Engineering, Health Engineering Innovation Center (HEIC), Khalifa University of Science and Technology, Abu Dhabi, United Arab Emirates
- 2Neurological Institute, Cleveland Clinic Abu Dhabi, Abu Dhabi, United Arab Emirates
Background: Gait dysfunction or impairment is considered one of the most common and devastating physiological consequences of stroke, and achieving optimal gait is a key goal for stroke victims with gait disability along with their clinical teams. Many researchers have explored post stroke gait, including assessment tools and techniques, key gait parameters and significance on functional recovery, as well as data mining, modeling and analyses methods.
Research Question: This study aimed to review and summarize research efforts applicable to quantification and analyses of post-stroke gait with focus on recent technology-driven gait characterization and analysis approaches, including the integration of smart low cost wearables and Artificial Intelligence (AI), as well as feasibility and potential value in clinical settings.
Methods: A comprehensive literature search was conducted within Google Scholar, PubMed, and ScienceDirect using a set of keywords, including lower extremity, walking, post-stroke, and kinematics. Original articles that met the selection criteria were included.
Results and Significance: This scoping review aimed to shed light on tools and technologies employed in post stroke gait assessment toward bridging the existing gap between the research and clinical communities. Conventional qualitative gait analysis, typically used in clinics is mainly based on observational gait and is hence subjective and largely impacted by the observer's experience. Quantitative gait analysis, however, provides measured parameters, with good accuracy and repeatability for the diagnosis and comparative assessment throughout rehabilitation. Rapidly emerging smart wearable technology and AI, including Machine Learning, Support Vector Machine, and Neural Network approaches, are increasingly commanding greater attention in gait research. Although their use in clinical settings are not yet well leveraged, these tools promise a paradigm shift in stroke gait quantification, as they provide means for acquiring, storing and analyzing multifactorial complex gait data, while capturing its non-linear dynamic variability and offering the invaluable benefits of predictive analytics.
1. Introduction
Stroke, defined as the sudden rupture or blockage of a cerebral blood vessel and consequent damage to central nervous system cells and tissues due to the interruption of oxygen supply, remains a major health challenge throughout the world. According to the World Health Organization (WHO), every year, 15 million people worldwide are diagnosed with stroke, of which, approximately 6 million die and another 5 million are left with permanent disabilities (1). Indeed, stroke is globally considered as the second leading cause of mortality for individuals above the age of 60 years, and the fifth leading cause of death in individuals aged 15–59 years (2).
While developed countries are reporting an overall decline in the incidence of stroke in the population below 65 years of age, its incidence is increasing in the developing world. Predictions for the next two decades indicate tripling in stroke mortality in Latin America, the Middle East, and Sub-Saharan Africa. In the United Arab Emirates (UAE), stroke is the second leading cause of disability, next to road accidents. Based on statistics from the Department of Health in Abu Dhabi (DOH), approximately 8,000–10,000 Emiratis experience a stroke each year, translating to at least one stroke occurring every hour (3). In addition to the high prevalence, 50% of stroke patients in the UAE are below the age of 45 years, which is approximately 20 years younger than the global average (3). Despite increased awareness and lifestyle changes, post stroke rehabilitation based on accurate and repeatable, objective assessment that leads to individualized rehabilitation protocols, and improved long-term mobility and quality of life is yet to be implemented on a large scale.
A variety of standardized stroke scales are currently used by clinicians to quantify stroke impairments, including neurological deficits and gait abnormalities (4) (see Table 1). Gait abnormalities in stroke patients are predominantly due to sensorimotor dysfunction, including muscle weakness, perceptual and proprioceptive deficits, spasticity or hypotonia. These impairments can affect a stroke victim in various degrees and combinations depending on the severity of the stroke (level A or minimal neurological deficit; level B or moderate deficit; and level C or severe deficit). Walking dysfunction for individuals with post-stroke gait impairment is often characterized by abnormal kinematic and kinetic patterns, deviations in the spatiotemporal features, altered muscle activation, and increased energy expenditure during walking. Early and effective rehabilitation with appropriate pharmacological and therapy interventions can help regain good ambulatory function with optimized modified gait patterns. While 52–85% of hemiplegic stroke patients regain their walking capacity, their gait patterns typically continue to differ from those of healthy individuals, with negative impact on biomechanics, overall body function, and quality of life (17, 18). Reduced walking speed, shorter and narrower steps, the inability to walk a mile (1,609 m) or difficulty to ascend a flight of stairs are observed to contribute to post-stroke disability (19).
1.1. Major Determinants of Ambulatory Function/Mobility in Stroke
Gait velocity of individuals with post-stroke gait impairment ranges from approximately 0.18 to 1.03 m/s, whereas that of healthy age-matched adults has an average of 1.4 m/s (20). Identifying the clinical features that are primarily associated with post-stroke walking ability is critical for the development of effective gait-training programs. In particular, impairments in muscle strength, motor function, and balance have been observed to be highly correlated with walking ability. The muscle strength of the affected hip flexor, ankle plantar flexors, knee extensors, knee flexor, as well as that of unaffected knee flexors and ankle plantarflexors are moderate to highly correlated (r = 0.5~0.8) with walking and stair climbing speed (20, 21). Motor function of the affected lower limb as rated by Fugl-Meyer Assessment is significantly correlated with gait velocity of patients with mild to moderate stroke (r ~ 0.6) (22). Indeed, balance function, as measured on the Berg Balance Scale, was highly correlated with functional walk distances [6 Min Walk Test (6 MWT) and 12 Min Walk Test (12 MWT)] at self-paced speed (r = 0.78–0.80) (23).
Other stroke-induced impairment, including spasticity, sensory function, and cardiovascular fitness have less impact on walking, and the association between these impairments with the walking velocity remains controversial (20). The correlation between the degree of spasticity and walking speed was also found non-significant (20, 24). Keenan et al. reported that tactile and proprioception impairments affect walking ability (25). However, Nadeau et al. and Dettmann et al. found no significant correlation between lower limb sensation and gait speed (22, 26). Further, cardiovascular fitness [Peak oxygen uptake (Vo2 peak)] was observed to be moderately associated with 6 MWT distance (r = 0.56) in sub-acute stroke (27).
1.2. Post-stroke Gait Assessment
In clinical gait assessment, both a person's “ability” to walk and “how” the individual walks are relevant. Walking ability of a person with stroke is a function of the stroke severity and is typically based on two main aspects: how far can an individual walk and what is his/her tolerance level. These are usually assessed using 3-, 6-, or 10- min walk tests. Functional Ambulation Category (FAC), Short Physical Performance Battery (SPPB), and/or Motor Assessment Scale (MAS) may also be employed for further assessment. On the other hand, the quality of gait or “how” the person walks is based on studying gait patterns and specific gait characteristics. Studied much more often in research settings rather than clinical settings, gait characteristics are nowadays assessed using instrumented gait analysis, including kinematic and kinetic assessment, which are beneficial for clinicians toward setting patient-specific quantitative functional ambulatory benchmarks and goals.
During the early stages of post-stroke recovery, patients often undergo qualitative observational, also referred to as visual gait analysis (using naked eye or video images) by physicians to evaluate gait performance and functional improvement (28). Table 2 lists various observational gait scales widely practiced in clinical settings. Current clinical assessment methods based on visual observation rely heavily on training and clinical judgment. However, despite being scrutinized for inter-observer variability (36), “observational gait analysis” methods continue to be popular among clinicians. This is due to the simplicity and availability of these tools, as well as their low cost (37). Nevertheless, validity, reliability, specificity, and responsiveness (38, 39) of these qualitative methods are questioned (36). In general, subjective observational gait scales may be useful for the rudimentary evaluation of some spatiotemporal and/or kinematic gait parameters but are not adequate for analyzing the multifaceted aspects of gait variability and complexity (for example kinetic and balance parameters). There is no consensus currently to when a more sophisticated gait analysis should be undertaken in stroke patients. The timing is likely to depend on the severity of the stroke, as well as parameters including fatigue, instability, pain, and consistent poor walking patterns despite fair to good muscle activity during passive examination.
Instrumented gait analysis, which has become standard in research settings in the 1990's, provides an accurate, reliable biomechanical gait evaluation approach incorporating key parameters (spatiotemporal, kinematic, and kinetic measures) (40). Gait labs typically include large, cumbersome, and expensive equipment, such as motion capture systems (Vicon, Motion Analysis Inc., Qualisys, OptiTrack, etc.), force plates (Bertec, Kistler, Noraxon, etc.), sensor-embedded walkways (Tekscan, GaitRite, etc.), and balance platforms, in addition to any other instruments deemed important for particular research applications, including post stroke gait assessment. Construction and configuration of these labs are specific to allow for accurate testing. For example, an area of at least 9 meter wide by 11 meter long with 3–5 meter ceilings (higher ceilings for stair climbing and sports applications) and special floors. Not surprisingly, the implementation of these labs in clinical settings remains scarce, not only due to the construction challenges and cost, but also the limited number of clinical practitioners appropriately skilled to conduct the tests and manage/interpret the large amount of generated data obtained from the array of possible sensor technology in use in post stroke gait rehabilitation. On the other hand, the clinical efficacy of 3-dimensional instrumented gait analysis (3DGA) has improved this last decade as reported by Wren et al. in their systematic reviews conducted in 2011 as well as in 2020 (41, 42). They classified studies based on the type of efficacy the studies addressed, which included technical, diagnostic, outcome prediction, diagnostic thinking and treatment, patient outcome, and societal efficacy. There is strong evidence suggesting a continued advancement of technology, including system accuracy and reliability, and data collection and analysis. From the clinical perspective, there is an increased use of 3DGA for understanding and evaluating the efficacy of treatment at a group-level. Also, the impact of 3DGA in treatment decision-making is evidenced. This includes diagnosing gait deficit and associated causes (e.g., toeing), devising treatment options (e.g., surgical or non-surgical), as well as changing and reinforcing treatment plans. In addition, the efficacy on individual treatment outcomes was also supported by studies. A scarcity of research analyzing the cost-effectiveness of 3DGA at a societal level recommends the need for further research toward this aspect (41, 42).
Today, small, light wearable sensors, such as inertial measurement units (IMU), pressure sensors, accelerometers, and various types of smart wearables are rapidly revolutionizing gait assessment in research settings and have the potential to be included in routine clinical practice. These sensors offer new opportunities for researchers to continuously record gait allowing the application of methods that quantify gait dynamics over time and can provide real-time feedback to patients and clinicians. Their light weight, portability and low-cost offer potential for research outside the lab and in natural environments (clinics, sports arenas, etc.). In addition, these sensors allow for easy synchronization with other physiological measurement equipment, such as EMG, ECG, and EEG, providing invaluable multifactorial continuous data of the subject/patient in various settings.
Recently, artificial intelligence, including machine learning techniques has emerged as a promising tool for processing instrumented gait data efficiently (43–47). These techniques have found applications in numerous problems relating to gait assessment, including dimensionality reduction, feature extraction, and classification. For instance, Zhou et al. employed Support Vector Machine (SVM), Random Forest (RF), and Artificial Neural Network (ANN) to classify young middle-aged, older adults, and geriatric patients, based on dynamic gait outcomes (46). Kernel Principal Component Analysis (KPCA) was incorporated for dimensionality reduction of the data for SVM classifiers. Lau et al. implemented SVM, ANN, and Radial Basis Function neural networks (RBF) classifiers to classify different walking conditions of hemiparetic patients, and found that SVM resulted in a highest overall classification accuracy of 97.5% (44). In addition, Lee et al. applied general regression NN for decision making process (43), whereas, Scheffer and Cloete used ANN, which was optimized to distinguish between hemiparetic stroke and able-bodied ambulation (45). These AI-based techniques have demonstrated capability of systematically analyzing and extracting information from an extensive amount of instrumented gait data that are multi-dimensional, highly non-linear, and/or too complex for analysis using traditional statistical approaches.
Considering the relevance of post stroke gait analysis in a clinical context and the potential value of a technological paradigm shift in that domain, this study includes the review of current literature up to January 2021 on post-stroke gait analysis with focus on conventional and advanced gait analysis tools and techniques that are low cost, commercially viable, easy to use, transportable and do not require excessive training for potential use in clinical practice. In particular, this review highlights:
1. basic measurement protocols required to assess post-stroke gait in clinical and/or research settings based on findings from the literature.
2. data processing and analysis protocols used by various researchers and the functional applicability of different techniques.
3. characteristics of gait in individuals with post-stroke gait impairment and the significant parameters used to assess gait deficits following a stroke.
This scoping review is primarily dedicated to bridging the gap between recent technology-driven engineering research gait studies and clinical applications, particularly in the area of post stroke gait. This gap can be seen in the faster adoption of the rapidly emerging gait assessment/analysis tools and technology in research labs as compared to clinical settings. Nowadays, gait studies can be performed using cost-effective, reliable and wearable sensors. These are invaluable when integrated into functional clinical application, where real-time gait analysis could be key to the development of patient-specific gait rehabilitation strategies and techniques. The main goal of this review is to provide a practical resource on technology-driven gait characterization and analyses tools and techniques and discuss their value and feasibility for clinical practice. The remainder of the review is structured as follows: section 2 describes the adopted methodology, including the approach, search strategy and selection criteria. Section 3 highlights the attributes of normal and pathophysiological gait, including post-stroke gait and mobility. Conventional and technology-driven gait characterization tools and technologies are reviewed in section 4, while section 5 summarizes associated conventional and technology-driven data processing and gait analysis. Section 6 sheds light on relevant gait and physiological parameters used to characterize post-stroke gait both in research labs and clinical settings. Novel state-of-the-Art AI techniques for gait analysis are described in section 7. Section 8 details the limitations of the study, while section 9 presents the conclusions and future work.
2. Methods
2.1. Study Approach
This paper appraises or evaluates the current use of biomechanics and physiological data recording and analyses for the assessment of post-stroke gait, as well as potential future directions including smart wearables and big data/artificial intelligence-based techniques. One of the main goals for this review is to serve as a practical resource for both engineers and physicians who deal with post-stroke gait assessment in research labs as well as clinics. As such, a broad topic area, including gait and its multifactorial physiological parameters, measurement tools and protocols, data analysis techniques, and future trends including AI and ML is included in this review.
As highlighted in (48), scoping reviews are an ideal tool for determining the coverage of a body of literature pertaining to the topic under study, determining the volume of the available studies, as well as, providing a broad or detailed overview of the focus. It can be used as a precursor to a systematic review. Scoping reviews also generally address a broader research question as compared to systematic reviews, and hence typically include more extensive inclusion criteria (48). Considering the nature of this study and intent, which are to identify the type of evidence and provide a broad overview about a wide scope of the aforementioned research area, the authors believe that a scoping review rather than a systematic review would best benefit both research and clinical communities.
2.2. Search Strategy
A comprehensive literature search was conducted within Google Scholar, ScienceDirect, and PubMed databases using a combination of keywords from the following groups: (1) “lower limb” or “lower extremity” or “lower-body”; (2) “walking” or “locomotion” or “movement” or “move” or “motion” or “gait”; (3) “analysis” or “assessment” or “quantification” or “evaluation”; (4) “stroke” or “post-stroke”; (5) “spatiotemporal” or “kinematics” or “kinetics” or “plantar pressure” or “Electromyography (EMG)” or “non-linear methods” or “machine learning” or “classification” or “statistical.” The search was limited to articles published in English until January 2021. The title and abstract of the search results were screened against the inclusion/exclusion criteria. The full text of the relevant articles was reviewed and those meeting the study criteria were considered for further analysis. The references were checked to identify additional articles for possible inclusion in this study (see Figure 1).
2.3. Study Selection Criteria
Articles were screened against the following inclusion/exclusion criteria. Articles for inclusion had to address (1) characteristics of post-stroke gait including biomechanical and physiological parameters, or (2) post-stroke gait quantification and analysis of lower extremity, or (3) gait measurement system/protocol, or (4) data analysis using traditional and non-traditional techniques. Studies addressing a sample population of post-stroke patients, who are able to walk are considered in this review. Studies that involve the application of special treatment strategies (e.g., use of botulinum toxin injection, aerobic exercise, etc.), movement therapy, robot-assisted therapy, and studies with robotic exoskeleton and/or ankle foot orthosis are outside the scope of this study and not included in the review. Articles analyzing subjects with spinal cord injury, multiple sclerosis, Parkinson's disease, and other diseases that interfere with gait were also excluded.
The articles meeting the inclusion and exclusion criteria were screened for value provided in gait quantification and analysis of post-stroke patients. The measurement methods were studied in detail, and the parameters considered were identified. The various techniques to analyze gait data and their applicability for clinical diagnostics/prognostics and personalized medicine were further examined.
2.4. Data Extraction
Following the screening of the full-text articles based on the criteria, a primary reviewer (DMM) performed the data extraction. A part of the data extraction was also carried out by an additional reviewer (SIIIA). The results were verified by (KK, HFJ, and AHK). The clinical application and relevance were discussed and confirmed by our clinical collaborator (SAW). The data extraction focused on three broad areas: characteristics of post-stroke gait, experimental/measurement/study protocol, and data analysis techniques. The characteristics included both biomechanical (e.g., spatiotemporal, kinematics, kinetics) and physiological parameters (e.g., heart rate variability). The measurement systems consisted of conventional techniques (e.g., footprint method) as well as the advanced techniques (e.g., pressure mat, motion capture, wearable sensors, etc.). The data analysis techniques incorporated statistical-based (e.g., ANOVA, linear regression, etc.) approaches as well as data-driven methods. Additionally, artificial intelligence techniques (e.g., ML, SVM) applicable to gait data analysis were also reviewed.
3. Introduction to Normal Gait and Pathophysiology of Human Gait
3.1. Normal Gait Pattern
According to (49), normal gait can be defined as a series of rhythmic, systematic, and coordinated movements of the limbs and trunk that results in the forward advancement of the body's center of mass. As such, human movement is characterized using individual gait cycles and functional phases. As illustrated in Figure 2, a gait cycle consists of two main phases, stance and swing, which are further divided into five and three functional phases, respectively (51, 52). The stance phase corresponds to the duration between heel strike and toe-off of the same foot, and it constitutes approximately 60% of the gait cycle. The swing phase begins with toe-off and ends with heel contact of the same foot and occupies 40% of the cycle. Each functional phase contributes to successfully establishing the goal of walking. Any abnormalities observed in the phases or events of gait may be linked to musculoskeletal and/or neuro-muscular complications, such as the case of individuals with post-stroke gait impairment.
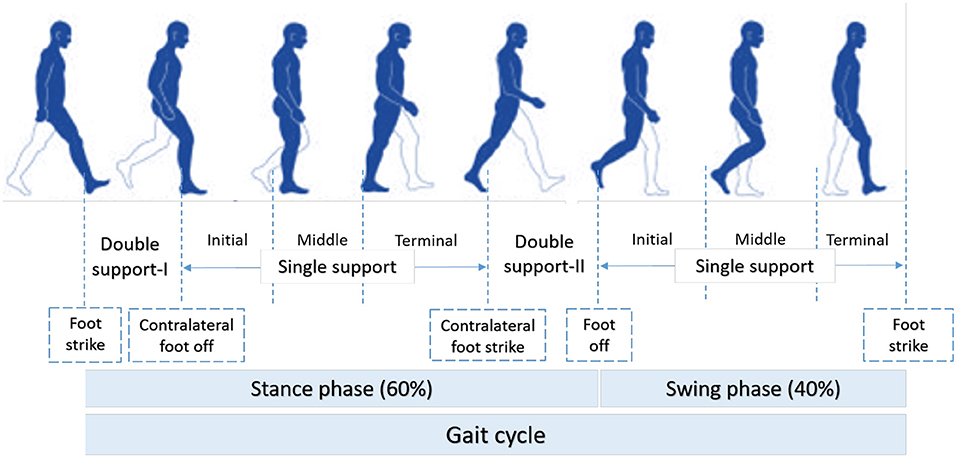
Figure 2. Functional phases of a normal gait cycle according to (50).
Many studies (53–62) have been conducted on healthy gait in order to derive a quantitative baseline for normal gait in terms of parameter ranges, so that deviations from the baseline could be identified as impairment or adaptation and proper treatment/rehabilitative therapies put in place. Adaptation may refer to any gait deviation that arises when an action is taken to mitigate the effects of an impairment. Table 3 provides gait parameter ranges as observed in studies on healthy adults. Determining an appropriate normal range for many of the features is highly challenging as individuals exhibit a wide range of gait patterns across different age groups and gender.
3.2. Stroke and Pathophysiology of Human Gait
Stroke patients may exhibit deficits in muscle strength and muscle tone, mobility, perception and motor-control, sensation, and balance (63, 64). This leads to significant changes in voluntary movement, thereby affecting gait patterns. Gait deviations in post-stroke patients are divided into (i) primary deviations, defined as those directly due to pathology, and (ii) secondary deviations, which are the result of the physical effects of the primary deviations (passive), or a compensatory mechanism (active).
Reduced gait speed and poor adaptation to daily-life tasks and environmental constraints are often observed (65) in persons with stroke. In sub-groups of stroke patients, functional adaptations include asymmetric steps, reduced weight bearing on the paretic limb, and reduced intra- and inter-limb coordination, leading to various compensatory adjustments including pelvic obliquity, hip hiking, and hip abduction with circumduction pattern to achieve foot clearance (66) (Figure 3). Kinematic deviations include reduced amplitude of the joint angle profiles at the lower extremities, whilst kinetic deviations include reduced joint moment and power. In addition, drop-foot is considered to be another major deficit following a stroke.
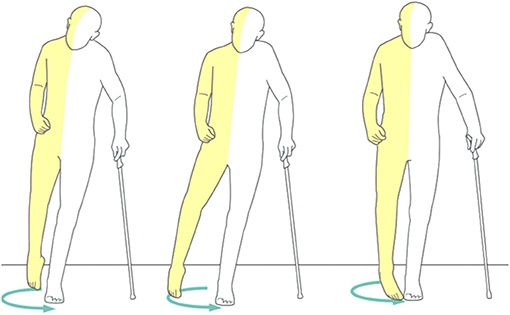
Figure 3. An example of uncorrected post-stroke spastic gait pattern (66).
3.3. Post-stroke Mobility
Independent mobility after stroke is key to intervention during the various stages of post-stroke. Mobility is defined as “the ability to move oneself (e.g., by walking, by using assistive devices, or by using transportation) within community environments that expand from one's home, to the neighborhood, and to regions beyond” (67). The assessment of functional mobility after stroke is very important as it determines the effectiveness of rehabilitation strategies.
A Timed Up & Go (TUG) task is a simple tool employed to assess improvements in functional mobility over time. A TUG task starts with a patient in a seated position in a chair. It calculates the time taken for the subject to stand up, walk 3 meters at a comfortable speed, turn around, walk back to the chair, and sit down. According to Persson et al., based on a study involving 91 patients with first-ever stroke during their 1st week, and at 3, 6, and 12 months post-stroke, the TUG time has reduced from 17 to 12 s between the 1st week and 3 months, with no statistically significant changes afterwards (68). Buvarp et al. investigated the longitudinal progression as well as the change in functional mobility between moderate and mild stroke (69). They reported improvement in functional mobility of moderate stroke group at 1 year post-stroke [patients with age <75 improved by 7.2 s in TUG time (P < 0.001), and patients with age ≥75 years improved by 5.8 s (P = 0.011)]. For the mild stroke group, no statistically significant improvement was reported.
Other methods that assess walking and balance of persons with stroke at the level of community walking include Step Test, Side-Step Test, and Four-Square Step Test that assess a single task, and Brunel Balance Assessment, Dynamic Gait Index, Modified Emory Functional Ambulation Profile, Community Balance and Mobility Scale, and mini-Balance Evaluation Systems Test that assess multiple tasks (70). Based on clinical utility, single-task measures may be recommended to use as screening tools or to identify basic components of walking and balance. On the other hand, multi-task measures enable a comprehensive evaluation of walking and balance and could be used for identifying mobility deficits and devising treatment strategies. Readers are directed to (70, 71) for a summary of the psychometric properties of these assessment techniques.
4. Tools and Techniques for post-Stroke Gait Characterization
Gait analysis typically involves measurement, quantification, assessment, and interpretation of parameters that characterize bipedal walking or gait. Measurement covers a range of techniques available for recording gait events. Quantification phase includes the extraction of the various biomechanical parameters of gait (spatiotemporal, kinematic, kinetic), while assessment is concerned with the application of analytical and computational techniques, and interpretation consists of inferring the implicit factors affecting the walking mechanism.
Considerable research efforts have been devoted to the measurement of gait parameters ranging from simple stop watches and measuring tapes, to emerging sophisticated hardware and software technologies including lightweight wearable sensors. This section will review both traditional and novel techniques applied for measuring gait characteristics as identified in the literature (see Table 4).
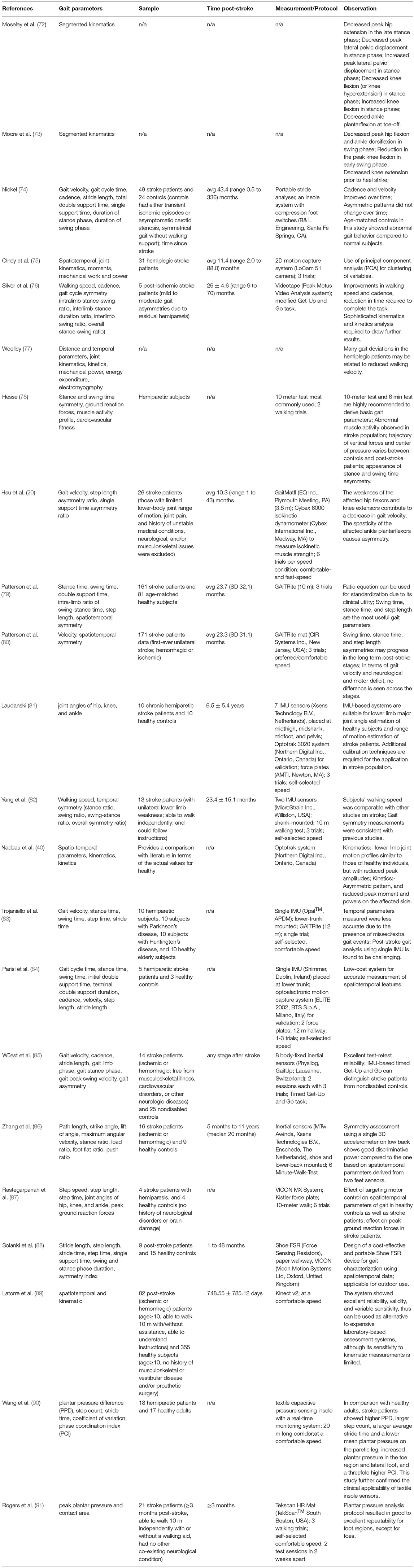
Table 4. An overview of the literature focusing on gait parameters and measurement devices for post-stroke gait studies considered in this review.
4.1. Conventional Approaches to Gait Characterization
The first documented experiments on gait analysis with major contribution to muscle and tendon biomechanics date back to the seventieth century conducted by Giovanni Alfonso Borelli (1,608–1,679) the Italian physiologist, physicist, and mathematician. Richard Baker (92) provides a comprehensive review of the history of gait analysis techniques before the emergence of modern-day computers. Rudimentary experiments involving only the use of stopwatches, measuring tapes, and basic telescopes were able to make significant contributions toward the quantitative study of gait.
Gait analysis generally includes the assessment of spatiotemporal parameters, such as gait velocity, step length, stride length, single-limb support time, double-limb support time, swing duration, and cadence by direct observation or from video tapes (93). These measures, as compared and discussed by McGinley et al. (93) have varied accuracy. Dynamic data includes both kinetic and kinematic data, such as vertical ground reaction forces, plantar pressure distribution, joint reaction forces, moments and power, as well as kinematic data describing joint angular motion, and EMG data reflecting muscle activity patterns.
In addition to qualitative observational/visual gait using the naked eye and/or video images to evaluate gait performance and functional improvement (Table 2), conventional post stroke gait measurement techniques include footprint methods to measure spatial parameters and stop watches for temporal features (94, 95). Although these techniques are simple, inexpensive, and relatively reliable, the subjectivity of measurement remains a major concern, particularly in the assessment of pathologic gait.
4.2. Technology-Driven Approaches to Gait Characterization
In the past two decades, the field of gait assessment and analysis has witnessed a remarkable technological advancement, particularly in gait assessment technology. Instrumented walkways, despite their relatively high cost, are now widely used, both in research and to a limited extent clinical practice (20, 79, 80, 83). These systems include low-profile floor walkway systems equipped with grids of embedded sensors below the surface, which record foot-strike patterns as a function of time and space as an individual walks across the platform, and dedicated software which computes the various spatiotemporal gait measures. Patterson et al. (79, 80) used a GAITRite mat (CIR Systems Inc., New Jersey, USA) to measure spatiotemporal data toward the evaluation of post-stroke gait symmetry. Trojaniello et al. (83) employed GAITRite as a reference system to measure temporal parameters to validate the findings from inertial sensors. A similar product, Walkway™(Tekscan Inc., South Boston, USA) offers dynamic plantar pressure in addition to spatiotemporal data. The company recently launched the Strideway™, a modular gait analysis platform featuring both spatiotemporal and plantar pressure assessment platforms (88). Such instrumented mats involve less setup time and are generally simple to operate. However, they require a specific operational environment and are restricted to trials involving over-ground tasks.
Marker-based optical motion capture (Mocap) systems are well recognized to be an effective technique for obtaining 3D kinematic movement data. Passive Mocap systems [e.g., Vicon (Vicon Motion Systems Ltd, Oxford, United Kingdom) and ELITE optoelectronic system (BTS S.p.A., Milano, Italy)], include retro-reflective markers (that reflect the light emitted by high-resolution infrared cameras) attached to specific anatomic landmarks. The location of the marker is identified by decoding the camera images. Here, the markers must be calibrated for identification before the recording session commences. Active systems (e.g., Optotrak motion capture system; Northern Digital Inc., Waterloo, Canada), on the other hand, use light-emitting diode (LED) markers (reflect their own light powered by a battery), which are automatically identified. Rastegarpanah et al. (87) used a Vicon system in conjunction with a Kistler force plate as part of their experimental study on a stroke population to investigate the significance of targeting effects on gait parameters including step speed and step length. Parisi et al. (84) employed the ELITE 2002 to acquire spatiotemporal data and compared it with IMU data as part of their validation study on both hemiparetic stroke patients and healthy controls. An Optotrak-based framework was proposed by Nadeau et al. (40) to investigate the kinematic changes in persons with post-stroke gait impairment. The patients were instructed to walk over-ground after being outfitted with markers, while the kinematic data was captured and processed by the system (40). Further, in (81), the Optotrak system was proposed as an efficient tool for capturing the ground truth data associated with lower-limb joint angles in a study involving chronic stroke patients. In contrast to the over-ground trials carried out in the earlier studies, this experimental work assessed gait during a typical stair ambulation task. Unlike walk mats, motion capture systems can be used for trials involving complex tasks, where analysis of motion in multiple planes is vital. In the context of clinical relevance, although such systems yield extremely accurate reliable data, operational factors including infrastructure, non-portability, high cost, additional time required for initial set-up and calibration, operational complexity, and restrictions to indoor setup impose hurdles to their functional deployment in clinics and rehabilitation centers (88). Therefore, more portable cost-effective alternatives, such as Microsoft Kinect became the application of choice (89). This system is based on a depth sensor-based markerless motion capture solution. Less expensive 2D motion capture systems (e.g., LoCam 51 camera), as well as video analysis systems (e.g., Peak Motus Video Analysis system), were also reported in the literature with acceptable resolution (75, 76).
Optoelectronic systems (e.g., Optogait®, Microgate, Italy) have also been used to capture spatiotemporal gait parameters. These mainly consist of a transmitting and a receiving bar containing an infrared light. Interruptions of the communication between the emitter and receiver are detected by the system to calculate the various gait parameters. Iosa et al. (96) used an Optogait system to compute the spatiotemporal parameters, including walking speed, stride duration, stance phase, swing phase, and double support phase in a study involving patients with subacute stroke, as well as healthy subjects.
Various techniques are available to measure kinetic parameters, including ground reaction forces, and joint moments and powers. Instrumented walkways offer dynamic pressure mapping but are expensive. Force plates are also used in various gait analysis studies (81, 84, 87, 97). Chen et al. (98) developed a novel remote sensing technology called “Electrostatic Field Sensing (EFS)” for measuring human gait including stepping, walking, and running, and further extended the work to post-stroke gait. This technology is credited with several advantages, such as being non-contact, affordable, and allows long-time monitoring (99).
Shoe insole systems represent another category of gait quantification tools and techniques. These systems are designed to allow for the recording of both dynamic plantar pressure and spatiotemporal data. F-scan (Tekscan Inc., South Boston, USA) is an ultra-thin in-shoe pressure measurement system utilizing Force-Sensitive Resistive films (FSR) technology (100). Focusing on portability, cost-effectiveness, and applicability to outdoor setting, Solanki and Lahiri (88) developed FSR-based shoes (ShoesFSR) that offered detailed gait characterization including abnormal gait, such as observed in post-stroke. In this design, two FSR sensors were placed at the heel region spaced at 30 mm apart, and one at the toe. Maintaining the complexity of the sensing circuit to the minimum, this system successfully extracted various spatiotemporal features, including stride length and stride time by detecting gait events, such as heel strike, heel-off, toe strike, and toe-off. A recent study identified that textile capacitive pressure sensing insole (TCPSI) is a promising tool for characterizing post-stroke gait patterns (90). Several gait parameters, including plantar pressure difference (PPD), step count, stride time, coefficient of variation, and phase coordination index (PCI) were evaluated, and results were compared with normal gait. The results confirmed that textile wearable sensors may be used as a gait evaluation tool, external feedback gait training device, and a compact gait analyzer for both stroke patients and healthy subjects.
More recently, wearable sensor-based gait analysis has become popular due to its ultra-small sensor size and low cost. Miniature sensors can be attached directly to body segments, and recording can be done without the need for a sophisticated laboratory environment. These systems have potential applications in both research laboratories and clinical settings and are either based on individual sensor fusion elements (accelerometers, gyroscopes, force/pressure sensors, EMG, inclinometers, goniometers), or combined together as an integrated IMU to measure gait characteristics (99, 101–103). IMU technology successfully integrates accelerometers, gyroscopes, and magnetometers within a single unit, and can estimate the spatiotemporal and kinematic parameters from the recording of acceleration forces, angular velocity, and magnetic field data (52). The technology has also been found to be useful for the analysis of pathological gait, such as post-stroke gait. Laudanski et al. (81) measured the range of motion of the lower limb joints of individuals with post-stroke gait impairment using seven IMU sensors placed at different body segments. He suggested adopting new improved calibration techniques applicable for IMU protocol to further extend this technology to a stroke population. Trojaniello et al. (83) tested the performance of a single IMU mounted at the lower trunk to measure temporal gait parameters and found that the use of a single IMU for abnormal gait characterization is challenging, as it resulted in less accurate measurements. On the other hand, Parisi et al. (84) used a single IMU for successfully estimating both spatial and temporal gait data. Further studies have reported IMU-based gait analysis and the discriminative power of the spatiotemporal parameters obtained (82, 85, 86, 104). The pros and cons, as well as the current manufacturers of the instruments are given in Table 5.
4.3. Gait Characterization in Clinics
The clinical application of quantitative gait assessment continues to encounter a number of barriers, including the cost of equipment, installation and infrastructure, difficulties in structured multifactorial gait assessment and interpreting a vast amount of complex gait data, low organizational support, lack of knowledge and training, and reliability and validity of the tools (105).
To overcome these limitations, researchers have proposed various low-cost gait analysis protocols with application to clinical practices (106, 107). This includes the use of commercially available technologies to develop clinically-relevant gait analysis systems with accepted levels of accuracy. On the other hand, novel wearable technology, such as inertial measurement units, are found to be cost-effective for clinical use and combined with AI-based techniques, evaluation and interpretation of the acquired gait parameters can become accessible to non-experts (103, 108). In (103), Caldas et al. presented a systematic review of gait analysis methods that use inertial sensors and adaptive algorithms. This paper has highlighted that various gait kinematic features could be acquired reliably using IMUs, but more work is needed to standardize the evaluation and report the results. Although the current research suggests promising results with regards to IMU-based gait analysis integrated with AI techniques, further assessment is needed prior to clinical implementation. As reported by Wikström et al. (108), the most widely adopted techniques include classification methods, dimensionality reduction, clustering, and expert systems. It is anticipated that these forthcoming assessment/evaluation tools, and technologies will improve clinical decision-making process and enable clinicians to devise specialized rehabilitation strategies that will be available to use in clinical settings and economical to implement. Although the value of innovation in clinical gait assessment, including post stroke, promises a paradigm shift toward data-driven precision/personalized rehabilitation, the various logistical challenges including clinical infrastructure and training, as well as the identification of target patients remain issues open for discussion.
5. Post-Stroke Gait Data Processing and Analysis Techniques
This section reviews both traditional and technology-driven methods of data processing and analysis protocols adopted by various researchers in the context of gait analysis. Technology driven approaches into gait characterization provide a vast amount of data that cannot be simply interpreted in clinical practice. Therefore, in addition to the measurement technology, we review data-mining technology, that allows vast amount of data to be interpreted in a meaningful time-frame for the use by the clinicians during the rehabilitation.
5.1. Conventional Approaches to Gait Data Processing and Analysis
Numerous computational techniques, including traditional statistical tests have been applied to analyzing quantitative gait data obtained from instrumented gait recording technology (79, 80, 109–115). For example, Lin et al. (110) employed stepwise regression analysis to identify important impairments in persons with stroke (muscle strength of plantarflexors and dorsiflexors, spasticity index, passive stiffness of plantarflexors, and position error) associated with gait parameters, and Pearson correlation coefficients to study the relationship between the gait parameters (i.e., gait velocity and spatiotemporal symmetry) and impairment parameters. Cruz et al. (113) based their analyses on multiple linear regression models. ANOVA tests were used to determine the variability between different stages of post-stroke, as well as the significant effect between variables within the same group (80). Another study used an unpaired t-test to compare stroke patients with controls, and further adopted correlation tests to examine the relationship between different symmetry measures, including symmetry ratio and symmetry index (79). Further, stepwise linear regression, as well as descriptive statistics (mean and standard deviation) and correlation tests were adopted to study the behavior of the hip and ankle joints during the swing phase of the gait cycle in individuals with post-stroke gait impairment (116). In (83), the difference between mean absolute values of initial contact timings associated with different gait event detection methods was calculated using the Wilcoxon signed-rank test. This method was further applied to examine the difference between the gait characteristics for affected and unaffected limbs. In addition, the Wilcoxon rank-sum and Friedman tests were also applied in the same work. Although such methods involve less computational cost, they often fail to incorporate a variety of high-dimensional inter-related data obtained from different devices requiring more complex statistical analysis methodology (108).
5.2. Technology-Driven Approaches to Gait Data Processing and Analysis
The field of big data provides powerful techniques to systematically analyze and extract information from instrumented gait data that are too large, heterogeneous, and/or complex to deal with traditional statistical approaches. Machine learning techniques, including predictive analytics and data mining techniques, have been incorporated to characterize both normal and pathological gait. Predictive analytics uses data modeling to understand trends in data to predict future outcomes (e.g., SVM). Data mining approach, on the other hand, helps to discover new patterns in data (e.g., clustering) (117).
Principal Components Analysis (PCA)-based techniques have widely been used as part of gait data analysis. Olney et al. (75) implemented PCA for dimensionality reduction, (118) applied the same technique on the kinematic data obtained from 27 stroke patients, and (119) employed Functional PCA (FPCA) to understand the relationship between velocity and functional assessment scales.
Several attempts have been made to classify the patterns of hemiplegic gait, particularly toward planning dedicated rehabilitation strategies. Most recently, the applicability of artificial intelligence has been explored. The most common adopted machine learning classifiers include Support Vector Machines (SVM) and Artificial Neural Networks (ANN). Lau et al. (44) implemented SVM, ANN, and Radial Basis Function neural networks (RBF) classifiers to classify five different walking conditions (level ground, stair ascent, stair descent, upslope, and downslope) for hemiparetic patients. The SVM based classifier outperformed ANN and RBF methods with a highest overall classification accuracy of 97.5%. Kaczmarczyk et al. (120) applied ANN for classifying post-stroke gait patterns.
Clustering analysis has also been used to classify the gait patterns of stroke patients by employing spatiotemporal and kinematic parameters as input features. The gait velocity, peak knee extension during mid stance, and peak dorsiflexion during swing phases were identified as key features that best discriminated the groups (121). A similar approach was used by (122) for post-stroke gait classification. Another study proposed a feature extraction algorithm for plantar pressure images obtained from stroke population (123).
6. Post-Stroke Gait Parameters
This section will review various gait and physiological parameters used to characterize post-stroke gait.
6.1. Spatiotemporal Parameters
Spatial and temporal parameters of post-stroke gait are significantly different from those of healthy individuals. Von Schroeder et al. in 1995 conducted a study on 49 ambulatory stroke patients and 24 controls to evaluate the changes in the spatiotemporal parameters and motion patterns (74). The patients who had no hemiplegia and those with bilateral symptoms were analyzed separately. The controls included in the study had either transient ischemic episodes or asymptomatic carotid stenosis but maintained symmetrical gait without walking support. Both comprehensive (gait velocity, cadence, stride length, gait cycle, total double support duration) and unilateral (single support duration, duration of swing phase, duration of stance phase) parameters of gait were analyzed. Compared to age-matched controls and non- disabled individuals, post-stroke patients exhibited decreased walking speed and cadence, as well as increased gait cycle and double limb support. It was also observed that the hemiplegic limb of patients spent more time in swing and stance, while their unaffected limb spent more time in stance and single support as compared to the controls. In addition, less time was spent in stance and more time in the swing phase on the paretic limb as compared to the contralateral side. However, the general gait parameters were observed to improve over time, with the exception of the asymmetrical patterns which did not change. Table 4 reports on various gait parameters applicable to post-stroke gait analysis as well as their significance.
In an attempt to recommend the most suitable gait measures for standardization, Patterson et al. (79) compared different asymmetry measures that describe post-stroke gait, including the symmetry ratio, symmetry index, log transformation of the symmetry ratio, and symmetry angle. The study recruited 161 unilateral stroke patients and 81 healthy controls. They found that no particular individual measure distinguished stroke patients better than others. However, they suggested symmetry ratio as a suitable measure based on its clinical utility. The most useful parameters of gait included step length, swing time, and stance time. Measures of gait speed and symmetry across the post-stroke stages also reflected both spatial and temporal symmetry deterioration in the later phases of post-stroke, whereas velocity, neurological impairment, and motor deficit did not change (80). These findings are in line with (124) but contradict (74), who found an improvement of gait velocity and cadence, especially during the first 12 months following a stroke, with no changes observed in asymmetry patterns. These contradictory findings may be due to selection bias and sample size variability that can affect the results in such studies. Although not explicitly reported in these papers, compensation strategies may have played a role in improving speed without enhancing symmetry.
A study on gait velocity and temporal symmetry (temporal stance ratio, temporal swing ratio, temporal swing-stance ratio, overall temporal symmetry ratio) involving 13 stroke patients with unilateral lower-limb weakness reported reduced gait velocity during a standardized 10-meter walk test as well as deterioration in temporal asymmetry, comparable with other related studies on individuals with post-stroke gait impairment (82). It has been reported that difference in spatiotemporal parameters could be influenced by gait speed, suggesting speed-matched trials for better post-stroke gait characterization. Consistent differences in the spatiotemporal parameters were noticed between stroke patients and healthy controls at speed-matched assessment (125, 126).
In summary, the literature agrees that the spatiotemporal characteristics of post-stroke gait include reduced step or stride length, increased step length on the hemiparetic side, slightly wider base of support, slightly greater toe-out angle, reduced walking speed and cadence. Stride time, stance period on both lower limb, and double support time are increased. In addition, less time in stance and more time in swing phase for the paretic side, as well as asymmetries in spatial and temporal factors have been reported (77).
Silver et al. assessed the improvement in the functional aspects of gait in patients with chronic hemiparesis after undergoing task-oriented aerobic training (76). The temporal parameters, including walking speed, cadence, gait symmetry (intralimb stance-swing ratio, interlimb stance duration ratio, interlimb swing ratio, overall stance-swing ratio) were examined before and after training using a modified TUG task. The post-ischemic stroke patients in this study exhibited mild to moderate gait asymmetry. A significant improvement in the walking speed and cadence, as well as a reduction in the overall time required to complete the TUG task were evident with time. However, improvements in gait asymmetry and temporal sequencing remained not significant. On the other hand, the gait patterns of chronic hemiparetic stroke patients were compared between over ground and treadmill walking by analyzing relative stance time, relative single-limb stance time, stance-swing ratio, peak force, and impulse (127). It has been observed that self-supported treadmill walking improved gait symmetry in hemiparetic stroke patients.
6.2. Kinematic Parameters
Available evidence indicates that changes in the stance phase of hemiplegic gait are attributed to reduced mean peak extension of the hip joint in late stance, alterations in the lateral displacement of the pelvis and flexion of the knee, and decreased plantarflexion of the ankle at toe-off (72, 73). Further, hemiplegic culprits include a significant decrease in peak hip and knee flexion during the swing phase, reduced knee extension prior to initial contact, as well as decreased ankle dorsiflexion during swing. In general, the literature suggests that the joint motion profiles of hemiplegic patients are influenced by abnormal muscle activation patterns, muscle shortening (72, 73), and/or reduced walking speed (128). In some patients, it was noted that compensatory walking mechanisms may also lead to abnormal angular motion patterns, for example, knee hyperextension to compensate for stable weight support during forward propulsion (129).
6.3. Kinetic Parameters
Kinetic analysis of gait includes accounting for the ground reaction forces (GRF) and joint moments and powers. GRF refers to the forces exerted by the body on to the ground and are usually measured by force sensors embedded in a walking platform. Moments and powers are obtained via inverse dynamics by combining GRF measurements with kinematic data.
Post-stroke GRF patterns differ from those of healthy subjects exhibiting an asymmetric pattern, as well as decreased amplitudes of the joint moments and joint powers at the hip, knee, and ankle joints on the paretic side (40). Overall, three main types of vertical GRF patterns have been observed in individuals with post-stroke gait impairment (77, 130): (1) a force curve with two vertical peaks occurring at loading and push-off and an intermediate trough at mid-stance phase, similar to the one seen in healthy groups, (2) a relatively constant vertical force component during stance, and several irregular peaks, (3) a single vertical peak force during early stance, which gradually reduces to zero during the late stance phase. In addition, a high correlation between the GRF pattern and the foot contact pattern (heel-, flatfoot-, forefoot-initial contact) of stroke patients has also been observed (131). These kinetic patterns can be easily computed from wearable sensors as detailed in (132).
It has been consistently reported that in stroke patients, the net positive moment and power at the hip, knee, and ankle joints follow profiles that are similar to those of healthy individuals while walking at self-selected comfortable speed and low gait speed, although with reduced amplitude in both limbs, and smaller amplitude on the affected side as compared to the non-affected side (40). Moreover, the amplitude of several kinetic parameters (e.g., hip power bursts) was found positively scaled to gait velocity or other functional ability (e.g., plantarflexors strength) measures of stroke patients (65). Some studies have revealed the applicability of plantar pressure dynamics in understanding foot and ankle movement patterns (65, 133–135). Spatial and temporal distribution of foot plantar pressure and the displacement of the Center of Pressure (CoP) are significant findings in this context. Several abnormalities, including lower plantar pressure peaks for the paretic foot as compared to the non-paretic foot (133), early paretic forefoot contact (134), asymmetries in the spatial and temporal distribution of plantar pressure along the plantar surface of the foot between affected and unaffected limbs, as well as the variability of the CoP, have been reported in individuals with post-stroke gait impairment (65, 134, 135). A recent study (91) addressed the repeatability of plantar pressure assessment in stroke patients and claimed good to excellent repeatability for the foot regions with exception to toes.
6.4. Electromyographic (EMG) Parameters
Surface EMG is a noninvasive technique used to capture muscle activity, which could provide further insight into post-stroke gait abnormalities since during walking EMG data reveals characteristic patterns of neural activation associated with each involved muscle in terms of onset timings, burst durations, and levels of activations (136). However, EMG-based assessment for gait asymmetry is not well explored as compared to assessment using other typical gait attributes. Similar to other gait parameters discussed above, EMG signals from stroke patients reveal abnormal amplitude and timing as compared to healthy controls. Flexor and extensor mechanisms seem to contribute to such abnormal behavior (137). Hesse (78) reported the most common EMG abnormalities including high tonic activity of the tibialis anterior, early onset of the gastrocnemius, delayed onset of the vastus lateralis, and highly paretic gluteus medius muscle activity at gait onset.
6.5. Additional Parameters
Autonomic dysfunction is another common complication after stroke, which inspired investigations regarding the role of Heart Rate Variability (HRV) (138, 139). Korpelainen et al. showed that HRV changes were present in patients with hemispheric and medullary unilateral brainstem stroke (138). Similarly, recovery of parasympathetic function was observed from the third day after the onset of stroke, while both parasympathetic and sympathetic functions recovered by the seventh day, depending on the extent and laterality (midline versus lateral) of stroke. It is therefore recommended that autonomic function be taken into account during the first week after stroke to prevent cardiac complications, such as postural hypotension during physical therapy (139). Another study explored the significance of HRV in predicting post stroke motor recovery and revealed a strong positive correlation between HRV and the movement of the affected extremities (140). Short-term and long-term variability of heart rate analysis of post-stroke patients also reported changes in HRV associated with impaired renal function which correlated with stroke severity.
Among other neurocontrol parameters, toe clearance (109, 141), foot clearance (142), claw toes (143), and mechanical energy cost (65), in association with post-stroke gait characteristics, were also reported to be useful in improving treatment outcomes and monitoring post-stroke rehabilitation. Particularly, Begg et al. (141) provided evidence that biofeedback training based on minimum toe clearance was useful in reducing tripping risk of post-stroke patients.
Analysis of additional biomechanical parameters suggested that multi-joint abnormalities (e.g., reduced flexion at knee and ankle) (142) contributed to the lower foot clearance in post-stroke individuals. In (143), 46% of 39 hemiplegic patients exhibited claw toe mostly before the end of the third month post-stroke. Claw toe was significantly correlated with equinus and/or varus foot. In addition, the mechanical energy cost was found be higher in post-stroke patients as compared to healthy subjects at similar walking speed (65).
7. Gait Analysis and Artificial Intelligence
Expert Systems (ES) represent early applications of analytical tools in Artificial Intelligence (AI). They consist of a combination of a database with a knowledge base, as well as rules including logic operators that utilize probability theory to provide possible outcomes as part of a user interface (144, 145). Expert systems initially require users to define variables and appropriate diagnostic values, as well as associated rules, confidence factors, and user specified questions. The expert system then generates a set of questions that guide the user toward a systematic diagnosis or evaluation (146). An important feature of ES is that functional evaluation, which is based on clinical observations, can be standardized by incorporating a set process. ES have been applied in numerous disciplines including general biomechanics, sports biome- chanics, orthopedics, and gait analysis for improving movement techniques, diagnostics, rehabilitation, and treatment. By far the most common use is in gait analysis (147). Early studies included automated detection of gait events using inductive learning (148) and an integrated gait analysis framework (149, 150). Further advances in the use of ES came with the advent of multi-sensor technology and analytics including video capture of movement with reflective sensors placed on the body, force plate derived features and physiological/neuromuscular features (151). Use of more ANN-based classification superseded ES with AI demonstrating better accuracy as compared to statistical expert systems models. ANN consists of a series of interconnected nodes that can be either designed as a single layer or multilayer approximating the relationships, or adaptive weightings determined from a training set, between input and output measures, which can then be applied to unseen data (150). Lugade and colleagues applied self-organizing maps (SOM) or so-called Kohonen maps to estimate gait balance control in the elderly using clinical evaluations (152). ANNs also include feedforward or feedbackward backpropagation neural networks (153). Further applications in gait included estimating joint kinetics and kinematics using electromyography and determining spatiotemporal gait patterns from EMG recordings to determine falls risk or control measures for retaining balance during gait (154–156).
As the volume of data from the variety of nowadays readily available body sensors used to quantify human gait and movement, including electroencephalography, electro-oculography, electro-cardiography, and electromyography video and force plate data, substantially increases, more sophisticated modeling is needed to quantify and interpret complex network physiology (157). In addition, today's advanced use of computer science has established novel features describing gait movement associated biomechanics, moving from discrete data to more realistic dynamic representations (158, 159). Multivariate statistical analysis, machine learning methods including Support Vector Machines (SVM) have been recently extended to Deep Artificial Neural Networks such as Layer-wise Relevance Propagation (LRP) methods to provide numerical data on the contributions of variables included in the model (160).
There remains a gap in literature regarding advanced neural networks that deal with gait individualized pattern characteristics. Since those are unique for each individual, they require models that not only include conventional features based on training static features of a sample population, such as maximum joint angles, but also the inclusion of dynamic variables such as the change of joint angle over time in conjunction with sophisticated data reduction and individualized feature selection of the most relevant gait characteristics in the context of personalized medicine (161). Applying new statistical learning algorithms, including deep learning combined with large data sets, has led to the emergence of Explainable Artificial Intelligence (XAI) designed to gain information about the individual features included in the final AI classification. However, this approach has had limited applications with clinicians who required decision support algorithms to provide information on causality as well (162). Table 6 provides an overview of the up-to-date AI techniques used in various literature resources.
8. Limitations
This review aimed to summarize available published work on the assessment, quantification, as well as analysis of gait dysfunction associated with post-stroke gait. The focus was to highlight recent technology-driven gait characterization and analysis approaches and their applicability to clinical practice toward data-driven informed treatment and precision rehabilitation strategies. As such, this article may have not covered the complete biomedical aspects of stroke assessment and/or rehabilitation. A non-systematic search methodology was selected in order to broaden the scope and integration of the three aspects of focus (assessment, quantification, and analysis). In addition, we do not recommend any specific protocol over the other, as most of the papers incorporate different inclusion/exclusion criteria for subject selection, as well as different sampling sizes, which renders comparisons unrealistic.
9. Conclusion and Future Work
The main contribution of this work is providing a multidisciplinary comprehensive review on post stroke gait assessment and analysis toward bridging the gap between research gait studies and clinical applications. The main goal is to offer a practical resource on the multidimensional aspects of post stroke gait focusing on novel tools and technologies for quantitative assessment that can be feasibly incorporated into clinical practice.
As generally agreed upon by many clinicians, quantitative gait analysis outperforms traditional observational scales as it generates unbiased outcomes that can be used as benchmarks for rehabilitation. Instrumented gait analysis has wide potential applications throughout the different phases post-stroke. For example, as a diagnostic tool to quantitatively assess the severity of a stroke and establish measurable benchmark parameters toward devising patient-specific rehabilitation strategies. It can also be used as means for continuously evaluating recovery while ensuring patient safety and leveraging the plasticity of the brain constrained by time during the various stroke phases. A variety of gait parameters, including spatiotemporal features, gait kinematics, kinetics, EMG patterns, toe and foot clearance, claw toes, mechanical energy cost, and HRV are available for consideration, where each signifies different aspects of motor and functional deficits associated with stroke that can be analyzed using a diverse menu of available statistical and analytical tools, including XAI. As can be observed from the literature, spatiotemporal symmetry and gait velocity are considered to be key parameters for post-stroke gait analysis. On the other hand, in recent sensor fusion approaches, multiple parameters are simultaneously assessed to reflect and evaluate the inherent complexity and variability of gait, including various physiological phenomena, such as EMG, ECG and EEG, in addition to gait. Future work is needed to recommend the best set of parameters for post-stroke gait assessment. This review also summarizes the variety of current measurement devices and tools available for acquiring gait data. The latest trend is toward smart wearable technology, which promises a paradigm shift in clinical gait assessment, including stroke, and the creation of low cost, portable, gait labs that can be transferred to the clinic for accurate and reliable dynamic gait assessment. Data analyses techniques help to understand the underlying information associated with these parameters. In a clinical context, it would be invaluable if such algorithms can predict post-stroke recovery status and time, although this still seems to be a highly challenging task. AI/NN models that include static and dynamic features, combined with sophisticated data reduction and individualized feature selection of the most relevant gait characteristics are needed to close the loop for this paradigm shift in alignment with personalized post stroke assessment and rehabilitation.
Researchers and clinicians may consider looking into the following key points:
1. Quantitative gait analysis is considered beneficial over the conventional techniques during various stages of post-stroke gait quantification, assessment, and analysis.
2. A combination of gait and physiological markers should be employed while analyzing pathological gait, such as post-stroke gait due to the complexity and variability of gait patterns involved.
3. Data mining, non-linear and AI techniques could be suitable options for post-stroke gait data analysis.
The authors are currently working on devising methods for identifying the most significant gait parameters for clinical use, cost and time-effective protocols for measuring the parameters in clinical settings, and the most effective techniques for data mining and analyses with the support of data from stroke patients. The relevant questions of the need for innovation in clinical post stroke gait assessment and identification of target patients remain open for debate. Further clinical applications of the above reviewed tools and technologies are needed to demonstrate the overall efficacy of this paradigm shift in comparison to conventional assessment methodologies.
Author Contributions
DMM and KK conceived the idea. DMM, KK, AHK, and HFJ formulated the objective for this review. DMM designed the search strategy, conducted abstract screening and full text review, extracted the data, and drafted the manuscript. KK, HFJ, and AHK contributed to writing the manuscript. SIIIA performed a part of the literature survey, including abstract screening, full text review, and data extraction. AHK, SAW, HFJ, and KK provided significant guidance on the content of the manuscript, overall supervision, and critical feedback. All authors contributed to the manuscript revision and approved the final version of the manuscript.
Funding
This publication is based upon work supported by the Khalifa University of Science and Technology under Award No. RC2-2018-022.
Conflict of Interest
The authors declare that the research was conducted in the absence of any commercial or financial relationships that could be construed as a potential conflict of interest.
References
1. Mackay J, Mensah GA. The Atlas of Heart Disease and Stroke. Geneva: World Health Organization (2004).
2. Johnston SC, Mendis S, Mathers CD. Global variation in stroke burden and mortality: estimates from monitoring, surveillance, and modelling. Lancet Neurol. (2009) 8:345–54. doi: 10.1016/S1474-4422(09)70023-7
3. Zain A. Every Hour One Person Gets a Stroke in UAE-Khaleej Times (2019). Available online at: https://www.khaleejtimes.com/news/uae-health/one-in-every-hour-gets-stroke-in-uae (Accessed March 16, 2019).
4. Lyden PD, Hantson L. Assessment scales for the evaluation of stroke patients. J Stroke Cerebrovasc Dis. (1998) 7:113–27. doi: 10.1016/S1052-3057(98)80138-9
5. Harrison JK, McArthur KS, Quinn TJ. Assessment scales in stroke: clinimetric and clinical considerations. Clin Intervent Aging (2013) 8:201. doi: 10.2147/CIA.S32405
7. Hunt WE, Hess RM. Surgical risk as related to time of intervention in the repair of intracranial aneurysms. J Neurosur. (1968) 28:14–20. doi: 10.3171/jns.1968.28.1.0014
9. Teasdale G, Jennett B. Assessment of coma and impaired consciousness: a practical scale. Lancet (1974) 304:81–4. doi: 10.1016/S0140-6736(74)91639-0
10. Gotoh F, Terayama Y, Amano T, of the Japan Stroke Society SSC. Development of a novel, weighted, quantifiable stroke scale: Japan stroke scale. Stroke (2001) 32:1800–7. doi: 10.1161/01.STR.32.8.1800
11. Côté R, Hachinski V, Shurvell B, Norris J, Wolfson C. The canadian neurological scale: a preliminary study in acute stroke. Stroke (1986) 17:731–7. doi: 10.1161/01.STR.17.4.73
12. Adams RJ, Meador KJ, Sethi KD, Grotta JC, Thomson DS. Graded neurologic scale for use in acute hemispheric stroke treatment protocols. Stroke (1987) 18:665–9. doi: 10.1161/01.STR.18.3.665
13. Jørgensen HS, Nakayama H, Raaschou HO, Vive-Larsen J, Støier M, Olsen TS. Outcome and time course of recovery in stroke. Part I: outcome. the copenhagen stroke study. Arch Phys Med Rehabil. (1995) 76:399–405. doi: 10.1016/S0003-9993(95)80567-2
14. Olesen J, Simonsen K, Nørgaard B, Grønbæk M, Johansen OS, Krogsgaard A, et al. Reproducibility and utility of a simple neurological scoring system for stroke patients (Copenhagen Stroke Scale). J Neurol Rehabil. (1988) 2:59–63. doi: 10.1177/136140968800200203
15. Sun Z, Yue Y, Leung C, Chan M, Gelb A, for Perioperative Stroke In China (POSIC) SG, et al. Clinical diagnostic tools for screening of perioperative stroke in general surgery: a systematic review. BJA Br J Anaest. (2016) 116:328–38. doi: 10.1093/bja/aev452
16. Hantson L, De Weerdt W, De Keyser J, Diener H, Franke C, Palm R, et al. The european stroke scale. Stroke (1994) 25:2215–9. doi: 10.1161/01.STR.25.11.2215
17. Bohannon R. Gait performance of hemiparetic stroke patients: selected variables. Arch Phys Med Rehabil. (1987) 68, 777–81.
18. Eng JJ, Chu KS. Reliability and comparison of weight-bearing ability during standing tasks for individuals with chronic stroke. Arch Phys Med Rehabil. (2002) 83:1138–44. doi: 10.1053/apmr.2002.33644
19. Eng JJ, Tang PF. Gait training strategies to optimize walking ability in people with stroke: a synthesis of the evidence. Exp Rev Neurother (2007) 7:1417–36. doi: 10.1586/14737175.7.10.1417
20. Hsu AL, Tang PF, Jan MH. Analysis of impairments influencing gait velocity and asymmetry of hemiplegic patients after mild to moderate stroke. Arch Phys Med Rehabil. (2003) 84:1185–93. doi: 10.1016/S0003-9993(03)00030-3
21. Kim CM, Eng JJ. The relationship of lower-extremity muscle torque to locomotor performance in people with stroke. Phys Therapy (2003) 83:49–57. doi: 10.1093/ptj/83.1.49
22. Nadeau S, Arsenault AB, Gravel D, Bourbonnais D. Analysis of the clinical factors determining natural and maximal gait speeds in adults with A Stroke1. Am J Phys Med Rehabil. (1999) 78:123–30. doi: 10.1097/00002060-199903000-00007
23. Eng JJ, Chu KS, Dawson AS, Kim CM, Hepburn KE. Functional walk tests in individuals with stroke: relation to perceived exertion and myocardial exertion. Stroke (2002) 33:756–61. doi: 10.1161/hs0302.104195
24. Lamontagne A, Malouin F, Richards CL, et al. Contribution of passive stiffness to ankle plantarflexor moment during gait after stroke. Arch Phys Med Rehabil. (2000) 81:351–8. doi: 10.1016/S0003-9993(00)90083-2
25. Keenan MA, Perry J, Jordan C. Factors affecting balance and ambulation following stroke. Clin Orthop Relat Res. (1984) 182:165–71. doi: 10.1097/00003086-198401000-00021
26. Dettmann MA, Linder MT, Sepic SB. Relationships among walking performance, postural stability, and functional assessments of the hemiplegic patient. Am J Phys Med. (1987) 66:77–90.
27. Tang A, Sibley KM, Bayley MT, McIlroy WE, Brooks D. Do functional walk tests reflect cardiorespiratory fitness in sub-acute stroke? J Neuroeng Rehabil. (2006) 3:1–8. doi: 10.1186/1743-0003-3-23
28. Daly J, Nethery J, McCabe J, Brenner I, Rogers J, Gansen J, et al. Development and testing of the Gait Assessment and Intervention Tool (GAIT): a measure of coordinated gait components. J Neurosci Methods (2009) 178, 334–9. doi: 10.1016/j.jneumeth.2008.12.016
29. Goodkin R, Diller L. Reliability among physical therapists in diagnosis and treatment of gait deviations in hemiplegics. Percept Motor Skills (1973) 37:727–34. doi: 10.1177/003151257303700311
30. Hughes KA, Bell F. Visual assessment of hemiplegic gait following stroke: pilot study. Arch Phys Med Rehabil. (1994) 75:1100–7. doi: 10.1016/0003-9993(94)90085-X
31. Lord S, Halligan P, Wade D. Visual gait analysis: the development of a clinical assessment and scale. Clin Rehabil. (1998) 12:107–19. doi: 10.1191/026921598666182531
32. Estrada-Barranco C, Cano-de-la Cuerda R, Molina-Rueda F. Construct validity of the wisconsin gait scale in acute, subacute and chronic stroke. Gait Posture (2019) 68:363–8. doi: 10.1016/j.gaitpost.2018.12.020
33. Rodriquez AA, Black PO, Kile KA, Sherman J, Stellberg B, McCormick J, et al. Gait training efficacy using a home-based practice model in chronic hemiplegia. Arch Phys Med Rehabil. (1996) 77:801–5. doi: 10.1016/S0003-9993(96)90260-9
34. Zimbelman J, Daly JJ, Roenigk KL, Butler K, Burdsall R, Holcomb JP. Capability of 2 gait measures for detecting response to gait training in stroke survivors: gait assessment and intervention tool and the tinetti gait scale. Arch Phys Med Rehabil. (2012) 93:129–36. doi: 10.1016/j.apmr.2011.08.011
35. VanSwearingen JM, Paschal KA, Bonino P, Yang JF. The modified gait abnormality rating scale for recognizing the risk of recurrent falls in community-dwelling elderly adults. Phys Therapy (1996;) 6:994–1002. doi: 10.1093/ptj/76.9.994
36. Toro B, Nester C, Farren P. A review of observational gait assessment in clinical practice. Physiother Theory Pract. (2003) 19:137–49. doi: 10.1080/09593980307964
37. Wallmann HW. Introduction to observational gait analysis. Home Health Care Manag Pract. (2009) 22:66–8. doi: 10.1177/1084822309343277
38. Ferrarello F, Bianchi VAM, Baccini M, Rubbieri G, Mossello E, Cavallini MC, et al. Tools for observational gait analysis in patients with stroke: a systematic review. Phys Therapy (2013) 93:1673–85. doi: 10.2522/ptj.20120344
39. Turani N, Kemiksizoğlu A, Karataş M, Özker R. Assessment of hemiplegic gait using the Wisconsin Gait Scale. Scand J Caring Sci. (2004) 18:103–8. doi: 10.1111/j.1471-6712.2004.00262.x
40. Nadeau S, Betschart M, Bethoux F. Gait analysis for poststroke rehabilitation: the relevance of biomechanical analysis and the impact of gait speed. Phys Med Rehabil Clin. (2013) 24:265–76. doi: 10.1016/j.pmr.2012.11.007
41. Wren TA, Gorton III GE, Ounpuu S, Tucker CA. Efficacy of clinical gait analysis: a systematic review. Gait Posture (2011) 34:149–53. doi: 10.1016/j.gaitpost.2011.03.027
42. Wren TA, Tucker CA, Rethlefsen SA, Gorton III GE, Õunpuu S. Clinical efficacy of instrumented gait analysis: systematic review 2020 update. Gait Posture (2020) 80:274–9. doi: 10.1016/j.gaitpost.2020.05.031
43. Lee H, Guan L, Burne JA. Human gait and posture analysis for diagnosing neurological disorders. In: Proceedings 2000 International Conference on Image Processing (Cat. No. 00CH37101). Vol. 2. Vancouver, BC: IEEE (2000). p. 435–8.
44. Lau Hy, Tong Ky, Zhu H. Support vector machine for classification of walking conditions of persons after stroke with dropped foot. Hum Mov Sci. (2009) 28:504–14. doi: 10.1016/j.humov.2008.12.003
45. Scheffer C, Cloete T. Inertial motion capture in conjunction with an artificial neural network can differentiate the gait patterns of hemiparetic stroke patients compared with able-bodied counterparts. Comp Methods Biomech Biomed Eng. (2012) 15:285–94. doi: 10.1080/10255842.2010.527836
46. Zhou Y, Romijnders R, Hansen C, van Campen J, Maetzler W, Hortobágyi T, et al. The detection of age groups by dynamic gait outcomes using machine learning approaches. Sci Rep. (2020) 10:1–12. doi: 10.1038/s41598-020-61423-2
47. Iosa M, Capodaglio E, Pelà S, Persechino B, Morone G, Antonucci G, et al. Artificial Neural Network analyzing wearable device gait data for identifying patients with stroke unable to return to work. Front Neurol. (2021) 12:561. doi: 10.3389/fneur.2021.650542
48. Munn Z, Peters MD, Stern C, Tufanaru C, McArthur A, Aromataris E. Systematic review or scoping review? Guidance for authors when choosing between a systematic or scoping review approach. BMC Med Res Methodol. (2018) 18:1–7. doi: 10.1186/s12874-018-0611-x
49. Yeo SS. Changes of gait variability by the attention demanding task in elderly adults. J Korean Phys Therapy (2017) 29:303–6. doi: 10.18857/jkpt.2017.29.6.303
50. Perry J, Davids JR. Gait analysis: normal and pathological function. J Pediatr Orthop. (1992) 12:815. doi: 10.1097/01241398-199211000-00023
51. Winter DA. Biomechanics and Motor Control of Human Gait: Normal, Elderly and Pathological. Waterloo, IA: University of Waterloo Press (1991).
52. Tao W, Liu T, Zheng R, Feng H. Gait analysis using wearable sensors. Sensors (2012) 12:2255–83. doi: 10.3390/s120202255
53. Mills PM, Barrett RS. Swing phase mechanics of healthy young and elderly men. Hum Mov Sci. (2001) 20:427–46. doi: 10.1016/S0167-9457(01)00061-6
54. Auvinet B, Berrut G, Touzard C, Moutel L, Collet N, Chaleil D, et al. Reference data for normal subjects obtained with an accelerometric device. Gait Posture (2002) 16:124–34. doi: 10.1016/S0966-6362(01)00203-X
55. Al-Obaidi S, Wall JC, Al-Yaqoub A, Al-Ghanim M. Basic gait parameters: a comparison of reference data for normal subjects 20 to 29 years of age from Kuwait and Scandinavia. J Rehabil Res Dev. (2003) 40:361–6. doi: 10.1682/JRRD.2003.07.0361
56. Lai DT, Begg RK, Palaniswami M. Computational intelligence in gait research: a perspective on current applications and future challenges. IEEE Trans Inform Technol Biomed. (2009) 13:687–702. doi: 10.1109/TITB.2009.2022913
57. Senden R, Grimm B, Heyligers I, Savelberg H, Meijer K. Acceleration-based gait test for healthy subjects: reliability and reference data. Gait Posture (2009) 30:192–6. doi: 10.1016/j.gaitpost.2009.04.008
58. Bovi G, Rabuffetti M, Mazzoleni P, Ferrarin M. A multiple-task gait analysis approach: kinematic, kinetic and EMG reference data for healthy young and adult subjects. Gait Posture (2011) 33:6–13. doi: 10.1016/j.gaitpost.2010.08.009
59. Hollman JH, McDade EM, Petersen RC. Normative spatiotemporal gait parameters in older adults. Gait Posture (2011) 34:111–8. doi: 10.1016/j.gaitpost.2011.03.024
60. Schwesig R, Leuchte S, Fischer D, Ullmann R, Kluttig A. Inertial sensor based reference gait data for healthy subjects. Gait Posture (2011) 33:673–8. doi: 10.1016/j.gaitpost.2011.02.023
61. McKay MJ, Baldwin JN, Ferreira P, Simic M, Vanicek N, Wojciechowski E, et al. Spatiotemporal and plantar pressure patterns of 1000 healthy individuals aged 3–101 years. Gait Posture (2017) 58:78–87. doi: 10.1016/j.gaitpost.2017.07.004
62. Lau LK, Wee SL, Pang WJB, Chen KK, Jabbar KA, Yap PLK, et al. Reference values of gait speed and gait spatiotemporal parameters for a south east Asian population: the yishun study. Clin Interv Aging (2020) 15:1753. doi: 10.2147/CIA.S270407
63. Yavuzer G. Walking After Stroke: Interventions to Restore Normal Gait Pattern. Rotterdam: Erasmus University Rotterdam (2006).
64. Beyaert C, Vasa R, Frykberg GE. Gait post-stroke: pathophysiology and rehabilitation strategies. Neurophysiol Clin Clin Neurophysiol. (2015) 45:335–55. doi: 10.1016/j.neucli.2015.09.005
65. Lamontagne A, Stephenson JL, Fung J. Physiological evaluation of gait disturbances post stroke. Clin Neurophysiol. (2007) 118:717–29. doi: 10.1016/j.clinph.2006.12.013
66. Aqueveque P, Ortega P, Pino E, Saavedra F, Germany E, Gómez B. After stroke movement impairments: a review of current technologies for rehabilitation. In: Physical Disabilities-Therapeutic Implications. InTech; 2017. p. 95–116.
67. Webber SC, Porter MM, Menec VH. Mobility in older adults: a comprehensive framework. Gerontologist (2010) 50:443–50. doi: 10.1093/geront/gnq013
68. Persson CU, Danielsson A, Sunnerhagen KS, Grimby-Ekman A, Hansson PO. Timed Up & Go as a measure for longitudinal change in mobility after stroke–Postural Stroke Study in Gothenburg (POSTGOT). J Neuroeng Rehabil. (2014) 11:1–7. doi: 10.1186/1743-0003-11-83
69. Buvarp D, Rafsten L, Sunnerhagen KS. Predicting Longitudinal Progression in Functional Mobility After Stroke: A Prospective Cohort Study. Stroke (2020) 51:2179–87. doi: 10.1161/STROKEAHA.120.029913
70. Pollock C, Eng J, Garland S. Clinical measurement of walking balance in people post stroke: a systematic review. Clin Rehabil. (2011) 25:693–708. doi: 10.1177/0269215510397394
71. Balasubramanian CK, Clark DJ, Fox EJ. Walking adaptability after a stroke and its assessment in clinical settings. Stroke Res Treat. (2014) 2014. doi: 10.1155/2014/591013
72. Moseley A, Wales A, Herbert R, Schurr K, Moore S. Observation and analysis of hemiplegic gait: stance phase. Aust J Physiother. (1993) 39:259–67. doi: 10.1016/S0004-9514(14)60486-4
73. Moore S, Schurr K, Wales A, Moseley A, Herbert R. Observation and analysis of hemiplegic gait: swing phase. Aus J Physiother. (1993) 39:271–78. doi: 10.1016/S0004-9514(14)60487-6
74. Von Schroeder HP, Coutts RD, Lyden PD, Billings E, Nickel VL. Gait parameters following stroke: a practical assessment. J Rehabil Res Dev. (1995) 32:25–5.
75. Olney SJ, Griffin MP, McBride ID. Multivariate examination of data from gait analysis of persons with stroke. Phys Therapy. (1998) 78:814–28. doi: 10.1093/ptj/78.8.814
76. Silver KH, Macko RF, Forrester LW, Goldberg AP, Smith GV. Effects of aerobic treadmill training on gait velocity, cadence, and gait symmetry in chronic hemiparetic stroke: a preliminary report. Neurorehabil Neural Repair (2000) 14:65–71. doi: 10.1177/154596830001400108
77. Woolley SM. Characteristics of gait in hemiplegia. Top Stroke Rehabil. (2001) 7:1–18. doi: 10.1310/JB16-V04F-JAL5-H1UV
78. Hesse S. Rehabilitation of gait after stroke: evaluation, principles of therapy, novel treatment approaches, and assistive devices. Top Geriatr Rehabil. (2003) 19:109–26. doi: 10.1097/00013614-200304000-00005
79. Patterson KK, Gage WH, Brooks D, Black SE, McIlroy WE. Evaluation of gait symmetry after stroke: a comparison of current methods and recommendations for standardization. Gait Posture (2010) 31:241–6. doi: 10.1016/j.gaitpost.2009.10.014
80. Patterson KK, Gage WH, Brooks D, Black SE, McIlroy WE. Changes in gait symmetry and velocity after stroke: a cross-sectional study from weeks to years after stroke. Neurorehabil Neural Repair (2010) 24:783–90. doi: 10.1177/1545968310372091
81. Laudanski A, Brouwer B, Li Q. Measurement of lower limb joint kinematics using inertial sensors during stair ascent and descent in healthy older adults and stroke survivors. J Healthcare Eng. (2013) 4:555–76. doi: 10.1260/2040-2295.4.4.555
82. Yang S, Zhang JT, Novak AC, Brouwer B, Li Q. Estimation of spatio-temporal parameters for post-stroke hemiparetic gait using inertial sensors. Gait Posture (2013) 37:354–8. doi: 10.1016/j.gaitpost.2012.07.032
83. Trojaniello D, Ravaschio A, Hausdorff JM, Cereatti A. Comparative assessment of different methods for the estimation of gait temporal parameters using a single inertial sensor: application to elderly, post-stroke, Parkinson's disease and Huntington's disease subjects. Gait Posture (2015) 42:310–6. doi: 10.1016/j.gaitpost.2015.06.008
84. Parisi F, Ferrari G, Baricich A, D'Innocenzo M, Cisari C, Mauro A. Accurate gait analysis in post-stroke patients using a single inertial measurement unit, In: 2016 IEEE 13th International Conference on Wearable and Implantable Body Sensor Networks (BSN). San Francisco, CA: IEEE (2016). p. 335–40.
85. Wüest S, Masse F, Aminian K, Gonzenbach R, De Bruin ED. Reliability and validity of the inertial sensor-based Timed Up and Go test in individuals affected by stroke. J Rehabil Res Dev. (2016) 53:599–610. doi: 10.1682/JRRD.2015.04.0065
86. Zhang W, Smuck M, Legault C, Ith M, Muaremi A, Aminian K. Gait symmetry assessment with a low back 3d accelerometer in post-stroke patients. Sensors (2018) 18:3322. doi: 10.3390/s18103322
87. Rastegarpanah A, Scone T, Saadat M, Rastegarpanah M, Taylor SJ, Sadeghein N. Targeting effect on gait parameters in healthy individuals and post-stroke hemiparetic individuals. J Rehabil Assi Technol Eng. (2018) 5:2055668318766710. doi: 10.1177/2055668318766710
88. Solanki D, Lahiri U. Design of instrumented shoes for gait characterization: a usability study with healthy and post-stroke hemiplegic individuals. Front Neurosci. (2018) 12:459. doi: 10.3389/fnins.2018.00459
89. Latorre J, Colomer C, Alcañiz M, Llorens R. Gait analysis with the Kinect v2: normative study with healthy individuals and comprehensive study of its sensitivity, validity, and reliability in individuals with stroke. J Neuroeng Rehabil. (2019) 16:97. doi: 10.1186/s12984-019-0568-y
90. Wang C, Kim Y, Shin H, Min SD. Preliminary clinical application of textile insole sensor for hemiparetic gait pattern analysis. Sensors (2019) 19:3950. doi: 10.3390/s19183950
91. Rogers A, Morrison SC, Gorst T, Paton J, Freeman J, Marsden J, et al. Repeatability of plantar pressure assessment during barefoot walking in people with stroke. J Foot Ankle Res. (2020) 13:1–7. doi: 10.1186/s13047-020-00407-x
92. Baker R. The history of gait analysis before the advent of modern computers. Gait Posture (2007) 26:331–42. doi: 10.1016/j.gaitpost.2006.10.014
93. McGinley JL, Goldie PA, Greenwood KM, Olney SJ. Accuracy and reliability of observational gait analysis data: judgments of push-off in gait after stroke. Phys Therapy (2003) 83:146–60. doi: 10.1093/ptj/83.2.146
94. Zverev Y. Spatial asymmetry of post-stroke hemiparetic gait: assessment and recommendations for physical rehabilitation. Tanzania J Health Res. (2015) 17:1–9. doi: 10.4314/thrb.v17i2
95. da Cunha IT Jr, Lim PA, Qureshy H, Henson H, Monga T, Protas EJ. Gait outcomes after acute stroke rehabilitation with supported treadmill ambulation training: a randomized controlled pilot study. Arch Phys Med Rehabil. (2002) 83:1258–65. doi: 10.1053/apmr.2002.34267
96. Iosa M, Bini F, Marinozzi F, Fusco A, Morone G, Koch G, et al. Stability and harmony of gait in patients with subacute stroke. J Med Biol Eng. (2016) 36:635–43. doi: 10.1007/s40846-016-0178-0
97. Sousa AS, Silva A, Santos R, Sousa F, Tavares JMR. Interlimb coordination during the stance phase of gait in subjects with stroke. Arch Phys Med Rehabil. (2013) 94:2515–22. doi: 10.1016/j.apmr.2013.06.032
98. Chen X, Zheng Z, Cui Zz, Zheng W. A novel remote sensing technique for recognizing human gait based on the measurement of induced electrostatic current. J Electrostat. (2012) 70:105–10. doi: 10.1016/j.elstat.2011.11.003
99. Li M, Tian S, Sun L, Chen X. Gait analysis for post-stroke hemiparetic patient by multi-features fusion method. Sensors (2019) 19:1737. doi: 10.3390/s19071737
100. Krishnan V, Khoo I, Marayong P, DeMars K, Cormack J. Gait training in chronic stroke using Walk-Even feedback device: a pilot study. Neurosci J. (2016) 2016:6808319. doi: 10.1155/2016/6808319
101. Bonato P. Wearable sensors/systems and their impact on biomedical engineering. IEEE Eng Med Biol Mag. (2003) 22:18–20. doi: 10.1109/MEMB.2003.1213622
102. Benoussaad M, Sijobert B, Mombaur K, Azevedo Coste C. Robust foot clearance estimation based on the integration of foot-mounted IMU acceleration data. Sensors (2016) 16:12. doi: 10.3390/s16010012
103. Caldas R, Mundt M, Potthast W, de Lima Neto FB, Markert B. A systematic review of gait analysis methods based on inertial sensors and adaptive algorithms. Gait Posture (2017) 57:204–10. doi: 10.1016/j.gaitpost.2017.06.019
104. Lefeber N, Degelaen M, Truyers C, Safin I, Beckwée D. Validity and reproducibility of inertial physilog sensors for spatiotemporal gait analysis in patients with stroke. IEEE Tran Neural Sys Rehabil Eng. (2019) 27:1865–74. doi: 10.1109/TNSRE.2019.2930751
105. Jang H, Kim Y, Oh J, Lee S. Barriers to using balance and gait assessment tools by physical therapists in patients with neurological impairments: a systematic review. J Clin Res Bioeth. (2017) 8:309–14. doi: 10.4172/2155-9627.1000309
106. Littrell ME, Chang YH, Selgrade BP. Development and assessment of a low-cost clinical gait analysis system. J Appl Biomech. (2018) 34:503–8. doi: 10.1123/jab.2017-0370
107. Ladha C, Del Din S, Nazarpour K, Hickey A, Morris R, Catt M, et al. Toward a low-cost gait analysis system for clinical and free-living assessment. In: 2016 38th Annual International Conference of the IEEE Engineering in Medicine and Biology Society (EMBC). Orlando, FL: IEEE (2016). p. 1874–1877.
108. Wikström J, Georgoulas G, Moutsopoulos T, Seferiadis A. Intelligent data analysis of instrumented gait data in stroke patients A systematic review. Comput Biol Med. (2014) 51:61–72. doi: 10.1016/j.compbiomed.2014.04.004
109. Matsuda F, Mukaino M, Ohtsuka K, Tanikawa H, Tsuchiyama K, Teranishi T, et al. Analysis of strategies used by hemiplegic stroke patients to achieve toe clearance. Jpn J Comprehens Rehabil Sci. (2016) 7:111–8. doi: 10.11336/jjcrs.7.111
110. Lin PY, Yang YR, Cheng SJ, Wang RY. The relation between ankle impairments and gait velocity and symmetry in people with stroke. Arch Phys Med Rehabil. (2006) 87:562–8. doi: 10.1016/j.apmr.2005.12.042
111. Den Otter A, Geurts A, Mulder T, Duysens J. Gait recovery is not associated with changes in the temporal patterning of muscle activity during treadmill walking in patients with post-stroke hemiparesis. Clin Neurophysiol. (2006) 117:4–15. doi: 10.1016/j.clinph.2005.08.014
112. Patterson KK, Parafianowicz I, Danells CJ, Closson V, Verrier MC, Staines WR, et al. Gait asymmetry in community-ambulating stroke survivors. Arch Phys Med Rehabil. (2008) 89:304–10. doi: 10.1016/j.apmr.2007.08.142
113. Cruz TH, Lewek MD, Dhaher YY. Biomechanical impairments and gait adaptations post-stroke: multi-factorial associations. J Biomech. (2009) 42:1673–7. doi: 10.1016/j.jbiomech.2009.04.015
114. Allen JL, Kautz SA, Neptune RR. Step length asymmetry is representative of compensatory mechanisms used in post-stroke hemiparetic walking. Gait Posture (2011) 33:538–43. doi: 10.1016/j.gaitpost.2011.01.004
115. Park J, Yoo I. Relationships of stroke patients' gait parameters with fear of falling. J Phys Therapy Sci. (2014) 26:1883–4. doi: 10.1589/jpts.26.1883
116. Roche N, Bonnyaud C, Geiger M, Bussel B, Bensmail D. Relationship between hip flexion and ankle dorsiflexion during swing phase in chronic stroke patients. Clin Biomech. (2015) 30:219–25. doi: 10.1016/j.clinbiomech.2015.02.001
117. Halilaj E, Rajagopal A, Fiterau M, Hicks JL, Hastie TJ, Delp SL. Machine learning in human movement biomechanics: best practices, common pitfalls, and new opportunities. J Biomech. (2018) 81:1–11. doi: 10.1016/j.jbiomech.2018.09.009
118. Milovanović I, Popović DB. Principal component analysis of gait kinematics data in acute and chronic stroke patients. Comput Math Methods Med. (2012) 2012:649743. doi: 10.1155/2012/649743
119. Lois JMB, Broseta MJV, del Horno SM, Sánchez-Sánchez ML, Matas M, Viosca E. Functional data analysis for gait analysis after stroke. In: Converging Clinical and Engineering Research on Neurorehabilitation. Springer (2013). p. 775–9. doi: 10.1007/978-3-642-34546-3_126
120. Kaczmarczyk K, Wit A, Krawczyk M, Zaborski J. Gait classification in post-stroke patients using artificial neural networks. Gait Posture (2009) 30:207–10. doi: 10.1016/j.gaitpost.2009.04.010
121. Mulroy S, Gronley J, Weiss W, Newsam C, Perry J. Use of cluster analysis for gait pattern classification of patients in the early and late recovery phases following stroke. Gait Posture (2003) 18:114–25. doi: 10.1016/S0966-6362(02)00165-0
122. Kinsella S, Moran K. Gait pattern categorization of stroke participants with equinus deformity of the foot. Gait Posture (2008) 27:144–51. doi: 10.1016/j.gaitpost.2007.03.008
123. Wang M, Fan Z, Chen F, Zhang S, Peng C, et al. Research on feature extraction algorithm for plantar pressure image and gait analysis in stroke patients. J Vis Commun Image Represent. (2019) 58:525–31. doi: 10.1016/j.jvcir.2018.12.017
124. Turnbull G, Wall J. Long-term changes in hemiplegic gait. Gait Posture (1995) 3:258–61. doi: 10.1016/0966-6362(96)82856-6
125. Chen G, Patten C, Kothari DH, Zajac FE. Gait differences between individuals with post-stroke hemiparesis and non-disabled controls at matched speeds. Gait Posture (2005) 22:51–6. doi: 10.1016/j.gaitpost.2004.06.009
126. Wang Y, Mukaino M, Ohtsuka K, Otaka Y, Tanikawa H, Matsuda F, et al. Gait characteristics of post-stroke hemiparetic patients with different walking speeds. Int J Rehabil Res Int Zeitschr Rehabil Rev Int Recherch Readapt. (2020) 43:69. doi: 10.1097/MRR.0000000000000391
127. Harris-Love ML, Forrester LW, Macko RF, Silver KH, Smith GV. Hemiparetic gait parameters in overground versus treadmill walking. Neurorehabil Neural Repair (2001) 15:105–12. doi: 10.1177/154596830101500204
128. Olney SJ, Griffin MP, Monga TN, McBride ID. Work and power in gait of stroke patients. Arch Phys Med Rehabil. (1991) 72:309–14.
129. Richards C, Knutsson E. Evaluation of abnormal gait patterns by intermittent-light photography and electromyography. Scand J Rehabil Med Suppl. (1974) 3:61.
130. Carlsöö S, Dahlöf A, Holm J. Kinetic analysis of the gait in patients with hemiparesis and in patients with intermittent claudication. Scand J Rehabil Med. (1974) 6:166.
131. Wong AM, Pei YC, Hong WH, Chung CY, Lau YC, Chen CP. Foot contact pattern analysis in hemiplegic stroke patients: an implication for neurologic status determination. Arch Phys Med Rehabil. (2004) 85:1625–30. doi: 10.1016/j.apmr.2003.11.039
132. Ancillao A, Tedesco S, Barton J, O'Flynn B. Indirect measurement of ground reaction forces and moments by means of wearable inertial sensors: a systematic review. Sensors (2018) 18:2564. doi: 10.3390/s18082564
133. Meyring S, Diehl R, Milani T, Hennig E, Berlit P. Dynamic plantar pressure distribution measurements in hemiparetic patients. Clin Biomech. (1997) 12:60–5. doi: 10.1016/S0268-0033(96)00050-2
134. Gaviria M, D'Angeli M, Chavet P, Pelissier J, Peruchon E, Rabischong P. Plantar dynamics of hemiplegic gait: a methodological approach. Gait Posture (1996) 4:297–305. doi: 10.1016/0966-6362(95)01055-6
135. Sanghan S, Chatpun S, Leelasamran W. Plantar pressure difference: decision criteria of motor relearning feedback insole for hemiplegic patients. Int Proc Chem Biol Environ Eng. (2012) 29:29–33.
137. Dimitrijević M, Faganel J, Sherwood A, McKay W. Activation of paralysed leg flexors and extensors during gait in patients after stroke. Scand J Rehabil Med. (1981) 13:109–15.
138. Korpelainen JT, Sotaniemi KA, Makikallio A, Huikuri HV, Myllyla VV. Dynamic behavior of heart rate in ischemic stroke. Stroke (1999) 30:1008–3. doi: 10.1161/01.STR.30.5.1008
139. Hoshino T, Oguchi K, Hoshiyama M. Recovery of autonomic function assessed by heart rate variability after stroke. Physiotherapy (2016) 102:105–6. doi: 10.1016/j.physio.2016.10.112
140. Sethi A, Callaway CW, Sejdić E, Terhorst L, Skidmore ER. Heart rate variability is associated with motor outcome 3-months after stroke. J Stroke Cerebrovasc. Dis. (2016) 25:129–35. doi: 10.1016/j.jstrokecerebrovasdis.2015.09.005
141. Begg RK, Tirosh O, Said CM, Sparrow WA, Steinberg N, Levinger P, et al. Gait training with real-time augmented toe-ground clearance information decreases tripping risk in older adults and a person with chronic stroke. Front Hum Neurosci. (2014) 8:243. doi: 10.3389/fnhum.2014.00243
142. Burpee JL, Lewek MD. Biomechanical gait characteristics of naturally occurring unsuccessful foot clearance during swing in individuals with chronic stroke. Clin Biomech. (2015) 30:1102–7. doi: 10.1016/j.clinbiomech.2015.08.018
143. Laurent G, Valentini F, Loiseau K, Hennebelle D, Robain G. Claw toes in hemiplegic patients after stroke. Ann Phys Rehabil Med. (2010) 53:77–85. doi: 10.1016/j.rehab.2009.12.005
144. Ledley RS, Lusted LB. Reasoning foundations of medical diagnosis. Science (1959) 130:9–21. doi: 10.1126/science.130.3366.9
145. Bartlett R. Artificial intelligence in sports biomechanics: new dawn or false hope? J Sports Sci Med. (2006) 5:474–9.
146. Junkes-Cunha M, Cardozo G, Boos CF, de Azevedo F. Implementation of expert systems to support the functional evaluation of stand-to-sit activity. Biomed Eng Online (2014) 13:98. doi: 10.1186/1475-925X-13-98
147. Bontrager E, Perry J, Bogey R, Gronley J, Barnes L, Bekey G, et al. GAIT-ER-AID: An expert system for analysis of gait with automatic intelligent pre-processing of data. In: Proceedings of the Annual Symposium on Computer Application in Medical Care. American Medical Informatics Association (1990). p. 625.
148. Kirkwood C, Andrews B, Mowforth P. Automatic detection of gait events: a case study using inductive learning techniques. J Biomed Eng. (1989) 11:511–6. doi: 10.1016/0141-5425(89)90046-0
149. Mainka C, Friedrich M, Boenick U. Integrated gait analysis: a complex expert system approach to detailed data evaluation. Biomed Technik Biomed Eng. (1995) 40:363–71. doi: 10.1515/bmte.1995.40.12.363
150. Osis ST, Kobsar D, Leigh RJ, Macaulay CA, Ferber R. An expert system feedback tool improves the reliability of clinical gait kinematics for older adults with lower limb osteoarthritis. Gait Posture (2017) 58:261–7. doi: 10.1016/j.gaitpost.2017.08.011
151. Muro-De-La-Herran A, Garcia-Zapirain B, Mendez-Zorrilla A. Gait analysis methods: An overview of wearable and non-wearable systems, highlighting clinical applications. Sensors (2014) 14:3362–94. doi: 10.3390/s140203362
152. Lugade V, Lin V, Farley A, Chou LS. An artificial neural network estimation of gait balance control in the elderly using clinical evaluations. PLoS ONE (2014) 9:e97595. doi: 10.1371/journal.pone.0097595
153. Wu WL, Su FC. Potential of the back propagation neural network in the assessment of gait patterns in ankle arthrodesis. Clin Biomech. (2000) 15:143–5. doi: 10.1016/S0268-0033(99)00037-6
154. Sepulveda F, Wells DM, Vaughan CL. A neural network representation of electromyography and joint dynamics in human gait. J Biomech. (1993) 26:101–9. doi: 10.1016/0021-9290(93)90041-C
155. Hahn ME, Farley AM, Lin V, Chou LS. Neural network estimation of balance control during locomotion. J Biomech. (2005) 38:717–24. doi: 10.1016/j.jbiomech.2004.05.012
156. Miller A. Gait event detection using a multilayer neural network. Gait Posture (2009) 29:542–5. doi: 10.1016/j.gaitpost.2008.12.003
157. Jelinek HF, Khalaf K, Poilvet J, Khandoker AH, Heale L, Donnan LA. The effect of ankle support on lower limb kinematics during the Y-balance test using nonlinear dynamic measures. Front Physiol. (2019) 10:935. doi: 10.3389/fphys.2019.00935
158. Al-Angari HM, Khandoker AH, Lee S, Almahmeed W, Al Safar HS, Jelinek HF, et al. Novel dynamic peak and distribution plantar pressure measures on diabetic patients during walking. Gait Posture (2017) 51:261–7. doi: 10.1016/j.gaitpost.2016.11.006
159. Andreo P, Khalaf K, Heale L, Jelinek HF, Donnan L. Effects of Kinesiology Tape on Non-linear Center of Mass Dispersion During the Y Balance Test. Front Physiol. (2018) 9:1527. doi: 10.3389/fphys.2018.01527
160. Horst F, Lapuschkin S, Samek W, Müller KR, Schöllhorn WI. Explaining the unique nature of individual gait patterns with deep learning. Sci Rep. (2019) 9:1–13. doi: 10.1038/s41598-019-38748-8
161. Schöllhorn W, Nigg B, Stefanyshyn D, Liu W. Identification of individual walking patterns using time discrete and time continuous data sets. Gait Posture (2002) 15:180–6. doi: 10.1016/S0966-6362(01)00193-X
162. Gershman SJ, Horvitz EJ, Tenenbaum JB. Computational rationality: a converging paradigm for intelligence in brains, minds, and machines. Science (2015) 349:273–8. doi: 10.1126/science.aac6076
163. Lau HY, Tong KY, Zhu H. Support vector machine for classification of walking conditions using miniature kinematic sensors. Med Biol Eng Comput. (2008) 46:563–73. doi: 10.1007/s11517-008-0327-x
164. Kaptein RG, Wezenberg D, IJmker T, Houdijk H, Beek PJ, Lamoth CJ, et al. Shotgun approaches to gait analysis: insights & limitations. J Neuroeng Rehabil. (2014) 11:120. doi: 10.1186/1743-0003-11-120
165. Laroche D, Tolambiya A, Morisset C, Maillefert JF, French RM, Ornetti P, et al. A classification study of kinematic gait trajectories in hip osteoarthritis. Comput Biol Med. (2014) 55:42–8. doi: 10.1016/j.compbiomed.2014.09.012
166. Karg M, Seiberl W, Kreuzpointner F, Haas JP, Kulić D. Clinical gait analysis: comparing explicit state duration HMMs using a reference-based index. IEEE Trans Neural Syst Rehabil Eng. (2014) 23:319–31. doi: 10.1109/TNSRE.2014.2362862
167. Cippitelli E, Gasparrini S, Spinsante S, Gambi E. Kinect as a tool for gait analysis: validation of a real-time joint extraction algorithm working in side view. Sensors (2015) 15:1417–34. doi: 10.3390/s150101417
168. Joyseeree R, Abou RS, Müller H. Applying machine learning to gait analysis data for disease identification. Stud Health Technol Inform. (2015) 210:850–4.
169. LeMoyne R, Mastroianni T, Hessel A, Nishikawa K. Implementation of machine learning for classifying prosthesis type through conventional gait analysis. In: 2015 37th Annual International Conference of the IEEE Engineering in Medicine and Biology Society (EMBC). Milan: IEEE (2015). p. 202–205.
170. Ferber R, Osis ST, Hicks JL, Delp SL. Gait biomechanics in the era of data science. J Biomech. (2016) 49:3759–61. doi: 10.1016/j.jbiomech.2016.10.033
171. Osis ST, Hettinga BA, Ferber R. Predicting ground contact events for a continuum of gait types: an application of targeted machine learning using principal component analysis. Gait Posture (2016) 46:86–90. doi: 10.1016/j.gaitpost.2016.02.021
172. Zeng W, Liu F, Wang Q, Wang Y, Ma L, Zhang Y. Parkinson's disease classification using gait analysis via deterministic learning. Neurosci Lett. (2016) 633:268–78. doi: 10.1016/j.neulet.2016.09.043
173. Hannink J, Kautz T, Pasluosta CF, Gaßmann KG, Klucken J, Eskofier BM. Sensor-based gait parameter extraction with deep convolutional neural networks. IEEE J Biomed Health Inf (2016) 21:85–93. doi: 10.1109/JBHI.2016.2636456
174. Park E, Lee SI, Nam HS, Garst JH, Huang A, Campion A, et al. Unobtrusive and continuous monitoring of alcohol-impaired gait using smart shoes. Methods Inf Med. (2017) 56:74–82. doi: 10.3414/ME15-02-0008
175. Pham TD, Yan H. Tensor decomposition of gait dynamics in Parkinson's disease. IEEE Trans Biomed Eng. (2017) 65:1820–7. doi: 10.1109/TBME.2017.2779884
176. Ertelt T, Solomonovs I, Gronwald T. Enhancement of force patterns classification based on Gaussian distributions. J Biomech. (2018) 67:144–9. doi: 10.1016/j.jbiomech.2017.12.006
177. Haji Ghassemi N, Hannink J, Martindale CF, Gaßner H, Müller M, Klucken J, et al. Segmentation of gait sequences in sensor-based movement analysis: a comparison of methods in Parkinson's disease. Sensors (2018) 1:145. doi: 10.3390/s18010145
178. Zhan A, Mohan S, Tarolli C, Schneider RB, Adams JL, Sharma S, et al. Using smartphones and machine learning to quantify Parkinson disease severity: the mobile Parkinson disease score. J Am Med Assoc Neurol. (2018) 75:876–80. doi: 10.1001/jamaneurol.2018.0809
179. Zhang X, Fiedler G, Cao Z, Liu Z. A support vector machine approach to detect trans-tibial prosthetic misalignment using 3-Dimensional ground reaction force features: a proof of concept. Technol Health Care (2018) 26:715–21. doi: 10.3233/THC-181338
180. Bastien GJ, Gosseye TP, Penta M. A robust machine learning enabled decomposition of shear ground reaction forces during the double contact phase of walking. Gait Posture (2019) 73:221–7. doi: 10.1016/j.gaitpost.2019.07.190
181. Galbusera F, Casaroli G, Bassani T. Artificial intelligence and machine learning in spine research. JOR Spine (2019) 2:e1044. doi: 10.1002/jsp2.1044
182. Jiang X, Gholami M, Khoshnam M, Eng JJ, Menon C. Estimation of Ankle Joint Power during Walking Using Two Inertial Sensors. Sensors (2019) 19:2796. doi: 10.3390/s19122796
183. Nguyen TQ, Young JH, Rodriguez A, Zupancic S, Lie DY. Differentiation of patients with balance insufficiency (vestibular hypofunction) versus normal subjects using a low-cost small wireless wearable gait sensor. Biosensors (2019) 9:29. doi: 10.3390/bios9010029
184. Prado A, Cao X, Robert MT, Gordon AM, Agrawal SK. Gait segmentation of data collected by instrumented shoes using a recurrent neural network classifier. Phys Med Rehabil Clin. (2019) 30:355–66. doi: 10.1016/j.pmr.2018.12.007
185. Waugh JL, Huang E, Fraser JE, Beyer KB, Trinh A, McIlroy WE, et al. Online learning of gait models from older adult data. IEEE Trans Neural Syst Rehabil Eng. (2019) 27:733–42. doi: 10.1109/TNSRE.2019.2904477
Keywords: post-stroke, gait, hemiplegia, machine learning, statistical tools, spatiotemporal, dynamics, artificial intelligence
Citation: Mohan DM, Khandoker AH, Wasti SA, Ismail Ibrahim Ismail Alali S, Jelinek HF and Khalaf K (2021) Assessment Methods of Post-stroke Gait: A Scoping Review of Technology-Driven Approaches to Gait Characterization and Analysis. Front. Neurol. 12:650024. doi: 10.3389/fneur.2021.650024
Received: 06 January 2021; Accepted: 07 May 2021;
Published: 08 June 2021.
Edited by:
Marialuisa Gandolfi, University of Verona, ItalyReviewed by:
Birgitta Langhammer, Oslo Metropolitan University, NorwayMarco Iosa, Sapienza University of Rome, Italy
Copyright © 2021 Mohan, Khandoker, Wasti, Ismail Ibrahim Ismail Alali, Jelinek and Khalaf. This is an open-access article distributed under the terms of the Creative Commons Attribution License (CC BY). The use, distribution or reproduction in other forums is permitted, provided the original author(s) and the copyright owner(s) are credited and that the original publication in this journal is cited, in accordance with accepted academic practice. No use, distribution or reproduction is permitted which does not comply with these terms.
*Correspondence: Kinda Khalaf, kinda.khalaf@ku.ac.ae