- 1Center for Innovation in Brain Science, University of Arizona, Tucson, AZ, United States
- 2Social, Cognitive, and Affective Neuroscience Lab, University of Arizona, Tucson, AZ, United States
Background: Mild traumatic brain injuries (mTBIs) are associated with novel or worsened sleep disruption. Several studies indicate that daily morning blue light therapy (BLT) is effective for reducing post-mTBI daytime sleepiness and fatigue. Studies demonstrating changes in brain structure and function following BLT are limited. The present study's purpose is to identify the effect of daily morning BLT on brain structure and functional connectivity and the association between these changes and self-reported change in post-mTBI daytime sleepiness.
Methods: A total of 62 individuals recovering from a mTBI were recruited from two US cities to participate in a double-blind placebo-controlled trial. Eligible individuals were randomly assigned to undergo 6 weeks of 30 min daily morning blue or placebo amber light therapy (ALT). Prior to and following treatment all individuals completed a comprehensive battery that included the Epworth Sleepiness Scale as a measure of self-reported daytime sleepiness. All individuals underwent a multimodal neuroimaging battery that included anatomical and resting-state functional magnetic resonance imaging. Atlas-based regional change in gray matter volume (GMV) and region-to-region functional connectivity from baseline to post-treatment were the primary endpoints for this study.
Results: After adjusting for pre-treatment GMV, individuals receiving BLT had greater GMV than those receiving amber light in 15 regions of interest, including the right thalamus and bilateral prefrontal and orbitofrontal cortices. Improved daytime sleepiness was associated with greater GMV in 74 ROIs, covering many of the same general regions. Likewise, BLT was associated with increased functional connectivity between the thalamus and both prefrontal and orbitofrontal cortices. Improved daytime sleepiness was associated with increased functional connectivity between attention and cognitive control networks as well as decreased connectivity between visual, motor, and attention networks (all FDR corrected p < 0.05).
Conclusions: Following daily morning BLT, moderate to large increases in both gray matter volume and functional connectivity were observed in areas and networks previously associated with both sleep regulation and daytime cognitive function, alertness, and attention. Additionally, these findings were associated with improvements in self-reported daytime sleepiness. Further work is needed to identify the personal characteristics that may selectively identify individuals recovering from a mTBI for whom BLT may be optimally beneficial.
Introduction
Mild traumatic brain injury (mTBI), or concussion, occurs when an individual experiences a blow to the head or other mechanical force that results in altered cognition and/or brief loss of consciousness. The short- and long-term effects of mTBIs are of significant concern across the spectrum of sports, military, and public health. In light of the conservatively estimated 3 million mTBIs reported to emergency rooms each year (1, 2) those sustained by athletes (3) and military Service members (4) that are cared for by embedded medical teams, the need for effective treatments is great. While considerable work is being done to identify biomarkers of injury (5–7) and improve clinical diagnoses (8–10), there is comparatively little in the way of efficacious treatments (11–14).
Among the myriad of neurobiological consequences faced by individuals who have sustained a mild traumatic brain injury, sleep disruption is among the most common and the most persistent (15–19). Up to 90% of individuals who sustain a mTBI report some form of sleep disruption—including insomnia, frequent wakefulness after sleep onset, and a general sense of poor sleep quality (19–24)—or associated complaints of increased daytime fatigue or sleepiness that interferes with one or more activities of daily living (25–28). These sleep-related complaints often go untreated and may persist for months to years post-injury.
Post-mTBI sleep disruption is also associated with slowed recovery (29–32), exacerbated symptom presentation (29, 31), and degraded overall functioning (cognitive, motor, emotional) (33–37). Notably, a reciprocal cycle appears to exist where daytime fatigue and sleepiness predispose individuals to future mTBIs, while mTBIs predispose individuals to future daytime fatigue and sleepiness (27). Consequently, identifying and developing effective treatments to deal with sleep-related disruptions is necessary to support high-quality academic, job, and sport performance. While there is evidence that pharmacologic intervention may be beneficial for some individuals following a mTBI (38–40), there is some suggestion that these may not be the best first course of treatment (41) and it is likely that non-pharmacologically driven treatments offer the added benefit of lower risk for substance dependence.
Among available non-pharmacologic treatments for sleep-related disruptions, morning blue light therapy (BLT) has been shown to be effective in reducing daytime fatigue in individuals recovering from a mTBI (42–44). Additionally, this work suggests that these effects on daytime fatigue may be associated with shifts in circadian phase in some patients (43). While these outward effects on daytime functioning are encouraging, the associated effects of blue light therapy on the brain in those recovering from a mTBI has not been extensively examined.
Work from our group has focused on using multimodal neuroimaging to examine both the acute effects of blue light on cognitive function and alertness, as well as the effects of treatment in those recovering from a mTBI. Our work suggests that – in comparison to amber light exposure—a single, short duration (30 min) blue light exposure modulates anterior cingulate activation in anticipation tasks (45), dorso- and ventrolateral prefrontal cortex activation in working memory tasks (46), and may improve neural efficiency during interference tasks (47) while being associated with improved task performance on anticipation, working memory, and verbal memory tasks (45, 46, 48).
Recently, we completed two separate randomized clinical trials using morning blue light therapy (BLT) as a treatment for mTBI-related sleep disruption vs. an amber light control condition. Across both trials, BLT was associated with reduced self-reported daytime sleepiness at the end of six of weeks of treatment (43, 44); a circadian phase advance and increased midday sleep onset latency (43); and improved actigraphically-measured nighttime sleep quality (44). Neuroimaging evidence from the first trial indicated that BLT was associated with increases in bilateral thalamic volume, particularly in the pulvinar region (43); improved functional and structural connectivity between the thalamus and areas of the parietal cortex (43); and altered white matter diffusion characteristics in white matter tracts passing the through the thalamus, corpus callosum, and left anterior corona radiata (43, 49). These neuroimaging findings suggest that altered brain structure and function in regions and circuits subserving alertness, attention, and cognitive control may be facilitated by improved nighttime sleep and reduced daytime sleepiness.
What remains unknown are the broader effects of BLT on brain structure and function following a mTBI. The effects on thalamic volume and connectivity described above were observed in a small sample (total n = 31) using targeted follow-up analyses (e.g., thalamic volume changes were observed using voxel-based morphometry and subsequently used as a seed region for examining function and structural connectivity changes) (43). The purpose of the present study is to expand these neuroimaging findings by (A) combining the samples from both trials and (B) applying an atlas-based approach to both gray matter volumetry and functional connectivity to explore the effects of BLT, as opposed to amber light therapy (ALT), in individuals recovering from a mTBI. We hypothesized that, consistent with prior findings, BLT would be associated with increased volume in and connectivity between cortical and subcortical regions involved in task-related attention and cognitive control.
Materials and Methods
Participants
Sixty-two individuals were recruited across two conceptually linked studies on the effects of BLT on sleep-related outcomes following mTBI. These studies took place in Boston, MA (study 1; n = 31) and Tucson, AZ (study 2; n = 31). Data on these two samples have been reported previously for individual samples (43, 44, 49), but the findings reported here are novel and have not been previously presented. Inclusion and exclusion criteria were the same across both studies and have been described elsewhere (43, 44, 50). Briefly, all individuals sustained a mTBI within 18 months of enrollment according to the mTBI definition consistent with the Veteran's Administration/Department of Defense guidelines (51). Individuals provided documentation of their head injury either from a medical provider, qualified third-party witness, or first responder. Qualified witness reports—including those from a coach, allied health professional, or emergency personnel—were accepted, as many mTBIs are not evaluated in a physician's office or emergency department. mTBI reports were further corroborated by the participants via the Ohio State University Traumatic Brain Injury ID self-report form (52, 53). Individuals were excluded on the basis of pre-existing medical or neuropsychiatric disorders, a history of a moderate to severe TBI, alcohol or illicit substance abuse, or contra-indications for neuroimaging. None of the participants were undergoing treatment or taking medications for sleep disorders. Complete exclusionary criteria have been previously described (43, 44). Prior to study initiation, all procedures were reviewed and approved by the Institutional Review Boards for Partner's Healthcare (study 1), the University of Arizona (study 2), and the Human Research Protections Office of the U.S. Army (both studies). All participants were fully informed of all study procedures and provided written informed consent.
Study Procedures
Participants completed three in-lab visits separated by 1- and 6-weeks, respectively. Individuals were first screened to confirm meeting eligibility criteria and given a wrist-worn accelerometer used to quantify 24-h sleep patterns for the duration of the study [Philips Respironics Actiwatch Spectrum; data previously reported and not included here (43, 44, 50)]. Participants returned to the lab 1 week later and completed a comprehensive neuropsychological and self-report assessment battery, as well as a multimodal imaging protocol (described below). Following these procedures, individuals were randomized to either the BLT (λ ~ 469 nm; total illuminance 214 lux; total irradiance: 248 μW/cm2 at 50 cm; Philips goLITE BLUE, Philips Electronics, Stamford Connecticut) or ALT (λ ~ 578 nm; total illuminance:188 lux; total irradiance: 35 μW/cm2 at 50 cm; Philips Electronics custom light box) treatment groups. Participants were provided a corresponding light box to be used at home, based on treatment group. Treatment consisted of 6 weeks of at-home daily light box use (30 min per day within 2 h of waking). The light device was placed at arm's length at a slight angle from the participant's direction of gaze, but facing the participant enough to bathe the face and eyes with the light. Participants returned to the lab after 6 weeks of light therapy and completed identical testing and neuroimaging procedures.
Image Acquisition
The multimodal neuroimaging protocol included the collection of structural, functional, and diffusion weighted imaging. Neuroimaging was conducted pre- and post-treatment, resulting in two sets of images for each participant. The results presented here include only the structural and functional imaging findings. With a few minor exceptions, imaging protocols were nearly identical between the two studies.
Study 1: Boston, MA
All imaging data were collected on a 3.0T Siemens Tim Trio (Erlanger, Germany) magnetic resonance imaging (MRI) scanner located at McLean Hospital, Belmont, MA. Imaging included a T1-weighted 3D magnetization-prepared rapid acquisition gradient echo sequence (MPRAGE; TE: 2.3 ms; TR: 2.1 s; flip angle: 12°; acquisition matrix: 256 × 256; slice thickness: 1 mm, voxel size: 1 mm3) and a resting-state functional MRI sequence (rsFMRI; TE: 30 ms; TR: 2 s; flip angle: 90°; acquisition matrix: 64 × 64; slice thickness: 3.5 mm; voxel size: 3.5 mm3). For distortion correction, a gradient echo field map sequence was also collected (TE: 4.92/7.38 ms; TR: 625 ms; flip angle: 90°; acquisition matrix: 64 × 64; slice thickness: 3.5 mm; voxel size: 3.5 mm3) resulting in two magnitude images and a phase difference map. The set of baseline scans were collected during the in-lab visit, prior to light therapy. The set of post-treatment scans were collected 6 weeks later during the final in-lab visit, following the completion of either BLT or ALT.
Study 2: Tucson, AZ
Imaging data were collected on a 3.0 Siemens Skyra (Erlanger, Germany) MRI scanner located at the University of Arizona. Imaging included a T1-weighted MPRAGE sequence (TE: 2.3 ms; TR: 2.1 s; flip angle: 12°; acquisition matrix: 256 × 256; slice thickness: 1 mm, voxel size: 1 mm) and a rsFMRI sequence (TE: 25 ms; TR: 2 s; flip angle: 90°; acquisition matrix: 84 × 84; voxel size: 2 mm3). For distortion correction, a gradient echo field map sequence was also collected (TE: 4.92/7.38 ms; TR: 625 ms; flip angle: 90°; acquisition matrix: 64 × 64; slice thickness: 3.5 mm; voxel size: 3.5 mm3) resulting in two magnitude images and a phase difference map. Baseline scans were collected prior to light therapy and post-treatment scans were collected 6 weeks later, following the completion of at-home light therapy.
Imaging Processing
Imaging from both studies underwent the same pre- and post-processing protocol. Prior to pre-processing all data were converted from DICOM to NIFTI format using HeuDiConv (v. 0.6.0) into a Brain Imaging Dataset (BIDS) compliant format. Image quality was initially assessed using MRIQC (v 0.15.1). All participants in the present analyses had complete structural imaging datasets (one usable T1-weighted image).
Structural Image Processing
All T1-weighted images were processed using the Computation Anatomy Toolbox 12 (CAT12 r1450; http://www.neuro.uni-jena.de/cat/) implemented through Statistical Parametric Mapping 12 (SPM12 r7219; https://www.fil.ion.ucl.ac.uk/spm/) using MATLAB R2016A (The MathWorks Inc, Natick, MA). Prior to processing, all images were realigned to the anterior-posterior commissure axis using Convert3D (http://www.itksnap.org/pmwiki/pmwiki.php?n=Convert3D.Documentation). These realigned data were subsequently segmented using CAT12's longitudinal pipeline, which included denoising, skull stripping, three tissue type segmentation, and normalizing to Montreal Neurological Institute (MNI) space with an output resolution of 1 mm3. Total intracranial volume (ICV) was additionally computed using CAT12. Following normalization, gray matter volume estimates were extracted for each of 400 cortical (54), 36 subcortical (55), and 37 cerebellar (56) regions of interest (ROIs) using a concatenation of three well-validated atlases that has been previously used for similar purposes (57). Each of the ROIs was assigned to one of nine networks based on the Yeo 17-network parcellation [using overarching network names when multiple sub-networks exist; e.g., the default mode A, B, and C networks were labels as DMN; (58)] for the cortical ROIs, one subcortical network, and a cerebellar network.
rsFMRI Image Pre-processing
Results included in this manuscript come from preprocessing performed using fMRIPrep 20.1.3 [RRID:SCR_016216, (59, 60)], which is based on Nipype 1.5.1 [RRID:SCR_002502, (61, 62)]. The descriptions of the pre-processing steps in fMRIPrep are provided by the creators of the software under a CC0 license and reproduced here without changes (aside from formatting for references).
Anatomical data preprocessing
A total of 2 T1-weighted (T1w) images were found within the input BIDS dataset. All of them were corrected for intensity non-uniformity (INU) with N4BiasFieldCorrection (63), distributed with ANTs 2.2.0 [RRID:SCR_004757, (64)]. The T1w-reference was then skull-stripped with a Nipype implementation of the antsBrainExtraction.sh workflow (from ANTs), using OASIS30ANTs as the target template. Brain tissue segmentation of cerebrospinal fluid (CSF), white-matter (WM) and gray-matter (GM) was performed on the brain-extracted T1w using fast [FSL 5.0.9, RRID:SCR_002823, (65)]. A T1w-reference map was computed after registration of 3 T1w images (after INU-correction) using mri_robust_template [FreeSurfer 6.0.1, (66)]. Brain surfaces were reconstructed using recon-all [FreeSurfer 6.0.1, RRID:SCR_001847, (67)], and the brain mask estimated previously was refined with a custom variation of the method to reconcile ANTs-derived and FreeSurfer-derived segmentations of the cortical gray-matter of Mindboggle (RRID:SCR_002438) (68). Volume-based spatial normalization to two standard spaces (MNI152NLin2009cAsym, MNI152NLin6Asym) was performed through non-linear registration with antsRegistration (ANTs 2.2.0), using brain-extracted versions of both T1w reference and the T1w template. The following templates were selected for spatial normalization: ICBM 152 Non-linear Asymmetrical template version 2009c [RRID:SCR_008796; TemplateFlow ID: MNI152NLin2009cAsym, (69)], FSL's MNI ICBM 152 non-linear 6th Generation Asymmetric Average Brain Stereotaxic Registration Model [RRID:SCR_002823; TemplateFlow ID: MNI152NLin6Asym, (70)].
Functional data processing
For each of the two BOLD rsFMRI runs found per subject (across all sessions), the following preprocessing was performed. First, a reference volume and its skull-stripped version were generated using a custom methodology of fMRIPrep. Head-motion parameters with respect to the BOLD reference (transformation matrices, and six corresponding rotation and translation parameters) are estimated before any spatiotemporal filtering using mcflirt [FSL 5.0.9, (71)]. BOLD runs were slice-time corrected using 3dTshift from AFNI 20160207 [RRID:SCR_005927, (72)]. A B0-non-uniformity map (or fieldmap) was estimated based on a phase-difference map calculated with a dual-echo GRE (gradient-recall echo) sequence, processed with a custom workflow of SDCFlows inspired by the epidewarp.fsl script and further improvements in HCP Pipelines (73). The fieldmap was then co-registered to the target EPI (echo-planar imaging) reference run and converted to a displacements field map (amenable to registration tools such as ANTs) with FSL's fugue and other SDCflows tools. Based on the estimated susceptibility distortion, a corrected EPI (echo-planar imaging) reference was calculated for a more accurate co-registration with the anatomical reference. The BOLD reference was then co-registered to the T1w reference using bbregister (FreeSurfer) which implements boundary-based registration (74). Co-registration was configured with six degrees of freedom. The BOLD time-series (including slice-timing correction when applied) were resampled onto their original, native space by applying a single, composite transform to correct for head-motion and susceptibility distortions. These resampled BOLD time-series will be referred to as preprocessed BOLD in original space, or just preprocessed BOLD.
The BOLD time-series were resampled into several standard spaces, correspondingly generating the following spatially-normalized, preprocessed BOLD runs: MNI152NLin2009cAsym, MNI152NLin6Asym. First, a reference volume and its skull-stripped version were generated using a custom methodology of fMRIPrep. Several confounding time-series were calculated based on the preprocessed BOLD: framewise displacement (FD), DVARS and three region-wise global signals. FD was computed using two formulations following Power [absolute sum of relative motions, (75)], and Jenkinson [relative root mean square displacement between affines, (71)]. FD and DVARS are calculated for each functional run, both using their implementations in Nipype [following the definitions by Power et al. (75)]. The three global signals are extracted within the CSF, the WM, and the whole-brain masks.
Additionally, a set of physiological regressors were extracted to allow for component-based noise correction [CompCor, (76)]. Principal components are estimated after high-pass filtering the preprocessed BOLD time-series (using a discrete cosine filter with 128 s cut-off) for the two CompCor variants: temporal (tCompCor) and anatomical (aCompCor). tCompCor components are then calculated from the top 5% variable voxels within a mask covering the subcortical regions. This subcortical mask is obtained by heavily eroding the brain mask, which ensures it does not include cortical GM regions. For aCompCor, components are calculated within the intersection of the aforementioned mask and the union of CSF and WM masks calculated in T1w space, after their projection to the native space of each functional run (using the inverse BOLD-to-T1w transformation). Components are also calculated separately within the WM and CSF masks. For each CompCor decomposition, the k components with the largest singular values are retained, such that the retained components' time series are sufficient to explain 50 percent of variance across the nuisance mask (CSF, WM, combined, or temporal). The remaining components are dropped from consideration.
The head-motion estimates calculated in the correction step were also placed within the corresponding confounds file. The confound time series derived from head motion estimates and global signals were expanded with the inclusion of temporal derivatives and quadratic terms for each (77). Frames that exceeded a threshold of 0.5 mm FD or 1.5 standardized DVARS were annotated as motion outliers. All resamplings can be performed with a single interpolation step by composing all the pertinent transformations (i.e., head-motion transform matrices, susceptibility distortion correction when available, and co-registrations to anatomical and output spaces). Gridded (volumetric) resamplings were performed using antsApplyTransforms (ANTs), configured with Lanczos interpolation to minimize the smoothing effects of other kernels (78). Non-gridded (surface) resamplings were performed using mri_vol2surf (FreeSurfer).
Many internal operations of fMRIPrep use Nilearn 0.6.2 [RRID:SCR_001362, (79)], mostly within the functional processing workflow. For more details of the pipeline, see the section corresponding to workflows in fMRIPrep's documentation.
Functional Connectivity
Post-processing and functional connectivity estimation from the preprocessed BOLD time series (in MNI152NLin2009cAsym space) was accomplished for each subject and session using the eXtensible Connectivity Pipeline (XCP Engine, v 1.2.3; https://github.com/PennBBL/xcpEngine). Confound regressors were collected from fMRIPrep and included the mean global, cerebrospinal fluid, and white matter signals, framewise motion (x-, y-, and z- axis translation and rotation) as well as the derivatives and quadratic expansions of these terms [36 parameter confound regressors, (80)]. Processing steps included demeaning, detrending, and temporal filtering (0.01–0.08 Hz Butterworth filter) of the time series and regressors, as well as despiking of the BOLD time-series using 3dDespike from AFNI [RRID:SCR_005927, (72)] followed by regression using the 36 parameters. The residual BOLD time-series following regression was the averaged in each of the 473 ROIs used in estimating the gray matter volume. However, due to varying levels of field of view coverage for the cerebellum during the resting state imaging, the 37 cerebellar ROIs were ultimately excluded. Functional connectivity was then computed as the Pearson r correlation between each ROI, yielding a 436 × 436 functional connectivity matrix per subject per session (400 cortical ROIs, 36 subcortical ROIs). These connectivity matrices were subsequently Fisher r-to-z transformed to improve the normality of the distribution of the correlation coefficients. Additional quality metrics provided by XCP Engine included mean root mean square (RMS) motion estimates and node coverage.
Data Harmonization
A necessary consideration in the analyses of these data is the collection of imaging on different systems in different locations over the course of several years. To ensure that the present findings were not confounded by site effects, the GMV and functional connectivity data were individually harmonized using neuroCombat [https://github.com/Jfortin1/ComBatHarmonization, (81)] implemented in R [v. 3.6.1, (82)]. neuroCombat uses an Empirical Bayes approach to minimize site-level effects in neuroimaging data while preserving biological effects. This approach has successfully been used to harmonize structural, functional, and diffusion-weighted data (81, 83, 84). To ensure that effects of interest and potentially meaningful regressors were preserved, we included age, sex, number of previous mTBIs, days post-injury, and group (BLT, ALT) in the modeling. For the functional connectivity data, we additionally included mean root mean square motion from XCP Engine as a potential covariate.
Statistical Analyses
All post-processing statistical analyses were conducted in R and Python (v. 3.7.6). All between-groups analyses were ultimately conducted using DABEST [v 0.3.0, (85)] in Python. DABEST computes effect sizes for both between-group and within-group comparisons as well as bootstrapped, bias-corrected, accelerated (BCa) 95% confidence intervals (using 20,000 bootstrap resamples) around these effect sizes. Additionally, DABEST provides traditional hypothesis testing using Welch's t, Student's t, and Mann-Whitney U test p-values as well as a permutation-based p-value (5,000 permutations).
Treatment-Related Effects on GMV
We fit an initial regression model to both baseline and post-treatment harmonized ROI GMV estimates separately to remove the effects of total intracranial volume, age, and sex and extracted the residuals to estimate covariate-adjusted ROI volumes. We then regressed baseline covariate-adjusted values against post-treatment covariate-adjusted values. The residuals from this model were then passed to DABEST to compare BLT to ALT. Results are reported as Hedges' g effect sizes. In reporting these findings, we employed a hierarchical approach to controlling family-wise error. First, we included only ROIs whose 95% confidence intervals did not include 0. Second, we applied false discovery rate (FDR) correction to the permutation p-values from DABEST and thresholded the findings at FDR corrected p < 0.05. Treatment-related effects on functional connectivity.
Univariate correlations were fit for each edge at baseline in the harmonized dataset in the adjusted baseline models to additionally assess age, sex, total number of prior mTBIs, days post-injury, and relative RMS motion as potential covariates. Approximately 6% of the edges were associated with either age, total number of prior mTBIs, or days post-injury and none of these correlations survived multiple comparisons correction and so no further covariate adjustment was considered. Post-treatment functional connectivity data were regressed on the baseline data to remove baseline effects. Residuals from these baseline-adjusted models were passed to DABEST to compare BLT to ALT on an edge-by-edge basis. Similar to the GMV models we report Hedges g effect sizes for each edge thresholded by (1) 95% confidence intervals not including 0 and (2) FDR corrected permutation p < 0.05. Results were plotted using in Python using the plot_connectome function from Nilearn [v 0.6.2; RRID:SCR_001362; (79)].
Exploratory Analyses
In order to further explore the neuroimaging findings in relationship to previously identified behavior outcomes, we conducted several exploratory analyses. These analyses included correlations between baseline and baseline-adjusted daytime sleepiness (ESS scores; the primary behavioral outcome previously reported) and both GMV and functional connectivity. For baseline-adjusted ESS scores, these were computed as the residual ESS scores after regressing post-treatment values on baseline values. Consistent with our prior findings, these baseline-adjusted values were computed without adjusting for additional covariates (e.g., sex, number of mTBIs, days post-injury) as none improved the models in stepwise model selection. These correlations were fit in Python using the bootstrap-stat (https://github.com/rwilson4/bootstrap-stat) package to compute 95% BCa confidence intervals. Findings were initially thresholded to include only correlations with 95% BCa confidence intervals not including 0. Results were visualized using BrainNetViewer [v. 1.7; RRID:SCR_009446; (86)] for GMV correlations and plot_connectome for functional connectivity.
These exploratory analyses were executed to provide comprehensive estimates of effects for planning future similar studies.
Results
A total of 62 individuals (25 males) completed all study procedures across both cohorts. In general, participants were young adults (mean age: 24.7 ± 7.8 years) who were in the chronic phase of mTBI recovery (mean weeks from index injury: 36.4 ± 20.9). Across the combined sample, ESS scores approached, on average, the threshold for excessive daytime sleepiness (mean ESS: 9.2 ± 3.4; threshold for EDS = 10) (87). We previously reported baseline balance between blue and amber groups in each of the cohorts separately (43, 44).
Treatment Effects on GMV
After controlling for total intracranial volume, age, sex, and baseline GMV, a total of 17 ROIs survived multiple comparisons correction (all FDR corrected p < 0.05) and demonstrated moderate to large differences, including greater post-treatment GMV after BLT (n = 15) and ALT (n = 2; Figure 1, Table 1). Compared to ALT, greater GMV following BLT was observed primarily in the left hemisphere in regions associated with the attention, default mode, and limbic networks. Consistent with prior findings in the Boston subset of this sample, greater GMV was also observed in the right caudal temporal and right occipital thalamic ROIs. Greater GMV following ALT compared to BLT was observed in two areas of the cerebellum.
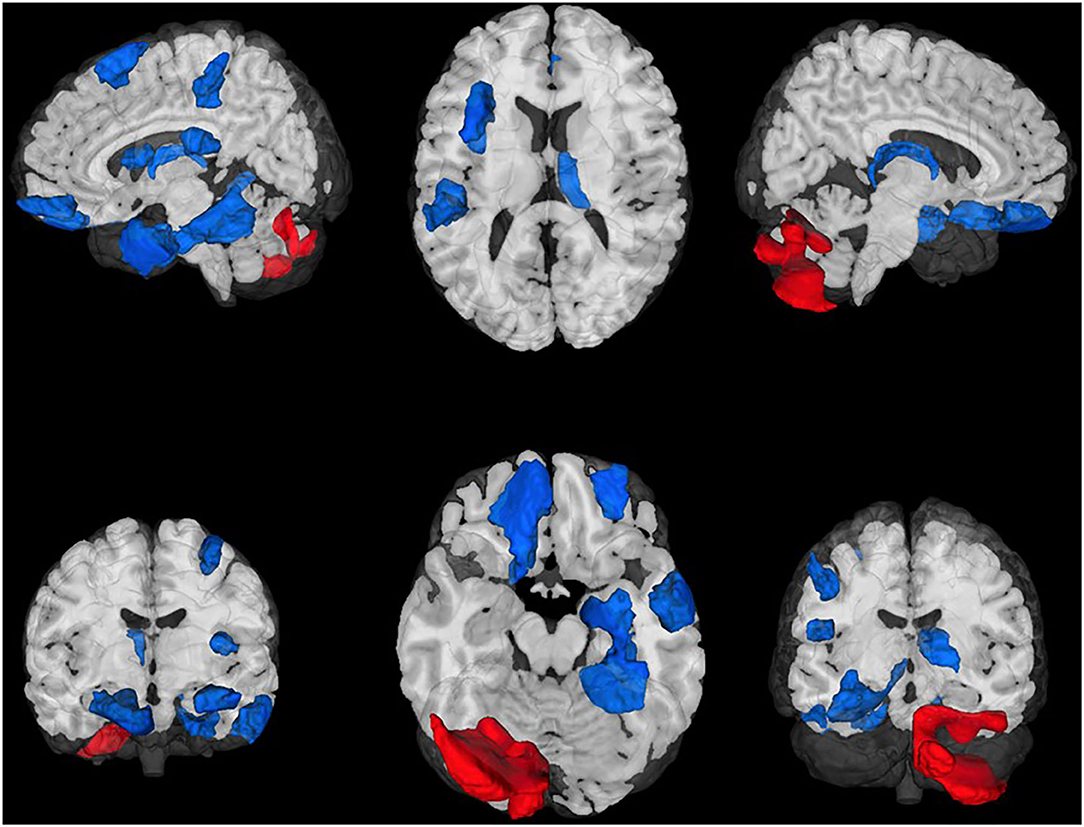
Figure 1. Regions of interest (ROIs) exhibiting moderate-to-large baseline adjusted differences in gray matter volume (GMV). Greater GMV was observed following blue light treatment (ROIs in blue) in 15 cortical and subcortical ROIs. Greater GMV was observed following amber light (red ROIs) in two cerebellar regions. All ROIs were false discovery rate corrected p < 0.05. Top Left: Lateral left view, Top Middle: Superior view; Top Right, Lateral right view. Bottom Left, anterior view; Bottom Middle: Inferior view; Bottom Right: Posterior view.
Treatment Effects on Functional Connectivity
After controlling for baseline functional connectivity, a total of 3,276 edges exhibited differences in connectivity (all FDR corrected p < 0.05) between the BLT and ALT groups. Greater connectivity following BLT was observed in n = 2,028 edges while lower connectivity was observed in n = 1,248. Based on the network labeling for the associated ROIs, these differences included a widespread network for connections between the default mode, somatomotor, cognitive control, subcortical gray matter, as well as dorsal and ventral attention networks.
The largest differences (FDR corrected p < 0.01; Figure 2) were observed in a total of 16 edges linking these same networks. These edges specifically include connections with the right thalamus, prefrontal and orbitofrontal cortices, and numerous somatomotor regions.
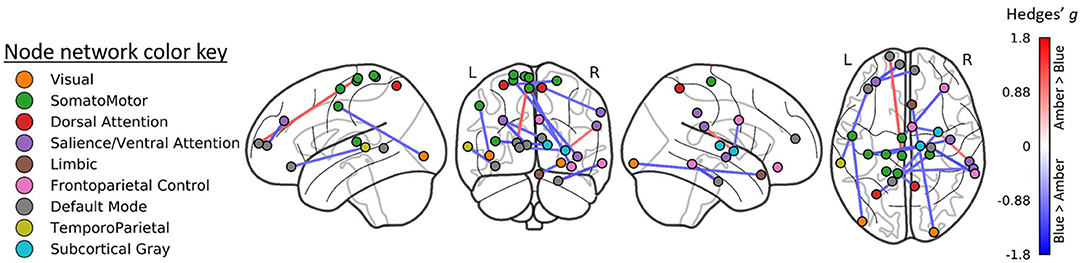
Figure 2. Region-to-region functional connectivity differences following treatment. Blue light treatment was associated with greater functional connectivity in 14 ROI-to-ROI connections linking the visual, default mode, somatomotor, and dorsal attention networks (all FDR p < 0.01).
Exploratory Analyses
Relationship Between ESS and GMV
At baseline, ESS scores were inversely correlated with GMV (i.e., greater ESS, lower GMV) in 20 cortical ROIs and positively associated with GMV 5 ROIs, including four subcortical ROIs. After adjusting for baseline GMV and ESS, decreased ESS scores at post-treatment were correlated with increased GMV in 15.6% of the 473 ROIs (n = 74; Figure 3) including the right caudal temporal thalamus. These ROIs were associated with the bilateral attention, cognitive control, visual, and default mode networks.
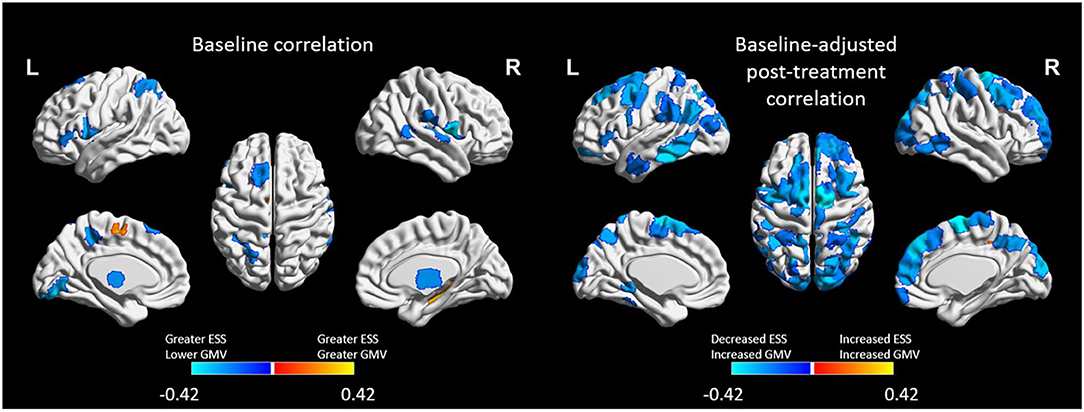
Figure 3. Regions of interest (ROIs) exhibiting moderate-to-large correlations (|r| > 0.4) between Epworth Sleepiness Scale scores and gray matter volume (GMV) at baseline (left) and at post-treatment (right). Treatment-related decreases in daytime sleepiness were associated with moderate-to-large increases in GMV in 74 ROIs. All ROIs were FDR corrected at p < 0.05.
Relationship Between ESS and Functional Connectivity
Prior to treatment, ESS scores were associated with functional connectivity across a total of 5,656 edges. Those with the strongest correlations (r > |0.40|, n = 144 edges) included positive correlations (greater ESS and greater connectivity; n = 72 edges) within the DMN as well as between the DMN, salience and cognitive control networks (n = 27/72 edges) and negative correlations (greater ESS, lower connectivity; n = 72 edges) primarily between the DMN and subcortical gray matter as well as between the temporoparietal network and the somatomotor and ventral attention networks (n = 27/72 edges across these connections).
A total 5,039 edges exhibited a change in connectivity that was associated with a change in daytime sleepiness, including 3,072 edges with increased connectivity and 1,967 with decreased connectivity. Examining those edges with the strongest correlations (r > |0.40|, n = 121 edges; Figure 4) revealed increased connectivity primarily associated with decreased ESS scores (negative correlation) in edges connecting the dorsal attention, somatomotor, and visual networks as well as the default mode, and subcortical networks (n = 48 edges total across these network connections; 82 total edges with negative correlations; Figure 4 bottom). Decreased connectivity was associated with decreased ESS scores in 39 edges (Figure 4 top).
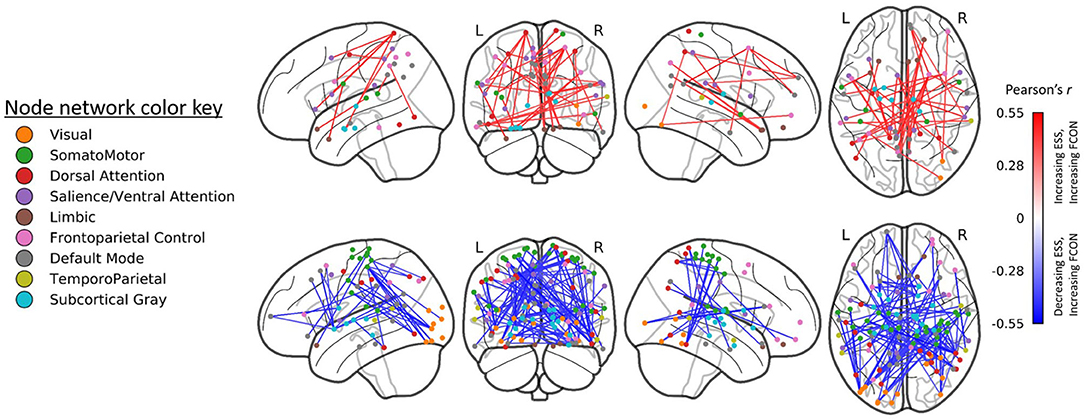
Figure 4. Edges (region-to-region connections) exhibiting moderate-to-large correlations (|r| > 0.4) between Epworth Sleepiness Scale scores and gray matter volume (GMV) at post-treatment (right). Treatment-related decreases in daytime sleepiness were associated with moderate-to-large increases in functional connectivity in 82 edges and with decreases in functional connectivity in 39 edges. All edgewise correlations were FDR corrected at p < 0.05.
Discussion
The primary purpose of this study was to extend our prior findings on the effects of 6 weeks of 30 min daily morning BLT on brain structure and function in individuals recovering from a mTBI. Consistent with our hypotheses and prior findings, we observed increased gray matter volume and modulated functional connectivity in and between areas of the brain associated with attention, cognitive control, salience, and visual processing among those who received BLT. Increased GMV after adjusting for baseline GMV was also observed in the right thalamus, consistent with the earlier voxel-based analysis of a subset of these data (43). These changes in both GMV and functional connectivity were additionally correlated with improvement in subjective daytime sleepiness.
In particular, individuals who reported improved daytime sleepiness exhibited increased GMV in the thalamus as well as orbitofrontal and prefrontal cortices and the precuneus, among other regions. Furthermore, increased functional connectivity associated with decreases in daytime sleepiness were observed in ROI-to-ROI connections linking these regions to other areas in the attention, default mode, visual, and somatomotor networks. This is consistent with other findings suggesting that daytime sleepiness is associated with reduced thalamocortical connectivity (88). These data provide evidence that, particularly for individuals experiencing daytime sleepiness following a mTBI, blue light therapy may confer beneficial effects, in part due to changes to both structure and function in brain networks associated with alertness and attention as well as linkage between these and the default mode network.
Blue Light Effects on GMV
The present findings extend our previous work on blue light therapy and GMV in several ways. Specifically, we provide further evidence that reduced thalamic volume is associated with daytime sleepiness following mTBI and that this volume increases following blue light therapy (as opposed to similar exposure to amber light). We additionally identified several other regions that were not previously observed in our voxel-wise analyses that may respond positively (i.e., increased GMV) to blue light therapy, including the orbitofrontal and prefrontal cortices. For both mTBI recovery and daytime sleepiness, these regions have important implications.
First, decreased thalamic volume has been observed following mTBIs, specifically in individuals with post-mTBI fatigue (89), while increased thalamic volume is positively associated with more rapid recovery from post-mTBI cognitive impairment (90). Second, both decreased thalamic volume and decreased volume in orbitofrontal and prefrontal regions has been observed in individuals with chronic insomnia, sleep loss, daytime fatigue, and daytime sleepiness (91–93). Relatedly, previous studies show that sleep loss is associated with decreases in glucose metabolism in pre-/orbitofrontal regions (94) as well as changes in the upregulation of A1 adenosine receptors which may underpin homeostatic sleep regulation (95). Given the metabolic crisis that accompanies an mTBI (96), it is possible that reduced GMV in these regions may be associated with specifically compromised glucose metabolism and sleep regulation, while increases in volume may either facilitate or be facilitated by improved sleep. Collectively, findings from those studies, as well as our present findings of decreased volume and increased ESS scores at baseline, suggest that post-mTBI sleep disruption and thalamic, orbitofrontal, and prefrontal volume may be closely linked. However, prospective studies are lacking to identify the directionality of this relationship as well as the effect of mTBI on factors associated with sleep regulation (e.g., glucose metabolism) in these areas.
Blue Light Effects on Functional Connectivity
The present work also extends prior findings on the effects of BLT and functional connectivity. Acute exposure to blue light (even as short as 1 min) modulates task-dependent fMRI activation in regions critical to cognitive control, attention, and memory including the anterior cingulate (45) and dorso- and ventrolateral prefrontal cortex (46, 97, 98) as well as the thalamus and anterior insula (97). These effects have also been observed in completely blind individuals with brief (<1 min) exposures to blue light, indicating that these effects are not dependent on visual perception of blue light (99). Task-dependent modulation is also evident for acute blue light exposure during emotion regulation tasks (100). Additionally, and highly relevant to the present work, these acute, task-dependent modulations may be strongly influenced by both circadian phase and sleep pressure (97). Collectively, these prior findings indicate that acute blue light exposure enhances functional activation during cognitive tasks and repeated or prolonged exposure may confer lasting effects. Future work should specifically examine the similarities and differences between acute and repeated blue light exposure on both resting-state and task-dependent activation.
Further, we previously reported increased functional connectivity specifically between the left thalamus and both frontal and parietal cortical areas that was associated with decreased daytime sleepiness following treatment in this population (43). The present findings demonstrate wider influences of blue light therapy on increased coherence between visual, attention, and subcortical gray matter networks, including bilateral thalamocortical connectivity. We further demonstrate greater dissociation between internally and externally oriented networks (i.e., the dorsal vs. ventral attention networks; default mode vs. frontoparietal control networks). Critically, these effects of BLT were associated with observed decreases in daytime sleepiness.
These findings are broadly in line with prior neuroimaging findings linking mTBI and sleep disrupted states. Decreased and disrupted thalamocortical connectivity—including dorsal attention and frontoparietal control networks—has previously been reported in individuals recovering from a mTBI who report fatigue (25, 101, 102). Additional work indicates that poor quality sleep and fatigue is associated with decreased functional connectivity within the default mode network and increased limbic network functional connectivity (including thalamocortical connectivity) in pediatric mTBI (103). Those studies also indicate that connectivity in the limbic and default mode networks normalizes in parallel with the recovery of cognitive function and sleep.
Sleep Treatments Facilitate mTBI Recovery
The present findings agree both with our prior analyses and more broadly with a recent randomized controlled trial investigating exogenous melatonin supplementation for adolescents recovering from a mTBI (38, 43). Findings from that study on melatonin also demonstrated increases in GMV following treatment that was associated with increased sleep quality (specifically, decreased wake after sleep onset as measured with actigraphy), particularly in the posterior cingulate cortex. Changes in WASO were also associated with increased functional connectivity between the default mode network and attention, visual, and somatosensory networks as well as an overall increase in whole brain functional connectivity (38).
While the exact mechanisms by which either blue light therapy or melatonin would alter brain structure and function remain to be fully elucidated, we posit that this is due primarily to effects on sleep, rather than directly the result of treatment. Recent work suggests that sleep is a critical component of synaptic plasticity (104, 105), and sleep is critical for the formation of oligodendrocyte precursor cells, which form the basis of the myelin sheath (106), which is often damaged in mTBI. Both blue light therapy (as a daytime melatonin suppressor) and melatonin supplementation (as a nighttime sleep-promotor) exert potent effects on circadian rhythm and sleep regulation (107–111). Therefore, it is likely that treatment-mediated changes in circadian rhythm and sleep enable plasticity and modulation of resting-state connectivity in the recovery from a mTBI, which may in turn facilitate clinical recovery, in keeping with the observed relationship between clinical outcomes and functional connectivity normalization. Future work is necessary to more conclusively determine the mechanisms by which these effects are observed.
Limitations
There are several limitations that should be noted in interpreting the present findings. First, we had limited capability to fully ensure treatment compliance and dose. Treatment was completed by participants in their home and required them to position the light box within their peripheral vision each day for 6 weeks. However, across both studies self-reported compliance was high (>80% of treatment sessions completed) and the individuals with the lowest compliance were those who had missing or late recording of treatments. These lapses in self-reporting do not necessarily mean that treatment was not completed, nor does high reporting compliance necessarily indicate strict adherence or truthful reporting. The data here were analyzed with an intention-to-treat design, so that all cases with available data were included, regardless of treatment compliance. Future work in this area should endeavor to develop methods for quantitatively and objectively determining light box treatment compliance.
Second, we enrolled participants ranging from 5 to 80 weeks post-injury. Spontaneous recovery from a mTBI has been noted at least up to 12 weeks post-injury (112) and so some changes here may be attributable to post-injury timing. However, the relationship between weeks post-injury and ESS scores was neither strong nor statistically significant and so the relative impact of treatment timing may be small. Third, no clinical reads were performed and so the presence of unidentified brain pathology (e.g., vascular lesions) may have affected the present findings. However, our sample is comprised of young adults all with diagnosed injury no more severe than mild, so we consider the likelihood of this potential confound to be small.
Fourth, this was not a prospective study and we did not include a non-injured control group as a baseline or post-treatment reference. Therefore, we are unable to determine whether BLT brings individuals recovering from a mTBI closer to a pre-injury or uninjured state from a neuroimaging perspective. However, the purpose the trial was neither to establish the effects of mTBIs on brain structure or function (a baseline comparison to self or an uninjured control group) nor to demonstrate that blue light therapy normalizes to a pre-injury or non-injured state. Rather, the overall purpose of the trial was to demonstrate that, in those individuals self-reporting adverse sleep-related outcomes following injury, blue light therapy is a viable treatment alternative for reducing daytime sleepiness and improving sleep (quantity and quality) as we have previously reported (43, 44), with the present findings as secondary outcomes. Future prospective studies should examine the capacity of BLT to minimize both the neurophysiological effects and long-term sleep disruption stemming from mTBIs relative to pre-injury or uninjured states.
Conclusion
For individuals reporting daytime sleepiness following a mild traumatic brain injury, BLT is a non-invasive treatment option that may help to facilitate neural plasticity and hasten recovery. Here, we demonstrated moderate to large increases in both gray matter volume and functional connectivity following blue light therapy in areas previously associated with both sleep regulation and daytime cognitive function, alertness, and attention. Further work is needed to more completely elucidate the exact mechanisms of these changes as well as precision medicine factors that may selectively identify individuals in whom the greatest benefits may be seen. These data add to a growing body of research suggesting that morning BLT may facilitate structural and functional brain recovery among some individuals who have sustained a mTBI.
Data Availability Statement
The raw data supporting the conclusions of this article will be made available by the authors, without undue reservation.
Ethics Statement
The studies involving human participants were reviewed and approved by Partners Healthcare, The University of Arizona, and The U.S. Army Human Protections Office. The patients/participants provided their written informed consent to participate in this study.
Author Contributions
AR conducted the analyses and drafted the initial manuscript. ND revised the manuscript. BF and AA assisted with data collection, study implementation, and manuscript preparation. WDSK designed the study, analyzed the data, and assisted with manuscript preparation and revision. All authors contributed to the article and approved the submitted version.
Funding
This work was funded by multiple grants to WK from the US Army Medical Research and Development Command (USAMRDC) including W81XWH-11-1-0056 and W81XWH-14-1-0571. The content, opinions, interpretations, conclusions, and recommendations are solely the responsibility of the authors and do not necessarily represent the views of Partners Healthcare, the University of Arizona College of Medicine, the Department of Defense, or the US Army Medical Research and Development Command.
Conflict of Interest
The authors declare that the research was conducted in the absence of any commercial or financial relationships that could be construed as a potential conflict of interest.
References
1. Langlois JA, Rutland-Brown W, Wald MM. The epidemiology and impact of traumatic brain injury: a brief overview. J Head Trauma Rehabil. (2006) 21:375–8. doi: 10.1097/00001199-200609000-00001
2. Faul M, Xu L, Wald MM, Coronado VG. Traumatic Brain Injury in the United States: Emergency Department Visits, Hospitalizations and Deaths 2002-2006. Atlanta (GA): Centers for Disease Control and Prevention, National Center for Injury Prevention and Control (2010). Available online at: https://www.cdc.gov/traumaticbraininjury/tbi_ed.html (accessed April 25, 2015). doi: 10.15620/cdc.5571
3. Kerr Z, Roos K, Dompier T, Dalton S, Djoko A, Broglio S, et al. Estimating concussion incidence in college sports: rates, risks, average per team and proportion of teams with concussions. In: Brain Injury. Philadelphia, PA: Taylor & Francis Inc. (2016), p. 504.
4. Defense and Veterans Brain Injury Center. DoD Worldwide TBI Numbers 2000-2018 Q1. (2018). Available online at: https://health.mil/Reference-Center/Publications/2020/09/30/2000-2019-DoD-Worldwide-Numbers-for-TBI (accessed August 27, 2019).
5. Pattinson CL, Meier TB, Guedes VA, Lai C, Devoto C, Haight T, et al. Plasma biomarker concentrations associated with return to sport following sport-related concussion in collegiate athletes—A Concussion Assessment, Research, and Education (CARE) Consortium Study. JAMA Netw Open. (2020) 3:e2013191. doi: 10.1001/jamanetworkopen.2020.13191
6. Master CL, Podolak OE, Ciuffreda KJ, Metzger KB, Joshi NR, McDonald CC, et al. Utility of pupillary light reflex metrics as a physiologic biomarker for adolescent sport-related concussion. JAMA Ophthalmol. (2020) 138:1135–41. doi: 10.1001/jamaophthalmol.2020.3466
7. Meyer J, Bartolomei C, Sauer A, Sajatovic M, Bailey CM. The relationship between fluid biomarkers and clinical outcomes in sports-related concussions: a systematic review. Brain Inj. (2020) 34:1435–45. doi: 10.1080/02699052.2020.1802780
8. Collins MW, Kontos AP, Okonkwo DO, Almquist J, Bailes J, Barisa M, et al. Statements of agreement from the Targeted Evaluation and Active Management (TEAM) approaches to treating concussion meeting held in Pittsburgh, October 15–16, 2015. Neurosurgery. (2016) 79:912–29. doi: 10.1227/NEU.0000000000001447
9. Kontos AP, Sufrinko A, Sandel N, Emami K, Collins MW. Sport-related concussion clinical profiles: clinical characteristics, targeted treatments, and preliminary evidence. Curr Sports Med Rep. (2019) 18:82–92. doi: 10.1249/JSR.0000000000000573
10. Garcia G-GP, Lavieri MS, Jiang R, McAllister TW, McCrea MA, Broglio SP. A data-driven approach to unlikely, possible, probable, and definite acute concussion assessment. J Neurotrauma. (2018) 36:1571–83. doi: 10.1089/neu.2018.6098
11. Kontos AP, Collins MW, Holland CL, Reeves VL, Edelman K, Benso S, et al. Preliminary evidence for improvement in symptoms, cognitive, vestibular, and oculomotor outcomes following targeted intervention with chronic mTBI patients. Mil Med. (2018) 183:333–8. doi: 10.1093/milmed/usx172
12. Dobney DM, Grilli L, Kocilowicz H, Beaulieu C, Straub M, Friedman D, et al. Evaluation of an active rehabilitation program for concussion management in children and adolescents. Brain Inj. (2017) 31:1753–9. doi: 10.1080/02699052.2017.1346294
13. Dobney DM, Miller MB, Tufts E. Non-pharmacological rehabilitation interventions for concussion in children: a scoping review. Disabil Rehabil. (2019) 41:727–39. doi: 10.1080/09638288.2017.1400595
14. Leddy JJ, Haider MN, Ellis MJ, Mannix R, Darling SR, Freitas MS, et al. Early subthreshold aerobic exercise for sport-related concussion: a randomized clinical trial. JAMA Pediatr. (2019) 173:319–25. doi: 10.1001/jamapediatrics.2018.4397
15. Mantua J, Grillakis A, Mahfouz SH, Taylor MR, Brager AJ, Yarnell AM, et al. A systematic review and meta-analysis of sleep architecture and chronic traumatic brain injury. Sleep Med Rev. (2018) 41:61–77. doi: 10.1016/j.smrv.2018.01.004
16. Theadom A, Cropley M, Parmar P, Barker-Collo S, Starkey N, Jones K, et al. Sleep difficulties one year following mild traumatic brain injury in a population-based study. Sleep Med. (2015) 16:926–32. doi: 10.1016/j.sleep.2015.04.013
17. Farrell-Carnahan L, Franke L, Graham C, McNamee S. Subjective sleep disturbance in veterans receiving care in the veterans affairs polytrauma system following blast-related mild traumatic brain injury. Mil Med. (2013) 178:951–6. doi: 10.7205/MILMED-D-13-00037
18. Farrell-Carnahan L, Barnett S, Lamberty G, Hammond FM, Kretzmer TS, Franke LM, et al. Insomnia symptoms and behavioural health symptoms in veterans 1 year after traumatic brain injury. Brain Inj. (2015) 29:1400–8. doi: 10.3109/02699052.2015.1063161
19. Wiseman-Hakes C, Gosselin N, Sharma B, Langer L, Gagnon I. A longitudinal investigation of sleep and daytime wakefulness in children and youth with concussion. ASN Neuro. (2019) 11:1759091418822405. doi: 10.1177/1759091418822405
20. Brooks BL, Sayers P, Virani S, Rajaram A, Tomfohr L. Insomnia in adolescents with slow recovery from concussion. J Neurotrauma. (2019) 36:2391–9. doi: 10.1089/neu.2018.6257
21. Ouellet M-C, Beaulieu-Bonneau S, Morin CM. Insomnia in patients with traumatic brain injury: frequency, characteristics, and risk factors. J Head Trauma Rehabil. (2006) 21:199–212. doi: 10.1097/00001199-200605000-00001
22. Williams BR, Lazic SE, Ogilvie RD. Polysomnographic and quantitative EEG analysis of subjects with long-term insomnia complaints associated with mild traumatic brain injury. Clin Neurophysiol. (2008) 119:429–38. doi: 10.1016/j.clinph.2007.11.003
23. Raikes AC, Schaefer SY. Sleep quantity and quality during acute concussion: a pilot study. Sleep. (2016) 39:2141–7. doi: 10.5665/sleep.6314
24. Hoffman NL, O'Connor PJ, Schmidt MD, Lynall RC, Schmidt JD. Differences in sleep between concussed and nonconcussed college students: a matched case–control study. Sleep. (2018) 42:1–11. doi: 10.1093/sleep/zsy222
25. Nordin LE, Möller MC, Julin P, Bartfai A, Hashim F, Li T-Q. Post mTBI fatigue is associated with abnormal brain functional connectivity. Sci Rep. (2016) 6:21183. doi: 10.1038/srep21183
26. Combs PR, Ford CB, Campbell KR, Carneiro KA, Mihalik JP. Influence of self-reported fatigue and sex on baseline concussion assessment scores. Orthop J Sports Med. (2019) 7:2325967118817515. doi: 10.1177/2325967118817515
27. Raikes AC, Athey A, Alfonso-Miller P, Killgore WDS, Grandner MA. Insomnia and daytime sleepiness: risk factors for sports-related concussion. Sleep Med. (2019) 58:66–74. doi: 10.1016/j.sleep.2019.03.008
28. Sullivan KA, Blaine H, Kaye S-A, Theadom A, Haden C, Smith SS. A systematic review of psychological interventions for sleep and fatigue after mild traumatic brain injury. J Neurotrauma. (2017) 35:195–209. doi: 10.1089/neu.2016.4958
29. Ludwig R, D'Silva L, Vaduvathiriyan P, Rippee MA, Siengsukon C. Sleep disturbances in the acute stage of concussion are associated with poorer long-term recovery: a systematic review. PM&R. (2020) 12:500–11. doi: 10.1002/pmrj.12309
30. Chung JS, Zynda AJ, Didehbani N, Hicks C, Hynan LS, Miller SM, et al. Association between sleep quality and recovery following sport-related concussion in pediatrics. J Child Neurol. (2019) 34:639–45. doi: 10.1177/0883073819849741
31. Howell DR, Oldham JR, Brilliant AN, Meehan WP. Trouble falling asleep after concussion is associated with higher symptom burden among children and adolescents. J Child Neurol. (2019) 34:256–61. doi: 10.1177/0883073818824000
32. Oyegbile TO, Dougherty A, Tanveer S, Zecavati N, Delasobera BE. High sleep disturbance and longer concussion duration in repeat concussions. Behav Sleep Med. (2020) 18:241–8. doi: 10.1080/15402002.2019.1578223
33. Bloomfield ILM, Espie CA, Evans JJ. Do sleep difficulties exacerbate deficits in sustained attention following traumatic brain injury? J Int Neuropsychol Soc. (2010) 16:17–25. doi: 10.1017/S1355617709990798
34. Mantua J, Mahan KM, Henry OS, Spencer RMC. Altered sleep composition after traumatic brain injury does not affect declarative sleep-dependent memory consolidation. Front Hum Neurosci. (2015) 9:328. doi: 10.3389/fnhum.2015.00328
35. Mantua J, Henry OS, Garskovas NF, Spencer RMC. Mild traumatic brain injury chronically impairs sleep- and wake-dependent emotional processing. Sleep. (2017) 40:zsx062. doi: 10.1093/sleep/zsx062
36. Kostyun RO, Milewski MD, Hafeez I. Sleep disturbance and neurocognitive function during the recovery from a sport-related concussion in adolescents. Am J Sports Med. (2015) 43:633–40. doi: 10.1177/0363546514560727
37. Sufrinko A, Pearce K, Elbin RJ, Covassin T, Johnson E, Collins M, et al. The effect of preinjury sleep difficulties on neurocognitive impairment and symptoms after sport-related concussion. Am J Sports Med. (2015) 43:830–8. doi: 10.1177/0363546514566193
38. Iyer KK, Zalesky A, Cocchi L, Barlow KM. Neural correlates of sleep recovery following melatonin treatment for pediatric concussion: a randomized controlled trial. J Neurotrauma. (2020) 37:2647–55. doi: 10.1089/neu.2020.7200
39. Jha A, Weintraub A, Allshouse A, Morey C, Cusick C, Kittelson J, et al. A randomized trial of modafinil for the treatment of fatigue and excessive daytime sleepiness in individuals with chronic traumatic brain injury. J Head Trauma Rehabil. (2008) 23:52. doi: 10.1097/01.HTR.0000308721.77911.ea
40. Lequerica A, Jasey N, Portelli Tremont JN, Chiaravalloti ND. Pilot study on the effect of ramelteon on sleep disturbance after traumatic brain injury: preliminary evidence from a clinical trial. Arch Phys Med Rehabil. (2015) 96:1802–9. doi: 10.1016/j.apmr.2015.05.011
41. Killgore WDS. Lightening the mood: evidence for blue light exposure in the treatment of post-concussion depression. Expert Rev Neurother. (2020) 20:1081–3. doi: 10.1080/14737175.2020.1814147
42. Sinclair KL, Ponsford JL, Rajaratnam SM. Actigraphic assessment of sleep disturbances following traumatic brain injury. Behav Sleep Med. (2014) 12:13–27. doi: 10.1080/15402002.2012.726203
43. Killgore WDS, Vanuk JR, Shane BR, Weber M, Bajaj S. A randomized, double-blind, placebo-controlled trial of blue wavelength light exposure on sleep and recovery of brain structure, function, and cognition following mild traumatic brain injury. Neurobiol Dis. (2020) 134:104679. doi: 10.1016/j.nbd.2019.104679
44. Raikes AC, Dailey NS, Shane BR, Forbeck B, Alkozei A, Killgore WDS. Daily morning blue light therapy improves daytime sleepiness, sleep quality, and quality of life following a mild traumatic brain injury. J Head Trauma Rehabil. (2020) 35:E405–21. doi: 10.1097/HTR.0000000000000579
45. Alkozei A, Smith R, Killgore WDS. Exposure to blue wavelength light modulates anterior cingulate cortex activation in response to ‘uncertain' versus ‘certain' anticipation of positive stimuli. Neurosci Lett. (2016) 616:5–10. doi: 10.1016/j.neulet.2016.01.034
46. Alkozei A, Smith R, Pisner DA, Vanuk JR, Berryhill SM, Fridman A, et al. Exposure to blue light increases subsequent functional activation of the prefrontal cortex during performance of a working memory task. Sleep. (2016) 39:1671–80. doi: 10.5665/sleep.6090
47. Killgore WDS, Dailey NS, Raikes AC, Vanuk JR, Taylor E, Alkozei A. Blue light exposure enhances neural efficiency of the task positive network during a cognitive interference task. Neurosci Lett. (2020) 735:135242. doi: 10.1016/j.neulet.2020.135242
48. Alkozei A, Smith R, Dailey NS, Bajaj S, Killgore WDS. Acute exposure to blue wavelength light during memory consolidation improves verbal memory performance. PLoS ONE. (2017) 12:e0184884. doi: 10.1371/journal.pone.0184884
49. Bajaj S, Vanuk JR, Smith R, Dailey NS, Killgore WDS. Blue-light therapy following mild traumatic brain injury: effects on white matter water diffusion in the brain. Front Neurol. (2017) 8:616. doi: 10.3389/fneur.2017.00616
50. Raikes AC, Satterfield BC, Killgore WDS. Evidence of actigraphic and subjective sleep disruption following mild traumatic brain injury. Sleep Med. (2019) 54:62–9. doi: 10.1016/j.sleep.2018.09.018
51. Management of Concussion/mTBI Working Group. VA/DoD Clinical Practice Guideline for Management of Concussion-Mild Traumatic Brain Injury. (2016). Available online at: https://www.healthquality.va.gov/guidelines/Rehab/mtbi/mTBICPGFullCPG50821816.pdf (accessed July 27, 2018).
52. Bogner J, Corrigan JD. Reliability and predictive validity of the Ohio State University TBI identification method with prisoners. J Head Trauma Rehabil. (2009) 24:279. doi: 10.1097/HTR.0b013e3181a66356
53. Corrigan JD, Bogner J. Initial reliability and validity of the Ohio State University TBI identification method. J Head Trauma Rehabil. (2007) 22:318. doi: 10.1097/01.HTR.0000300227.67748.77
54. Schaefer A, Kong R, Gordon EM, Laumann TO, Zuo X-N, Holmes AJ, et al. Local-global parcellation of the human cerebral cortex from intrinsic functional connectivity MRI. Cereb Cortex. (2018) 28:3095–114. doi: 10.1093/cercor/bhx179
55. Fan L, Li H, Zhuo J, Zhang Y, Wang J, Chen L, et al. The human brainnetome atlas: a new brain atlas based on connectional architecture. Cereb Cortex. (2016) 26:3508–26. doi: 10.1093/cercor/bhw157
56. Buckner RL, Krienen FM, Castellanos A, Diaz JC, Yeo BTT. The organization of the human cerebellum estimated by intrinsic functional connectivity. J Neurophysiol. (2011) 106:2322–45. doi: 10.1152/jn.00339.2011
57. Mohajer B, Abbasi N, Mohammadi E, Khazaie H, Osorio RS, Rosenzweig I, et al. Gray matter volume and estimated brain age gap are not linked with sleep-disordered breathing. Hum Brain Mapp. (2020) 41:3034–44. doi: 10.1002/hbm.24995
58. Yeo BTT, Krienen FM, Sepulcre J, Sabuncu MR, Lashkari D, Hollinshead M, et al. The organization of the human cerebral cortex estimated by intrinsic functional connectivity. J Neurophysiol. (2011) 106:1125–65. doi: 10.1152/jn.00338.2011
59. Esteban O, Markiewicz CJ, Blair RW, Moodie CA, Isik AI, Erramuzpe A, et al. fMRIPrep: a robust preprocessing pipeline for functional MRI. Nat Methods. (2019) 16:111. doi: 10.1038/s41592-018-0235-4
60. Esteban O, Blair R, Markiewicz CJ, Berleant SL, Moodie C, Ma F, et al. fMRIPrep: A Robust Preprocessing Pipeline for Functional MRI. Zenodo (2019). doi: 10.1101/306951
61. Gorgolewski K, Burns CD, Madison C, Clark D, Halchenko YO, Waskom ML, et al. Nipype: a flexible, lightweight and extensible neuroimaging data processing framework in python. Front Neuroinform. (2011) 5:13. doi: 10.3389/fninf.2011.00013
62. Esteban O, Markiewicz CJ, Burns C, Goncalves M, Jarecka D, Ziegler E, et al. nipy/nipype: 1.5.1. Zenodo (2020).
63. Tustison NJ, Avants BB, Cook PA, Zheng Y, Egan A, Yushkevich PA, et al. N4ITK: improved N3 bias correction. IEEE Trans Med Imaging. (2010) 29:1310–20. doi: 10.1109/TMI.2010.2046908
64. Avants BB, Epstein CL, Grossman M, Gee JC. Symmetric diffeomorphic image registration with cross-correlation: evaluating automated labeling of elderly and neurodegenerative brain. Med Image Anal. (2008) 12:26–41. doi: 10.1016/j.media.2007.06.004
65. Zhang Y, Brady M, Smith S. Segmentation of brain MR images through a hidden Markov random field model and the expectation-maximization algorithm. IEEE Trans Med Imaging. (2001) 20:45–57. doi: 10.1109/42.906424
66. Reuter M, Rosas HD, Fischl B. Highly accurate inverse consistent registration: a robust approach. NeuroImage. (2010) 53:1181–96. doi: 10.1016/j.neuroimage.2010.07.020
67. Dale AM, Fischl B, Sereno MI. Cortical surface-based analysis: I. Segmentation and surface reconstruction. NeuroImage. (1999) 9:179–94. doi: 10.1006/nimg.1998.0395
68. Klein A, Ghosh SS, Bao FS, Giard J, Häme Y, Stavsky E, et al. Mindboggling morphometry of human brains. PLoS Comput Biol. (2017) 13:e1005350. doi: 10.1371/journal.pcbi.1005350
69. Fonov V, Evans A, McKinstry R, Almli C, Collins D. Unbiased nonlinear average age-appropriate brain templates from birth to adulthood. NeuroImage. (2009) 47:S102. doi: 10.1016/S1053-8119(09)70884-5
70. Evans AC, Janke AL, Collins DL, Baillet S. Brain templates and atlases. NeuroImage. (2012) 62:911–22. doi: 10.1016/j.neuroimage.2012.01.024
71. Jenkinson M, Bannister P, Brady M, Smith S. Improved optimization for the robust and accurate linear registration and motion correction of brain images. NeuroImage. (2002) 17:825–41. doi: 10.1006/nimg.2002.1132
72. Cox RW, Hyde JS. Software tools for analysis and visualization of fMRI data. NMR Biomed. (1997) 10:171–8
73. Glasser MF, Sotiropoulos SN, Wilson JA, Coalson TS, Fischl B, Andersson JL, et al. The minimal preprocessing pipelines for the Human Connectome Project. NeuroImage. (2013) 80:105–24. doi: 10.1016/j.neuroimage.2013.04.127
74. Greve DN, Fischl B. Accurate and robust brain image alignment using boundary-based registration. NeuroImage. (2009) 48:63–72. doi: 10.1016/j.neuroimage.2009.06.060
75. Power JD, Mitra A, Laumann TO, Snyder AZ, Schlaggar BL, Petersen SE. Methods to detect, characterize, and remove motion artifact in resting state fMRI. NeuroImage. (2014) 84:320–41. doi: 10.1016/j.neuroimage.2013.08.048
76. Behzadi Y, Restom K, Liau J, Liu TT. A component based noise correction method (CompCor) for BOLD and perfusion based fMRI. NeuroImage. (2007) 37:90–101. doi: 10.1016/j.neuroimage.2007.04.042
77. Satterthwaite TD, Elliott MA, Gerraty RT, Ruparel K, Loughead J, Calkins ME, et al. An improved framework for confound regression and filtering for control of motion artifact in the preprocessing of resting-state functional connectivity data. NeuroImage. (2013) 64:240–56. doi: 10.1016/j.neuroimage.2012.08.052
78. Lanczos C. Evaluation of noisy data. J Soc Ind Appl Math Ser B Numerical Anal. (1964) 1:76–85. doi: 10.1137/0701007
79. Abraham A, Pedregosa F, Eickenberg M, Gervais P, Mueller A, Kossaifi J, et al. Machine learning for neuroimaging with scikit-learn. Front Neuroinform. (2014) 8:14. doi: 10.3389/fninf.2014.00014
80. Ciric R, Wolf DH, Power JD, Roalf DR, Baum GL, Ruparel K, et al. Benchmarking of participant-level confound regression strategies for the control of motion artifact in studies of functional connectivity. NeuroImage. (2017) 154:174–87. doi: 10.1016/j.neuroimage.2017.03.020
81. Fortin J-P, Cullen N, Sheline YI, Taylor WD, Aselcioglu I, Cook PA, et al. Harmonization of cortical thickness measurements across scanners and sites. NeuroImage. (2018) 167:104–20. doi: 10.1016/j.neuroimage.2017.11.024
82. R Core Team. R: A Language and Environment for Statistical Computing. Vienna, Austria: R Foundation for Statistical Computing (2018). Available online at: https://www.R-project.org/ (accessed November 30, 2020).
83. Fortin J-P, Parker D, Tunç B, Watanabe T, Elliott MA, Ruparel K, et al. Harmonization of multi-site diffusion tensor imaging data. NeuroImage. (2017) 161:149–70. doi: 10.1016/j.neuroimage.2017.08.047
84. Yu M, Linn KA, Cook PA, Phillips ML, McInnis M, Fava M, et al. Statistical harmonization corrects site effects in functional connectivity measurements from multi-site fMRI data. Hum Brain Mapp. (2018) 39:4213–27. doi: 10.1002/hbm.24241
85. Ho J, Tumkaya T, Aryal S, Choi H, Claridge-Chang A. Moving beyond P values: data analysis with estimation graphics. Nat Methods. (2019) 16:565–6. doi: 10.1101/377978
86. Xia M, Wang J, He Y. BrainNet viewer: a network visualization tool for human brain connectomics. PLoS ONE. (2013) 8:e68910. doi: 10.1371/journal.pone.0068910
87. Johns M, Hocking B. Daytime sleepiness and sleep habits of Australian workers. Sleep. (1997) 20:844–7. doi: 10.1093/sleep/20.10.844
88. Killgore WD, Vanuk JR, Knight SA, Markowski SM, Pisner D, Shane B, et al. Daytime sleepiness is associated with altered resting thalamocortical connectivity. Neuroreport. (2015) 26:779–84. doi: 10.1097/WNR.0000000000000418
89. Clark AL, Sorg SF, Holiday K, Bigler ED, Bangen KJ, Evangelista ND, et al. Fatigue is associated with global and regional thalamic morphometry in veterans with a history of mild traumatic brain injury. J Head Trauma Rehabil. (2018) 33:382–92. doi: 10.1097/HTR.0000000000000377
90. Munivenkatappa A, Devi BI, Shukla DP, Rajeswaran J. Role of the thalamus in natural recovery of cognitive impairment in patients with mild traumatic brain injury. Brain Inj. (2016) 30:388–92. doi: 10.3109/02699052.2015.1089599
91. Altena E, Vrenken H, Werf YDVD, Heuvel OA van den, Someren EJWV. Reduced orbitofrontal and parietal gray matter in chronic insomnia: a voxel-based morphometric study. Biol Psychiatry. (2010) 67:182–5. doi: 10.1016/j.biopsych.2009.08.003
92. Stoffers D, Moens S, Benjamins J, van Tol M-J, Penninx BWHJ, Veltman DJ, et al. Orbitofrontal gray matter relates to early morning awakening: a neural correlate of insomnia complaints? Front Neurol. (2012) 3:105. doi: 10.3389/fneur.2012.00105
93. Dai X-J, Jiang J, Zhang Z, Nie X, Liu B-X, Pei L, et al. Plasticity and susceptibility of brain morphometry alterations to insufficient sleep. Front Psychiatry. (2018) 9:266. doi: 10.3389/fpsyt.2018.00266
94. Thomas M, Sing H, Belenky G, Holcomb H, Mayberg H, Dannals R, et al. Neural basis of alertness and cognitive performance impairments during sleepiness. I. Effects of 24 h of sleep deprivation on waking human regional brain activity. J Sleep Res. (2000) 9:335–52. doi: 10.1046/j.1365-2869.2000.00225.x
95. Elmenhorst D, Meyer PT, Winz OH, Matusch A, Ermert J, Coenen HH, et al. Sleep deprivation increases A1 adenosine receptor binding in the human brain: a positron emission tomography study. J Neurosci. (2007) 27:2410–5. doi: 10.1523/JNEUROSCI.5066-06.2007
96. Giza CC, Hovda DA. The new neurometabolic cascade of concussion. Neurosurgery. (2014) 75:S24–33. doi: 10.1227/NEU.0000000000000505
97. Vandewalle G, Archer SN, Wuillaume C, Balteau E, Degueldre C, Luxen A, et al. Effects of light on cognitive brain responses depend on circadian phase and sleep homeostasis. J Biol Rhythms. (2011) 26:249–59. doi: 10.1177/0748730411401736
98. Vandewalle G, Gais S, Schabus M, Balteau E, Carrier J, Darsaud A, et al. Wavelength-dependent modulation of brain responses to a working memory task by daytime light exposure. Cereb Cortex. (2007) 17:2788–95. doi: 10.1093/cercor/bhm007
99. Vandewalle G, Collignon O, Hull JT, Daneault V, Albouy G, Lepore F, et al. Blue light stimulates cognitive brain activity in visually blind individuals. J Cogn Neurosci. (2013) 25:2072–85. doi: 10.1162/jocn_a_00450
100. Vandewalle G, Schwartz S, Grandjean D, Wuillaume C, Balteau E, Degueldre C, et al. Spectral quality of light modulates emotional brain responses in humans. PNAS. (2010) 107:19549–54. doi: 10.1073/pnas.1010180107
101. Tang L, Ge Y, Sodickson DK, Miles L, Zhou Y, Reaume J, et al. Thalamic resting-state functional networks: disruption in patients with mild traumatic brain injury. Radiology. (2011) 260:831–40. doi: 10.1148/radiol.11110014
102. Banks SD, Coronado RA, Clemons LR, Abraham CM, Pruthi S, Conrad BN, et al. Thalamic functional connectivity in mild traumatic brain injury: longitudinal associations with patient-reported outcomes and neuropsychological tests. Arch Phys Med Rehabil. (2016) 97:1254–61. doi: 10.1016/j.apmr.2016.03.013
103. Iyer KK, Barlow KM, Brooks B, Ofoghi Z, Zalesky A, Cocchi L. Relating brain connectivity with persistent symptoms in pediatric concussion. Ann Clin Transl Neurol. (2019) 6:954–61. doi: 10.1002/acn3.764
104. Puentes-Mestril C, Aton SJ. Linking network activity to synaptic plasticity during sleep: hypotheses and recent data. Front Neural Circuits. (2017) 11:61. doi: 10.3389/fncir.2017.00061
105. Cirelli C, Tononi G. Linking the need to sleep with synaptic function. Science. (2019) 366:189–90. doi: 10.1126/science.aay5304
106. Bellesi M, Pfister-Genskow M, Maret S, Keles S, Tononi G, Cirelli C. Effects of Sleep and wake on oligodendrocytes and their precursors. J Neurosci. (2013) 33:14288–300. doi: 10.1523/JNEUROSCI.5102-12.2013
107. Berson DM, Dunn FA, Takao M. Phototransduction by retinal ganglion cells that set the circadian clock. Science. (2002) 295:1070–3. doi: 10.1126/science.1067262
108. Hattar S, Kumar M, Park A, Tong P, Tung J, Yau K-W, et al. Central projections of melanopsin-expressing retinal ganglion cells in the mouse. J Comp Neurol. (2006) 497:326–49. doi: 10.1002/cne.20970
109. Dewan K, Benloucif S, Reid K, Wolfe LF, Zee PC. Light-induced changes of the circadian clock of humans: increasing duration is more effective than increasing light intensity. Sleep. (2011) 34:593–9. doi: 10.1093/sleep/34.5.593
110. Cajochen C, Kräuchi K, Wirz-Justice A. Role of melatonin in the regulation of human circadian rhythms and sleep. J Neuroendocrinol. (2003) 15:432–7. doi: 10.1046/j.1365-2826.2003.00989.x
111. Williams WP (Trey), McLin DE, Dressman MA, Neubauer DN. comparative review of approved melatonin agonists for the treatment of circadian rhythm sleep-wake disorders. Pharmacotherapy. (2016) 36:1028–41. doi: 10.1002/phar.1822
Keywords: concussion, phototherapy, daytime sleepiness, fatigue, gray matter volume, functional connectivity
Citation: Raikes AC, Dailey NS, Forbeck B, Alkozei A and Killgore WDS (2021) Daily Morning Blue Light Therapy for Post-mTBI Sleep Disruption: Effects on Brain Structure and Function. Front. Neurol. 12:625431. doi: 10.3389/fneur.2021.625431
Received: 03 November 2020; Accepted: 15 January 2021;
Published: 05 February 2021.
Edited by:
Shadab Rahman, Harvard Medical School, United StatesReviewed by:
Elise Maree McGlashan, Monash University, AustraliaMarco Zucconi, San Raffaele Hospital (IRCCS), Italy
Copyright © 2021 Raikes, Dailey, Forbeck, Alkozei and Killgore. This is an open-access article distributed under the terms of the Creative Commons Attribution License (CC BY). The use, distribution or reproduction in other forums is permitted, provided the original author(s) and the copyright owner(s) are credited and that the original publication in this journal is cited, in accordance with accepted academic practice. No use, distribution or reproduction is permitted which does not comply with these terms.
*Correspondence: Adam C. Raikes, cmFpa2VzLnJlc2VhcmNoQGdtYWlsLmNvbQ==