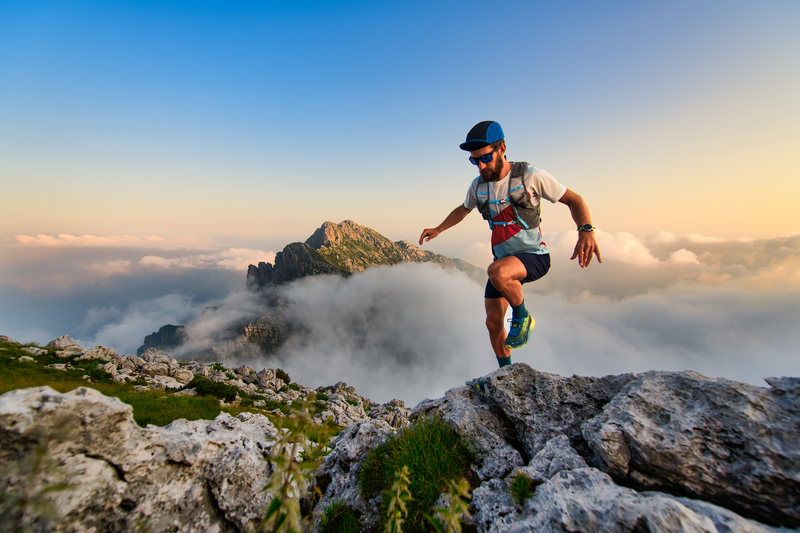
94% of researchers rate our articles as excellent or good
Learn more about the work of our research integrity team to safeguard the quality of each article we publish.
Find out more
ORIGINAL RESEARCH article
Front. Neurol. , 11 February 2021
Sec. Neuroinfectious Diseases
Volume 12 - 2021 | https://doi.org/10.3389/fneur.2021.604984
This article is part of the Research Topic Advances in Understanding NeuroHIV Associated Changes in Neuroimmune Communication in the Combined Anti-retroviral Therapy (cART) Era View all 12 articles
Cognitive impairment remains frequent and heterogeneous in presentation and severity among virally suppressed (VS) women with HIV (WWH). We identified cognitive profiles among 929 VS-WWH and 717 HIV-uninfected women from 11 Women's Interagency HIV Study sites at their first neuropsychological (NP) test battery completion comprised of: Hopkins Verbal Learning Test-Revised, Trail Making, Symbol Digit Modalities, Grooved Pegboard, Stroop, Letter/Animal Fluency, and Letter-Number Sequencing. Using 17 NP performance metrics (T-scores), we used Kohonen self-organizing maps to identify patterns of high-dimensional data by mapping participants to similar nodes based on T-scores and clustering those nodes. Among VS-WWH, nine clusters were identified (entropy = 0.990) with four having average T-scores ≥45 for all metrics and thus combined into an “unimpaired” profile (n = 311). Impaired profiles consisted of weaknesses in: (1) sequencing (Profile-1; n = 129), (2) speed (Profile-2; n = 144), (3) learning + recognition (Profile-3; n = 137), (4) learning + memory (Profile-4; n = 86), and (5) learning + processing speed + attention + executive function (Profile-5; n = 122). Sociodemographic, behavioral, and clinical variables differentiated profile membership using Random Forest models. The top 10 variables distinguishing the combined impaired vs. unimpaired profiles were: clinic site, age, education, race, illicit substance use, current and nadir CD4 count, duration of effective antiretrovirals, and protease inhibitor use. Additional variables differentiating each impaired from unimpaired profile included: depression, stress-symptoms, income (Profile-1); depression, employment (Profile 2); depression, integrase inhibitor (INSTI) use (Profile-3); employment, INSTI use, income, atazanavir use, non-ART medications with anticholinergic properties (Profile-4); and marijuana use (Profile-5). Findings highlight consideration of NP profile heterogeneity and potential modifiable factors contributing to impaired profiles.
Early in the HIV epidemic, people with HIV (PWH) frequently exhibited distinct clinical features including cognitive, behavioral, and motor dysfunction characteristic of a subcortical dementia (1, 2). The clinical syndrome was progressive, severe and included slow mental processing, memory impairment, gait disturbance, tremors, apathy, and depressive symptoms. Since the advent of effective and accessible antiretroviral therapy (ART), PWH are living longer and may be more likely to develop comorbidities that include hypertension, diabetes, cardiovascular disease, chronic liver and renal disease, and malignancies (3, 4). Although it remains unclear as to whether these comorbidities accelerate and/or potentiate CNS dysfunction, different combinations of comorbidities are likely to result in diverse patterns of cognitive function. Thus, in PWH there is a need to understand cognitive profiles and their correlates, including sociodemographic, clinical, and behavioral factors in the context of viral suppression. Cognitive phenotyping in NeuroHIV research may facilitate a better understanding of the underlying pathophysiological mechanisms of each specific cognitive profile.
Several studies using different methodological approaches focus on patterns and predictors of cognitive function in PWH (5–7). Cognitive patterns in PWH were first investigated by Lojek and Bornstein (5), who identified four patterns in 162 predominately White (93%), young (mean age = 34 years), and educated (mean years of education = 14) men at various stages of HIV infection. Using dimension reduction (factor analysis) of seven neuropsychological (NP) outcome metrics from 16 tests followed by k-means clustering, the four profiles consisted of (1) a generally unimpaired group; and weaknesses or impairments in (2) only psychomotor speed, (3) only memory and learning, and (4) most domains. A recent cross-sectional study identified three profiles using five cognitive domain T-scores in a latent profile analysis in almost 3,000 predominately White (69%), educated (mean years of education = 15) men with HIV (MWH; 53%) and without HIV from the Multicenter AIDS Cohort Study (MACS; mean age = 40 years) (7). The three profiles included an unimpaired profile, a profile below average on learning and memory, and a profile below average on all domains. Similarly, three profiles were identified using 10 NP outcome metrics in a latent profile analysis in 361 PWH who were predominately men (88%), actively receiving ART (94%) at the Southern Alberta Clinic (6). Again, an unimpaired profile was identified along with a profile with specific weaknesses in executive function and memory and one with more global NP impairment. Notably, each of these studies focused on all or predominately White, educated MWH and included mixed samples of virological suppressed (VS) and non-suppressed (NVS) individuals. Findings in MWH cannot necessarily be generalized to women with HIV (WWH). WWH may be at greater risk for cognitive impairment due, in part, to a disproportionate burden of poverty, low literacy levels, substance abuse, poor mental health, barriers to health care services, and environmental exposures prevalent in predominantly minority urban communities in which they reside (8, 9). Biological factors, such as sex steroid hormones and female-specific factors (e.g., pregnancy, menopause), may also contribute to the pattern and magnitude of cognitive impairment in PWH (9). Combining samples of NVS and VS individuals introduces heterogeneity in cognitive function and findings from combined samples may not be generalizable to VS-PWH, a population that is expanding with the introduction of increasingly tolerable and available medication options.
As the pattern and predictors of cognitive function are likely not the same in (1) MWH and WWH as well as in (2) VS vs. NVS individuals (9), we examined heterogeneity in NP performance in the largest sample to date of VS-WWH and HIV-uninfected women. We accomplished this by applying novel machine learning methods to identify subgroups who demonstrated similar NP profiles. This approach may help guide our understanding of profiles that are associated with patterns of NP weakness. We also identified factors associated with each profile from a constellation of sociodemographic, behavioral, and clinical factors that have been found to be import distinguishing factors in prior studies (5–7), with the addition of female-specific factors (e.g., pregnancy, menopausal stage) that could not be examined in mixed-sex studies.
The Women's Interagency HIV Study (WIHS) is a multi-center, longitudinal, study of WWH and HIV-uninfected women. The first three waves of study enrollment occurred between October 1994 and November 1995, October 2001 and September 2002, and January 2011 and January 2013 from six sites (Brooklyn, Bronx, Chicago, DC, Los Angeles, and San Francisco). A more recent wave of enrollment occurred at sites in the southern US (Chapel Hill, Atlanta, Miami, Birmingham, and Jackson) between October 2013 and September 2015. Study methodology including recruitment procedures and eligibility criteria, training, and quality assurance procedures were previously published (10–12). This analysis was restricted to all participants completing the first NP test battery. NP data for the initial six sites were collected between 2009 and 2011, while NP data from the southern sites were collected between 2013 and 2015.
The NP test battery included the Hopkins Verbal Learning Test-Revised (HVLT-R; outcomes: trial 1 learning, total learning, delayed free recall, percent retention, recognition), Letter-Number Sequencing (LNS; outcomes: total correct on the working memory and attention conditions), Trail Making Test (TMT; outcomes: time to complete Parts A and B), Stroop (outcome: time to complete Trials 1 [color reading], 2 [color naming], and 3 [color-word]), Symbol Digit Modalities Test (SDMT; outcome: total correct), Letter-guided verbal fluency (Controlled Oral Word Associations Test (COWAT; outcome: total correct words generated across three trials [F, A, S]), Animal fluency (outcome: total correct animals generated), and Grooved Pegboard (GPEG; outcomes: time to completion, dominant, and non-dominant hand). Timed outcomes were log transformed to normalize distributions and reverse scored so higher equated to better performance. Demographically-adjusted T-scores were calculated for each outcome (13, 14). T-scores are normalized to have an average of 50 and a standard deviation of 10. Mean T-scores >55 were considered high performing, between 45 and 55 were considered within the normal range, <45 were considered as weaknesses, and those <40 were considered impaired.
Factors of interest were based on prior NP WIHS studies (13, 14) and included: clinic site; enrollment wave; sociodemographic, mental health, behavioral, clinical, and female-specific factors; and common non-ART medications with known neurocognitive adverse effects (NCAEs) (15, 16). Sociodemographic factors included age, education, WRAT-III reading subscale score, race/ethnicity, employment status, average annual household income (≤$12,000), and health insurance status. Mental health factors included depressive symptoms (Center for Epidemiological Studies Depression scale [CES-D] ≤16]), perceived stress (perceived stress scale [PSS]-10 top tertile cutoff), and post-traumatic stress symptoms (PTSD Checklist—Civilian Scale) (17). Behavioral factors included current smoking status, recent alcohol intake, marijuana, and crack, cocaine, and heroin use. General clinical, metabolic, and cardiovascular factors included Hepatitis C antibody positive, body mass index (BMI), non-ART medication use [e.g., NCAEs, statins, NCAE medications with a higher anticholinergic burden (16)], and history of stroke, hypertension, and diabetes mellitus. Female-specific factors included ever pregnant, history of hysterectomy and/or bilateral oophorectomy, hormonal contraceptive use, hormone therapy use, and menopausal stage [defined using the Study of Women's Health Across the Nation [SWAN] criteria (18) which is also used in previous WIHS studies (19)]. HIV-related clinical factors included HIV RNA, nadir and current CD4+ T lymphocyte count, ART use and adherence, duration of ART use, and previous AIDS diagnosis.
All 17 NP measures were used to find groups of similar cognitive profiles within each participant subset (VS-WWH, HIV-uninfected) utilizing Kohonen self-organizing maps (SOM) followed by clustering with MClust. SOM is an unsupervised machine learning technique used to identify patterns in high dimensional data by producing a two-dimensional grid representation consisting of multiple nodes which have a fixed position in the SOM grid along with associated participants who are mapped to that node. The coordinates of the node represent the similarity to other nodes (i.e., nodes that are closer together in the grid have similar patterns than nodes that are further away) and one node can represent multiple participants. Following the identification of the nodes, the nodes were clustered using the MClust package. Once the clustering of the nodes was completed, cluster profiles were assigned to the participants associated to that node. Profiles where the mean T-Score on all cognitive outcomes was ≥45 were combined into an “unimpaired” profile. By using SOM and MClust in sequence, we were able to achieve fine-tuned clustering based on patterns of NP performance.
Factors associated with profile membership between each impaired profile and the unimpaired profile within each group (VS-WWH, HIV-uninfected) were explored by creating Random Forest (RF) models and then extracting variable importance. The datasets were randomly separated into training (70%) and testing (30%) sets. RF models were created on the training sets using internal validation via a 10-fold resampling method repeated five times. Prior to model creation, the Synthetic Minority Over-sampling Technique (SMOTE) was used to control for bias due to any imbalance in the number of cases. Variables were removed from the model if they had low variance or if they had >30% missing data. Any missing data in the remaining variables was imputed before model creation using RF imputations and ridge regression (α size of 0.0001 for a compromise between stability and lack of bias). For comparison to previous studies we also created RF models for each group comparing the combined unimpaired and impaired profiles. Models were also validated on the testing set to confirm that they still had predictive power balanced between classes and that success of the trained models was not due to overfitting. All variables were plotted by relative variable importance based on the training set models, and attention was given to the top 10 variables in each profile.
All analysis was done using R analysis packages. SOM was achieved using the Kohonen package in R (20) and clustering was done using the MClust package (21). MClust is an R Software package used for model-based clustering using finite normal mixture modeling that provides functions for parameter estimation via the Expectation-Maximization algorithm with an assortment of covariance structures. This program identifies the best model for 10 parameterized covariance structures and chooses the best one based on the lowest Bayesian Information Criterion (BIC). The covariance structures consist of varying distributions (spherical, diagonal, or ellipsoidal), volumes (equal or variable), shapes (equal of variable), and orientation (equal or variable, only for ellipsoidal distribution). Random Forest model creation was achieved using the Caret (22) package in R. SMOTE resampling was done using the DMwR (23) package. Imputation of missing data was done using the Multivariate Imputation by Chained Equations (24) (MICE) package in R. ROC confidence intervals were calculated using the pROC package in R with 2,000 stratified bootstrap replicates (95%CI).
Participants included 929 VS-WWH and 717 HIV-uninfected women at their first study visit with complete NP testing (Supplementary Table 1). On average, participants were 45.1 ± 9.3 years of age with 12.7 years of education. Thirty percent were from the southern WIHS sites, 69% were non-Hispanic Black, and 15% identified as Hispanic. Only 41% were employed and 48% reported having an average annual household income <$12,000/year, while 87% were currently insured. Thirty percent had depressive symptoms while 35% were identified as having higher perceived stress levels. Nineteen percent had recently used marijuana, 7% were currently using crack, cocaine, and/or heroin, and 40% were current smokers. Ninety percent reported ever having been pregnant and 41% were post-menopausal. The average T-score for all NP tests in VS-WWH and HIV- women was in the normal range between 45 and 55 (Supplementary Table 2).
For both VS-WWH and HIV-uninfected women, clusters of participants with similar patterns of relative performance on all 17 NP were profiled using a sequence of SOM and MClust. VS-WWH and HIV-uninfected women had good fits (entropy = 0.99) and were then assigned names based on their relative patterns of weaknesses after consultation with a clinical neuropsychologist. The profiles are visualized in Figure 1 and univariate differences between the test scores, as well as univariate differences in predictor variables, are given in Tables 1, 2 (Supplementary Tables 3, 4).
Figure 1. Neuropsychological profiles in (A) virally suppressed women with HIV(VS-WWH) and (B) HIV-uninfected women. HVLT-R, Hopkins Verbal Learning Test-Revised; LNS, Letter-Number Sequencing; GPEG, Grooved Pegboard.
Table 1. Sociodemographic, clinical, and behavioral factors by subgroup of virally suppressed [VS] women with HIV [WWH].
Table 2. Sociodemographic, clinical, and behavioral factors by subgroup of HIV-uninfected (UN) women.
Profiling of the 929 VS-WWH resulted in nine total clusters using an ellipsoidal multivariate mixture model with equal orientation (VVE) with an entropy of 0.99. Of these clusters, four were combined into a large “unimpaired” cluster consisting of 311 women (Figure 1A; Table 1). Of the remaining clusters:
Profiling of the 1,666 PWH resulted in three total groups from a using an ellipsoidal multivariate mixture model with equal orientation with an entropy of 0.982 (Figure 1A).
- Profile 1-VS (n = 129): sequencing indicated by weaknesses on tests of sequencing (LNS, TMT-Part B). Learning, memory, verbal fluency, processing speed, inhibition, and manual speed were preserved.
- Profile 2-VS (n = 144): speed indicated by weaknesses or impairments on most tests of speed (weak: Stroop, TMT, SDMT, GPEG-dominant hand; impaired: GPEG non-dominant hand). Verbal fluency, attention, working memory, learning, and memory were preserved.
- Profile 3-VS (n = 137): learning and recognition indicated by weak learning (HVLT-R trial 1 and total learning) and recognition (HVLT-R recognition). Retention, verbal fluency, attention, working memory, processing speed, executive functioning, and manual speed were preserved.
- Profile 4-VS (n = 86): learning and memory indicated primarily by impaired memory (HVLT-R delayed recall, recognition, retention) and weak learning (HVLT-R trial 1 and total learning), with mild weaknesses on select speeded measures (Stroop-trial 1, TMT, SDMT). Verbal fluency, attention, working memory, executive functioning, and manual speed were relatively preserved.
- Profile 5-VS (n = 122): learning, processing speed, attention, and executive functioning indicated by impaired and/or weak learning, processing speed, attention, and executive functioning (impaired: COWAT, TMT-Part A; weak: HVLT-R trial 1 and total learning, animal fluency, LNS, SDMT, Stroop-trial 3, TMT-Part B). Manual speed and memory were preserved.
Profiling of the 717 HIV-uninfected women also resulted in nine total clusters (Figure 1B; Table 2) from an ellipsoidal multivariate model with equal volume and orientation (EVE) with an entropy of 0.99. Of these clusters, four did not have mean T-scores that were <45 on any test and were therefore combined into a large “unimpaired” cluster consisting of 400 women. Of the remaining clusters:
- Profile 1-UN (n = 68): visual and motor speed indicated by weaknesses on tests of visual and motor speed (Stroop, TMT, SDMT). Learning, memory, verbal fluency, attention, working memory, and manual speed were preserved.
- Profile 2-UN (n = 58): learning, recall, and verbal fluency indicated primarily by weak learning, recall, and verbal fluency (HVLT-R trial 1, total learning, delayed recall, retention, COWAT, animal fluency). Recognition, processing speed, executive functioning, and manual speed were relatively preserved.
- Profile 3-UN (n = 72): manual speed indicated primarily by impaired manual speed (GPEG) and weak TMT. Learning and memory were spared and all other domains remained relatively preserved.
- Profile 4-UN (n = 75): learning and memory indicated by impaired recall (HVLT-R delayed recall and retention) and weak learning and recognition (HVLT-R trial 1, total learning, and recognition). All other domains were spared.
- Profile 5-UN (n = 44): learning, memory, speed indicated primarily by impaired learning and memory (HVLT-R total learning, delayed recall, and recognition) with impairments or weaknesses on select speeded tests (TMT, GPEG, Stroop-trial 3). Verbal fluency, attention, working memory, and visuo-verbal processing speed (Stroop Trials 1 and 2) were relatively preserved.
For each group of women, a RF model was created to help identify variables contributing in a non-linear fashion to distinguishing between each impaired and the unimpaired profile. An additional model was created to distinguish between all combined impairment profiles and the unimpaired profile in order to compare the differences in variables. For each model, variable importance was calculated and those that ranked as the top 10 were identified.
In RF models (Figure 2), the top 10 variables distinguishing women in the impaired from unimpaired profiles (ROC = 0.91) included clinic site, sociodemographic factors (age, race, education), behavioral (crack, cocaine, and/or heroin use), and clinical factors (BMI, protease inhibitor [PI]-based regimen, current and nadir CD4 count, and years of cART use). Specifically, women in the impaired vs. unimpaired profiles had: a higher minority and southern site representation (non-Hispanic Black, 76 vs. 66%; southern clinics, 36 vs. 32%), less education (12.6 vs. 12.8 years), PI use(70 vs. 60%), healthy BMI of 18.5–24.9 kg/m2 (19 vs. 24%), and ever use of crack, cocaine, and/or heroin (49 vs. 40%). Many of these variables were also important contributors to the individual impairment profiles, although some additional variables were found to be important distinguishers. Compared to the unimpaired profile:
- Profile 1-VS (n = 129): sequencing (ROC = 0.89, Testing Accuracy = 0.89, Testing Sensitivity = 0.84, Testing Specificity = 1.0) were more likely to have a lower annual household income (48 vs. 40%), depressive symptoms (30 vs. 21%), and higher perceived (40 vs. 26%) and post-traumatic stress (25 vs. 13%).
- Profile 2-VS (n = 144): speed (ROC = 0.90, Testing Accuracy = 0.90, Testing Sensitivity = 0.85, Testing Specificity = 1.0) were more likely to be unemployed (71 vs. 51%) and have depressive symptoms (35 vs. 21%).
- Profile 3-VS (n = 137): learning and recognition (ROC = 0.90, Testing Accuracy = 0.88, Testing Sensitivity = 0.83, Testing Specificity = 1.0) were more likely to have depressive symptoms (34 vs. 21%) and be on an integrase inhibitor (INSTI)-based regimen (27 vs. 19%).
- Profile 4-VS (n = 86): learning and memory (ROC = 0.88, Testing Accuracy = 0.89, Testing Sensitivity = 0.87, Testing Specificity = 0.96) were more likely to be unemployed (73 vs. 51%, P<0.001), have a lower annual household income (70 vs. 40%), be on INSTI-based regimen (28 vs. 19%), atazanavir (ATZ, 24 vs. 15%), and non-ART medications with higher anticholinergic burden (22 vs. 11%).
- Profile 5-VS (n = 122): learning, processing speed, and executive function (ROC = 0.91, Testing Accuracy = 0.86, Testing Sensitivity = 0.81, Testing Specificity = 1.0) were less likely to currently use marijuana (15 vs. 20%).
Figure 2. Variable Importance results from the Random Forest Models in virally suppressed women with HIV (VS-WWH). The length of the bars indicates the relative importance of the variable in that model. The top 10 variables in each model have been highlighted in black. 3TC, lamivudine; ABC, abacavir; ART, antiretroviral therapy; ATV, atazanavir; BMI, body mass index; COBI, cobicistat; DRV, darunavir; DTG, dolutegravir; EFV, efavirenz; EVG, elvitegravir; FTC, emtricitabine; INSTI, integrase inhibitor; PI, protease inhibitor; RAL, raltegravir; RPV, rilpivirine; RTV, ritonavir; TDF, tenofovir disoproxil fumarate; ZDV, Zidovudine; NCAE, non-ART medications with neurocognitive adverse effects.
In RF models (Figure 3), the top 10 variables distinguishing the impaired profiles from unimpaired profiles (ROC = 0.93) included: clinic site, cohort wave, sociodemographic factors (age, education, race, employment status), behavioral (smoking, marijuana, crack, cocaine, and/or heroin use), and clinical factors (BMI). Many of these factors were also important contributors to the individual impairment profiles, with some differences. Compared to women in the unimpaired profile:
- Profile 1-UN (n = 68): visual and motor speed (ROC = 0.83, Testing Accuracy = 0.67, Testing Sensitivity = 0.90, Testing Specificity = 0.63) were more likely to have diabetes (22 vs. 16%), depressive symptoms (44 vs. 25%), high perceived stress (43 vs. 34%), use antidepressants (9 vs. 7%), and more non-ART drugs with anticholinergic properties (3 vs. 1%).
- Profile 2-UN (n = 58): learning, recall, and verbal fluency (ROC = 0.72, Testing Accuracy = 0.71, Testing Sensitivity = 1.0, Testing Specificity = 0.67) were more likely to have a lower annual household income (47 vs. 41%), have had an oophorectomy (17 vs. 13%), depressive symptoms (33 vs. 25%), high perceived stress (45 vs. 34%), diabetes (21 vs. 16%), and hypertension (36 vs. 38%).
- Profile 3-UN (n = 72): motor speed (ROC = 0.85, Testing Accuracy = 0.75, Testing Sensitivity = 1.0, Testing Specificity = 0.71) were more likely to have depressive symptoms (47 vs. 25%), perceived stress (47 vs. 34%) and post-traumatic symptoms (28 vs. 19%), HCV (18 vs. 10%), and less likely to have had an oophorectomy (9 vs. 13%).
- Profile 4-UN (n = 75): learning and memory (ROC = 0.79, Testing Accuracy = 0.76, Testing Sensitivity = 0.95, Testing Specificity = 0.73) were less likely to have ever been pregnant (88 vs. 91%).
- Profile 5-UN (n = 44): learning, memory, and speed (ROC = 0.76, Testing Accuracy = 0.73, Testing Sensitivity = 1.0, Testing Specificity = 0.70) were more likely to have diabetes (32 vs. 16%) and hypertension (53 vs. 38%).
Figure 3. Variable Importance results from the Random Forest Models in HIV-uninfected (UN) women. The length of the bars indicates the relative importance of the variable in that model. The top 10 variables in each model have been highlighted in black. BMI, body mass index; NCAE, non-ART medications with neurocognitive adverse effects; OOPH, oopherectomy.
We used machine learning models to identify distinct homogenous subgroups (profiles) in the largest dataset to date in VS-WWH and HIV-uninfected women. Separate patterns of cognitive performance, as well as associated factors of those patterns among each subgroup of women, were identified. The factors identified allow for screening and intervention, including potentially changing non-ART medications, as well as mental health and substance use screening and intervention.
In the context of viral suppression, we identified several profiles with distinct patterns of performance across 17 NP outcomes. While these profiles are statistically-derived, some of the profiles found here parallel commonly identified patterns in other neurological conditions or processes. Among the virally suppressed group, Profile 1-VS revealed a unique pattern reflecting exclusive weaknesses in cognitive sequencing (LNS Attention and Working Memory) and motor set-shifting (TMT-Part B). While to our knowledge, this combination of isolated deficits in cognitive sequencing and motor set-shifting has not been appreciated in other disease populations, specific deficits in cognitive sequencing/verbal working memory have been observed in individuals with schizophrenia and their first-degree relatives (25). Additionally, McDonald et al. (26) identified specific problems with motor set-shifting (TMT-Part B) in individuals with frontal lobe epilepsy. In contrast to the very specific weaknesses identified in Profile 1-VS, Profile 2-VS reflects general slowing, which is most often associated with typical (i.e., “healthy”) aging (27). Profile 3-VS, characterized by poor encoding and recognition with intact retention, is more of a typical HIV-associated profile (28) compared to mild cognitive impairment due to Alzheimer's disease (AD) (29). Profile 4-VS showed a mostly amnestic profile with some evidence of cognitive slowing, as can be observed in AD or in AD with vascular contributions (30). This profile is similar to Profile 4-UN, which reflected an amnestic profile that is often observed in typical AD (31). Profile 5-VS, showing intact memory storage and manual speed/dexterity, but weak or impaired attention, processing speed, learning, and executive functioning is similar to what is observed in individuals with diffuse frontal-subcortical small vessel disease (32). Interestingly, a profile did not emerge among VS-WWH reflecting specific motor slowing which has been linked to HIV infection. This is consistent with prior cross-sectional WIHS analyses, where motor slowing was not a prominent feature among WWH but rather verbal learning and memory (13).
Among the seronegative group, Profile1-UN was more likely to have diabetes, raising the possibility that their specific visual and motor deficits could be related to physical complications of diabetes, including diabetic retinopathy and neuropathy (33, 34). Profile2-UN reflects unique impairments on the most verbally mediated tasks (i.e., verbal learning and recall, and verbal fluencies). While we are unaware of any specific disease process or syndrome that shows the same pattern, this group of individuals has clear weakness in verbal skills, which could be due to many factors, including learning differences or damage to brain regions associated with verbally mediated tasks. Profile 3-UN, reflecting specific motor slowing, is commonly observed in individuals with basal ganglia dysfunction, such as Parkinson's disease (35). Profile 5-UN, revealing rather generalized cognitive weaknesses or impairments, but relatively preserved attention and visual processing, does not reflect any specific disease process or syndrome to our knowledge.
Even though the HIV group was virally suppressed, the dominant profiles did not fully align with the HIV-uninfected women, suggesting that HIV affects cognitive function even in the era of effective ART. There is a wealth of literature postulating neuronal damage as a result of ART agents (36), a viral reservoir that persists possibly due to poor CNS penetration of ART (37), or even legacy effects of damage occurring earlier during infection (38). Indeed, in the VS-WWH RF model where all impaired groups were grouped together, nadir CD4 was a top predictor of group membership. This also points to how existing studies that consider impairment to be a unidimensional construct may only be able to detect differences in these variables and miss those associated more strongly with some profiles than others.
Despite different cognitive profiles among VS-WWH, the most discriminative factors between each impaired profile vs. the unimpaired profile were similar and included a number of well-established sociodemographic cognitive correlates, such as years of education, age, and race/ethnicity (39). Clinic site location also emerged, a factor that we have also seen using more standard statistical approaches in the WIHS (13, 14). The factors underlying this rather robust association is unknown but may involve neighborhood factors, such as violence and food insecurity. Additionally, common behavioral correlates of cognition emerged including illicit substance use (40, 41), which in the case of marijuana was more likely to be used in the unimpaired profile compared to the impaired profile demonstrating weaknesses in learning, processing speed, and executive function (Profile 5-VS). This finding is consistent with some studies demonstrating the protective effects of marijuana use on cognition in PWH (42). We also found common clinical correlates of cognition that distinguished cognitive profiles among VS-WWH including BMI (43, 44) and PI use (45). Likely proxies of HIV disease burden, including nadir CD4 count and years of ART use, were also discriminators (38, 46, 47). In contrast, sociodemographic and medical variables were unable to distinguish cognitive profiles based on seven major cognitive domains (48).
Mental health factors also emerged as important profile discriminators among VS-WWH, including depressive and stress-related symptoms. Depressive symptoms differentiated a number of impaired profiles (4 of 5 profiles) compared to the unimpaired profile, whereas stress-related symptoms only emerged for two profiles including Profile 1-VS (sequencing) and Profile 4-VS (learning and memory). These findings align with our WIHS studies demonstrating numerous cognitive correlates of depressive symptoms (19, 49, 50), whereas stress-related symptoms related most strongly to learning and memory in the context of HIV (49, 51). Importantly, mental health factors are an unmet medical need and are modifiable targets to improve cognition in WWH (52).
INSTI use discriminated both Profile3-VS (learning and recognition) and Profile 4-VS (learning and memory) from the unimpaired profile. This finding is consistent with a number of recently published studies indicating INSTI use as a contributor to NP function. One study demonstrated an association between INSTI use and poorer learning and memory but not any other cognitive domains (53). A second study also demonstrated that switching or starting an INSTI was primarily associated with poorer learning among WWH (54). A third study demonstrated that long-term INSTI exposure distinguished two impaired profiles from an unimpaired profile (55).
Our study also allows us to investigate female-specific factors that are often ignored and identify the importance of oophorectomy and/or hysterectomy (Profile2-UN[Learning, Recall, Verbal Fluency], and Profile3-UN[Motor Speed]) and menopause status (Profile4-UN[Learning and Memory]). Interestingly, these female-specific factors only emerged as important profile discriminators among HIV-uninfected women. As the proportion of menopause-inducing and non-inducing oophorectomy and/or hysterectomy was similar across VS-WWH and HIV-uninfected women, one possible explanation is that the virus itself and clinical factors, such as ART may explain more of the variance in cognitive function in VS-WWH. However, in the absence of HIV, negative effects of oophorectomy and/or hysterectomy on cognition may become more apparent. Overall, these female-specific factors are potential contributions that are missed in other studies, which are predominately male. Future studies of women should evaluate these variables in a similar stratified form to identify potential mechanistic contributions.
The existence of distinctive patterns of cognitive performance, as well as distinct factors associated with those patterns, also adds to existing evidence of differing neuropathological mechanisms. The dominant profiles often contained patterns of weaknesses that were subclinical, yet still lower than the unimpaired profiles. In many cases, the associated factors are intervenable and should be followed up with mechanistic and longitudinal studies.
Differences between the profiles identified here and previous efforts to identify cognitive patterns can be attributed to both the methods used and the study population. To identify meaningful cognitive patterns, we used a combination of SOM and MClust, which is a slight deviation from tradition k-means clustering. The nature of k-means is that it yields clusters where the most dramatic differences are shown, which may ignore subtle differences in patterns. Even Molsberry et al. (7) and Amusan et al. (55) who used latent profile analysis using domain T-scores had their fit dominated by a high-performing and a low-performing group. Using SOM for dimension reduction on the T-scores for individual tests prevented us from following pre-conceived notions about the latent structures of cognitive domains, which have been shown to be different in HIV (56). Another reason that we may have found different profiles than prior studies is that we focused on a diverse sample of underserved lower-income, African-American and Hispanic WWH where social correlates of health are common (e.g., low educational attainment, poverty, food insecurity, etc.) (8, 57) and may lead to more heterogeneous patterns of cognitive function. Of importance, this demographic is a more accurate reflection of the HIV epidemic as opposed to the predominantly White populations evaluated in other cohorts.
The addition of machine learning models to traditional univariate statistics to identify dominating predictor variables is another distinguishing aspect of the current study. It is important to point out that RF modeling is a non-linear model, and that the variable importance measure does not take into account directionality. Therefore, it is possible to have a top predictor variable from RF that does not have P < 0.05 using a t-test. RF models are also multivariate; instead of, the predictive capabilities of variables are always observed within the context of other variables. This is important considering that none of these factors exist in isolation. This makes the model more powerful, but one limitation of this statistical approach is that it becomes more difficult to interpret and should be used as a springboard for more mechanistic studies and interventions, which is why machine learning models are often thought of as “hypothesis-generating” models.
In conclusion, in the largest sample of women to date in the United States, we have used a novel pipeline of machine learning methods to identify subgroups of patterns in NP performance and created predictive models to identify the factors that distinguish each pattern from an overall “unimpaired” group. We identified distinct patterns of cognitive weaknesses in VS-WWH that differed from the distinct patterns in HIV-uninfected women. We also identified factors that may contribute to these specific profiles as a springboard for mechanistic or interventional studies. Future studies should also investigate the stability of these profiles over time, and identify the ones, if any, that are prone to future decline.
Data from our study are available upon request to the MACS/WIHS Combined Cohort Study (MWCCS; https://statepi.jhsph.edu/mwccs/). This committee may be contacted via email at https://statepi.jhsph.edu/mwccs/contact-us/.
The studies involving human participants were reviewed and approved by Institutional Review Board. The patients/participants provided their written informed consent to participate in this study.
LR has primary responsibility for final content and conceived the study idea. RD conducted the statistical analyses. RD, AB, and LR wrote the paper. All authors contributed to manuscript editing and statistical review, read, and approved the final manuscript.
This work was supported by the Johns Hopkins University NIMH Center for novel therapeutics for HIV-associated cognitive disorders (P30MH075773) 2018 pilot award to LR and Johns Hopkins University Center for AIDS Research (CFAR) Scholar Award (P30AI094189 and R03MH123290-02). Data in this manuscript were collected by the Women's Interagency HIV Study, now the MACS/WIHS Combined Cohort Study (MWCCS). The contents of this publication are solely the responsibility of the authors and do not represent the official views of the National Institutes of Health (NIH). MWCCS (Principal Investigators): Atlanta CRS (Ighovwerha Ofotokun, Anandi Sheth, and Gina Wingood), U01-HL146241; Baltimore CRS (Todd Brown and Joseph Margolick), U01-HL146201; Bronx CRS (KA and Anjali Sharma), U01-HL146204; Brooklyn CRS (DG and Tracey Wilson), U01-HL146202; Data Analysis and Coordination Center (Gypsyamber D'Souza, Stephen Gange, and Elizabeth Golub), U01-HL146193; Chicago-Cook County CRS (Mardge Cohen and Audrey French), U01-HL146245; Chicago-Northwestern CRS (Steven Wolinsky), U01-HL146240; Connie Wofsy Women's HIV Study, Northern California CRS (Bradley Aouizerat and Phyllis Tien), U01-HL146242; Los Angeles CRS (Roger Detels), U01-HL146333; Metropolitan Washington CRS (Seble Kassaye and Daniel Merenstein), U01-HL146205; Miami CRS (Maria Alcaide, Margaret Fischl, and Deborah Jones), U01-HL146203; Pittsburgh CRS (Jeremy Martinson and Charles Rinaldo), U01-HL146208; UAB-MS CRS (Mirjam-Colette Kempf and Deborah Konkle-Parker), U01-HL146192; UNC CRS (AA), U01-HL146194. The MWCCS was funded primarily by the National Heart, Lung, and Blood Institute (NHLBI), with additional co-funding from the Eunice Kennedy Shriver National Institute of Child Health & Human Development (NICHD), National Human Genome Research Institute (NHGRI), National Institute on Aging (NIA), National Institute of Dental & Craniofacial Research (NIDCR), National Institute of Allergy and Infectious Diseases (NIAID), National Institute of Neurological Disorders and Stroke (NINDS), National Institute of Mental Health (NIMH), National Institute on Drug Abuse (NIDA), National Institute of Nursing Research (NINR), National Cancer Institute (NCI), National Institute on Alcohol Abuse and Alcoholism (NIAAA), National Institute on Deafness and Other Communication Disorders (NIDCD), National Institute of Diabetes and Digestive and Kidney Diseases (NIDDK). MWCCS data collection was also supported by UL1-TR000004 (UCSF CTSA), P30-AI-050409 (Atlanta CFAR), P30-AI-050410 (UNC CFAR), and P30-AI-027767.
The authors declare that the research was conducted in the absence of any commercial or financial relationships that could be construed as a potential conflict of interest.
We would also like to thank Kendra Radtke, Pharm.D. and Bani Tamraz, Pharm.D., Ph.D. for their work in coding all of the non-ART medications and to the study participants, for without them this work would not be possible.
The Supplementary Material for this article can be found online at: https://www.frontiersin.org/articles/10.3389/fneur.2021.604984/full#supplementary-material
1. Navia BA, Jordan BD, Price RW. The AIDS dementia complex: I. Clinical features. Ann Neurol. (1986) 19:517–24. doi: 10.1002/ana.410190602
2. McArthur JC, Brew BJ, Nath A. Neurological complications of HIV infection. Lancet Neurol. (2005) 4:543–55. doi: 10.1016/S1474-4422(05)70165-4
3. Vance DE, Fazeli PL, Dodson JE, Ackerman M, Talley M, Appel SJ. The synergistic effects of HIV, diabetes, and aging on cognition: implications for practice and research. J Neurosci Nurs. (2014) 46:292–305. doi: 10.1097/JNN.0000000000000074
4. Stoff DM, Goodkin K, Jeste D, Marquine M. Redefining aging in HIV infection using phenotypes. Curr HIV/AIDS Rep. (2017) 14:184–99. doi: 10.1007/s11904-017-0364-x
5. Lojek E, Bornstein RA. The stability of neurocognitive patterns in HIV infected men: classification considerations. J Clin Exp Neuropsychol. (2005) 27:665–82. doi: 10.1081/13803390490918426
6. Gomez D, Power C, Gill MJ, Koenig N, Vega R, Fujiwara E. Empiric neurocognitive performance profile discovery and interpretation in HIV infection. J Neurovirol. (2018) 25:72–84. doi: 10.1007/s13365-018-0685-6
7. Molsberry SA, Cheng Y, Kingsley L, Jacobson L, Levine AJ, Martin E, et al. Neuropsychological phenotypes among men with and without HIV disease in the multicenter AIDS cohort study. AIDS. (2018) 32:1679–88. doi: 10.1097/QAD.0000000000001865
8. Maki PM, Martin-Thormeyer E. HIV, cognition and women. Neuropsychol Rev. (2009) 19:204–14. doi: 10.1007/s11065-009-9093-2
9. Rubin LH, Neigh GN, Sundermann EE, Xu Y, Scully EP, Maki PM. Sex differences in neurocognitive function in adults with HIV: patterns, predictors, and mechanisms. Curr Psychiatry Rep. (2019) 21:94. doi: 10.1007/s11920-019-1089-x
10. Barkan SE, Melnick SL, Preston-Martin S, Weber K, Kalish LA, Miotti P, et al. The Women's Interagency HIV Study. WIHS Collaborative Study Group. Epidemiology. (1998) 9:117–25. doi: 10.1097/00001648-199803000-00004
11. Bacon MC, Von Wyl V, Alden C, Sharp G, Robison E, Hessol N, et al. The Women's Interagency HIV Study: an observational cohort brings clinical sciences to the bench. Clin Diagn Lab Immunol. (2005) 12:1013–9. doi: 10.1128/CDLI.12.9.1013-1019.2005
12. Adimora AA, Ramirez C, Benning L, Greenblatt RM, Kempf MC, Tien PC, et al. Cohort profile: the Women's Interagency HIV Study (WIHS). Int J Epidemiol. (2018) 47:393–394i. doi: 10.1093/ije/dyy021
13. Maki PM, Rubin LH, Valcour V, Martin E, Crystal H, Young M, et al. Cognitive function in women with HIV: findings from the Women's Interagency HIV Study. Neurology. (2015) 84:231–40. doi: 10.1212/WNL.0000000000001151
14. Rubin LH, Maki PM, Springer G, Benning L, Anastos K, Gustafson D, et al. Cognitive trajectories over 4 years among HIV-infected women with optimal viral suppression. Neurology. (2017) 89:1594–603. doi: 10.1212/WNL.0000000000004491
15. Radtke KK, Bacchetti P, Anastos K, Merenstein D, Crystal H, Karim R, et al. Use of nonantiretroviral medications that may impact neurocognition: patterns and predictors in a large, long-term HIV cohort study. J Acquir Immune Defic Syndr. (2018) 78:202–8. doi: 10.1097/QAI.0000000000001658
16. Rubin LH, Radtke KK, Eum S, Tamraz B, Kumanan KN, Springer G, et al. Cognitive burden of common non-antiretroviral medications in HIV-infected women. J Acquir Immune Defic Syndr. (2018) 79:83–91. doi: 10.1097/QAI.0000000000001755
17. Rubin LH, Cook JA, Springer G, Weber KM, Cohen MH, Martin EM, et al. Perceived and post-traumatic stress are associated with decreased learning, memory, and fluency in HIV-infected women. AIDS. (2017) 31:2393–1401. doi: 10.1097/QAD.0000000000001625
18. Greendale GA, Wight RG, Huang MH, Avis N, Gold EB, Joffe H, et al. Menopause-associated symptoms and cognitive performance: results from the study of women's health across the nation. Am J Epidemiol. (2010) 171:1214–24. doi: 10.1093/aje/kwq067
19. Rubin LH, Sundermann EE, Cook JA, Martin EM, Golub ET, Weber KM, et al. Investigation of menopausal stage and symptoms on cognition in human immunodeficiency virus-infected women. Menopause. (2014) 21:997–1006. doi: 10.1097/GME.0000000000000203
20. Wehrens R, Buydens LMC. Self- and super-organizing maps in R: the Kohonen package. J. Stat. Softw. (2007) 21:1–19. doi: 10.18637/jss.v021.i05
21. Fraley C, Raftery A, Scrucca L. mclust: Normal Mixture Modeling for Model-Based Clustering, Classification, and Density Estimation (2014).
22. Kuhn M. Building predictive models in R using the caret package. J. Stat. Softw. (2008) 28:1–26. doi: 10.18637/jss.v028.i05
24. Van Buuren S, Groothuis-Oudshoorn K. mice: Multivariate imputation by chained equations in R. J. Stat. Softw. (2011) 45:1–67. doi: 10.18637/jss.v045.i03
25. Horan WP, Braff DL, Nuechterlein KH, Sugar CA, Cadenhead KS, Calkins ME, et al. Verbal working memory impairments in individuals with schizophrenia and their first-degree relatives: findings from the Consortium on the Genetics of Schizophrenia. Schizophr Res. (2008) 103:218–28. doi: 10.1016/j.schres.2008.02.014
26. McDonald CR, Delis DC, Norman MA, Wetter SR, Tecoma ES, Iragui VJ. Response inhibition and set shifting in patients with frontal lobe epilepsy or temporal lobe epilepsy. Epilepsy Behav. (2005) 7:438–46. doi: 10.1016/j.yebeh.2005.05.005
27. Salthouse TA. The processing-speed theory of adult age differences in cognition. Psychol Rev. (1996) 103:403–28. doi: 10.1037/0033-295X.103.3.403
28. Scott JC, Woods SP, Carey CL, Weber E, Bondi MW, Grant I, et al. Neurocognitive consequences of HIV infection in older adults: an evaluation of the “cortical” hypothesis. AIDS Behav. (2011) 15:1187–96. doi: 10.1007/s10461-010-9815-8
29. Milanini B, Allen I, Javandel S, Joanna H, Paul R, Valcour V. Discriminant analysis of neuropsychological testing differentiates HIV-associated neurocogntive disorder from mild cognitive impairment due to Alzheimer's disease. Int. Soc. Neurovirol. (2016) 22:55.
30. Snyder HM, Corriveau RA, Craft S, Faber JE, Greenberg SM, Knopman D, et al. Vascular contributions to cognitive impairment and dementia including Alzheimer's disease. Alzheimers Dement. (2015) 11:710–7. doi: 10.1016/j.jalz.2014.10.008
31. Rubin LH, Sundermann EE, Moore DJ. The current understanding of overlap between characteristics of HIV-associated neurocognitive disorders and Alzheimer's disease. J Neurovirol. (2019) 25:661–72. doi: 10.1007/s13365-018-0702-9
32. Wallin A, Roman GC, Esiri M, Kettunen P, Svensson J, Paraskevas GP, et al. Update on vascular cognitive impairment associated with subcortical small-vessel disease. J Alzheimers Dis. (2018) 62:1417–41. doi: 10.3233/JAD-170803
33. Brands AM, Biessels GJ, De Haan EH, Kappelle LJ, Kessels RP. The effects of type 1 diabetes on cognitive performance: a meta-analysis. Diabetes Care. (2005) 28:726–35. doi: 10.2337/diacare.28.3.726
34. Allen RS, Feola A, Motz CT, Ottensmeyer AL, Chesler KC, Dunn R, et al. Retinal deficits precede cognitive and motor deficits in a rat model of type II diabetes. Invest Ophthalmol Vis Sci. (2019) 60:123–33. doi: 10.1167/iovs.18-25110
35. Magrinelli F, Picelli A, Tocco P, Federico A, Roncari L, Smania N, et al. Pathophysiology of motor dysfunction in Parkinson's disease as the rationale for drug treatment and rehabilitation. Parkinsons Dis. (2016) 2016:9832839. doi: 10.1155/2016/9832839
36. Robertson K, Liner J, Meeker RB. Antiretroviral neurotoxicity. J Neurovirol. (2012) 18:388–99. doi: 10.1007/s13365-012-0120-3
37. Letendre S, Marquie-Beck J, Capparelli E, Best B, Clifford D, Collier AC, et al. Validation of the CNS penetration-effectiveness rank for quantifying antiretroviral penetration into the central nervous system. Arch Neurol. (2008) 65:65–70. doi: 10.1001/archneurol.2007.31
38. Ellis RJ, Badiee J, Vaida F, Letendre S, Heaton RK, Clifford D, et al. CD4 nadir is a predictor of HIV neurocognitive impairment in the era of combination antiretroviral therapy. AIDS. (2011) 25:1747–51. doi: 10.1097/QAD.0b013e32834a40cd
39. Manly JJ, Smith C, Crystal HA, Richardson J, Golub ET, Greenblatt R, et al. Relationship of ethnicity, age, education, and reading level to speed and executive function among HIV+ and HIV– women: the Women's Interagency HIV Study (WIHS) neurocognitive substudy. J Clin Exp Neuropsychol. (2011) 33:853–63. doi: 10.1080/13803395.2010.547662
40. Meyer VJ, Rubin LH, Martin E, Weber KM, Cohen MH, Golub ET, et al. HIV and recent illicit drug use interact to affect verbal memory in women. J Acquir Immune Defic Syndr. (2013) 63:67–76. doi: 10.1097/QAI.0b013e318289565c
41. Meyer VJ, Little DM, Fitzgerald DA, Sundermann EE, Rubin LH, Martin EM, et al. Crack cocaine use impairs anterior cingulate and prefrontal cortex function in women with HIV infection. J Neurovirol. (2014) 20:352–61. doi: 10.1007/s13365-014-0250-x
42. Saloner R, Campbell LM, Serrano V, Montoya JL, Pasipanodya E, Paolillo EW, et al. Neurocognitive superaging in older adults living with HIV: demographic, neuromedical and everyday functioning correlates. J Int Neuropsychol Soc. (2019) 25:507–19. doi: 10.1017/S1355617719000018
43. Gustafson DR, Mielke MM, Tien PC, Valcour V, Cohen M, Anastos K, et al. Anthropometric measures and cognition in middle-aged HIV-infected and uninfected women. The Women's Interagency HIV Study. J Neurovirol. (2013) 19:574–85. doi: 10.1007/s13365-013-0219-1
44. Rubin LH, Gustafson D, Hawkins KL, Zhang L, Jacobson LP, Becker JT, et al. Midlife adiposity predicts cognitive decline in the prospective Multicenter AIDS Cohort Study. Neurology. (2019) 93:e261–71. doi: 10.1212/WNL.0000000000007779
45. Rubin LH, Li Y, Fitzgerald KC, Dastgheyb R, Spence AB, Maki PM, et al. Associations between antiretrovirals and cognitive function in women with HIV. J Neuroimmune Pharmacol. (2020). doi: 10.1007/s11481-020-09910-1. [Epub ahead of print].
46. Stankoff B, Calvez V, Suarez S, Bossi P, Rosenblum O, Conquy L, et al. Plasma and cerebrospinal fluid human immunodeficiency virus type-1 (HIV-1) RNA levels in HIV-related cognitive impairment. Eur J Neurol. (1999) 6:669–75. doi: 10.1046/j.1468-1331.1999.660669.x
47. Valcour V, Yee P, Williams AE, Shiramizu B, Watters M, Selnes O, et al. Lowest ever CD4 lymphocyte count (CD4 nadir) as a predictor of current cognitive and neurological status in human immunodeficiency virus type 1 infection–the Hawaii aging with HIV cohort. J Neurovirol. (2006) 12:387–91. doi: 10.1080/13550280600915339
48. Dawes S, Suarez P, Casey CY, Cherner M, Marcotte TD, Letendre S, et al. Variable patterns of neuropsychological performance in HIV-1 infection. J Clin Exp Neuropsychol. (2008) 30:613–26. doi: 10.1080/13803390701565225
49. Rubin LH, Pyra M, Cook JA, Weber KM, Cohen MH, Martin E, et al. Post-traumatic stress is associated with verbal learning, memory, and psychomotor speed in HIV-infected and HIV-uninfected women. J Neurovirol. (2016) 22:159–69. doi: 10.1007/s13365-015-0380-9
50. Rubin LH, Springer G, Martin EM, Seaberg EC, Sacktor NC, Levine A, et al. Elevated depressive symptoms are a stronger predictor of executive dysfunction in HIV-infected women than men. J Acquir Immune Defic Syndr. (2019) 81:274–83. doi: 10.1097/QAI.0000000000002029
51. Rubin LH, Cook JA, Weber KM, Cohen MH, Martin E, Valcour V, et al. The association of perceived stress and verbal memory is greater in HIV-infected versus HIV-uninfected women. J Neurovirol. (2015) 21:422–32. doi: 10.1007/s13365-015-0331-5
52. Pence BW, O'Donnell JK, Gaynes BN. Falling through the cracks: the gaps between depression prevalence, diagnosis, treatment, and response in HIV care. AIDS. (2012) 26:656–8. doi: 10.1097/QAD.0b013e3283519aae
53. O'Halloran JA, Cooley SA, Strain JF, Boerwinkle A, Paul R, Presti RM, et al. Altered neuropsychological performance and reduced brain volumetrics in people living with HIV on integrase strand transfer inhibitors. AIDS. (2019) 33:1477–83. doi: 10.1097/QAD.0000000000002236
54. O'Halloran JA, Wang K, Williams DW, Dastgheyb R, Fitzgerald K, Kamkwalala A, et al. Integrase inhibitor start or switch impacts learning in women with HIV. In: Conference on Retroviruses and Opportunistic Infections. Boston, MA (2020).
55. Amusan P, Power C, Gill MJ, Gomez D, Johnson E, Rubin LH, et al. Lifetime antiretroviral exposures and neurocognitive impairment in HIV. J Neurovirol. (2020) 26:743–53. doi: 10.1007/s13365-020-00870-z
56. May PE, Heithoff AJ, Wichman CS, Phatak VS, Moore DJ, Heaton RK, et al. Assessing cognitive functioning in people living with HIV (PLWH): factor analytic results from CHARTER and NNTC cohorts. J Acquir Immune Defic Syndr. (2020) 83:251–9. doi: 10.1097/QAI.0000000000002252
Keywords: HIV, cognition, women, heterogeneity, phenotypes, random forest, machine learning
Citation: Dastgheyb RM, Buchholz AS, Fitzgerald KC, Xu Y, Williams DW, Springer G, Anastos K, Gustafson DR, Spence AB, Adimora AA, Waldrop D, Vance DE, Milam J, Bolivar H, Weber KM, Haughey NJ, Maki PM and Rubin LH (2021) Patterns and Predictors of Cognitive Function Among Virally Suppressed Women With HIV. Front. Neurol. 12:604984. doi: 10.3389/fneur.2021.604984
Received: 10 September 2020; Accepted: 18 January 2021;
Published: 11 February 2021.
Edited by:
Pankaj Seth, National Brain Research Centre (NBRC), IndiaReviewed by:
Robert Paul, University of Missouri–St. Louis, United StatesCopyright © 2021 Dastgheyb, Buchholz, Fitzgerald, Xu, Williams, Springer, Anastos, Gustafson, Spence, Adimora, Waldrop, Vance, Milam, Bolivar, Weber, Haughey, Maki and Rubin. This is an open-access article distributed under the terms of the Creative Commons Attribution License (CC BY). The use, distribution or reproduction in other forums is permitted, provided the original author(s) and the copyright owner(s) are credited and that the original publication in this journal is cited, in accordance with accepted academic practice. No use, distribution or reproduction is permitted which does not comply with these terms.
*Correspondence: Leah H. Rubin, bHJ1YmluQGpodS5lZHU=
Disclaimer: All claims expressed in this article are solely those of the authors and do not necessarily represent those of their affiliated organizations, or those of the publisher, the editors and the reviewers. Any product that may be evaluated in this article or claim that may be made by its manufacturer is not guaranteed or endorsed by the publisher.
Research integrity at Frontiers
Learn more about the work of our research integrity team to safeguard the quality of each article we publish.