- 1Allied Health Department, Royal Melbourne Hospital, Parkville, VIC, Australia
- 2Department of Medicine (Royal Melbourne Hospital), University of Melbourne, Parkville, VIC, Australia
Tele-neurorehabilitation has the potential to reduce accessibility barriers and enhance patient outcomes through a more seamless continuum of care. A growing number of studies have found that tele-neurorehabilitation produces equivalent results to usual care for a variety of outcomes including activities of daily living and health related quality of life. Despite the potential of tele-neurorehabilitation, this model of care has failed to achieve mainstream adoption. Little is known about feasibility and acceptability of tele-neurorehabilitation and most published studies do not use a validated model to guide and evaluate implementation. The technology acceptance model (TAM) was developed 20 years ago and is one of the most widely used theoretical frameworks for predicting an individual's likelihood to adopt and use new technology. The TAM3 further built on the original model by incorporating additional elements from human decision making such as computer anxiety. In this perspective, we utilize the TAM3 to systematically map the findings from existing published studies, in order to explore the determinants of adoption of tele-neurorehabilitation by both stroke survivors and prescribing clinicians. We present evidence suggesting that computer self-efficacy and computer anxiety are significant predictors of an individual's likelihood to use tele-neurorehabilitation. Understanding what factors support or hinder uptake of tele-neurorehabilitation can assist in translatability and sustainable adoption of this technology. If we are to shift tele-neurorehabilitation from the research domain to become a mainstream health sector activity, key stakeholders must address the barriers that have consistently hindered adoption.
Stroke
Great advances have been made in acute stroke management, which has led to a marked decrease in mortality rates (1). However, incidence remains high with almost 14 million new strokes occurring annually and more than 80 million prevalent cases globally in 2016. The annual cost to society for first-ever stroke in Australia is AUD $5 billion and in the United States USD $50 billion and includes hospitalization, informal care and loss of productivity (2, 3).
Stroke is the main cause of acquired disability in the adult population with high numbers of survivors experiencing sensorimotor impairment, reduced cognition, and reduced function (4–7). There is strong evidence showing that neurorehabilitation in the acute, subacute, and chronic phases of recovery improves patient outcomes across numerous domains including activities of daily living and health-related quality of life (8–13). Improvement in function and subsequent reduction of disability, by as little as 1-point on the Modified Rankin Scale (mRS), can reduce the costs of care by 85% (2). Despite the evidence of the effectiveness of neurorehabilitation and the potential to reduce burden of care and associated costs, access to rehabilitation is inequitable. A recent audit of acute stroke care in Australia found that only 39% of patients admitted with a primary diagnosis of stroke were assessed for rehabilitation yet 75% were found to have rehabilitation needs (14). This suggests that a large number of Australian stroke survivors may be missing out on the opportunity to maximize their recovery. An Australian study exploring rehabilitation referral patterns for stroke survivors found there were significant variations in selection resulting in inequitable access to rehabilitation (15–17). The reasons for the variation in referrals for neurorehabilitation are multiple and include clinical and non-clinical factors such as reduced workforce capacity and limited access to rehabilitation beds requiring a prioritization approach (17).
Factors that impact on the provision of specialize neurorehabilitation are common across both developing and developed countries (14–18). In Australia, funding models typically emphasize reducing length of stay in an effort to reduce the cost of an episode of rehabilitation. In developing countries, access to organized stroke care, particularly neurorehabilitation, is limited (1, 19, 20). The need for alternative models of neurorehabilitation that are effective and efficient and can overcome current barriers has become an urgent priority, particularly in the more recent context of the COVID-19 pandemic where the demand for remote healthcare has increased rapidly. Telehealth strategies have shown great potential globally as an effective strategy to improve accessibility to healthcare (21, 22). Neurorehabilitation delivered using a telehealth platform, known as tele-neurorehabilitation, may overcome some of the barriers evident in more traditional, center-based models of care. This is particularly true for low and middle-income countries where access to specialized health and rehabilitation services is limited but information and communication technologies (ICT) are readily available and commonly used (23).
Tele-neurorehabilitation
Tele-neurorehabilitation refers to a model of care that uses ICT to deliver clinical rehabilitation and education to patients with a neurological condition at a remote location, such as the patient's home (24, 25). There is a broad range of ICT that may be used in tele-neurorehabilitation from simple devices such as telephones and videoconferencing, up to more complex sensor-based systems with inertial measurement units (25–28).
The number of studies exploring effectiveness of tele-neurorehabilitation has grown over the last 10 years. This increased focus reflects advances in ICT and the growing need to find efficient, effective and economical models of care in the context of fiscally constrained healthcare settings. Laver et al. recently completed a systematic review of tele-neurorehabilitation for stroke services which included evidence from 22 randomized controlled trials with a total of 1,937 participants (29). The studies encompassed a large range of interventions such as mobility retraining, communication therapy and upper limb programs. The technologies used were equally varied and included telephone follow ups, electrical stimulation, IMU sensors and a virtual online library. The authors of the review found there was moderate-quality evidence that tele-neurorehabilitation for stroke survivors achieves results equivalent to usual care for activities of daily living, depressive symptoms, and health related quality of life. However, Laver et al. noted that the studies included in the systematic review did not address feasibility of ICT from the perspective of either the participants or the prescribing clinicians. This raises questions regarding what type of patient is most appropriate for a tele-neurorehabilitation program, how much training is needed for both user and prescriber and what infrastructure is necessary to support sustainable implementation of this model. The large body of research on implementation science suggests that a theoretical model can provide a framework to guide both implementation and evaluation of tele-neurorehabilitation and potentially enhance sustainable adoption of this model (30).
Tele-neurorehabilitation and Technology Acceptance
The technology acceptance model (TAM) is one of the most widely utilized theoretical models explaining an individual's intention to use new technology (31, 32). The first iteration of the TAM was developed in the 1980's and proposed that a person's intention to use and subsequent use of technology can be predicted two beliefs: (1) their perception of how useful the technology is, and (2) their perception as to whether it is easy to use. A large body of research on the TAM found that it consistently predicted 40% of the variance in the intention to use and subsequent use of technology (31). Twenty years later, the TAM was extended to become the TAM2 and included output quality, results demonstrability, job relevance, subjective norm and perceived ease of use as determinants of perceived usefulness. Another model, the determinants of perceived ease of use, was developed at the same time and included factors that anchor beliefs about technology, including computer self-efficacy, computer anxiety, computer playfulness and perceptions of external control (31). Furthermore, experience and voluntary use of the technology were considered to be factors that moderated perceived usefulness.
Most recently, the TAM2 and the determinants of perceived ease of use have been combined to become the TAM3. This integrated model proposes that perceived usefulness is influenced by a number of factors including the quality of output from the technology and how relevant it is to the needs of the user. Perceived ease of use is determined by the person's beliefs about their own skills and includes computer self-efficacy and anxiety. Importantly, both perceived usefulness and perceived ease of use can be mediated through external factors such as increased practice / experience using the technology and adequate resources to support the person's use of technology (31).
There is a significant body of research on application of all iterations of the TAM in health settings, particularly in relation to the adoption of electronic health records and telehealth (33, 34). A recent study used the TAM to predict if a group (n = 325) of Canadians would use electronic medical health records to manage health information such as making future appointments (35). The authors found that perceived ease of use was the strongest predictor of perceived usefulness. The users' prior experiences with technology, needs and values all correlated with intention to use the electronic medical health record. Another study exploring patient uptake of electronic health records found that difficulties logging in and a complex user interface impacted on adoption (36). Despite the growing evidence-base using the TAM to predict user adoption of technology, none of the published studies focus on tele-neurorehabilitation. The aim of this perspective is to explore if published studies on tele-neurorehabilitation can be mapped onto the variables in the TAM3.
Methods
A systematic mapping review approach was selected as the intention was to describe and categorize the body of tele-neurorehabilitation evidence using the TAM3 framework. A traditional systematic review aims to identify and assess the quality of published literature in order to answer a very specific question. By contrast, a systematic mapping review characterizes the literature and catalogs it according to a criteria or framework or model. In this study, the published literature on tele-neurorehabilitation will be described and categorized using the TAM3 framework. A systematic mapping review process is particularly useful when the topic area is broad and the quality and range of studies is diverse (37–39). This approach can provide information about knowledge gaps and therefore direct future research, including systematic reviews.
The methods applied to a systematic mapping review process are as follows: (1) literature search, (2) literature selection, and (3) literature mapping to the TAM3.
Literature Search
Databases relevant to the health sciences were searched including CINAHL (EBSCO), PsycINFO (EBSCO), PubMed (National Center for Biotechnology Information), and SCOPUS. Search terms were telerehabilitation or tele-rehabilitation or telehealth or remote rehabilitation AND stroke or cerebrovascular accident or CVA AND home or remote. Limitations included English-language, adult population and peer-reviewed papers. The search date was for studies published from 2000 to July 2020.
Literature Selection
The aim of this study was to determine if the existing literature on tele-neurorehabilitation could be mapped using the TAM3, with a specific focus on the user experience. Therefore, studies which included any information on patient or therapist experience of tele-neurorehabilitation, were a particular target. Following removal of protocols, center-based interventions and systematic reviews, a total of 22 studies were identified. Table 1 presents data extracted from the studies including aims, population and outcomes. The studies included pre/post-studies, evaluation of devices, and qualitative exploration of user experience with tele-neurorehabilitation. Participants were stroke survivors in acute, sub-acute or chronic phases of recovery and varied in their impairments. Consequently, the tele-neurorehabilitation interventions included sit-to-stand practice, communication therapies, psychosocial interventions and activities of daily living practice. The type of ICT used also varied widely from telephones to apps with associated sensor data.
Literature Mapping to TAM3
All 22 studies were readily able to be mapped on to the TAM3 and revealed patterns in relation to the barriers and facilitators for tele-neurorehabilitation. Figure 1 displays the findings using the TAM3 model which is expanded on in the following sections.
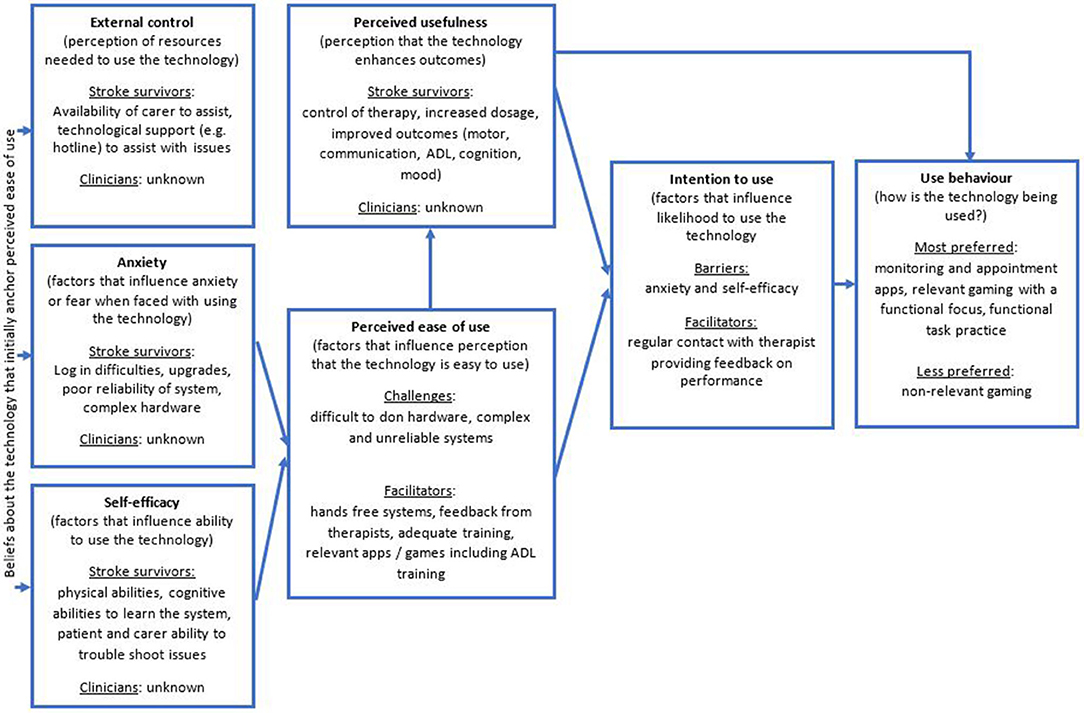
Figure 1. Result of mapping the 22 studies to the variables of the TAM3 (31).
Results
Tele-Neurorehabilitation and Perceived Usefulness
Do Stroke Survivors and Clinicians Perceive That Tele-Neurorehabilitation Will Be Beneficial?
The majority of studies found that tele-neurorehabilitation interventions were not inferior to conventional center-based models of care (41, 42, 46, 47, 50, 54–56, 60). Patients and carers/family reported subjective improvements in communication, gait, activities of daily living, and motivation. Only one study found that patients preferred a conventional home exercise program over tele-neurorehabilitation due to the perception that the tele-neurorehabilitation was too complex (59). None of the studies explored therapist perceptions of the usefulness of tele-neurorehabilitation, particularly in comparison to conventional models of center-based neurorehabilitation.
Tele-Neurorehabilitation and Perceived Ease of Use
Do Stroke Survivors and Clinicians Perceive That Tele-Neurorehabilitation Is Easy to Use?
A number of studies found that participants enjoyed gaming technology associated with some of the tele-neurorehabilitation interventions and found them easy to use, engaging and motivating (41, 43, 47, 48, 52, 54). Experiences with both hardware and software had a marked effect on the user perception of tele-neurorehabilitation. For example, hands-free systems were perceived as easier to use (60) than those that required the participant to don/doff splints, sensors, and other similar hardware (40–42, 45, 56, 57).
Low self-efficacy related to the tele-neurorehabilitation system used was a frequently reported problem that affected perception of ease of use, compliance, and subsequent intention to continue using the system in the future (57, 60). Conversely, prior experience with relevant technology, such as computers, correlated to improved compliance and perceptions of ease of use (46, 53).
Anxiety and frustration with tele-neurorehabilitation was apparent when more complex ICT and hardware was used (42, 44, 46). Unreliable internet bandwidth, and technical issues which were not easily resolved further contributed to anxiety and perceptions of ease of use (46). Studies where the ICT was familiar (e.g., telephones) and consisted of easy to understand tasks (e.g., sit-to-stand practice) appeared to reduce anxiety secondary to the perception that they were easier to use (47, 56).
Increased exposure and practice with tele-neurorehabilitation systems improved compliance and reduced anxiety related to low confidence and proficiency with technology (46, 48, 49, 59). However, the amount of experience necessary to reduce technology-related anxiety remains unclear with studies reporting variable amounts of time spent on training participants and therapists. Conversely, one study found there was a correlation between number of training sessions to achieve proficiency in using the technology and poorer outcomes, indicating that the technology may not be suitable for all disorders or patients (49).
The most commonly reported external variable necessary to support engagement in tele-neurorehabilitation was the presence of a carer/family member (44, 46, 61, 62). This was the case irrespective of the nature of intervention being delivered or the type of ICT being used.
Tele-Neurorehabilitation and Behavioral Intentions
Are Stroke Survivors and Clinicians Motivated or Willing to Exert the Effort to Engage in Tele-Neurorehabilitation?
A number of studies found that participants reported an intention to continuing engaging in tele-neurorehabilitation, with some provisos, including a request for easier and more reliable technology and access to their performance results (40, 41, 47, 52, 53).
Tele-Neurorehabilitation and Use Behavior
How Are Stroke Survivors and Clinicians Using Tele-Neurorehabilitation?
Tele-neurorehabilitation with a focus on relevant, easy to use components was rated more highly by participants than complex systems with multiple componentry (46, 53). For example, appointment reminder systems, monitoring apps and ADL focused gaming was selected more often than motor-based gaming. Participants also preferred tele-neurorehabilitation systems where the therapist could observe and provide feedback and encouragement via a videoconferencing or other interactive system (41, 45, 54, 56).
Discussion
It is anticipated that the demand for neurorehabilitation will continue to grow due to an aging population and high incidence rates of diseases such as stroke. Rehabilitation resources in both developing and developed countries are limited and the need to find alternative yet effective and efficient models is imperative. Tele-neurorehabilitation has great potential to increase accessibility to rehabilitation for individuals with neurological impairments. However, consideration must be given for both human and ICT factors that can hinder or facilitate adoption of tele-neurorehabilitation.
A review of the published evidence on tele-neurorehabilitation through the lens of TAM3 reveals that perception of ease of use is influenced by the user's belief that they have the requisite skills and ability to use the technology (computer self-efficacy) and the degree of apprehension or fear they experience when faced with learning to use the technology (computer anxiety). Easy to use ICT and adequate experience using the technology assists the user to adjust their beliefs about computer self-efficacy and reduces computer anxiety (46, 63). Some studies found that patients were open to and excited about tele-neurorehabilitation but experienced numerous technological malfunctions which increased computer anxiety and reduced perceived enjoyment (64). Nearly all studies found that carers were critical to ensure that patients were able to overcome barriers related to system set-up, thus reducing computer anxiety (63).
There is little to no evidence on the feasibility of tele-neurorehabilitation for the “typical” stroke survivor who is likely to have cognitive impairment. Published studies have found that patients with cognitive impairment can benefit from computer training programs, suggesting that at least some of these patients may have computer self-efficacy and be appropriate for a tele-neurorehabilitation intervention. There is little to no evidence on how much experience or practice and training with a system is needed for the stroke survivor to become a confident user. Understanding the type and frequency of training necessary to establish and maintain computer self-efficacy would contribute to a more informed implementation and sustainable adoption of tele-neurorehabilitation.
Although gains have been made in design of ICT to potentially enhance tele-neurorehabilitation, barriers to adoption that were identified more than 20 years ago remain apparent today (65). These barriers are relevant for both patients and prescribing clinicians and include poor computer self-efficacy, high computer anxiety, low perception of usefulness and a belief that the technology is not user-friendly (65, 66). If we are to realize the full potential of tele-neurorehabilitation, it is of critical importance that we approach the topic using a validated and well-tested theoretical framework to guide and evaluate implementation. This would make a significant contribution to the evidence-base on tele-neurorehabilitation.
Data Availability Statement
The original contributions presented in the study are included in the article/supplementary material, further inquiries can be directed to the corresponding author/s.
Author Contributions
MK and MG conceived the ideas presented. MK completed the initial draft of the manuscript which was reviewed and edited by MG resulting in the final manuscript. All authors contributed to the article and approved the submitted version.
Conflict of Interest
The authors declare that the research was conducted in the absence of any commercial or financial relationships that could be construed as a potential conflict of interest.
References
1. Johnson CO, Nguyen M, Roth GA, Nichols E, Alam T, Abate D, et al. Global, regional, and national burden of stroke, 1990–2016: a systematic analysis for the global burden of disease study 2016. Lancet Neurol. (2019) 18:439–58. doi: 10.1016/S1474-4422(19)30034-1
2. Majersik JJ, Woo D. The enormous financial impact of stroke disability. Neurology. (2020) 94:377–8. doi: 10.1212/WNL.0000000000009030
3. Cadilhac DA, Carter R, Thrift AG, Dewey HM. Estimating the long-term costs of ischemic and hemorrhagic stroke for Australia: new evidence derived from the North East Melbourne stroke incidence study (NEMESIS). Stroke. (2009) 40:915–21. doi: 10.1161/STROKEAHA.108.526905
4. Sun JH, Tan L, Yu JT. Post-stroke cognitive impairment: epidemiology, mechanisms and management. Ann Transl Med. (2014) 2:80. doi: 10.3978/j.issn.2305-5839.2014.08.05
5. Douiri A, Rudd AG, Wolfe CD. Prevalence of poststroke cognitive impairment: South London stroke register 1995–2010. Stroke. (2013) 44:138–45. doi: 10.1161/STROKEAHA.112.670844
6. Langhorne P, Coupar F, Pollock A. Motor recovery after stroke: a systematic review. Lancet Neurol. (2009) 8:741–54. doi: 10.1016/S1474-4422(09)70150-4
7. Crichton SL, Bray BD, McKevitt C, Rudd AG, Wolfe CD. Patient outcomes up to 15 years after stroke: survival, disability, quality of life, cognition and mental health. J Neurol Neurosurg Psychiatry. (2016) 87:1091–8. doi: 10.1136/jnnp-2016-313361
8. Hubbard IJ, Harris D, Kilkenny MF, Faux SG, Pollack MR, Cadilhac DA. Adherence to clinical guidelines improves patient outcomes in Australian audit of stroke rehabilitation practice. Arch Phys Med Rehabil. (2012) 93:965–71. doi: 10.1016/j.apmr.2012.01.011
9. Hubbard IJ, Parsons MW, Neilson C, Carey LM. Task-specific training: evidence for and translation to clinical practice. Occup Ther Int. (2009) 16:175–89. doi: 10.1002/oti.275
10. Wright L, Hill KM, Bernhardt J, Lindley R, Ada L, Bajorek BV, et al. Stroke management: updated recommendations for treatment along the care continuum. Intern Med J. (2012) 42:562–9. doi: 10.1111/j.1445-5994.2012.02774.x
11. Korner-Bitensky N. When does stroke rehabilitation end? Int J Stroke. (2013) 8:8–10. doi: 10.1111/j.1747-4949.2012.00963.x
12. Granger CV, Markello SJ, Graham JE, Deutsch A, Ottenbacher KJ. The uniform data system for medical rehabilitation: report of patients with stroke discharged from comprehensive medical programs in 2000-2007. Am J Phys Med Rehabil. (2009) 88:961–72. doi: 10.1097/PHM.0b013e3181c1ec38
13. Andrusin J, Breen JC, Keenan J, Monico S, Garth H. Abstract TP181: community based outpatient stroke rehabilitation program achieve excellent outcomes including return to work, driving, stroke education knowledge, and other rehabilitation outcomes. Stroke. (2019) 50(Suppl. 1):ATP181. doi: 10.1161/str.50.suppl_1.TP181
15. Hakkennes SJ, Brock K, Hill KD. Selection for inpatient rehabilitation after acute stroke: a systematic review of the literature. Arch Phys Med Rehabil. (2011) 92:2057–70. doi: 10.1016/j.apmr.2011.07.189
16. Ilett PA, Brock KA, Graven CJ, Cotton SM. Selecting patients for rehabilitation after acute stroke: are there variations in practice? Arch Phys Med Rehabil. (2010) 91:788–93. doi: 10.1016/j.apmr.2009.11.028
17. Kennedy GM, Brock KA, Lunt AW, Black SF. Factors influencing selection for rehabilitation after stroke: a questionnaire using case scenarios to investigate physician perspectives and level of agreement. Arch Phys Med Rehabil. (2012) 93:1457–9. doi: 10.1016/j.apmr.2011.11.036
18. Jørgensen HS, Kammersgaard LP, Houth J, Nakayama H, Raaschou HO, Larsen K, et al. Who benefits from treatment and rehabilitation in a stroke unit? A community-based study. Stroke. (2000) 31:434–9. doi: 10.1161/01.STR.31.2.434
19. Pandian JD, Sudhan P. Stroke epidemiology and stroke care services in India. J Stroke. (2013) 15:128–34. doi: 10.5853/jos.2013.15.3.128
20. Wissel J, Olver J, Sunnerhagen KS. Navigating the poststroke continuum of care. J Stroke Cerebrovasc Dis. (2013) 22:1–8. doi: 10.1016/j.jstrokecerebrovasdis.2011.05.021
21. Moffatt JJ, Eley DS. The reported benefits of telehealth for rural Australians. Aust Health Rev. (2010) 34:276–81. doi: 10.1071/AH09794
22. Bagayoko CO, Traore D, Thevoz L, Diabate S, Pecoul D, Niang M, et al. Medical and economic benefits of telehealth in low- and middle-income countries: results of a study in four district hospitals in Mali. BMC Health Serv Res. (2014) 14(Suppl. 1):S9. doi: 10.1186/1472-6963-14-S1-S9
23. Combi C, Pozzani G, Pozzi G. Telemedicine for developing countries. A survey and some design issues. Appl Clin Inform. (2016) 7:1025–50. doi: 10.4338/ACI-2016-06-R-0089
24. Brennan DM, Barker LM. Human factors in the development and implementation of telerehabilitation systems. J Telemed Telecare. (2008) 14:55–8. doi: 10.1258/jtt.2007.007040
25. Russell TG. Physical rehabilitation using telemedicine. J Telemed Telecare. (2007) 13:217–20. doi: 10.1258/135763307781458886
26. Hoffmann T, Russell T, Cooke H. Remote measurement via the internet of upper limb range of motion in people who have had a stroke. J Telemed Telecare. (2007) 13:401–5. doi: 10.1258/135763307783064377
27. Crocher V, Fong J, Klaic M, Oetomo D, Tan Y. A tool to address movement quality outcomes of post-stroke patients. Replace, Repair, Restore, Relieve – Bridging Clinical and Engineering Solutions in Neurorehabilitation 2014. Cham: Springer International Publishing (2014). doi: 10.1007/978-3-319-08072-7_53
28. Holden M, Dyar T, Dayan-Cimadoro L, Schwamm L, Bizzi E. Virtual environment training in the home via telerehabilitation. Arch Phys Med Rehabil. (2004) 85:E12. doi: 10.1016/j.apmr.2004.06.046
29. Laver KE, Adey-Wakeling Z, Crotty M, Lannin NA, George S, Sherrington C. Telerehabilitation services for stroke. Cochrane Database Syst Rev. (2020) 1:CD010255. doi: 10.1002/14651858.CD010255.pub3
30. Grimshaw JM, Eccles MP, Lavis JN, Hill SJ, Squires JE. Knowledge translation of research findings. Implement Sci. (2012) 7:50. doi: 10.1186/1748-5908-7-50
31. Venkatesh V, Bala H. Technology acceptance model 3 and a research agenda on interventions. J Decis Sci Inst. (2008) 39:273–315. doi: 10.1111/j.1540-5915.2008.00192.x
32. Davis FD, Bagozzi RP, Warshaw PR. User acceptance of computer technology: a comparison of two theoretical models. Manag Sci. (1989) 35:982–1003. doi: 10.1287/mnsc.35.8.982
33. Rahimi B, Nadri H, Lotfnezhad Afshar H, Timpka T. A systematic review of the technology acceptance model in health informatics. Appl Clin Inform. (2018) 9:604–34. doi: 10.1055/s-0038-1668091
34. Holden RJ, Karsh BT. The technology acceptance model: its past and its future in health care. J Biomed Inform. (2010) 43:159–72. doi: 10.1016/j.jbi.2009.07.002
35. Razmak J, Bélanger C. Using the technology acceptance model to predict patient attitude toward personal health records in regional communities. Inform Technol People. (2018) 31:306–326. doi: 10.1108/ITP-07-2016-0160
36. Portz JD, Bayliss EA, Bull S, Boxer RS, Bekelman DB, Gleason K, et al. Using the technology acceptance model to explore user experience, intent to use, and use behavior of a patient portal among older adults with multiple chronic conditions: descriptive qualitative study. J Med Internet Res. (2019) 21:e11604. doi: 10.2196/11604
37. Grant MJ, Booth A. A typology of reviews: an analysis of 14 review types and associated methodologies. Health Inform Librar J. (2009) 26:91–108. doi: 10.1111/j.1471-1842.2009.00848.x
38. Hammick M. A BEME review: a little illumination. Med Teach. (2005) 27:1–3. doi: 10.1080/01421590500046858
40. Burdea GC, Grampurohit N, Kim N, Polistico K, Kadaru A, Pollack S, et al. Feasibility of integrative games and novel therapeutic game controller for telerehabilitation of individuals chronic post-stroke living in the community. Top Stroke Rehabil. (2020) 27:321–36. doi: 10.1080/10749357.2019.1701178
41. Chen Y, Chen Y, Zheng K, Dodakian L, See J, Zhou R, et al. A qualitative study on user acceptance of a home-based stroke telerehabilitation system. Top Stroke Rehabil. (2020) 27:81–92. doi: 10.1080/10749357.2019.1683792
42. Cherry CO, Chumbler NR, Richards K, Huff A, Wu D, Tilghman LM, et al. Expanding stroke telerehabilitation services to rural veterans: a qualitative study on patient experiences using the robotic stroke therapy delivery and monitoring system program. Disabil Rehabil Assist Technol. (2017) 12:21–7. doi: 10.3109/17483107.2015.1061613
43. Cronce A, Gerald Fluet P, Patel J, Alma Merians PJJOAMR. Home-based virtual rehabilitation for upper extremity functional recovery post-stroke. J Alt Med Res. (2018) 10:27–35.
44. Deng H, Durfee WK, Nuckley DJ, Rheude BS, Severson AE, Skluzacek KM, et al. Complex versus simple ankle movement training in stroke using telerehabilitation: a randomized controlled trial. Physical Ther. (2012) 92:197–209. doi: 10.2522/ptj.20110018
45. Deutsch JE, Maidan I, Dickstein R. Patient-centered integrated motor imagery delivered in the home with telerehabilitation to improve walking after stroke. Physical Ther. (2012) 92:1065–77. doi: 10.2522/ptj.20110277
46. Dodakian L, McKenzie AL, Le V, See J, Pearson-Fuhrhop K, Burke Quinlan E, et al. A home-based telerehabilitation program for patients with stroke. Neurorehabil Neural Repair. (2017) 31:923–33. doi: 10.1177/1545968317733818
47. Ellington A, Adams R, White M, Diamond P. Behavioral intention to use a virtual instrumental activities of daily living system among people with stroke. Am J Occupat Ther. (2015) 69:6903290030p1–8. doi: 10.5014/ajot.2015.014373
48. Flynn S, Palma P, Bender A. Feasibility of using the sony playstation 2 gaming platform for an individual poststroke: a case report. J Neurol Phys Ther. (2007) 31:180–9. doi: 10.1097/NPT.0b013e31815d00d5
49. Kurland J, Liu A, Stokes P. Effects of a tablet-based home practice program with telepractice on treatment outcomes in chronic aphasia. J Speech Lang Hear Res. (2018) 61:1140–56. doi: 10.1044/2018_JSLHR-L-17-0277
50. Lai JC, Woo J, Hui E, Chan W. Telerehabilitation—a new model for community-based stroke rehabilitation. J Telemed Telecare. (2004) 10:199–205. doi: 10.1258/1357633041424340
51. Langan J, Subryan H, Nwogu I, Cavuoto L. Reported use of technology in stroke rehabilitation by physical and occupational therapists. Disabil Rehabil Assist Technol. (2018) 13:641–7. doi: 10.1080/17483107.2017.1362043
52. Piron L, Turolla A, Tonin P, Piccione F, Lain L, Dam M. Satisfaction with care in post-stroke patients undergoing a telerehabilitation programme at home. J Telemed Telecare. (2008) 14:257–60. doi: 10.1258/jtt.2008.080304
53. Rogerson L, Burr J, Tyson S. The feasibility and acceptability of smart home technology using the Howz system for people with stroke. Disabil Rehabil Assist Technol. (2020) 15:148–52. doi: 10.1080/17483107.2018.1541103
54. Seo NJ, Arun Kumar J, Hur P, Crocher V, Motawar B, Lakshminarayanan K. Usability evaluation of low-cost virtual reality hand and arm rehabilitation games. J Rehabil Res Dev. (2016) 53:321–34. doi: 10.1682/JRRD.2015.03.0045
55. Simpson LA, Eng JJ, Chan M. H-GRASP: the feasibility of an upper limb home exercise program monitored by phone for individuals post stroke. Disabil Rehabil. (2017) 39:874–82. doi: 10.3109/09638288.2016.1162853
56. Simpson DB, Bird ML, English C, Gall SL, Breslin M, Smith S, et al. Connecting patients and therapists remotely using technology is feasible and facilitates exercise adherence after stroke. Top Stroke Rehabil. (2020) 27:93–102. doi: 10.1080/10749357.2019.1690779
57. Standen PJ, Threapleton K, Connell L, Richardson A, Brown DJ, Battersby S, et al. Patients' use of a home-based virtual reality system to provide rehabilitation of the upper limb following stroke. Physical Ther. (2015) 95:350–9. doi: 10.2522/ptj.20130564
58. Threapleton K, Newberry K, Sutton G, Worthington E, Drummond A. Virtually home: Exploring the potential of virtual reality to support patient discharge after stroke. Br. J. Occup. Ther. (2017) 80:99–107.
59. Triandafilou KM, Tsoupikova D, Barry AJ, Thielbar KN, Stoykov N, Kamper DG. Development of a 3D, networked multi-user virtual reality environment for home therapy after stroke. J Neuroeng Rehabil. (2018) 15:88. doi: 10.1186/s12984-018-0429-0
60. Warland A, Paraskevopoulos I, Tsekleves E, Ryan J, Nowicky A, Griscti J, et al. The feasibility, acceptability and preliminary efficacy of a low-cost, virtual-reality based, upper-limb stroke rehabilitation device: a mixed methods study. Disabil Rehabil. (2019) 41:2119–34. doi: 10.1080/09638288.2018.1459881
61. Woolf C, Caute A, Haigh Z, Galliers J, Wilson S, Kessie A, et al. A comparison of remote therapy, face to face therapy and an attention control intervention for people with aphasia: a quasi-randomised controlled feasibility study. Clin Rehabil. (2016) 30:359–73. doi: 10.1177/0269215515582074
62. Standen PJ, Threapleton K, Richardson A, Connell L, Brown DJ, Battersby S, et al. A low cost virtual reality system for home based rehabilitation of the arm following stroke: a randomised controlled feasibility trial. Clin Rehabil. (2017) 31:340–50. doi: 10.1177/0269215516640320
63. Cikajlo I, Hukić A, Dolinšek I, Zajc D, Vesel M, Krizmanič T, et al. Can telerehabilitation games lead to functional improvement of upper extremities in individuals with Parkinson's disease? Int J Rehabil Res. (2018) 41:230–8. doi: 10.1097/MRR.0000000000000291
64. Brown EVD, Dudgeon BJ, Gutman K, Moritz CT, McCoy SW. Understanding upper extremity home programs and the use of gaming technology for persons after stroke. Disabil Health J. (2015) 8:507–13. doi: 10.1016/j.dhjo.2015.03.007
65. Standing C, Standing S, McDermott ML, Gururajan R, Kiani Mavi R. The paradoxes of telehealth: a review of the literature 2000–2015. Syst Res Behav Sci. (2018) 35:90–101. doi: 10.1002/sres.2442
Keywords: stroke, neurorehabilitation after stroke, tele-neurorehabilitation, technology—ICT, telehealth acceptance
Citation: Klaic M and Galea MP (2020) Using the Technology Acceptance Model to Identify Factors That Predict Likelihood to Adopt Tele-Neurorehabilitation. Front. Neurol. 11:580832. doi: 10.3389/fneur.2020.580832
Received: 07 July 2020; Accepted: 11 November 2020;
Published: 02 December 2020.
Edited by:
Paolo Tonin, Sant'Anna Institute, ItalyReviewed by:
Robin E. A. Green, University Health Network, CanadaToshiyuki Fujiwara, Juntendo University, Japan
Copyright © 2020 Klaic and Galea. This is an open-access article distributed under the terms of the Creative Commons Attribution License (CC BY). The use, distribution or reproduction in other forums is permitted, provided the original author(s) and the copyright owner(s) are credited and that the original publication in this journal is cited, in accordance with accepted academic practice. No use, distribution or reproduction is permitted which does not comply with these terms.
*Correspondence: Marlena Klaic, bWFybGVuYS5rbGFpY0BtaC5vcmcuYXU=