- 1Department of Physical Medicine and Rehabilitation, University of Pittsburgh School of Medicine, Pittsburgh, PA, United States
- 2Department of Population Health Sciences, Duke University School of Medicine, Durham, NC, United States
- 3Safar Center for Resuscitation Research, Division of Pediatric Critical Care Medicine, UPMC Children's Hospital of Pittsburgh, Department of Critical Care and Pediatrics, University of Pittsburgh School of Medicine, Pittsburgh, PA, United States
- 4Department of Health Promotion and Development, School of Nursing, University of Pittsburgh, Pittsburgh, PA, United States
- 5Department of Psychology, Alberta Children's Hospital Research Institute, Hotchkiss Brain Institute, University of Calgary, Calgary, AB, Canada
Introduction: Unexplained heterogeneity in outcomes following pediatric traumatic brain injury (TBI) is one of the most critical barriers to the development of effective prognostic tools and therapeutics. The addition of personal biological factors to our prediction models may account for a significant portion of unexplained variance and advance the field toward precision rehabilitation medicine. The overarching goal of the Epigenetic Effects on Pediatric Traumatic Brain Injury Recovery (EETR) study is to investigate an epigenetic biomarker involved in both childhood adversity and postinjury neuroplasticity to better understand heterogeneity in neurobehavioral outcomes following pediatric TBI. Our primary hypothesis is that childhood adversity will be associated with worse neurobehavioral recovery in part through an epigenetically mediated reduction in brain-derived neurotrophic factor (BDNF) expression in response to TBI.
Methods and analysis: EETR is an observational, prospective, longitudinal concurrent cohort study of children aged 3–18 years with either TBI (n = 200) or orthopedic injury (n = 100), recruited from the UPMC Children's Hospital of Pittsburgh. Participants complete study visits acutely and at 6 and 12 months postinjury. Blood and saliva biosamples are collected at all time points—and cerebrospinal fluid (CSF) when available acutely—for epigenetic and proteomic analysis of BDNF. Additional measures assess injury characteristics, pre- and postinjury child neurobehavioral functioning, childhood adversity, and potential covariates/confounders. Recruitment began in July 2017 and will occur for ~6 years, with data collection complete by mid-2023. Analyses will characterize BDNF DNA methylation and protein levels over the recovery period and investigate this novel biomarker as a potential biological mechanism underlying the known association between childhood adversity and worse neurobehavioral outcomes following pediatric TBI.
Ethics and dissemination: The study received ethics approval from the University of Pittsburgh Institutional Review Board. Participants and their parents provide informed consent/assent. Research findings will be disseminated via local and international conference presentations and manuscripts submitted to peer-reviewed journals.
Trial Registration: The study is registered with clinicaltrials.org (ClinicalTrials.gov Identifier: NCT04186429).
Introduction
Traumatic brain injury (TBI) is a leading cause of morbidity and mortality in childhood (1). The scientific evidence available to guide prognosis, management, and treatment is disproportionately low relative to TBI's medical and societal burden. Children sustaining moderate to severe TBI often demonstrate neurobehavioral impairments that hamper their long-term functioning (2–4). Previous large-scale cohort studies in pediatric TBI have identified injury (e.g., severity, neuropathological, accidental vs. nonaccidental), developmental (e.g., age at injury, time since injury), child (e.g., premorbid functioning), and psychosocial (e.g., socioeconomic status, family functioning) factors as significant determinants of outcomes (5–12). Current prediction models including these factors, however, explain only ~35% of variance in outcomes (13). Without the identification of additional factors influencing recovery, this unexplained heterogeneity will remain one of the most critical barriers to accurate prognostication and to the development of evidence-based treatments for the neurobehavioral consequences of pediatric TBI (14, 15).
The addition of personal biological factors or “biomarkers” in our prediction models may account for a significant portion of unexplained variance. This would advance the field of pediatric TBI toward precision rehabilitation medicine, to thereby improve individual prognostication, predict response to rehabilitation, and identify novel targets for therapy development. A biomarker gaining traction in TBI research is genetic variation, with the hypothesis being that certain individuals may be genetically predisposed to worse recovery and outcomes (16–18). The investigation of genetics without consideration of factors that modulate gene expression, however, precludes a more integrative and comprehensive understanding of the biological and environmental mechanisms affecting recovery.
Over the last decade, the field of epigenetics has become central to studying the modulation of genetic phenotypes by environmental factors. Broadly defined, epigenetics involves biochemical processes that regulate gene expression without altering the corresponding primary DNA sequence (19). Through epigenetic processes, the social and biological environment of an individual impacts when and to what extent genes are expressed within each cell type. The best-characterized epigenetic mechanism is DNA methylation, with higher levels rendering the gene less transcriptionally active (20).
Among the strongest epigenetic influences on child development is the family environment, especially when it involves exposure to childhood adversity (21, 22). Children who experience greater adversity, such as poverty, separation from a parent, and maltreatment, show altered methylation profiles in genes involved in the function of the hypothalamic–pituitary–adrenal axis, immune response, and neuronal development and neuroplasticity (21, 23, 24). In turn, these altered methylation profiles are associated with higher prevalence of chronic health conditions (25), as well as a multitude of neurobehavioral phenotypes, including depression and anxiety, borderline personality disorder, attention problems, and poor impulse control (23, 24, 26–28).
In pediatric TBI, decades of research demonstrate worse outcomes in children exposed to greater adversity, including lower socioeconomic status, greater family dysfunction, and abusive head trauma as the injury mechanism (4, 5, 10, 29, 30). Despite these established findings, the biological mechanisms underlying these effects are unknown. Given the known influence of childhood adversity on both children's epigenetic profiles and recovery from pediatric TBI, the effect of childhood adversity on recovery may be mediated by epigenetic mechanisms.
The brain-derived neurotrophic factor (BDNF) gene is an ideal candidate for the first exploratory examination of epigenetic influences on neurobehavioral recovery following pediatric TBI because it is involved in both: (1) the biological response to childhood adversity and (2) neuroplasticity following TBI. BDNF is a well-studied member of the neurotrophin family of growth factors. It is released from neurons both pre- and postsynaptically and mediates apoptosis, neuronal differentiation, outgrowth of neurites, cell survival, and synaptic strengthening (31, 32). Thus, BDNF is intimately involved in brain development, neuroplasticity, and neuronal survival (33, 34), as well as complex cognitive processes, including learning/memory (35, 36) and executive functioning (37–40). A single-nucleotide polymorphism producing a valine-to-methionine substitution at codon 66 (val66met) in the BDNF gene is associated with reduced activity-dependent secretion of BDNF (41) and may modify methylation level at this position on the gene (42–44). In addition to other transcriptional and translational regulators (45, 46), BDNF gene expression, as is the case with many genes, is regulated in part by DNA methylation, such that higher methylation is associated with lower neuronal synthesis of BDNF (47).
BDNF DNA methylation epigenetically encodes the effects of childhood adversity on neurodevelopment. Multiple recent studies show that early adversity produces changes in BDNF methylation detectable in the brain and peripheral biosamples in both animal models (48–50) and humans (23, 51–53). Both higher BDNF methylation (23, 54) and lower BDNF protein levels (55–60) are associated with worse neurobehavioral outcomes in children with preterm birth, attention-deficit/hyperactivity disorder (ADHD), bipolar disorder, anorexia, and autism spectrum disorder.
The BDNF pathway is also involved in neural recovery from TBI. BDNF is upregulated in the frontal cortex and the hippocampus after experimental TBI and may mediate recovery processes after brain injury (61–63). In adult clinical studies, BDNF levels in cerebrospinal fluid (CSF) show a sharp spike within 24 h postinjury and steadily decline over 1 week (64, 65), providing evidence for BDNF upregulation and involvement in recovery post-TBI consistent with animal models. BDNF levels during the first week postinjury tend to be comparable in CSF (65) but lower in serum in adults with TBI relative to nonbrain-injured controls (65, 66). Furthermore, acute and chronic BDNF levels in CSF and serum/plasma after TBI in adults predict outcomes, including mortality (65), global outcomes (66, 67), and long-term functional cognition and depression (68, 69). Far fewer studies have examined BDNF levels after pediatric TBI. Initial evidence shows a sharp peak in BDNF levels immediately following injury, followed by subsequent declines (70, 71), similar to that shown in adults. Higher CSF and plasma/serum BDNF levels predicted better global recovery in pediatric TBI studies (71, 72), but to our knowledge, no known studies have examined BDNF genetics or epigenetics in association with TBI outcomes in children.
Thus, the overarching goal of our study is to investigate an epigenetic biomarker involved in both childhood adversity and postinjury neuroplasticity to better understand heterogeneity in neurobehavioral outcomes following TBI. Our primary hypothesis is that childhood adversity will be associated with worse neurobehavioral recovery in part through an epigenetically mediated reduction in BDNF expression in response to TBI (see Figure 1).
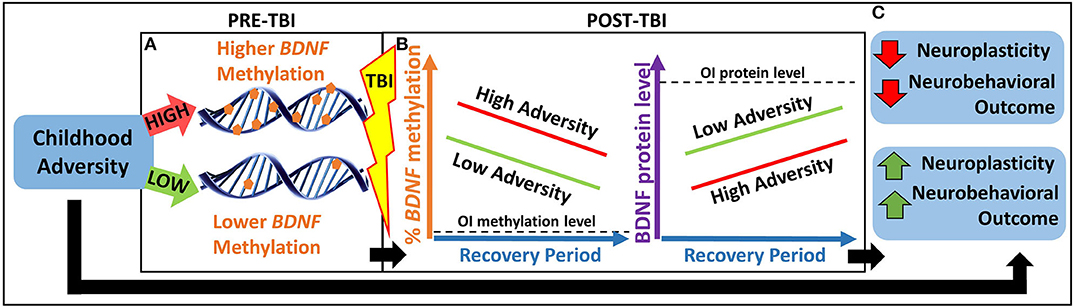
Figure 1. Heuristic model of our primary hypothesis. (A) Children with greater childhood adversity may have higher pre-TBI BDNF methylation, which may lead to (B) higher BDNF methylation and lower BDNF protein levels in response to TBI and over the recovery period, resulting in lower neuroplasticity and worse neurobehavioral outcomes. (C) This pathway may contribute to a biological explanation for the association between greater childhood adversity and worse neurobehavioral outcomes following pediatric TBI. BDNF, brain-derived neurotrophic factor; OI, orthopedic injury; TBI, traumatic brain injury.
The study uses an observational, prospective, longitudinal concurrent cohort design to examine the epigenetic influence of the biologically relevant BDNF pathway on neurobehavioral recovery in 200 children with nonpenetrating complicated mild to severe TBI relative to 100 children with orthopedic injury (OI) but no brain injury at three time points (acute, 6 months, 12 months) during the first year of recovery. As one of the first studies of epigenetic effects in pediatric TBI, this is an exploratory pilot study. The study will establish a longitudinal biorepository that is innovative in its integration of acute and chronic biological samples with comprehensive data characterizing preinjury childhood adversity and postinjury neurobehavioral functioning. Only a portion of each biosample will be used for the analyses proposed in the present study; the remaining biosamples will be stored in the biorepository. This rich biorepository will be expanded longitudinally in subsequent studies and will provide opportunities to evaluate other epigenetic, genetic, and protein biomarkers and biological mechanisms in future secondary analyses. The results of the present pilot study will guide the design of a subsequent larger multisite study that will be sufficiently powered to examine additional epigenetic biomarkers while controlling for additional sources of heterogeneity in outcomes.
Our specific aims and hypotheses are as follows:
Aim 1: Examine differences between TBI and OI in BDNF methylation and BDNF protein levels over the recovery period. We hypothesize that BDNF methylation will be higher, and BDNF protein levels will be lower, in children with TBI relative to OI at all time points, and that BDNF methylation will decrease, and BDNF protein levels will increase, in the TBI group over time but will remain relatively stable in the OI group.
Aim 2: Characterize trajectory classes of BDNF methylation and BDNF protein levels following TBI while controlling for potential demographic, injury, and lifestyle covariates. We hypothesize the presence of at least two BDNF methylation trajectory classes and two BDNF protein level trajectory classes representing varying degrees of recovery from TBI. We will explore age, sex, pubertal status, TBI severity, overall injury severity, body mass index (BMI), and smoking as potential covariates.
Aim 3: Test the indirect effect of childhood adversity on neurobehavioral recovery following pediatric TBI, as mediated by BDNF methylation and BDNF protein level trajectory classes.
Methods and Analysis
Study Overview
Children aged 3–18 years with either complicated mild to severe nonpenetrating TBI (n = 200) or OI (n = 100) are recruited from consecutive overnight admissions to the UPMC Children's Hospital of Pittsburgh (CHP). The sample size of 200 children with TBI and 100 children with OI was chosen based on two considerations: (1) feasibility of recruitment over the study period based on historic numbers of children with TBI meeting eligibility criteria at our site and (2) adequacy of statistical power for proposed analyses taking into consideration participant attrition over the study period (see section Statistical Methods and Power). We selected the age and severity ranges to optimize sample size for this initial exploratory pilot study and to examine the effects of demographic and injury factors as potential covariates to inform design of future studies. In addition, most neurobehavioral measures selected for use in the study have age-specific forms spanning this age range. To ensure an adequate distribution across TBI severity and because disproportionately more children sustain complicated mild relative to moderate to severe TBI, we will stratify TBI recruitment into two severity groups (complicated mild vs. moderate/severe) and recruit consecutively within each group until we have at least 20% of the total sample in the moderate to severe TBI group. Children with OI were selected as a comparison group to control for premorbid risk factors for injury of any kind and to equate for the experience of having a traumatic injury and associated medical treatment (73, 74). In addition, comparing biomarkers between traumatically injured children with and without injury to the brain will help differentiate general injury effects on systemic biomarkers from those that are specific to brain injury (75). Children with spinal cord injury are excluded from both the TBI and OI groups to avoid confounding from neuroinflammation and brain involvement.
Data are collected at three time points postinjury. Acute data collection occurs before hospital discharge, and chronic data collection occurs at 6 and 12 months postinjury during outpatient follow-up visits to CHP. Data are collected pertaining to hypothesized predictors of neurobehavioral recovery (e.g., demographics, injury information, child premorbid functioning, childhood adversity, and biomarkers), neurobehavioral outcomes (e.g., neuropsychological test performance, parent-rated neuropsychological, emotional/behavioral, and adaptive functioning), and potential covariates/confounders (e.g., BMI, pubertal status, tobacco/nicotine exposure, bodily injury severity). Recruitment began in July 2017 and will occur for ~6 years, with data collection complete by mid-2023.
DNA methylation patterns are tissue specific and reflect the local environment of each cell type, and examination of brain tissue is not feasible in patients who survive their injuries; therefore, determining which types of biosamples serve as effective proxies for the CNS environment is an important methodological consideration. Moreover, to be useful as a clinical biomarker, markers must be found in readily accessible biosamples, such as blood or saliva. Several previous studies have shown that methylation in peripheral biosamples, including blood and saliva, is strongly correlated with methylation in brain tissue or CSF (46, 76–81) for several target genes, including BDNF (46, 77). Methylation measured in peripheral biosamples may be of even greater prognostic value in TBI compared to neuropsychiatric conditions because brain-specific biomarkers may enter blood circulation in TBI not only through disruption to the blood–brain barrier but also through the glymphatic system (82). Finally, a recent review of the “tissue issue” concluded that the limitations of examining epigenetic brain biomarkers in peripheral tissues or biosamples are surmountable and discussed methodological advantages of using peripheral biosamples: serial biosampling, longitudinal study designs and related causal modeling options, large participant sample sizes yielding statistical power to detect modest effects, and translational utility in clinical settings (83).
In light of these methodological considerations, we collect saliva and blood biosamples at acute and chronic time points in all participants. We also collect acute serial CSF samples in the small subsample of participants with extraventricular drain (EVD) placement to manage intracranial pressure as part of guidelines based standard of care (84).
Inclusion Criteria
Children in both groups must be from 3 to 18 years of age at the time of the injury. Children with TBI must be hospitalized overnight for a complicated mild to severe nonpenetrating TBI as defined by the lowest postresuscitation Glasgow Coma Scale (GCS) score (85). Complicated mild TBI is defined as a GCS of 13–15 with neuroimaging indicating intracranial or parenchymal injury or depressed/displaced skull fracture. Moderate TBI is defined as GCS of 9–12. Severe TBI is defined as GCS of 3–8. Children are included in the OI group if they sustain a bone fracture, excluding to the skull or face, without any signs of head trauma or brain injury (e.g., nausea/vomiting, headache, loss of consciousness, GCS below 15 at any point).
Exclusion Criteria
Children in both the TBI and OI groups meet the following exclusion criteria: (a) non-English-speaking child or non-English-speaking parents/guardians; (b) documented or parent-reported history of previous TBI/concussion requiring overnight hospitalization; (c) preinjury neurological disorder or intellectual disability; (d) preinjury psychiatric disorder requiring hospitalization; (e) spinal cord injury; (f) sensory or motor impairment precluding study measure completion; and (g) pregnancy at the time of study participation. Participants are also excluded if at least one biosample is not able to be collected within 7 days of the injury. Children in the TBI group are not excluded if they also have an OI.
Study Procedures
The study staff screen the CHP electronic medical record (EMR) daily to identify children who are potentially eligible for study participation. Children are housed in one of two settings when they are identified: (1) the acute care trauma unit or (2) the pediatric intensive care unit (PICU). Children housed in the acute care trauma unit within the first week of their injury are approached about the study. The study staff approach families and conduct brief interviews about the child's preinjury functioning and medical history to confirm eligibility and obtain informed consent prior to any data collection. Interested parent(s) of minor children, and 18-year-old patients capable of consenting on their own behalf, provide written informed consent. Children over the age of 8 capable of understanding study procedures provide assent.
Children with more severe injuries are sometimes housed in the PICU during the acute biosample window (i.e., 1 week postinjury). If a potentially eligible child is in the PICU, the bedside nurse collects the acute blood sample at the same time as a clinical blood draw, before consent has been obtained per an Institutional Review Board (IRB)-approved waiver of informed consent. In addition, if a child with severe TBI has an EVD placed as part of routine clinical care, the bedside nurse collects daily CSF samples up to 1 week postinjury prior to study consent. Families of children whose samples are collected preconsent are approached about study participation once the child is medically stable, usually after discharge to the acute care trauma unit. If the family declines to participate or the child is discovered through screening questions to be ineligible, the preconsent samples are destroyed. Destruction of preconsent samples is requested by the research coordinator and completed by research nurses in the CHP Pediatric Clinical and Translational Research Center (PCTRC). Documentation of sample destruction is maintained both by the PCTRC and by the research coordinator.
For children and families who consent to study participation, an additional postconsent blood sample is obtained by the bedside nurse in addition to any preconsent acute blood and CSF samples. The study staff collect a saliva sample from the child. Prior to discharge, parents complete measures pertaining to demographic information, premorbid child functioning, childhood adversity, child resilience, and the child's pre- and postnatal exposure to nicotine/tobacco. Children 8 years and older complete a self-report measure of puberty status, and children 10 years and older also complete a self-report measure of tobacco/nicotine use. Injury information (e.g., mechanism, neuroimaging results, GCS) and BMI are obtained from the child's EMR.
The study staff contact families to schedule their 6- and 12-month follow-up research visits at CHP. Follow-up visits last ~90 min. At each visit, PCTRC research nurses collect blood via venipuncture, and the study staff collect saliva. Parents complete the same measures as the acute visit, this time to assess postinjury functioning (see Table 1 and Section Measures). Children complete the National Institutes of Health Toolbox-Cognition Battery (NIHTB-CB) and self-report measures of puberty and tobacco/nicotine use. Families who refuse to return for follow-up research visits are given the option to return parent study measures and the child saliva sample via mail. Families are provided with instructions for how to collect the saliva sample and complete the parent measures as well as return packaging and postage.
Families are compensated for their time at the acute and follow-up visits and reimbursed for parking and transportation costs. Multiple methods are used to track and contact participants and reduce attrition.
Measures
Table 1 provides an overview of study measures organized by domain, source of data, and assessment occasion.
Hypothesized Predictors
Demographics
Child and family demographic information is collected at both the acute time point and at the 6- and 12-month follow-ups. Parents answer questions about the child and family members' age, sex, race, ethnicity, and relationship to the child (e.g., biological parent, legal guardian, etc.), as well as socioeconomic status (e.g., family income, parent education level, parent employment, use of financial assistance programs), household composition, and marital status.
Injury Information
The study staff extract information about the characteristics of the child's injury from their EMR. Specifically, the child's initial, postresuscitation, and best in 24-h GCS scores, MRI or CT findings, date and time of injury, and mechanism of injury are noted.
Preinjury Child Function
At the acute visit, parents report on their child's preinjury functioning through retrospective recall. The efficacy of this method has been demonstrated in previous studies (86, 87).
To assess everyday executive functioning, parents complete the Behavior Rating Inventory of Executive Function, Second Edition (BRIEF-2) or Preschool Version (BRIEF-P) (88, 89). Three composite scores are computed for behavioral regulation, emotion regulation, and cognitive regulation, as well as a global executive composite.
The Strengths and Difficulties Questionnaire (SDQ) measures psychological adjustment. Subscales include Emotional Symptoms, Conduct Problems, Hyperactivity–Inattention, Peer Problems, and Prosocial Behavior. A Total Difficulties score is also provided. Four different versions are administered based on the child's age.
Adaptive functioning is measured using the Vineland Adaptive Behavior Scales, Third Edition (Vineland-3). Parents complete items designed to assess their child's ability to perform day-to-day activities in the domains of Communication, Daily Living, and Socialization. Composite scores are computed for each domain, as well as a general Adaptive Behavior Composite (90).
Childhood Adversity
Parents complete the Psychosocial Assessment Tool (PAT) as a measure of psychosocial risk and childhood adversity, particularly in the context of the family in a pediatric setting. The PAT evaluates a variety of domains: family structure and resources, social support, patient, sibling, and caregiver problems (internalizing, externalizing, social, cognitive), family beliefs, and caregiver stress reactions. Subscale scores are calculated for each of these domains, as well as a total PAT score (91).
Resilience may buffer the effects of childhood adversity (92) and has recently gained interest in predicting outcomes following TBI (93, 94). Parents complete the Resilience items from the Child Health and Illness Profile (CHIP), child edition (6–11 years; 19 items) or adolescent edition (12–18 years; 38 items) (95, 96). The Resilience domain measures the parent's assessment of the child's family involvement, social problem solving, and physical activity.
Biomarker Collection and Processing
CSF is collected by the bedside nurse into a CSF vial and refrigerated until processing within 48 h by the CHP PCTRC. Processing involves separation and aliquoting of supernatant for future protein level analysis and the cell pellet for genetic and epigenetic analysis. Processed samples are stored at −80°C.
Acute blood samples are collected by the child's bedside nurse from his or her IV if patent or via venipuncture with parent and child permission. Chronic blood samples are collected via venipuncture by a PCTRC nurse. Up to 10 cc of blood is collected at each draw into ethylenediaminetetraacetic acid (EDTA) vacuum tubes and refrigerated until processing within 48 h by the PCTRC. Processing involves separation and aliquoting of plasma for future protein level analysis and the buffy coat (leukocytes) for DNA extraction using a simple salting protocol for genetic and epigenetic analysis. Processed samples are stored at −80°C.
Up to 2 ml of saliva is collected by the study staff using Oragene DNA sample collection kits (DNA Genotek, Ontario, Canada). To collect saliva, the child spits into the Oragene collection tube for 2–5 min until liquid saliva reaches the fill line. If the child is unconscious or unable to spit, study staff instead use a sponge to gently swab the child's gums and inner cheeks and then wring the saliva out of the sponge into the collection tube until the fill line is reached. The study staff then close the tube's lid to release the stabilizing liquid and store the sample at ambient temperature until processing. DNA is extracted using the reagents and protocol from the manufacturer.
Outcomes
Child neuropsychological function is assessed acutely and at each follow-up visit using the NIHTB-CB (97). The NIHTB-CB is a 30-min battery of standardized neuropsychological tests administered on an iPad. The NIHTB-CB provides norm-referenced scores for the domains of language, episodic memory, processing speed, working memory, and executive function, as well as an overall cognitive function composite score.
Parent-reported child postinjury executive, emotional/ behavioral, and adaptive functioning are measured at follow-up visits using the BRIEF-2/P, SDQ, Vineland-3, and resilience items from the CHIP. Postinjury adversity is measured using the PAT and child resilience by the CHIP.
Potential Covariates/Confounds
BDNF methylation and protein levels vary by age, sex, and pubertal status (98–101), as well as lifestyle factors including BMI and smoking (101–103); therefore, we are collecting information on these factors at all time points to be investigated as potential covariates. We will also examine the effect of overall bodily injury severity because polytrauma can be a significant confounder in biomarker studies (75, 104).
Pubertal status is measured for children ≥8 years using the self-report Pubertal Development Scale (105). The scale provides a Pubertal Development Score, which shows adequate agreement with direct clinical assessment of Tanner staging (106).
The child's BMI is obtained at each visit from the EMR or study staff.
Parents are given a brief screener measuring the child's exposure to nicotine and tobacco. The screener measures maternal use and secondhand exposure during pregnancy, as well as the child's secondhand exposure. Children 10 and up also complete a brief self-report measure about their own nicotine and tobacco use. They are asked how frequently they have used a variety of nicotine/tobacco products. Children complete the questionnaire on paper while their parents are out of the room and are informed that their responses will be kept private.
The Injury Severity Score (ISS) (107) measures overall bodily injury severity and is obtained from the local trauma registry. The score for injury to the head will be excluded to avoid confounding with TBI severity.
Data Management
All electronic and paper data storage is IRB and HIPAA compliant through separation of identifiable and deidentified data, storage of paper data, and samples in locked cabinets in locked offices, use of secure servers, and encrypted and password-protected files and computers. Research Electronic Data Capture (REDCap) is used for electronic data storage. REDCap is a web-based platform that allows a common database for data entry and rules for data handling. All data that are entered into REDCap are checked for discrepancies using double verification and error checks.
Data Reduction, Missing Data, and Attrition
Measures were selected that provide composites or summary indices representing each construct, reducing the need for substantial data reduction. Children will be eligible to remain in the study if at least one acute biosample (CSF, blood, or saliva) is obtained and both primary parent questionnaires (BRIEF-2/P, SDQ) are completed. This will reduce missing data on key variables and retain participants who refuse blood collection or are unable to produce sufficient saliva. In addition, statistical techniques such as mixed models, latent class trajectory analysis, and path analysis will be used, which estimate models based on all available data using maximum likelihood estimation (108). Consistent with previous studies in this population, we conservatively estimate that ~75% of participants will complete the follow-up visits (150 TBI; 75 OI) (2, 4, 5). Our power analyses account for this reduced participant sample size at follow-up.
Data Analyses
Biomarker Analysis
DNA extracted from saliva, blood, and CSF will be processed for methylation analysis. We currently plan to use EpiTect Methyl II PCR assays (Qiagen, Germantown, MD) to assess targeted methylation sites in and around the BDNF gene; however, due to rapid changes in technology for this type of data collection, when data collection is to begin, we will evaluate whether this method remains our best choice. We will target a select number of BDNF CpG islands for this initial study that showed the strongest correlation between blood and CSF in our preliminary data (109) and show significant correlations between BDNF methylation in blood and postmortem prefrontal cortex brain tissue according to the searchable online Blood Brain DNA Methylation Comparison Tool (110). Additional BDNF methylation targets may be examined in secondary analyses. The resulting data will estimate the methylation level at each CpG site by beta values (i.e., percent methylation). Beta values range from zero to 1, with zero indicating a null methylated site and 1 indicating a fully methylated site. M values will then be calculated and used in statistical analysis. (111, 112) Plasma BDNF protein levels will be measured using an ELISA kit according to the manufacturer's instructions. BDNF ELISA kits are available from several manufacturers (e.g., RayBiotech, R&D Systems). We will select a manufacturer at the time of analysis based on available specifications. For quality control, biosamples will be batch analyzed, assays will include biological duplicates and technical replicates, and data will be evaluated by two individuals blinded to phenotype.
Before our main analyses, we will examine the concordance of BDNF methylation across saliva, blood, and CSF and compare the predictive value of epigenetic results across biosample types. Based on these results, we may determine that saliva or blood biosamples are more informative than the other, and these results will guide which biosample(s) are used for measurement of BDNF methylation in our primary analyses.
Statistical Methods and Power
Aim 1: Examine differences between TBI and OI in BDNF methylation and BDNF protein levels over the recovery period
Unadjusted BDNF methylation and protein levels will be compared between TBI and OI groups using mixed effects analysis of variance (ANOVA; time as within effect; group as between effect). Mixed effects ANOVA allows for the modeling of effects on continuous outcomes (BDNF methylation and protein levels), is flexible with missing data, and provides explicit estimation of between group and within group variance. We will use appropriate transformations if normality is violated. We expect a significant group × time interaction and group differences at each time point. Alpha will be set at 0.05, and we will adjust for multiple comparisons using Bonferroni corrections. We expect sufficient power (≥0.8) to detect even a small interaction effect (partial η2 = 0.01) assuming correlation of 0.5 between repeated measures (G*Power 3.1.9.2).
Aim 2: Characterize trajectory classes of BDNF methylation and BDNF protein levels following TBI while controlling for potential demographic, injury, and lifestyle covariates
We will use latent class trajectory analysis (LCTA) (113) of BDNF methylation and protein levels separately, with the goal of identifying clusters of children with TBI who follow similar recovery trajectories. Some of the major benefits of LCTA are identification of distinct classes, simplification of complex data, and identification of adverse trajectories for potential intervention targets. Another strength given our heterogeneous sample is that probability of membership in each latent class is identified based on biomarker values over time as well as scores on significant covariates. Covariates to be tested will include age, sex, pubertal status, TBI severity, overall injury severity, BMI, and smoking. We will examine biomarker distributions to determine the need for transformations (114). Model selection will involve the iterative estimation of the number and shape of trajectory classes and identification of the best fitting model utilizing the following verified fit indices: Bayesian information criterion statistic, model estimation convergence, percentage of population in each subgroup (>10%), minimization of the residual variance statistic, and examination of posterior subgroup classification probabilities (115–117). Although the literature on power analyses for LCTA is limited (117), the power to identify the appropriate number of classes is highly dependent on separation of the trajectories (115). We believe our final sample is sufficient for several reasons: (1) we have a strong theoretical basis for our hypothesis of at least two classes and expect the classes to be relatively distinct; (2) we intend to evaluate different forms of the extracted model using verified fit indices (115); and (3) LCTA with covariates has been successfully applied in smaller TBI samples, n = 100–138 (75, 118–120).
Aim 3: Test the indirect effect of childhood adversity on neurobehavioral recovery following pediatric TBI, as mediated by BDNF methylation and BDNF protein level trajectory classes
We will conduct path analysis to test the theoretical model shown in Figure 2 within the TBI group. The theoretical model represents the relation among constructs rather than specific measures to allow for fluidity in our approach. For example, we will examine this model for each of the four primary measures of neurobehavioral outcome (NIHTB-CB, BRIEF-2/P, SDQ, Vineland-3). The specific biosamples used for BDNF methylation will be informed by preliminary analyses (see Biomarker Analysis). Path analysis enables a graphical representation of complex relationships, can explore direct and indirect effects among the variables (e.g., mediation), and infer causal directionality between effects. BDNF methylation and protein level trajectory class adjusted for covariates (Aim 2) will be tested as categorical mediators. Neurobehavioral recovery on each outcome will be modeled controlling for premorbid functioning (with the exception of NIHTB-CB). We estimated sample size requirements to adequately power the three single mediator models that would comprise our double-mediator model, as current guidelines on sample size for double mediator models are limited. The power of a single mediator model depends on the effect of the independent variable (IV) on the mediator and the effect of the mediator on the DV, controlling for the IV. Using Cohen's effect size criteria, we expect to have sufficient power (≥0.8) if both effects are at least “halfway” (between small and medium effects) (121). However, we would only need 115 participants if one effect is “halfway” and the other is medium. Thus, our final sample is conservative.
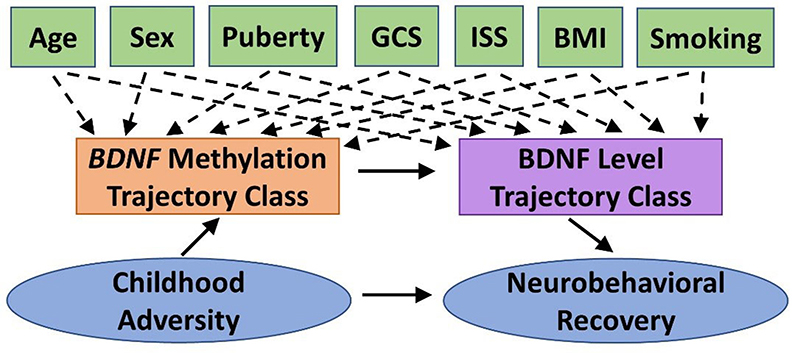
Figure 2. Theoretical model guiding Aim 3 statistical analyses. We will test the indirect effect of childhood adversity on neurobehavioral recovery following pediatric traumatic brain injury (TBI), as mediated by BDNF methylation and BDNF protein level trajectory classes while adjusting for potential covariates and confounders. BDNF, brain-derived neurotrophic factor; BMI, body mass index; GCS, Glasgow Coma Scale; ISS, Injury Severity Score.
Ethics and Dissemination
The study received ethics approval from the University of Pittsburgh Institutional Review Board, STUDY19040402. No significant risks are associated with participation in the study, and participation does not affect the care provided to participants.
The waiver of informed consent does not adversely affect the rights and welfare of the research participants because the participants are already undergoing blood draws for clinical care and the additional research blood draw alongside the clinical blood draw does not increase risk to the participant. In addition, the collection of CSF from the EVD does not impose any risk to the participant, and CSF samples would otherwise be discarded. Finally, the waiver does not adversely affect the rights and welfare of the research participants because consent will be obtained from the parent/child prior to drawing additional samples and the preconsent samples are destroyed if the parent/child does not consent to the study.
The study procedures are associated with a minimal risk of parent or child psychological discomfort. Parents and children are only approached with the bedside nurse's approval once the child is medically stable. The study staff approach families sensitively and respectfully to minimize any discomfort associated with the study and terminate all procedures immediately upon request or if the child or parents are experiencing any significant overt discomfort.
Although families do not receive any direct benefit for their participation, they do receive several incentives at each time point. Parents receive a letter summarizing their child's results on the NIHTB-CB along with the offer of referral for outpatient neuropsychological evaluation if indicated. All families of children with TBI receive brochures about CHP clinical neuropsychology services and other local brain injury resources.
Personal biomarker results will not be disclosed to research participants for several reasons: (1) the biological samples are being analyzed solely in the context of a research study; (2) the research findings, if provided, may be clinically irrelevant to available strategies for treatment; and (3) the research laboratory is not Clinical Laboratory Improvement Amendments (CLIA) certified to provide data upon which to base subsequent clinical decisions. The consent form describes that additional biomarkers may be examined in participant biosamples in the future.
The study staff are trained to ensure that data are secure and confidentiality is maintained at all times. All staff sign a confidentiality agreement and are only given the minimum access to data and patient information necessary to perform their duties. All identifiable data are kept in a secure and locked private office and is accessible only to study staff. Biological samples and all study forms are labeled with nonidentifying ID codes, and any links between the ID codes and identifiers are stored separately. Any data that are exported from the secure REDCap database is deidentified prior to export unless the identifier is necessary for analysis.
Research findings will be disseminated via local and international conference presentations and manuscripts submitted to peer-reviewed journals.
Discussion
Strengths and Potential Limitations
Our study has several strengths and potential limitations. In terms of strengths, the epigenetic focus, establishment of a longitudinal biorepository, and administration of the PAT and NIHTB-CB are, to our knowledge, novel contributions relative to previous large-scale cohort studies in pediatric TBI. The study of an epigenetic biomarker integrates the effects of genetics and the child's environmental context on recovery, providing a potentially powerful explanation of heterogeneity in neurobehavioral outcomes following pediatric TBI. Comparing biomarkers between traumatically injured children with and without injury to the brain will help differentiate general injury effects on systemic biomarkers from those that are specific to brain injury. The biorepository established for this study is innovative in its integration of acute and chronic biological samples with comprehensive data characterizing preinjury childhood adversity and postinjury neurobehavioral functioning and can be built upon longitudinally in subsequent studies, providing opportunities for the evaluation of many other epigenetic, genetic, and protein biomarkers and biological mechanisms contributing to recovery from pediatric TBI.
Regarding potential weaknesses, the wide age range and breadth of TBI severity of participants introduces greater heterogeneity than more restrictive age and severity inclusion criteria. Given that this is a single-site exploratory pilot study, however, we chose to be more inclusive to ensure a sufficient sample size for the proposed analyses. The effects of demographic and injury factors will be explored as potential covariates to inform the design of future studies. Similarly, BDNF methylation can be affected by other factors that are not measured in our study, including alcohol and drug exposure, diet, and exercise. We would look to consider these factors in future larger studies. Whether methylation in peripheral biosamples provides meaningful information regarding methylation in the brain is an inherent challenge to epigenetic research and remains to be further elucidated. Should the targets examined fail to show correlation of BDNF methylation between peripheral biosamples (blood and saliva) and CSF or fail to show associations with childhood adversity and neurobehavioral outcomes, the establishment of the biorepository in this study will allow for many additional epigenetic biomarkers to be explored. Finally, we acknowledge that BDNF is only one of many epigenetic pathways potentially influencing recovery. Biomarkers of interest to our research questions and available for subsequent analyses will include additional BDNF methylation targets, BDNF, neurotransmitter (17, 122) and inflammatory (123) genotypes, and additional biomarkers related to neuroplasticity and inflammation, given their role in both childhood adversity and recovery from TBI.
Significance
Successful completion of the proposed aims has the potential to improve patient outcomes by advancing the field of pediatric TBI toward precision rehabilitation medicine. The unexplained heterogeneity in outcomes that plagues the field of TBI suggests a critical need to harness the potential of personal biology in predicting individual patient outcomes and response to interventions. The measurement of BDNF methylation and levels from accessible biosamples may serve as an early postinjury biomarker that more accurately predicts neurobehavioral outcomes following pediatric TBI, thereby allowing for earlier identification of children at greatest risk for poor recovery and the early provision of targeted intervention. Further understanding of the role of BDNF in recovery from TBI and the preinjury environmental determinants of its expression may bring us closer to being able to translate interventions to humans that have increased BDNF expression and improved cognitive outcomes in animal models, namely, environmental enrichment (63, 124) and exercise (125, 126). Finally, no neuroprotective therapies are currently available for TBI (127). Thus, demonstrating a possible role for BDNF epigenetics in mediating neurobehavioral outcomes will identify BDNF methylation as a possible target for the development of medical therapeutics. Interventions using epigenetic-based therapies have shown promise in experimental models of TBI (128, 129). This work will provide the foundation to develop biologically grounded, personalized approaches to treatment and rehabilitation that may apply across multiple child and adult brain disorders.
Author Contributions
AT-B, KY, and YC designed the study with input from EF, PK, and CZ. AT-B and YC developed the biosampling protocol and analysis plan. AT-B and CZ developed the biostatistical plan. AT-B and JP wrote the first draft of the manuscript. JP, SU, and NM collected data and contributed to procedure improvements and clinical research coordination. All authors read, critically revised, and approved the final version of the manuscript.
Funding
This research was supported in part by grants K01 HD097030 (PI AT-B) and T32 HD040686 (PI PK) from the National Institute of Child Health and Human Development (NICHD) and KL2 TR001856 (PI Rubio) from the National Center for Advancing Translational Sciences (NCATS), the Society for Clinical Neuropsychology Early Career Pilot Award (PI AT-B), the UPMC Children's Hospital of Pittsburgh Scientific Program (PI AT-B), and the University of Pittsburgh/UPMC Rehabilitation Institute Pilot Award (PI AT-B). The content is solely the responsibility of the authors and does not necessarily represent the official views of the funders.
Conflict of Interest
The authors declare that the research was conducted in the absence of any commercial or financial relationships that could be construed as a potential conflict of interest.
Acknowledgments
The authors thank all current and future participants and their families, as well as the CHP PICU, Trauma Unit, and Inpatient Rehabilitation Unit staff, for their support of the study. The authors also want to thank the research coordinators, research assistants, and other staff involved in the conduct of the study (in alphabetical order): Nicholas Cardamone, Sandra Deslouches, Lauren Kaseman, Leah Kelly, Aboli Kesbhat, Lindsay Kutash, Greta Lyons, Sophie McCormick, Amy Papinchak, Sameer Shetty, Namita Thomas, and the Children's Hospital of Pittsburgh Pediatric Clinical and Translational Research Center staff.
References
1. Taylor CA, Bell JM, Breiding MJ, Xu L. Traumatic brain injury-related emergency department visits, hospitalizations, and deaths - united states, 2007 and 2013. MMWR Surveill Summ. (2017) 66:1–16. doi: 10.15585/mmwr.ss6609a1
2. Treble-Barna A, Zang H, Zhang N, Taylor HGG, Yeates KO, Wade SL. Long-term neuropsychological profiles and their role as mediators of adaptive functioning after traumatic brain injury in early childhood. J Neurotrauma. (2017) 34:353–62. doi: 10.1089/neu.2016.4476
3. Treble-Barna A, Schultz H, Minich N, Taylor H, Yeates K, Stancin T, et al. Long-term classroom functioning and its association with neuropsychological and academic performance following traumatic brain injury during early childhood. Neuropsychology. (2017) 31:486–98. doi: 10.1037/neu0000325
4. Treble-Barna A, Zang H, Zhang N, Taylor HGG, Stancin T, Yeates KOKO, et al. Observed parent behaviors as time-varying moderators of problem behaviors following traumatic brain injury in young children. Dev Psychol. (2016) 52:1777–92. doi: 10.1037/dev0000208
5. Yeates KO, Taylor HG, Walz NC, Stancin T, Wade SL. The family environment as a moderator of psychosocial outcomes following traumatic brain injury in young children. Neuropsychology. (2010) 24:345–56. doi: 10.1037/a0018387
6. Anderson V, Catroppa C, Morse S, Haritou F, Rosenfeld J. Functional plasticity or vulnerability after early brain injury? Pediatrics. (2005) 116:1374–82. doi: 10.1542/peds.2004-1728
7. Keenan HT, Clark AE, Holubkov R, Cox CS, Ewing-Cobbs L. Psychosocial and executive function recovery trajectories one year after pediatric traumatic brain injury: the influence of age and injury severity. J Neurotrauma. (2018) 35:286–96. doi: 10.1089/neu.2017.5265
8. Ewing-Cobbs L, Prasad MR, Kramer L, Cox CS, Baumgartner J, Fletcher S, et al. Late intellectual and academic outcomes following traumatic brain injury sustained during early childhood. J Neurosurg. (2006) 105:287–96. doi: 10.3171/ped.2006.105.4.287
9. Ewing-Cobbs L, Fletcher JM, Levin HS, Frances DJ, Davidson K, Miner ME. Longitudinal neuropsychological outcome in infants and preschoolers with traumatic brain injury. J Int Neuropsychol Soc. (1997) 3:581–91. doi: 10.1017/S135561779700581X
10. Keenan HT, Runyan DK, Nocera M. Child outcomes and family characteristics 1 year after severe inflicted or noninflicted traumatic brain injury. Pediatrics. (2006) 117:317–24. doi: 10.1542/peds.2005-0979
11. Lindsey HM, Wilde EA, Caeyenberghs K, Dennis EL. Longitudinal neuroimaging in pediatric traumatic brain injury: current state and consideration of factors that influence recovery. Front Neurol. (2019) 10:1296. doi: 10.3389/fneur.2019.01296
12. Roberts RM, Mathias JL, Rose SE. Relationship between diffusion tensor imaging (DTI) findings and cognition following pediatric tBI: a Meta-Analytic review. Dev Neuropsychol. (2016) 41:176–200. doi: 10.1080/87565641.2016.1186167
13. Maas AIR, Lingsma HF, Roozenbeek B. Predicting outcome after traumatic brain injury. Handb Clin Neurol. (2015) 128:455–74. doi: 10.1016/B978-0-444-63521-1.00029-7
14. Saatman KE, Duhaime A-C, Bullock R, Maas AIR, Valadka A, Manley GT. Classification of traumatic brain injury for targeted therapies. J Neurotrauma. (2008) 25:719–38. doi: 10.1089/neu.2008.0586
15. Hawryluk GWJ, Manley GT. Classification of traumatic brain injury: past, present, and future. Handb Clin Neurol. (2015) 127:15–21. doi: 10.1016/B978-0-444-52892-6.00002-7
16. Kurowski BG, Treble-Barna A, Pitzer AJ, Wade SL, Martin LJ, Chima RS, et al. Applying systems biology methodology to identify genetic factors possibly associated with recovery after traumatic brain injury. J Neurotrauma. (2017) 34:2280–90. doi: 10.1089/neu.2016.4856
17. Treble-Barna A, Wade SL, Martin LJ, Pilipenko V, Yeates KO, Taylor HG, et al. Influence of dopamine-related genes on neurobehavioral recovery after traumatic brain injury during early childhood. J Neurotrauma. (2017) 34:1919–31. doi: 10.1089/neu.2016.4840
18. Myrga JM, Failla MD, Ricker JH, Dixon CE, Conley YP, Arenth PM, et al. A dopamine pathway gene risk score for cognitive recovery following traumatic brain injury: methodological considerations, preliminary findings, and interactions with sex. J Head Trauma Rehabil. (2015) 31:E15–E29. doi: 10.1097/HTR.0000000000000199
19. Nikolova YS, Hariri AR. Can we observe epigenetic effects on human brain function? Trends Cogn Sci. (2015) 19:366–73. doi: 10.1016/j.tics.2015.05.003
20. Egger G, Liang G, Aparicio A, Jones PA. Epigenetics in human disease and prospects for epigenetic therapy. Nature. (2004) 429:457–63. doi: 10.1038/nature02625
21. Vaiserman AM. Epigenetic programming by early-life stress: evidence from human populations. Dev Dyn An Off Publ Am Assoc Anat. (2015) 244:254–65. doi: 10.1002/dvdy.24211
22. Szyf M, Bick J. DNA methylation: a mechanism for embedding early life experiences in the genome. Child Dev. (2013) 84:49–57. doi: 10.1111/j.1467-8624.2012.01793.x
23. Weder N, Zhang H, Jensen K, Yang BZ, Simen A, Jackowski A, et al. Child abuse, depression, and methylation in genes involved with stress, neural plasticity, and brain circuitry. J Am Acad Child Adolesc Psychiatry. (2014) 53:417–24.e5. doi: 10.1016/j.jaac.2013.12.025
24. Grigorenko EL, Kornilov SA, Naumova OY. Epigenetic regulation of cognition: a circumscribed review of the field. Dev Psychopathol. (2016) 28:1285–304. doi: 10.1017/S0954579416000857
25. Cicchetti D, Hetzel S, Rogosch FA, Handley ED, Toth SL. An investigation of child maltreatment and epigenetic mechanisms of mental and physical health risk. Dev Psychopathol. (2016) 28:1305–17. doi: 10.1017/S0954579416000869
26. Tyrka AR, Parade SH, Welch ES, Ridout KK, Price LH, Marsit C, et al. Methylation of the leukocyte glucocorticoid receptor gene promoter in adults: associations with early adversity and depressive, anxiety and substance-use disorders. Transl Psychiatry. (2016) 6:e848. doi: 10.1038/tp.2016.112
27. Dadds MR, Schollar-Root O, Lenroot R, Moul C, Hawes DJ. Epigenetic regulation of the dRD4 gene and dimensions of attention-deficit/hyperactivity disorder in children. Eur Child Adolesc Psychiatry. (2016) 25:1081–1089. doi: 10.1007/s00787-016-0828-3
28. Cattane N, Rossi R, Lanfredi M, Cattaneo A. Borderline personality disorder and childhood trauma: exploring the affected biological systems and mechanisms. BMC Psychiatry. (2017) 17:221. doi: 10.1186/s12888-017-1383-2
29. Max JE, Schachar RJ, Levin HS, Ewing-Cobbs L, Chapman SB, Dennis M, et al. Predictors of secondary attention-deficit/hyperactivity disorder in children and adolescents 6 to 24 months after traumatic brain injury. J Am Acad Child Adolesc Psychiatry. (2005) 44:1041–9. doi: 10.1097/01.chi.0000173292.05817.f8
30. Gerring JP, Wade SL. The essential role of psychosocial risk and protective factors in pediatric traumatic brain injury research. J Neurotrauma. (2012) 29:621–8. doi: 10.1089/neu.2011.2234
32. Lu B, Pang PT, Woo NH. The yin and yang of neurotrophin action. Nat Rev Neurosci. (2005) 6:603–14. doi: 10.1038/nrn1726
33. Chen Z, Patel P, Sant G, Meng C, Teng K, Hempstead B, et al. Variant brain-Derived neurotrophic factor (BDNF) (Met66) alters the intracellular trafficking and activity-dependent secretion of wild-type bDNF in neurosecretory cells and cortical neurons. J Neurosci. (2004) 24:4401–11. doi: 10.1523/JNEUROSCI.0348-04.2004
34. Huang EJA, Reichardt LF. Neurotrophins: roles in neuronal development and function. Annu Rev Neurosci. (2001) 24:677–736. doi: 10.1146/annurev.neuro.24.1.677
35. Cortés-Mendoza J, Díaz de León-Guerrero S, Pedraza-Alva G, Pérez-Martínez L. Shaping synaptic plasticity: the role of activity-mediated epigenetic regulation on gene transcription. Int J Dev Neurosci. (2013) 31:359–69. doi: 10.1016/j.ijdevneu.2013.04.003
36. Manju S, Vignoli B, Canossa M, Blum R. Neurobiology of local and intercellular bDNF signaling. Pflugers Arch Eur J Physiol. (2017) 3:1–18. doi: 10.1007/s00424-017-1964-4
37. Leckie RL, Oberlin LE, Voss MW, Prakash RS, Szabo-Reed A, Chaddock-Heyman L, et al. BDNF mediates improvements in executive function following a 1-year exercise intervention. Front Hum Neurosci. (2014) 8:1–2. doi: 10.3389/fnhum.2014.00985
38. Wiłkość M, Szałkowska A, Skibinska M, Zajac-Lamparska L, Maciukiewicz M, Araszkiewicz A. BDNF gene polymorphisms and haplotypes in relation to cognitive performance in polish healthy subjects. Acta Neurobiol Exp (Wars). (2016) 76:43–52. doi: 10.21307/ane-2017-004
39. van der Kolk NM, Speelman AD, van Nimwegen M, Kessels RPC, IntHout J, Hakobjan M, et al. BDNF polymorphism associates with decline in set shifting in parkinson's disease. Neurobiol Aging. (2015) 36:1605.e1-e6. doi: 10.1016/j.neurobiolaging.2014.08.023
40. Koven NS, Collins LR. Urinary brain-derived neurotrophic factor as a biomarker of executive functioning. Neuropsychobiology. (2014) 69:227–34. doi: 10.1159/000362242
41. Egan MF, Kojima M, Callicott JH, Goldberg TE, Kolachana BS, Bertolino A, et al. The bDNF val66met polymorphism affects activity-dependent secretion of bDNF and human memory and hippocampal function. Cell. (2003) 112:257–69. doi: 10.1016/S0092-8674(03)00035-7
42. Ursini G, Cavalleri T, Fazio L, Angrisano T, Iacovelli L, Porcelli A, et al. BDNF rs6265 methylation and genotype interact on risk for schizophrenia. Epigenetics. (2016) 11:11–23. doi: 10.1080/15592294.2015.1117736
43. Januar V, Ancelin ML, Ritchie K, Saffery R, Ryan J. BDNF promoter methylation and genetic variation in late-life depression. Transl Psychiatry. (2015) 5:e619–7. doi: 10.1038/tp.2015.114
44. Kang H, Kim K, Kim J, Kim S, Park M, Kim H, et al. A longitudinal study of the associations of bDNF genotype and methylation with poststroke anxiety. Int J Geriatr Psychiatry. (2019) 34:1706–14. doi: 10.1002/gps.5185
45. Lewin GR, Carter BD. Neurotrophic factors. Berlin: Springer (2014). doi: 10.1007/978-3-642-45106-5
46. Hing B, Sathyaputri L, Potash JB. A comprehensive review of genetic and epigenetic mechanisms that regulate bDNF expression and function with relevance to major depressive disorder. Am J Med Genet Part B Neuropsychiatr Genet. (2018) 177:143–67. doi: 10.1002/ajmg.b.32616
47. Martinowich K, Hattori D, Wu H, Fouse S, He F, Hu Y, et al. DNA methylation - related chromatin remodeling in activity- dependent bdnf gene regulation. Science. (2003) 302:890–4. doi: 10.1126/science.1090842
48. Li M, Du W, Shao F, Wang W. Cognitive dysfunction and epigenetic alterations of the bDNF gene are induced by social isolation during early adolescence. Behav Brain Res. (2016) 313:177–83. doi: 10.1016/j.bbr.2016.07.025
49. Seo MK, Ly NN, Lee CH, Cho HY, Choi CM, Nhu LH, et al. Early life stress increases stress vulnerability through bDNF gene epigenetic changes in the rat hippocampus. Neuropharmacology. (2016) 105:388–97. doi: 10.1016/j.neuropharm.2016.02.009
50. Blaze J, Asok A, Roth TL. The long-term impact of adverse caregiving environments on epigenetic modifications and telomeres. Front Behav Neurosci. (2015) 9:79. doi: 10.3389/fnbeh.2015.00079
51. Perroud N, Salzmann A, Prada P, Nicastro R, Hoeppli M-E, Furrer S, et al. Response to psychotherapy in borderline personality disorder and methylation status of the bDNF gene. Transl Psychiatry. (2013) 3:e207. doi: 10.1038/tp.2012.140
52. Unternaehrer E, Meyer AH, Burkhardt SCA, Dempster E, Staehli S, Theill N, et al. Childhood maternal care is associated with dNA methylation of the genes for brain-derived neurotrophic factor (bDNF) and oxytocin receptor (oXTR) in peripheral blood cells in adult men and women. Stress. (2015) 18:451–61. doi: 10.3109/10253890.2015.1038992
53. Thaler L, Gauvin L, Joober R, Groleau P, de Guzman R, Ambalavanan A, et al. Methylation of bDNF in women with bulimic eating syndromes: associations with childhood abuse and borderline personality disorder. Prog Neuro-Psychopharmacology Biol Psychiatry. (2014) 54:43–9. doi: 10.1016/j.pnpbp.2014.04.010
54. Heinrich H, Grunitz J, Stonawski V, Frey S, Wahl S, Albrecht B, et al. Attention, cognitive control and motivation in aDHD: linking event-related brain potentials and dNA methylation patterns in boys at early school age. Sci Rep. (2017) 7:3823. doi: 10.1038/s41598-017-03326-3
55. Kuban KCK, Heeren T, O'Shea TM, Joseph RM, Fichorova RN, Douglass L, et al. Among children born extremely preterm a higher level of circulating neurotrophins is associated with lower risk of cognitive impairment at school age. J Pediatr. (2018) 201:40–48.e4. doi: 10.1016/j.jpeds.2018.05.021
56. Newton DF, Naiberg MR, Andreazza AC, Scola G, Dickstein DP, Goldstein BI. Association of lipid peroxidation and brain-Derived neurotrophic factor with executive function in adolescent bipolar disorder. Psychopharmacology. (2017) 234:647–56. doi: 10.1007/s00213-016-4500-x
57. Zeni CP, Tramontina S, Aguiar BW, Salatino-Oliveira A, Pheula GF, Sharma A, et al. BDNF val66Met polymorphism and peripheral protein levels in pediatric bipolar disorder and attention-deficit/hyperactivity disorder. Acta Psychiatr Scand. (2016) 134:268–74. doi: 10.1111/acps.12587
58. Zwipp J, Hass J, Schober I, Geisler D, Ritschel F, Seidel M, et al. Serum brain-derived neurotrophic factor and cognitive functioning in underweight, weight-recovered and partially weight-recovered females with anorexia nervosa. Prog Neuro-Psychopharmacology Biol Psychiatry. (2014) 54:163–9. doi: 10.1016/j.pnpbp.2014.05.006
59. Li H, Liu L, Tang Y, Ji N, Qian Q, Wang Y. Sex-specific association of brain-derived neurotrophic factor (BDNF) val66Met polymorphism and plasma bDNF with attention-deficit/hyperactivity disorder in a drug-naïve han chinese sample. Psychiatry Res. (2014) 217:191–7. doi: 10.1016/j.psychres.2014.03.011
60. Taurines R, Segura M, Schecklmann M, Albantakis L, Grünblatt E, Walitza S, et al. Altered peripheral bDNF mRNA expression and bDNF protein concentrations in blood of children and adolescents with autism spectrum disorder. J Neural Transm. (2014) 121:1117–28. doi: 10.1007/s00702-014-1162-x
61. Rostami E, Krueger F, Plantman S, Davidsson J, Agoston D, Grafman J, et al. Alteration in bDNF and its receptors, full-length and truncated trkB and p75NTR following penetrating traumatic brain injury. Brain Res. (2014) 1542:195–205. doi: 10.1016/j.brainres.2013.10.047
62. Hicks RR, Numan S, Dhillon HS, Prasad MR, Seroogy KB. Alterations in bDNF and nT-3 mRNAs in rat hippocampus after experimental brain trauma. Mol Brain Res. (1997) 48:401–6. doi: 10.1016/S0169-328X(97)00158-7
63. Chen X, Li Y, Kline AE, Dixon CE, Zafonte RD, Wagner AK. Gender and environmental effects on regional brain-derived neurotrophic factor expression after experimental traumatic brain injury. Neuroscience. (2005) 135:11–7. doi: 10.1016/j.neuroscience.2005.05.041
64. Munoz MJ, Kumar RG, Oh B, Conley YP. Cerebrospinal fluid cortisol mediates brain-Derived neurotrophic factor relationships to mortality after severe tBI : a Prospective cohort study. Front Mol Neurosci. (2017) 10:44. doi: 10.3389/fnmol.2017.00044
65. Failla MD, Conley YP, Wagner AK. Brain-Derived neurotrophic factor (BDNF) in traumatic brain injury-related mortality: interrelationships between genetics and acute systemic and central nervous system bDNF profiles. Neurorehabil Neural Repair. (2016) 30:83–93. doi: 10.1177/1545968315586465
66. Korley FK, Diaz-Arrastia R, Wu AHB, Yue JK, Manley GT, Sair HI, et al. Circulating brain-Derived neurotrophic factor has diagnostic and prognostic value in traumatic brain injury. J Neurotrauma. (2016) 33:215–25. doi: 10.1089/neu.2015.3949
67. Schuster A, Treble-Barna A, Dekman M, Wagner AK. Chronic serum bDNF associations with global outcome and disability post-TBI. J Neurotrauma. (2018) 35:A275. doi: 10.1089/neu.2018.29013.abstracts
68. Failla MD, Juengst SB, Arenth PM, Wagner AK. Preliminary associations between brain-Derived neurotrophic factor, memory impairment, functional cognition, and depressive symptoms following severe tBI. Neurorehabil Neural Repair. (2016) 30:419–30. doi: 10.1177/1545968315600525
69. Treble-Barna A, Schuster A, Dekman M, Wagner AK. Serum bDNF at 6 months following tBI in adults predicts chronic functional cognitive impairment and post-traumatic depression. J Neurotrauma. (2018) 35:A273.
70. Chiaretti A, Piastra M, Polidori G, Di Rocco C, Caresta E, Antonelli A, et al. Correlation between neurotrophic factor expression and outcome of children with severe traumatic brain injury. Intensive Care Med. (2003) 29:1329–38. doi: 10.1007/s00134-003-1852-6
71. Pinelis VG, Sorokina EG, Semenova JB, Karaseva OV, Mescherayakov SV, Chernisheva TA, et al. Biomarkers in children with traumatic brain inury. Zh Nevrol Psikhiatr Im S S Korsakova. (2015) 115:66–72. doi: 10.17116/jnevro20151158166-72
72. Madurski C, Beers SR, Houtrow A, Smith C, Doughty L, Feldman K, et al. Serum biomarker relationships with functional impairment following acute pediatric brain injury. Crit Care Med. (2018) 46:393. doi: 10.1097/01.ccm.0000528827.52141.9a
73. Asarnow R, Satz P, Light R, McCleary C. The uCLA study of mild closed head injury in children and adolescents. Trauma Brain Inj Child. (1995) 67:653–8.
74. Yeates KO. Mild traumatic brain injury and postconcussive symptoms in children and adolescents. J Int Neuropsychol Soc. (2010) 16:953–60. doi: 10.1017/S1355617710000986
75. Kumar RG, Diamond ML, Boles JA, Berger RP, Tisherman SA, Kochanek PM, et al. Acute cSF interleukin-6 trajectories after tBI: associations with neuroinflammation, polytrauma, and outcome. Brain Behav Immun. (2015) 45:253–62. doi: 10.1016/j.bbi.2014.12.021
76. Liu B, Cheng J, Zhang W, Zhang X, Wang R, Lin H, et al. Quantitative detection of multiple gene promoter hypermethylation in tumor tissue, serum, and cerebrospinal fluid predicts prognosis of malignant gliomas. Neuro Oncol. (2010) 12:540–8. doi: 10.1093/neuonc/nop064
77. Stenz L, Zewdie S, Laforge-Escarra T, Prados J, La Harpe R, Dayer A, et al. BDNF promoter i methylation correlates between post-mortem human peripheral and brain tissues. Neurosci Res. (2015) 91:1–7. doi: 10.1016/j.neures.2014.10.003
78. Masliah E, Dumaop W, Galasko D, Desplats P. Distinctive patterns of dNA methylation associated with parkinson disease: identification of concordant epigenetic changes in brain and peripheral blood leukocytes. Epigenetics. (2013) 8:1030–8. doi: 10.4161/epi.25865
79. Davies MN, Volta M, Pidsley R, Lunnon K, Dixit A, Lovestone S, et al. Functional annotation of the human brain methylome identifies tissue-specific epigenetic variation across brain and blood. Genome Biol. (2012) 13:R43. doi: 10.1186/gb-2012-13-6-r43
80. Smith AK, Kilaru V, Klengel T, Mercer KB, Bradley B, Conneely KN, et al. DNA extracted from saliva for methylation studies of psychiatric traits: evidence tissue specificity and relatedness to brain. Am J Med Genet Part B Neuropsychiatr Genet. (2015) 168:36–4. doi: 10.1002/ajmg.b.32278
81. Marioni RE, McRae AF, Bressler J, Colicino E, Hannon E, Li S, et al. Meta-analysis of epigenome-wide association studies of cognitive abilities. Mol Psychiatry. (2018) 23:2133–44. doi: 10.1038/s41380-017-0008-y
82. Plog BA, Dashnaw ML, Hitomi E, Peng W, Liao Y, Lou N, et al. Biomarkers of traumatic injury are transported from brain to blood via the glymphatic system. J Neurosci. (2015) 35:518–26. doi: 10.1523/JNEUROSCI.3742-14.2015
83. Bakulski KM, Halladay A, Hu VW, Mill J, Daniele M, Arbor A, et al. Epigenetic research in neuropsychiatric disorders: the “Tissue issue.” Curr Behav Neurosci Rep. (2016) 3:264–74. doi: 10.1007/s40473-016-0083-4
84. Kochanek PM, Tasker RC, Carney N, Totten AM, Adelson PD, Selden NR, et al. Guidelines for the management of pediatric severe traumatic brain injury, third edition. Pediatr Crit Care Med. (2019) 20:S1–82. doi: 10.1097/PCC.0000000000001735
85. Teasdale GM, Jennett B. Assessment of coma and impaired consciousness: a practical scale. Lancet. (1974) 304:81–4. doi: 10.1016/S0140-6736(74)91639-0
86. Rivara JB, Jaffe KM, Polissar NL, Fay GC, Martin KM, Shurtleff HA, et al. Family functioning and children's academic performance and behavior problems in the year following traumatic brain injury. Arch Phys Med Rehabil. (1994) 75:369–79. doi: 10.1016/0003-9993(94)90157-0
87. Rutter M, Chadwick O, Shaffer D. Head injury. In: Rutter M, editor. Dev Neuropsychiatry. New York, NY: Guilford (1983). p. 83–111.
88. Gioia GA, Isquith PK, Guy SC, Kenworthy L. Behavior rating inventory of executive function. Second Edition (BRIEF-2). Odessa, FL: Psychological Assessment Resources (2015).
89. Gioia GA, Espy KA, Isquith PK. Behavior Rating Inventory of Executive Function-Preschool Version (BRIEF-P). Odessa, FL: Psychological Assessment Resources (2003).
90. Sparrow SS, Cicchetti DV., Saulnier CA. Vineland Adaptive Behavior Scale, Third Edition. Antonio: Psychological Corporation (2016).
91. Kazak AE, Schneider S, Didonato S, Pai ALH. Family psychosocial risk screening guided by the pediatric psychosocial preventative health model (PPPHM) using the psychosocial assessment tool (PAT). Acta Oncol (Madr). (2015) 54:574–80. doi: 10.3109/0284186X.2014.995774
92. Traub F, Boynton-Jarrett R. Modifiable resilience factors to childhood adversity for clinical pediatric practice. Pediatrics. (2017) 139:e20162569. doi: 10.1542/peds.2016-2569
93. Durish CL, Yeates KO, Brooks BL. Psychological resilience as a predictor of persistent post-Concussive symptoms in children with single and multiple concussion. J Int Neuropsychol Soc. (2018) 24:759–68. doi: 10.1017/S1355617718000437
94. Sherer M, Sander AM, Nick TG, Melguizo MS, Tulsky DS, Kisala P, et al. Key dimensions of impairment, self-report, and environmental supports in persons with traumatic brain injury. Rehabil Psychol. (2015) 60:138. doi: 10.1037/rep0000030
95. Riley AW, Forrest CB, Starfield B, Rebok GW, Robertson J a., Green BF. The parent report form of the cHIP-Child edition. Med Care. (2004) 42:210–220. doi: 10.1097/01.mlr.0000114909.33878.ca
96. Starfield B, Riley AW, Green BF, Ensminger ME, Ryan SA, Kelleher K, et al. The adolescent child health and illness profile: a population-based measure of health. Med Care. (1995) 33:553–66. doi: 10.1097/00005650-199505000-00008
97. Weintraub S, Dikmen SS, Heaton RK, Tulsky DS, Zelazo PD, Bauer PJ, et al. Cognition assessment using the nIH toolbox. Neurology. (2013) 80:S54–S64. doi: 10.1212/WNL.0b013e3182872ded
98. Jones MJ, Goodman SJ, Kobor MS. DNA methylation and healthy human aging. Aging Cell. (2015) 14:924–32. doi: 10.1111/acel.12349
99. Thompson EE, Nicodemus-Johnson J, Kim KW, Gern JE, Jackson DJ, Lemanske RF, et al. Global dNA methylation changes spanning puberty are near predicted estrogen-responsive genes and enriched for genes involved in endocrine and immune processes. Clin Epigenetics. (2018) 10:62. doi: 10.1186/s13148-018-0491-2
100. Maschietto M, Bastos LC, Tahira AC, Bastos EP, Euclydes VLV, Brentani A, et al. Sex differences in dNA methylation of the cord blood are related to sex-bias psychiatric diseases. Sci Rep. (2017) 7:44547. doi: 10.1038/srep44547
101. Iughetti L, Casarosa E, Predieri B, Patianna V, Luisi S. Plasma brain-derived neurotrophic factor concentrations in children and adolescents. Neuropeptides. (2011) 45:205–211. doi: 10.1016/j.npep.2011.02.002
102. Dick KJ, Nelson CP, Tsaprouni L, Sandling JK, Aïssi D, Wahl S, et al. DNA methylation and body-mass index: a genome-wide analysis. Lancet. (2014) 383:1990–8. doi: 10.1016/S0140-6736(13)62674-4
103. Joehanes R, Just AC, Marioni RE, Pilling LC, Reynolds LM, Mandaviya PR, et al. Epigenetic signatures of cigarette smoking. Circ Cardiovasc Genet. (2016) 9:436–47. doi: 10.1161/CIRCGENETICS.116.001506
104. Hergenroeder GW, Moore AN, McCoy JP, Samsel L, Ward NH, Clifton GL, et al. Serum iL-6: a candidate biomarker for intracranial pressure elevation following isolated traumatic brain injury. J Neuroinflammation. (2010) 7:19. doi: 10.1186/1742-2094-7-19
105. Petersen AC, Crockett L, Richards M, Boxer A. A self-report measure of pubertal status: reliability, validity, and initial norms. J Youth Adolesc. (1988) 17:117–33. doi: 10.1007/BF01537962
106. Carskadon MA, Acebo C. A self-administered rating scale for pubertal development. J Adolesc Heal. (1993) 14:190–5. doi: 10.1016/1054-139X(93)90004-9
107. Baker SP, O'Neill B, Haddon W, Long WB. The injury severity score: a method for describing patients with multiple injuries and evaluating emergency care. J Trauma. (1974) 14:187–96. doi: 10.1097/00005373-197403000-00001
108. Muthén L., Muthén B. Mplus User's Guide: Seventh Edition. Los Angeles, CA: Muthén & Muthén (2015).
109. Treble-Barna A, Zigler C, Uchani S, Conley Y. Latent class trajectory analysis of cSF brain-Derived neurotrophic factor methylation following subarachnoid hemorrhage in adults. J Neurotrauma. (2019) 36:A1–56. doi: 10.1089/neu.2019.29100.abstracts
110. Hannon E, Lunnon K, Schalkwyk L, Mill J. Interindividual methylomic variation across blood, cortex, and cerebellum: implications for epigenetic studies of neurological and neuropsychiatric phenotypes. Epigenetics. (2015) 10:1024–32. doi: 10.1080/15592294.2015.1100786
111. Irizarry RA, Ladd-Acosta C, Carvalho B, Wu H, Brandenburg SA, Jeddeloh JA, et al. Comprehensive high-throughput arrays for relative methylation (CHARM). Genome Res. (2008) 18:780–90. doi: 10.1101/gr.7301508
112. Du P, Zhang X, Huang CC, Jafari N, Kibbe WA, Hou L, et al. Comparison of beta-value and m-value methods for quantifying methylation levels by microarray analysis. BMC Bioinformatics. (2010) 11:587. doi: 10.1186/1471-2105-11-587
113. Jones BL, Nagin DS, Roeder K. A sAS procedure based on mixture models for estimating developmental trajectories. Sociol Methods Res. (2001) 29:374–393. doi: 10.1177/0049124101029003005
114. Nagin D. Group-Based Modeling of Development. Cambridge, MA: Harvard University Press. (2005) doi: 10.4159/9780674041318
115. Tofighi D, Enders CK. Identifying the correct number of classes in growth mixture models. In: Hancock GK, editor. Advances in Latent Variable Mixture Models. Samuelsen: Information Age Publishing Inc. (2010). p. 317–41.
116. Kass RE, Raftery AE. Bayes factors. J Am Stat Assoc. (1995) 90:773–95. doi: 10.1080/01621459.1995.10476572
117. Frankfurt S, Frazier P, Syed M, Jung KR. Using group-Based trajectory and growth mixture modeling to identify classes of change trajectories. Couns Psychol. (2016) 44:622–60. doi: 10.1177/0011000016658097
118. Niyonkuru C, Wagner AK, Ozawa H, Amin K, Goyal A, Fabio A. Group-based trajectory analysis applications for prognostic biomarker model development in severe tBI: a practical example. J Neurotrauma. (2013) 30:938–45. doi: 10.1089/neu.2012.2578
119. Berger RP, Bazaco MC, Wagner AK, Kochanek PM, Fabio A. Trajectory analysis of serum biomarker concentrations facilitates outcome prediction after pediatric traumatic and hypoxemic brain injury. Dev Neurosci. (2011) 32:396–405. doi: 10.1159/000316803
120. Wagner AK, McCullough EH, Niyonkuru C, Ozawa H, Loucks TL, Dobos JA, et al. Acute serum hormone levels: characterization and prognosis after severe traumatic brain injury. J Neurotrauma. (2011) 28:871–88. doi: 10.1089/neu.2010.1586
121. Fritz MS, MacKinnon DP. Required sample size to detect the mediated effect. Psychol Sci. (2007) 18:233–9. doi: 10.1111/j.1467-9280.2007.01882.x
122. Kurowski BG, Treble-Barna A, Zang H, Zhang N, Yeates KO, Taylor HG, et al. Catechol-O-Methyltransferase genotypes and parenting influence long-term executive functioning after early childhood traumatic brain injury. Arch Phys Med Rehabil. (2015) 96:e2–e3. doi: 10.1016/j.apmr.2015.08.004
123. Treble-Barna A, Pilipenko V, Wade SL, Jegga AG, Yeates KO, Taylor HG, et al. Cumulative influence of inflammatory response genetic variation on long-Term neurobehavioral outcomes after pediatric traumatic Brain injury relative to orthopedic injury: an exploratory polygenic risk score. J Neurotrauma. (2020). doi: 10.1089/neu.2019.6866. [Epub ahead of print].
124. Chen X, Zhang X, Liao W, Wan Q. Effect of physical and social components of enriched environment on astrocytes proliferation in rats after cerebral ischemia/Reperfusion injury. Neurochem Res. (2017) 42:1308–16. doi: 10.1007/s11064-016-2172-x
125. Griesbach GS, Hovda DA, Gomez-Pinilla F. Exercise-induced improvement in cognitive performance after traumatic brain injury in rats is dependent on bDNF activation. Brain Res. (2009) 1288:105–15. doi: 10.1016/j.brainres.2009.06.045
126. Griesbach GS, Gómez-Pinilla F, Hovda DA. Time window for voluntary exercise-induced increases in hippocampal neuroplasticity molecules after traumatic brain injury is severity dependent. J Neurotrauma. (2007) 24:1161–71. doi: 10.1089/neu.2006.0255
127. Kochanek PM, Jackson TC, Ferguson NM, Carlson SW, Simon DW, Brockman EC, et al. Emerging therapies in traumatic brain injury. Semin Neurol. (2015) 35:83–100. doi: 10.1055/s-0035-1544237
128. Gao W-M, Chadha MS, Kline AE, Clark RSB, Kochanek PM, Dixon CE, et al. Immunohistochemical analysis of histone h3 acetylation and methylation–evidence for altered epigenetic signaling following traumatic brain injury in immature rats. Brain Res. (2006) 1070:31–4. doi: 10.1016/j.brainres.2005.11.038
Keywords: traumatic brain injury, precision medicine, childhood adversity, epigenetics, brain-derived neurotrophic factor
Citation: Treble-Barna A, Patronick J, Uchani S, Marousis NC, Zigler CK, Fink EL, Kochanek PM, Conley YP and Yeates KO (2020) Epigenetic Effects on Pediatric Traumatic Brain Injury Recovery (EETR): An Observational, Prospective, Longitudinal Concurrent Cohort Study Protocol. Front. Neurol. 11:460. doi: 10.3389/fneur.2020.00460
Received: 08 December 2019; Accepted: 29 April 2020;
Published: 12 June 2020.
Edited by:
Eric Peter Thelin, Karolinska Institutet (KI), SwedenReviewed by:
Karen Caeyenberghs, Deakin University, AustraliaHibah Omar Awwad, University of Oklahoma Health Sciences Center, United States
Copyright © 2020 Treble-Barna, Patronick, Uchani, Marousis, Zigler, Fink, Kochanek, Conley and Yeates. This is an open-access article distributed under the terms of the Creative Commons Attribution License (CC BY). The use, distribution or reproduction in other forums is permitted, provided the original author(s) and the copyright owner(s) are credited and that the original publication in this journal is cited, in accordance with accepted academic practice. No use, distribution or reproduction is permitted which does not comply with these terms.
*Correspondence: Amery Treble-Barna, YW1lcnkudHJlYmxlLWJhcm5hJiN4MDAwNDA7cGl0dC5lZHU=