- 1School of Medicine, Federal University of Amazonas (UFAM), Manaus, Brazil
- 2Division of Neurosurgery, Hospital das Clinicas, University of São Paulo, São Paulo, Brazil
- 3Department of Anesthesiology, Hospital das Clinicas, University of São Paulo, São Paulo, Brazil
- 4Anhembi Morumbi Univesity, São Paulo, Brazil
- 5Department of Neurosurgery, Wexner Medical Center, Ohio State University, Columbus, OH, United States
- 6Neurosciences Institute, El Bosque University, Bogota, Colombia
- 7NIHR Global Health Research Group on Neurotrauma, University of Cambridge, Cambridge, United Kingdom
Background: In a time when the incidence of severe traumatic brain injury (TBI) is increasing in low- to middle-income countries (LMICs), it is important to understand the behavior of predictive variables in an LMIC's population. There are few previous attempts to generate prediction models for TBI outcomes from local data in LMICs. Our study aim is to design and compare a series of predictive models for mortality on a new cohort in TBI patients in Brazil using Machine Learning.
Methods: A prospective registry was set in São Paulo, Brazil, enrolling all patients with a diagnosis of TBI that require admission to the intensive care unit. We evaluated the following predictors: gender, age, pupil reactivity at admission, Glasgow Coma Scale (GCS), presence of hypoxia and hypotension, computed tomography findings, trauma severity score, and laboratory results.
Results: Overall mortality at 14 days was 22.8%. Models had a high prediction performance, with the best prediction for overall mortality achieved through Naive Bayes (area under the curve = 0.906). The most significant predictors were the GCS at admission and prehospital GCS, age, and pupil reaction. When predicting the length of stay at the intensive care unit, the Conditional Inference Tree model had the best performance (root mean square error = 1.011), with the most important variable across all models being the GCS at scene.
Conclusions: Models for early mortality and hospital length of stay using Machine Learning can achieve high performance when based on registry data even in LMICs. These models have the potential to inform treatment decisions and counsel family members.
Level of evidence: This observational study provides a level IV evidence on prognosis after TBI.
Background
Traumatic brain injury (TBI) is a significant healthcare and economic problem in low- and middle-income countries (LMICs) (1, 2), accounting for a major burden regarding morbidity, mortality, disability, socioeconomic losses, reduced life expectancy, and the quality of life (2, 3). Road traffic accidents account for over 60% of all traumatic brain injuries, followed by falls (30%) and violence (10%), with the most affected age group being between 21 and 30 years old (1, 2). Although being able to predict a patient's prognosis at presentation accurately is a significant step in clinical decision making and the assessment of the quality of care (4), to our knowledge there are few prediction models from LMICs that have used machine learning methods based on data directly captured from registries in these environments.
To date, most attempts to predict outcomes after TBI has relied on the use of manually calculated scores. As one of its primary examples, the Glasgow Coma Scale (GCS) has been widely used to predict outcomes, associating patients with a score of 13–14 with longer post-traumatic amnesia and a higher rate of abnormal brain image findings at 6 months after the initial trauma. This score is not perfect, however, with no significant differences noticed for neuropsychiatric status, psychological distress, the frequency of somatic complaints, and the rate of return to work, among other factors (5). The GCS also has the potential to present its predictive performance enhanced by the inclusion of other variables, as has been demonstrated in the improvement of prediction accuracy of hospital mortality (6) through the integration of variables such as age and brain stem reflexes (7). In another example, when the GCS was combined with the Injury Severity Score, their joint performance significantly improved in comparison with isolated scores or the Abbreviated Injury Score for outcomes measured 12 months after the initial injury (8). Recently, GCS prediction models have been enhanced with pupillary response (9). In summary, prediction models including multiple variables tend to exceed isolated, manually calculated scores (10), opening up an opportunity for the use of prediction models as they can increase predictive performance (11, 12).
About the validation of prediction models in precise patient populations, one size does not fit all. In previous systematic reviews, prediction models demonstrated a wide variation in accuracy across different populations (11–13). Accounting for this variability were factors such as model validation using small samples, poor modeling methodology, and the lack of validation using external populations. Of particular relevance in the context of LMICs, models drawn up with samples from developed countries presented a worse performance when applied in LMICs, likely due to the mismatch about case mix as well as overall healthcare infrastructure (14). Hence, these results call for the specific development of models for use within a particular local population. However, to achieve this, local registry data collection is required, which can be a significant challenge in countries with scarce resources.
In the face of this gap in the literature, the objective of this article is to evaluate the predictive performance of a machine-learning-based model for mortality and length of stay applied to a LMIC cohort of TBI patients. We based this model on a prospective registry of a tertiary hospital from São Paulo, Brazil.
Methods
Our objective was to develop a machine learning predictive model based on a prospective registry with consecutively enrolled TBI patients in Brazil. We described our modeling strategy according to the TRIPOD statement recommendations for the reporting of prognostic models (15).
Ethics
The Institutional Review Board of the University of São Paulo (São Paulo, Brazil) approved of our study (CAAE 46831315.3.0000.0068), and informed consent was offered to all potential participants and subsequently signed before the implementation of any study protocol.
Setting
We collected data from consecutive patients coming to the main trauma hospital of the state of São Paulo, Brazil (Hospital das Clinicas, University of São Paulo Medical School). Participant data were captured between March of 2012 and January of 2015, with the end of follow-up occurring in June of 2015.
Participants
Our registry includes patients with TBI, defined as any patient requiring admission to an intensive care trauma unit as referred by the neurosurgery team. Pre-hospital data were collected through the analysis of the clinical chart of the rescue team. We only included patients aged 14 years old and above and patients depicting intracranial abnormality on initial head computerized tomography (CT) scan. We excluded patients with penetrating TBI, as well as those with a GCS of 15 and not associated intracranial lesions on the CT scan. In our institution, any patient with intracranial abnormalities is eligible to be transferred to ICU, which is subject to the availability of bed. Therapeutic planning followed recommendations provided by the Advanced Trauma Life Support as well as guidelines by the Brain Trauma Foundation whenever possible. A total of 517 participants were part of this analysis.
Outcomes
The primary outcome was death within 14 days. We choose this outcome since previous studies in our institution showed a great number of missing data with long-term outcomes in this population. A recent randomized control trial performed in Latin America (16) used the long-term outcome to evaluate the value of intracranial pressure monitoring (ICP) regarding prognosis. There was a trend of benefit in early mortality in those who received ICP monitoring; however, no differences were found in the long term. Maybe the scarce rehabilitation programs in LMICs may have offset the initial benefit found. As we decided to use a strong outcome, which could also be compared with another predicting model (e.g., CRASH prognostic model), we choose 14 day mortality. Those patients who had been discharged before 14 days were contacted by telephone or mail. Secondary outcomes were hospital mortality (defined as death occurring during the hospital stay), the number of days spent in the ICU, and the number of days spent in the hospital.
Predictors
Predictors were selected based on previously described models from TBI literature (4, 11–13). Specifically, we selected gender, age, level of pupil reactivity at admission, GCS at the scene where the trauma occurred (prehospital GCS), GCS at admission, the motor component score of the GCS, and presence of hypoxia and hypotension. Also included were midline shift bigger than 5 mm, brain herniation detected on CT (defined as effacement of the third ventricle or the basal cisterns), subarachnoid hemorrhage, epidural hemorrhage, subdural hemorrhage, intracerebral hemorrhage, trauma severity, prothrombin time, and partial thromboplastin time. We chose a larger amount of variables in order to find any predictor not found in calculators such as CRASH or IMPACT since our study population was composed only of cases from a LMIC hospital. These variables were all collected at ICU admission.
Data Analysis
We initially performed a graphical exploratory analysis evaluating the frequency, percentage, and near-zero variance for all categorical variables, distribution for numeric variables, and missing values and patterns of all variables (17). Also, a MINE algorithm (18) was run in an exploratory manner to guide bivariate plot inspection. We proceed then with feature engineering including variable transformations and dummy coding for variables with distributions that were not normal at inspection, variable re-categorization removal for near-zero variation, and different imputation algorithms for variables with missing values. We modeled outcomes and predictors in the described format. To train and test our models, we used a 5-fold model validation.
Machine learning classification models for the prediction of categorical variables included regularized least squares and linear regression. Machine learning regression models, i.e., those directed at numeric outcomes, included random forest, neural network, decision tree, boosting, generalized linear model, partial least squares, and multivariate adaptive regression splines. Regression models for the classification of numeric variables included random forest, discriminant analysis, Bayesian methods, neural network, decision tree, boosting, generalized linear model, partial least squares, and multivariate adaptive regression splines. Comparison across models was performed using the area under the curve, sensitivity, specificity, kappa values as well as positive and negative predictive values. Since the length of stay in hospital and ICU did not present a normal distribution, all models were run with log-transformed variables and then subsequently exponentiated so that results could be clinically interpretable.
All calculations were performed using the statistical language R and the packages ggplot2, caret, knitr, vcd, randomForest, MASS, glmnet, mda, pROC, corrplot, and tabplot.
Results
Five hundred seventy patients were admitted to the ICU with diagnosis of TBI. We excluded 26 patients who were victims of gunshot wound, 14 patients who had chronic subdural hematomas, 9 patients with GCS of 15 and no abnormalities on CT scan, 2 patients aged <2 years old, and 2 patients transferred after more than 48 h of trauma. Table 1 reports information on our total study sample as well as stratification by TBI severity. Comparisons were performed using chi-square and t-tests. Most patients were male (85.1%) with a mean age of 41.5 ± 18.1 [standard deviation (SD)] years. The average length of stay was 25.4 (SD 28.9) days, with 13.1 (SD 15.9) days spent in the intensive care unit. We found overall mortality at 14 days of 22.8%, with mortality rates increasing proportionally to severity levels. Higher severity scores were also associated with increased rates of hemorrhage at the epidural, subdural, and subarachnoid levels, as well as with worse GC.
Exploratory Analysis
When evaluating the association between GCS and various outcomes of interest, we found that a worse GCS at admission was significantly associated with an increased risk of mortality at 14 days and in-hospital mortality (p < 0.001). However, there was no significant association between age and length of stay at the hospital and in the intensive care unit.
Model Performance
When evaluating models for mortality prediction, we found that naive Bayes had the best predictive performance (area under the curve = 0.906), followed by Bayesian generalized linear model (area under the curve = 0.881), random forest (area under the curve = 0.880), and penalized discriminant analysis (area under the curve = 0.880) (Figure 1).
Across all top-performing models, the following variables were consistently considered among the most important: prehospital GCS and GCS at admission, age, and Glasgow motor score (Figure 2).
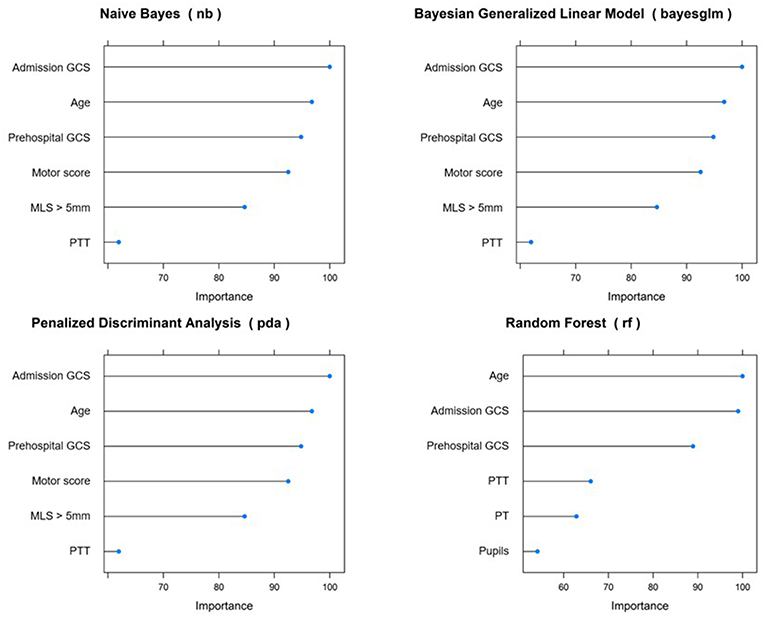
Figure 2. Variable importance across top-performing predictive models for 14 day mortality. GCS, Glasgow Coma Score; MLS, midline shift; PTT, partial thromboplastin time; PT, prothrombin time.
When predicting in-hospital mortality, random forest was the best performing model (area under the curve = 0.838), closely followed by generalized partial least squares (area under the curve = 0.831), stochastic gradient boosting (area under the curve = 0.823), and penalized discriminant analysis (area under the curve = 0.803) (Figure 3).
The most important variables predicting in-hospital mortality across all models were GCS at admission, age, prehospital GCS, and thromboplastin time partial test (Figure 4).
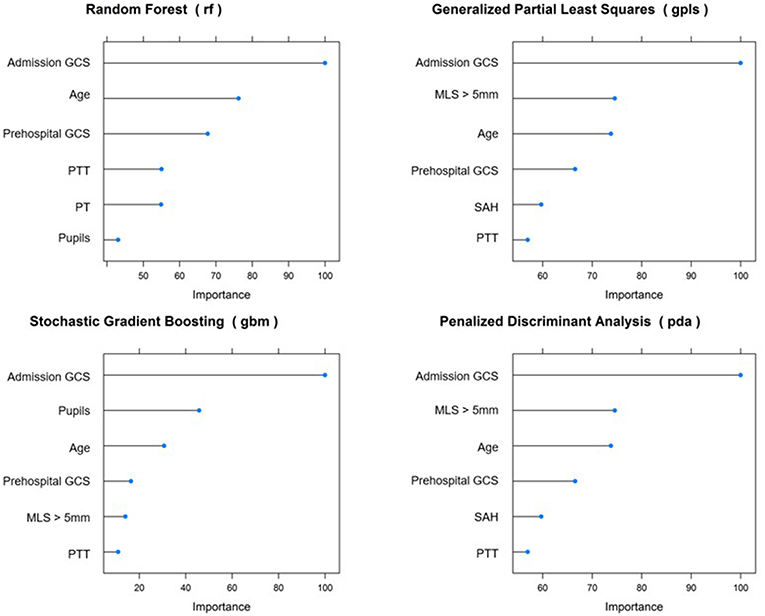
Figure 4. Most important variables predicting in-hospital mortality. GCS, Glasgow Coma Score; MLS, midline shift; PTT, partial thromboplastin time; PT, prothrombin time.
Discussion
The majority of the literature in TBI, including prediction models, comes from developed countries in North America and Europe. However, there are several differences between high-income countries and LMICs that may hinder the external validation of these models including population characteristic, health system, prehospital care, in-hospital care, financial resources, etc. To the best of our knowledge, this is the first study using machine learning to predict mortality and the length of stay after TBI in a LMIC. We found overall mortality at 14 days of 22.8%, with mortality rates increasing proportionally to severity levels. Higher severity scores were also associated with increased rates of epidural, subdural, subarachnoid, and intracerebral hemorrhage levels, as well as with worse GCS scores. Models had a high prediction performance, with the best prediction for overall mortality achieved by naive Bayes (area under the curve = 0.908), with the most significant predictors being GCS at admission, prehospital GCS, age, and pupil reaction. When predicting the length of stay at the intensive care unit, the conditional inference tree model presented the best performance (root mean square error = 1.011), with the most important variable across all models being prehospital GCS. Importantly, our results are equivalent or superior to the best predictive models in the literature reporting on patients in developed countries.
In alignment with our findings, the literature has consistently associated higher mortality rates with lower admission GCS (19, 20). A few studies have also evaluated whether specific sub-components of the GCS could predict mortality, with motor scores equal to or lower than 3 having been associated with higher risk (21). In addition, because the GCS can be measured across the whole care pathway, some authors have sought to investigate at which point the assessment had a stronger association with mortality risk. Previous findings point to the best prediction occurring when combining scores measured at the trauma and admission sites, which is reinforced by our findings (22). Finally, previous publications have frequently predicted GCS themselves by using a range of other clinical characteristics including advanced age and CT findings, which once again points to a possible improvement in their predictive performance if these variables were to be taken into consideration while generating predictive models (23, 24).
Also in consonance with our study, higher mortality has been previously associated with subarachnoid hemorrhage and altered pupil response including bilateral mydriasis, anisocoria, and absence of pupil reflex (19, 24). These findings agree with our current mechanistic understanding of causes leading to death in that subarachnoid hemorrhage may cause vasospasm and subsequent ischemia (25). Specifically, the maximum thickness of the subarachnoid blood has been previously demonstrated to independently predict mortality (26). As expected, previous studies have illustrated that adding a variable indicating sub-arachnoid hemorrhage to predictive models might enhance their predictive accuracy (27).
Concerning the partial thromboplastin time test which was found to be an important predictor of in-hospital mortality, we found that early coagulopathy is associated with a 4-fold increase in mortality among traumatic brain injury patients (28). Also, an increase in partial thromboplastin time of over 1.5 times the standard value was associated with a decrease in median survival times (28), which strongly suggests that this clinical factor should be taken into consideration while evaluating prognosis in LMICs. Other researches have already focused on the relation between partial thromboplastin time test and TBI prognosis. In 2013, Chhabra et al. reinforced the high incidence of coagulopathy following TBI and demonstrated that the presence of coagulopathy is a strong predictor of in-hospital mortality (29). In agreement with these results, a recent article by Yuan et al. revealed that coagulation tests could improve the predictive power of the standard models for in-hospital mortality after TBI (30). The finding of PTT as predictor deserves special attention, especially since the main validated prognostic models, Corticosteroid Randomization After Significant Head Injury (CRASH) and International Mission for Prognosis and Analysis of Clinical Trials in Traumatic Brain Injury (IMPACT), do not consider such variable. It has to be further clarified whether the partial thromboplastin time test can be especially useful in low- and middle-income countries, where investigative tools are scarce. Interestingly, most of the modeling techniques identified the importance of PTT, but did not identify such strong association with prothrombin time (PT) which is somehow hard to explain but should be better analyzed in a future cohort of patients.
Lastly, although the literature on the association between GCS and length of stay in the intensive care unit is scarce, indirect evidence comes from an increase in hyperthermia, which in turn can predict the increased length of stay (31). In contrast with the length of stay in the intensive care unit, there is previous evidence pointing to the severity of traumatic brain injury as a predictor of increases in the overall length of stay (32). Both of these findings corroborate our results.
This paper evaluated several modeling techniques, including logistic regression (the most commonly used in other papers). Even though some studies depict some criticisms regarding their use in medicine, we believe that due to the paucity of studies regarding such methodology, if the investigators can perform several techniques simultaneously (i.e., R packages), this issue should be further investigated (33).
Despite bringing novelty into the literature, our study does have limitations frequently associated with observational designs. First, our diagnoses and outcomes were not validated through observer agreement studies, thus introducing a potential bias. Second, we did not include long-term functional outcomes or self-reported measures of quality of life. In LMICs, the long-term follow-up may be challenging due to difficulties in contacting patients even through mobile phone. Additionally, lack of standardized rehabilitation programs for such patients may contribute for this inadequate follow-up. Due to the inherent features of this LMICs' cohort, collection of variables such as length of coma or duration of post-traumatic amnesia was not possible. Although the endpoint of “14 day mortality” is a short outcome, we do believe it has great importance in patients of LMICs. A couple of the major issues are the pre-hospital care and medical treatment in the acute phase. Therefore, we considered that 14 day mortality should be a strong endpoint to evaluate the treatment in the acute phase indirectly. It may be additionally compared with existing models such as the CRASH trial, which has the same endpoint. Such analysis will be performed in a future study of our group.
Moreover, the self-reported measure constitutes an essential parameter in that they introduce a direct perspective from patients, which is missing when only provider-driven measures are used. This deficiency primarily resulted from the logistical issues related to collecting data in our environment. Additionally, the fact that the sample population includes only patients admitted to ICU may affect generalizability since patients sustaining brain abnormalities could have faced the absence of an ICU bed and had not been transferred. Finally, given that we did not randomly draw out our sample from a larger patient population, its external validity can be questioned. Although future studies should aim at larger and more representative samples, our sample is by no means atypical for its setting, making our conclusions valid for similar populations around the globe. In conclusion, we believe that our model may serve as basis for the development of machine-learning-based algorithms in the prediction of short-term outcomes in LMICs, provided that their population and healthcare system bear a reasonable similarity to ours. Besides the clinical information and assessment of the medical care provided in LMICs, the prediction models may be a useful tool to establish priorities and guide the allocation of limited resources. We also expect that our study might provide the incentive for other centers in LMICs to create similar registries, thus optimizing the prediction of clinical outcomes and improving the quality of care provided to patients with traumatic brain injuries.
Data Availability Statement
The datasets generated for this study are available on request to the corresponding author.
Ethics Statement
The studies involving human participants were reviewed and approved by Cappesq–Ethics Committee for Analysis of Research Projects, University of São Paulo Medical School. The patients/participants provided their written informed consent to participate in this study.
Author Contributions
RA: conception and design, data collection, analysis and interpretation, drafting of the manuscript, reviewing, and final approval of the manuscript. LO and LMi: conception and design, data collection, and reviewing. LMa, AK, and WP: conception and design and reviewing. MN and MS: data collection and reviewing. EB-S-S, AD, and AR: drafting and reviewing of the manuscript. AB-F: reviewing the manuscript. MT: conception and design, drafting, and reviewing of the manuscript.
Funding
This research was commissioned by the National Institute for Health Research (NIHR) Global Health Research Group on Neurotrauma (project 16/137/105) using UK aid from the UK Government. The views expressed in this publication are those of the authors and not necessarily those of the NIHR or the Department of Health and Social Care.
Conflict of Interest
The authors declare that the research was conducted in the absence of any commercial or financial relationships that could be construed as a potential conflict of interest.
References
1. Umerani MS, Abbas A, Sharif S. Traumatic brain injuries: experience from a tertiary care centre in Pakistan. Turk Neurosurg. (2014) 24:19–24. doi: 10.5137/1019-5149.JTN.7080-12.1
2. Gururaj G. Epidemiology of traumatic brain injuries: Indian scenario. Neurol Res. (2002) 24:24–8. doi: 10.1179/016164102101199503
3. Hofman K, Primack A, Keusch G, Hrynkow S. Addressing the growing burden of trauma and injury in low- and middle-income countries. Am J Public Health. (2005) 95:13–7. doi: 10.2105/AJPH.2004.039354
4. Lingsma HF, Roozenbeek B, Steyerberg EW, Murray GD, Maas AIR. Early prognosis in traumatic brain injury: from prophecies to predictions. Lancet Neurol. (2010) 9:543–54. doi: 10.1016/S1474-4422(10)70065-X
5. McCullagh S, Oucherlony D, Protzner A, Blair N, Feinstein A. Prediction of neuropsychiatric outcome following mild trauma brain injury: an examination of the Glasgow Coma Scale. Brain Inj. (2001) 15:489–97. doi: 10.1080/02699050118966
6. Moore L, Lavoie A, Camden S, Le Sage N, Sampalis JS, Bergeron E, et al. Statistical validation of the Glasgow Coma Score. J Trauma. (2006) 60:1238–43. doi: 10.1097/01.ta.0000195593.60245.80
7. Prasad K. The Glasgow Coma Scale: a critical appraisal of its clinimetric properties. J Clin Epidemiol. (1996) 49:755–63. doi: 10.1016/0895-4356(96)00013-3
8. Foreman BP, Caesar RR, Parks J, Madden C, Gentilello LM, Shafi S, et al. Usefulness of the abbreviated injury score and the injury severity score in comparison to the Glasgow Coma Scale in predicting outcome after traumatic brain injury. J Trauma Inj Infect Crit Care. (2007) 62:946–50. doi: 10.1097/01.ta.0000229796.14717.3a
9. Brennan PM, Murray GD, Teasdale GM. Simplifying the use of prognostic information in traumatic brain injury. Part 1: the GCS–Pupils score: an extended index of clinical severity. J Neurosurg. (2018) 128:1612–20. doi: 10.3171/2017.12.JNS172780
10. Honeybul S, Ho KM, Lind CR, Corcoran T, Gillett GR. The retrospective application of a prediction model to patients who have had a decompressive craniectomy for trauma. J Neurotrauma. (2009) 26:2179–83. doi: 10.1089/neu.2009.0989
11. Perel P, Edwards P, Wentz R, Roberts I. Systematic review of prognostic models in traumatic brain injury. BMC Med Inform Decis Mak. (2006) 6:38. doi: 10.1186/1472-6947-6-38
12. Signorini DF, Andrews PJD, Jones PA, Wardlaw JM, Miller JD. Predicting survival using simple clinical variables: a case study in traumatic brain injury. J Neurol Neurosurg Psychiatry. (1999) 66:20–5. doi: 10.1136/jnnp.66.1.20
13. Hukkelhoven CW, Steyerberg EW, Habbema JDF, Farace E, Marmarou A, Murray GD, et al. Predicting outcome after traumatic brain injury: development and validation of a prognostic score based on admission characteristics. J Neurotrauma. (2005) 22:1025–39. doi: 10.1089/neu.2005.22.1025
14. MRC CRASH Trial Collaborators. Predicting outcome after traumatic brain injury: practical prognostic models based on large cohort of international patients. BMJ. (2008) 336:425–9. doi: 10.1136/bmj.39461.643438.25
15. Collins GS, Reitsma JB, Altman DG, Moons KGM. Transparent reporting of a multivariable prediction model for individual prognosis or diagnosis (tripod): the tripod statement. J Clin Epidemiol. (2015) 68:134–43. doi: 10.7326/M14-0698
16. Chesnut R, Temkin N, Carney N, Dikmen S, Rondina C, Videtta W, et al. A trial of intracranial-pressure monitoring in traumatic brain injury. N Eng J Med. (2012) 367:2471–81. doi: 10.1056/NEJMoa1207363
18. Reshef DN, Reshef YA, Finucane HK, Grossman SR, McVean G, Turnbaugh PJ, et al. Detecting novel associations in large data sets. Science. (2011) 334:1518–24. doi: 10.1126/science.1205438
19. Schreiber MA, Aoki N, Scott BG, Beck J. Determinants of mortality in patients with severe blunt head injury. Arch Surg. (2002) 137:285–90. doi: 10.1001/archsurg.137.3.285
20. Jain S, Dharap SB, Gore MA. Early prediction of outcome in very severe closed head injury. Injury. (2008) 39:598–603. doi: 10.1016/j.injury.2007.06.003
21. Rodríguez M. Predicting mortality from head injury: experience of Sancti Spíritus Province, Cuba. MEDICC Rev. (2013) 15:30–3.
22. Davis DP, Serrano JA, Vilke GM, Sise MJ, Kennedy F, Eastman AB, et al. The predictive value of field versus arrival Glasgow Coma Scale Score and TRISS calculations in moderate-to-severe traumatic brain injury. J Trauma Inj Infect Crit Care. (2006) 60:985–90. doi: 10.1097/01.ta.0000205860.96209.1c
23. Hsiao KY, Hsiao CT, Weng HH, Chen KH, Lin LJ, Huang YM. Factors predicting mortality in victims of blunt trauma brain injury in emergency department settings. EMJ. (2008) 25:670–3. doi: 10.1136/emj.2007.053405
24. Martins ET, Linhares MN, Sousa DS, Schroeder HK, Meinerz J, Rigo LA, et al. Mortality in severe traumatic brain injury: a multivariated analysis of 748 Brazilian patients from Florianópolis City. J Trauma Inj Infect Crit Care J. (2009) 67:85–90. doi: 10.1097/TA.0b013e318187acee
25. Armin SS, Colohan ART, Zhang JH. Traumatic subarachnoid hemorrhage: our current understanding and its evolution over the past half century. Neurol Res. (2006) 28:445–52. doi: 10.1179/016164106X115053
26. Tu CJ, Liu JS, Song DG, Zhen G, Luo HM, Liu WG, et al. Maximum thickness of subarachnoid blood is associated with mortality in patients with traumatic subarachnoid haemorrhage. J Int Med Res. (2011) 39:1757–65. doi: 10.1177/147323001103900518
27. Maas AI, Hukkelhoven CW, Marshall LF, Steyerberg EW. Prediction of outcome in traumatic brain injury with computed tomographic characteristics: a comparison between the computed tomographic classification and combinations of computed tomographic predictors. Neurosurgery. (2005) 57:1173–82. doi: 10.1227/01.NEU.0000186013.63046.6B
28. Whittaker B, Christiaans SC, Altice JL, Chen MK, Bartolucci AA, Morgan CJ, et al. Early coagulopathy is an independent predictor of mortality in children after severe trauma. Shock. (2013) 39:421–6. doi: 10.1097/SHK.0b013e31828e08cb
29. Chhabra G, Sharma S, Subramanian A, Agrawal D, Sinha S, Mukhopadhyay AK. Coagulopathy as prognostic marker in acute traumatic brain injury. J Emerg Trauma Shock. (2013) 6:180–5. doi: 10.4103/0974-2700.115332
30. Yuan Q, Yu J, Wu X, Sun Y, Li Z, Du Z, et al. Prognostic value of coagulation tests for in-hospital mortality in patients with traumatic brain injury. Scand J Trauma Resusc Emerg Med. (2018) 26:3. doi: 10.1186/s13049-017-0471-0
31. Natale JE, Joseph JG, Helfaer MA, Shaffner DH. Early hyperthermia after traumatic brain injury in children: risk factors, influence on length of stay, and effect on short-term neurologic status. Crit Care Med. (2000) 28:2608–15. doi: 10.1097/00003246-200007000-00071
32. Lehmkuhl DI, Hall KM, Mann N, Gordon WA. Factors that influence costs and length of stay of persons with traumatic brain injury in acute care and inpatient rehabilitation. J Head Trauma Rehabil. (1993) 8:88–100. doi: 10.1097/00001199-199308020-00010
Keywords: prognostic, traumatic brain injury, machine learning, mortality, LMICs
Citation: Amorim RL, Oliveira LM, Malbouisson LM, Nagumo MM, Simoes M, Miranda L, Bor-Seng-Shu E, Beer-Furlan A, De Andrade AF, Rubiano AM, Teixeira MJ, Kolias AG and Paiva WS (2020) Prediction of Early TBI Mortality Using a Machine Learning Approach in a LMIC Population. Front. Neurol. 10:1366. doi: 10.3389/fneur.2019.01366
Received: 17 June 2019; Accepted: 10 December 2019;
Published: 24 January 2020.
Edited by:
Nicole Osier, University of Texas at Austin, United StatesReviewed by:
Lanfranco Iodice, Federico II University Hospital, ItalyJohn K. Yue, University of California, San Francisco, United States
Copyright © 2020 Amorim, Oliveira, Malbouisson, Nagumo, Simoes, Miranda, Bor-Seng-Shu, Beer-Furlan, De Andrade, Rubiano, Teixeira, Kolias and Paiva. This is an open-access article distributed under the terms of the Creative Commons Attribution License (CC BY). The use, distribution or reproduction in other forums is permitted, provided the original author(s) and the copyright owner(s) are credited and that the original publication in this journal is cited, in accordance with accepted academic practice. No use, distribution or reproduction is permitted which does not comply with these terms.
*Correspondence: Wellingson Silva Paiva, d2VsbGluZ3NvbnBhaXZhJiN4MDAwNDA7eWFob28uY29tLmJy