- Department of Neurology, University Medical Center Hamburg-Eppendorf, Hamburg, Germany
Background: White matter hyperintensities of presumed vascular origin (WMH) are a common finding in elderly people and a growing social malady in the aging western societies. As a manifestation of cerebral small vessel disease, WMH are considered to be a vascular contributor to various sequelae such as cognitive decline, dementia, depression, stroke as well as gait and balance problems. While pathophysiology and therapeutical options remain unclear, large-scale studies have improved the understanding of WMH, particularly by quantitative assessment of WMH. In this review, we aimed to provide an overview of the characteristics, research subjects and segmentation techniques of these studies.
Methods: We performed a systematic review according to the PRISMA statement. One thousand one hundred and ninety-six potentially relevant articles were identified via PubMed search. Six further articles classified as relevant were added manually. After applying a catalog of exclusion criteria, remaining articles were read full-text and the following information was extracted into a standardized form: year of publication, sample size, mean age of subjects in the study, the cohort included, and segmentation details like the definition of WMH, the segmentation method, reference to methods papers as well as validation measurements.
Results: Our search resulted in the inclusion and full-text review of 137 articles. One hundred and thirty-four of them belonged to 37 prospective cohort studies. Median sample size was 1,030 with no increase over the covered years. Eighty studies investigated in the association of WMH and risk factors. Most of them focussed on arterial hypertension, diabetes mellitus type II and Apo E genotype and inflammatory markers. Sixty-three studies analyzed the association of WMH and secondary conditions like cognitive decline, mood disorder and brain atrophy. Studies applied various methods based on manual (3), semi-automated (57), and automated segmentation techniques (75). Only 18% of the articles referred to an explicit definition of WMH.
Discussion: The review yielded a large number of studies engaged in WMH research. A remarkable variety of segmentation techniques was applied, and only a minority referred to a clear definition of WMH. Most addressed topics were risk factors and secondary clinical conditions. In conclusion, WMH research is a vivid field with a need for further standardization regarding definitions and used methods.
Introduction
Cerebrovascular disease represents a major burden on an individual as well as societal level, with growing importance in the aging western societies. Stroke as the most prominent example is the second most frequent cause of death in the world and the most frequent cause of acquired permanent disability (1). Vascular dementia represents another manifestation of cerebrovascular disease and is the second most frequent type of dementia following Alzheimer's disease (2). In Alzheimer's disease, cerebrovascular pathology is also a frequent finding (3). Among other causes, these disease entities are considered to be associated with cerebral small vessel disease (CSVD). CSVD comprises different structural changes observed in post-mortem or in-vivo brain imaging, all of them related to alterations of small brain arteries. These include small subcortical infarcts, lacunes, dilated perivascular spaces, cerebral microbleeds, and particularly white matter hyperintensities of presumed vascular origin (WMH).
According to the Standards for Reporting Vascular changes on nEuroimaging (STRIVE)—an international consensus on the definition of cerebral small vessel disease—WMH are hyperintensities on T2-weighted magnetic resonance images (MRIs), which are located in the white matter and of varying size (4). Affecting preferentially the elderly, WMH are associated with cognitive impairment, mortality, increased risk of stroke and play a role in the development of late-onset depression (5–7). They are further considered to worsen gait (8), balance (9), and urinary function (10). Common cardiovascular risk factors associated with WMH (11), include hypertension (12), smoking (13), and diabetes (14). Nevertheless, the exact etiology and pathogenesis of WMH, as well as their role in neurodegeneration, is not fully understood. Therefore, further research on WMH is necessary to clarify these questions and guide future treatment and preventive interventions.
For epidemiological research, quantitative assessment of WMH is a crucial requirement for adequate analysis of associated risk factors and clinical deficits. Semi-quantitative assessments using visual rating scales (15, 16) carry certain disadvantages such as limited accuracy, high intra- and inter-rater-variation (17), low comparability (18), and inadequate depiction of longitudinal changes (19). Moreover, visual rating scales usually do not reflect precise localization of observed WMH. Although correlating with visual rating scales (20), quantitative measurements based on WMH segmentation offer a more reliable, sensitive, and objective alternative (21), which also enables the anatomical analysis. Technically, WMH segmentation is the process of subdividing image voxels into subgroups based on predefined features such as signal intensity. Figure 1 illustrates representative results of different segmentation techniques for exemplary purposes. Since segmenting brain lesions by hand is a highly demanding process, the vivid research field produced various automated and semi-automated segmentation techniques (24). Nevertheless, there are no standardized approaches to quantitative or semi-quantitative WMH segmentations. Also, inconsistent definitions of WMH (4) and differing standards for the qualitative evaluation and quantitative comparison of the results to a so-called gold standard exist, not to mention the reporting of these. The research community has recognized these problems and addressed them over the last years, with the STRIVE as a major milestone achieved in 2013: in this position paper, experts in the field provided an unification of cerebral small vessel disease definitions including a clear definition of white matter hyperintensities of presumed vascular origin (4).
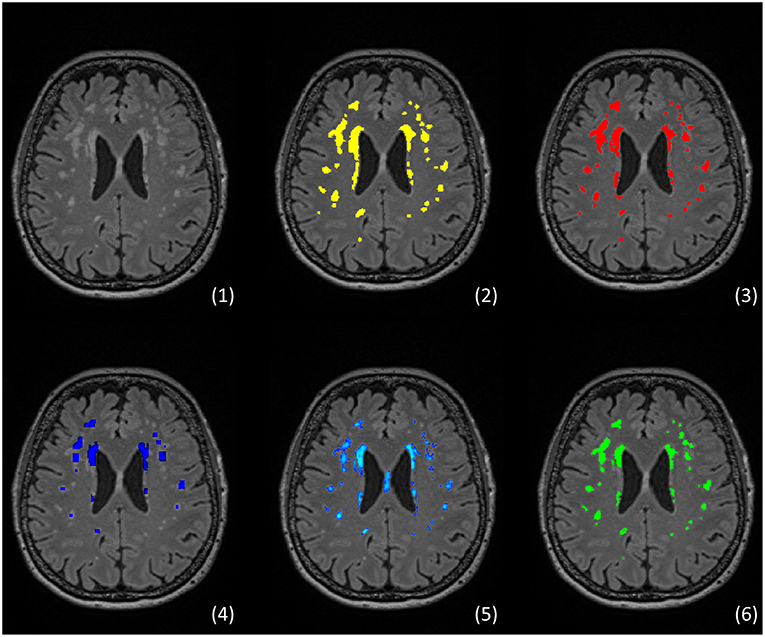
Figure 1. Example of segmentation of white matter hyperintensities (WMH) using different approaches The figure shows an example from an own unpublished dataset: (1) FLAIR showing typical distribution of WMH, (2) manual segmentation rater 1 (MP), (3) manual segmentation rater 2 (CM), (4) automated segmentation via Lesion growth algorithm (LGA) of LST toolbox version 2.0.15 (22), (5) automated segmentation via Lesion prediction algorithm (LPA) also of LST toolbox, (6) automated segmentation via the Brain Intensity AbNormality Classification Algorithm (BIANCA) implemented in FSL (23).
Currently, there is accumulating evidence pointing to a clinical relevance of WMH, substantially driven by large-scale studies. Thus, standardization of methodological approaches for WMH characterization in these studies is of crucial importance. In this systematic review, we provide an overview of large-scale studies assessing WMH quantitatively over the past 14 years. We describe their characteristics, research subjects, approaches on WMH segmentation, and the study-specific and general development of segmentation techniques. Furthermore, we continue the discussion about the heterogeneity issues in this particular field of research. By this, we aim to contribute to the unification work of the field started previously by other research groups.
Methods
We conducted a systematic review according to the Preferred Reporting Items for Systematic Reviews and Meta-Analysis (PRISMA) Statement (25). The review protocol was not registered in advance, the completed PRISMA checklist can be found in the Supplementary Material.
Search Strategy and Study Selection
The methods of study selection, including searched data sources and selection criteria, were determined in advance. Two reviewers (BF, MP) carried out the literature research in December 2018 by searching the online-database Pubmed for eligible records. Search terms and applied filters are presented in the Supplementary Material.
Study selection was performed by both reviewers independently by screening abstracts or if necessary full-text papers for exclusion criteria. Exclusion criteria were specified as follows: (1) sample size <500, (2) a publication date earlier than 01.01.2005, (3) age <18 years, (4) written in another language than English, (5) no WMH segmentation has been performed, (6) review articles, (7) investigation of WMH of non-vascular origin (studies on WMH occurring in inflammatory or neurodegenerative conditions like multiple sclerosis, lupus, Sneddon syndrome, Huntington-like diseases, neurofibromatosis, leukodystrophies, cerebral autosomal dominant arteriopathy with subcortical infarcts and leukoencephalopathy, Fabry disease, sickle cell disease, progressive multifocal leukoencephalopathy, cerebral amyloid angiopathy, posterior leukoencephalopathy syndrome). Studies were included if no exclusion criteria were met.
Data Extraction and Analysis
Data extraction was conducted independently by both reviewers reading the full-text articles. Resulting data were cross-checked afterwards. Extracted information included the name of the population study the articles belong to, year of publication, sample size, mean age of subjects in the study, the cohort included, and segmentation details like the definition of WMH, the segmentation method, reference to methods papers as well as validation measurements. Additionally, referenced methods papers were surveyed for further details on segmentation methods. All descriptive results are given by the mean ± the standard error of the mean. Data that was not available is reported as missing as long as there was no possibility to compute it.
In accordance with previous work in this field, the methods underlying the image segmentation were categorized into manual, semi-automated, and automated (24). A method was considered “manual” if the researcher annotates all lesion voxels himself; “semi-automated,” if the researcher intervenes in certain situations and “automated,” if there is no necessity of human intervention in the computing process. The latter was again classified in supervised and unsupervised depending on whether or not the classification algorithm requires a previously produced reference segmentation dataset, defining the affiliation of voxels to a particular group, e.g., WMH or non-WMH.
Furthermore, papers were characterized by the type of the underlying research question related to WMH, i.e., whether they studied the association of risk factors and WMH, the influence of WMH on a certain pathology, both directions of causation, or neither of them. All research subjects (e.g., IL-6 or CRP) were extracted and assigned to subcategories defined by umbrella terms (e.g., Inflammatory markers). Since age and sex are regularly control variables, they are not mentioned as distinct research subjects.
Results
Search Results
A flowchart summarizing the search and selection process is provided in Figure 2. Applying the aforementioned search terms and filters, the PubMed search yielded 1,196 potentially relevant records. We ruled out 1,065 of them as they met the exclusion criteria. Six further articles classified as relevant were added manually. A total of 137 articles fitting the criteria remained and were included in this systematic review. An overview of the six studies with most included articles is also part of the results section, encompassing study characteristics and their segmentation approach.
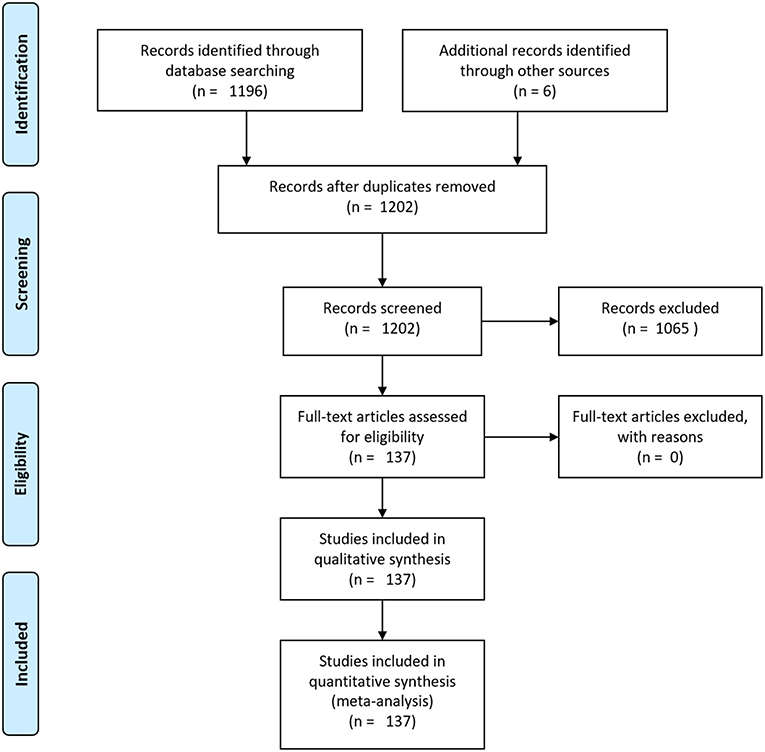
Figure 2. Flowchart of the search and selection process: the Pubmed research yielded 1,196 articles at baseline. No other sources for article identification were used. After application of exclusion criteria, 137 articles remained.
Study Characteristics
The main characteristics of the studies incorporated in this review are shown in Table 1. 137 articles were included, whereas 134 belonged to 37 large-scale prospective cohort studies Box 1 delineates the 5 cohort studies that contributed the most articles to this review. The median sample size was 1,030, ranging from 501 to 9,361. Mean study sample size did not increase over the 14 years investigated (Figure 3). The mean age of subjects in the studies ranged from 46 to 83 years with a total mean of 67 ± 0.8 years. Regarding sample characteristics, 88 of the 137 studies described investigations in a standard population, while 32 included patients with a specific pathology. Seventeen studies compared their pathological cohort with a healthy control group. Concerning the underlying research question, 80 studies analyzed the relationship of risk factors and WMH, which could be categorized into 50 different thematic groups (Table 2). Sixty-four studies examined the link of WMH to diseases and vice versa, covering 25 different thematic groups (Table 3). Two papers did not fit this way of categorization. Their research subjects were “White matter hyperintensities and normal-appearing white matter integrity in the aging brain” (121) and “Incidental Findings on MRI” (122). Two studies, the Leukoaraiosis And DISability Study (LADIS) and the Genetics of Microangiopathic Brain Injury (GMBI) study, were originally established especially for research in WMH and their associations, not for other or more general topics.
Box 1. The Big 5: Cohort studies with the most contributing articles in this work.
SMART-MR
With 22 articles the Second Manifestations of ARTerial disease—Magnetic Resonance Study (SMART-MR) made up the biggest proportion of all included studies. Localized in the Netherlands, SMART-MR had initially been designed to investigate the brain changes on MRI in patients with symptomatic atherosclerotic disease, namely, manifest coronary artery disease, cerebrovascular disease, peripheral artery disease, and abdominal aortic aneurysm. Recruitment took place from May 2001 until December 2005 and resulted in a baseline sample size of 1,309 subjects (49, 50).
3C
Established in the three French cities Bordeaux, Dijon, and Montpellier, the objective of the 3C-study was the assessment of risk of dementia and cognitive impairment attributable to vascular factors. 9294 older adults form the original sample size, recruited from March 1999 to March 2001 (51).
Framingham Offspring Cohort
The Framingham Offspring Cohort contains the offspring of participants from the original Framingham Heart Study. Founded in requirement of a young study sample, the enrolment phase in 1971 supplied an initial study sample of 5,124. The study's purpose is described as the identification of common factors contributing to cardiovascular disease (52, 53).
WHICAP
The Washington/Hamilton Heights-Inwood Columbia Aging Project, located in New York, investigates in Alzheimer's Dementia and Aging in a cohort of multiple ethnicities. The original cohorts size counts 3,452 members (54).
Rotterdam Study
Situated in the Netherlands, the enrolment of the Rotterdam study started in 1990 with the baseline sample size of 7,983 participants. Having a broader approach, the study covers multiple diseases of elderly people in its investigations, i.e., cardiovascular, neurological, ophthalmological, endocrinological, and psychiatric diseases (55).
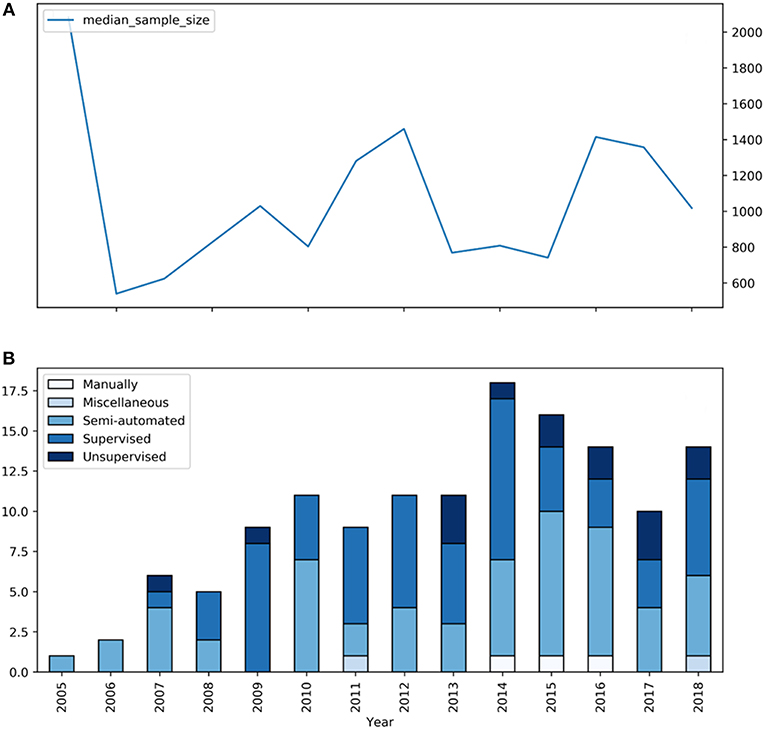
Figure 3. Segmentation types and mean sample size of studies on WMH between 2005 and 2018. (A) The blue graph represents the median sample size of the according studies. (B) The blue bars represent the number of large-scale studies for each year included in our review with the specific segmentation type.
Segmentation
Definition of White Matter Hyperintensities
Only 24 (17.5%) articles contained an explicit definition of WMH. The remaining studies either gave an implicit explanation through their segmentation method or had no specific definition of WMH. Of the studies included in our review, 72 were published since 2014, i.e., after publication of the STRIVE paper. Of these, 15 defined WMH explicitly, 10 of them according to STRIVE. Forty-seven studies did not refer to any explicit definition of WMH at all.
Segmentation Types and Segmentation Techniques
The largest proportion of studies applied automated segmentation techniques: supervised and unsupervised segmentation were used in 60 and 15 articles, respectively. Fifty-seven articles described a semi-automated segmentation technique, while only 3 papers relied on manual segmentation and 2 papers described a miscellaneous approach. Studies using fully automated methods had a significantly higher sample size (p = 0.002; Student's t-test) compared to semi-automated methods (mean 1017.0 vs. 1650.8). Figure 3 shows the distribution of the segmentation types over the years. The peak of published articles on WMH was in 2014. We identified 17 different segmentation techniques used in the studies included in our review (Table 1). Box 2 delivers an introductory explanation for the 5 most employed techniques.
Box 2. Top 5 most used methods for WMH-segmentation in large-scale studies.
Intensity thresholding—DeCarli et al. (32, 35)
The semi-automated method is based on the work of DeCarli et al. Taking the dataset with unclassified voxels, the examiner models a gaussian curve based on the voxel intensity values. Afterwards a threshold value of 3.5 standard deviations above the mean is set. Every voxel with an intensity value higher than the threshold value is defined as a white matter hyperintensity voxel.
Region Growing—Brickman et al. (47)
Similar to the approach by DeCarli, the approach by Brickman and colleagues starts with an intensity thresholding step (2.5 standard deviations) to determine seed voxels for each hemisphere. The seeds are the origin of region growing processes. Every seed voxel intensity value serves as calculation base of an interval (±5%). The algorithm determines class membership of every adjacent voxel by looking whether its intensity value falls into that interval. The algorithm moves further by considering every new WMH defined voxel as a seed with its own interval.
k-Nearest Neighbors—Anbeek et al. (43, 44) and de Boer et al. (40)
Utilized by the Rotterdam study as well as SMART-MR, k-NN is a supervised machine learning algorithm that aims to classify objects based on multiple features, e.g., voxel intensities in T1-w, IR, PD, T2-w, and FLAIR as well as spatial information. For every preclassified voxel a certain location in a multi-dimensional feature space is calculated. Subsequently, a probability is allocated to every voxel of unknown classification based on the labels of its k nearest neighbors in the feature space.
Naïve Bayesian Classifier—Maillard et al. (27)
The Naïve Bayesian Classifier is a machine learning algorithm utilizing Bayesian statistics. As a learning step a preclassified dataset is consigned. The algorithm takes this dataset and calculates its baseline probabilities: simple probabilities like the likelihood of choosing a WMH through a random pick [P(WMH)] and conditional probabilities like the likelihood of a choosing a WMH through a random pick under the assumption of certain features [P(WMH|Features)]. Next, unclassified voxels are handed over to the algorithm. Based on the baseline probabilities the algorithm delivers probability values of group membership given certain features for every voxel. Finally, those membership values are compared and the voxel is assigned to the group with the highest (24).
Artificial Neural networks —Zijdenbos et al. (29)
Artificial neural networks are algorithms inspired by the architecture of biological neural networks, containing neurons and in-between connections. The network established by Zijdenbos and colleagues consists of three layers: an input layer counting consisting of six nodes/neurons where the spatial and intensity information is handed over to the algorithm, a hidden layer with 10 nodes that processes information the input layer delivers and an output layer with two nodes determining the classification of non-WMH and WMH.
Validation Methods
Methodological validation was done by application of accuracy and reproducibility measurements. Of 60 articles with semi-automated or manual segmentation techniques, 18 (30.0%) validated their results with reproducibility metrics, namely the intraclass-correlation coefficient and intra-rater repeatability. Of 132 articles using semi-automated and automated segmentation techniques, 112 (84.8%) reported accuracy metrics like Dice similarity index, intraclass-correlation coefficient, mean absolute error, Pearson's correlation, Cronbach's alpha, Spearman's correlation coefficient, ANOVA, and ANCOVA to validate their results. The gold standard the segmentation techniques were tested against was manual segmentation in 84 studies, while 16 and 13 tested against visual rating scales and semi-automated techniques, respectively.
Discussion
In this systematic review, we identified 137 papers from large-scale studies applying a quantitative analysis of WMH over the past 14 years. With 134 of these being part of a longitudinal prospective cohort study, this indicates to the relevance of these studies in this particular field of research. The large number of studies included in this review reflects the current scientific relevance of WMH in cerebrovascular research. The sample size of these studies ranged from 501 to more than 9,000, which demonstrates the feasibility of WMH segmentation in large samples resulting from the scalability of largely automated image analysis techniques. However, although the past years have brought ongoing improvements in automated image analysis techniques, we did not observe a clear increase of sample size over time. This may either reflect the typical delay until new analysis methods are implemented in large epidemiological studies, which usually are running over a long period. This may also be explained by other factors limiting sample size in large-scale studies beyond factors related to image analysis, e.g., recruitment, or limited capacity of study centers for clinical or imaging studies. Mean age of study subjects across all studies was 67 years, which is likely due to the fact of cerebrovascular diseases being aggregated primarily in the elderly.
The research questions addressed in the studies included in our review could be divided into two groups: the association of risk factors with WMH and supposed clinical or other consequences of WMH. The five most frequently investigated risk factors studied with regards to their association with WMH were hypertension, common risk factors, diabetes, ApoE genotype and inflammatory markers. The majority represents risk factors or markers of atherosclerosis (123).
With regard to clinical manifestations of WMH, there were two areas of interest in the focus of the reviewed studies: a large number of studies looked at WMH in the context of cognitive decline, mild cognitive impairment, or brain volumetric changes and brain atrophy, which are considered as biomarkers of neurodegeneration. This research focus appears obvious, as cerebral small vessel disease is a known risk factor for vascular cognitive impairment and vascular dementia (3). Depressive symptoms were the second clinical focus, as well-thematized in multiple studies. This is in line with the vascular depression hypothesis which proposes an association between the disruption of frontostriatal pathways by WMH and late-life depression (124, 125).
The lack of studies addressing e.g., the association of WMH and ischemic stroke and intracerebral hemorrhage (37, 126) might represent a bias in our search criteria.
Our review focused on the methods utilized for WMH characterization. To some parts, the heterogeneity and lack of standardization seem not only to be a problem of imaging analysis but also of the definition and nomenclature of findings related to cerebral small vessel disease. In an analysis of 1,144 studies dealing with WMH research, 275 used a variant term to “white matter hyperintensity” in their titles or abstracts (4). Efforts to overcome this lack of consensus on terminology and definition of white matter hyperintensities led to publishing the STRIVE consensus criteria in 2013, defining standards for research into cerebral small vessel disease (4). We also wanted to see, whether this initiative and publication of research standards had an impact on scientific studies of WMH in large cohorts. Still, a lot of unifying potential remains here, harboring the problem of arbitrary WMH segmentation and contributing another aspect to the discussion. These numbers suggest that there is still much room for the unification of scientific standards in this research area. In line with this, a recent contribution to the discussion suggested that the descriptive nature of most definitions of white matter hyperintensities is accountable for low-quality segmentation (127). The authors propose a statistical definition as a solution due to its better measurability and provide competitive results with it.
Virtually all studies relied on either semi-automated or fully-automated techniques for WMH segmentation. This finding reflects the trend toward segmentation automation resulting from the acknowledgment of limitations of manual segmentation: it is laborious, thus expensive; is prone to errors; subjective and shows high intra-rater and inter-rater variability (36). Since semi-automated segmentation techniques succumb automated ones regarding human intervention while showing similar segmentation quality, a further trend from semi-automated segmentation methods to fully automated techniques was assumable. Although automated segmentation techniques constituted the largest proportion over the past 14 years from observation of the time course of our data a clear trend toward automated segmentation was not derivable. The significantly higher sample size of studies using automated methods compared to studies using semi-automated methods can be explained by the fact that with higher sample size approaches requiring interaction with a human observer become less feasible.
One striking result of our review is the manifoldness of segmentation techniques used. Almost every cohort study identified had its own segmentation approach. Our review was not designed to answer the question, whether any of the segmentation methods is superior for WMH segmentation. Due to the inherent complexity of the segmentation task, the research field's demand for one proper automated segmentation technique remains unresolved. However, the diversity of segmentation approaches used in large-scale studies is remarkable, which in turn reflect the total lack of any consensus or agreed methodological standard for WMH segmentation.
The existence of a large variety of segmentation techniques is not inherently harmful to the field of research, as it may also be interpreted as a reflection of its vividness. However, the multiplicity of methods used for segmentation and quantification of WMH represents a scientific problem, because it leads to potential incoherence and incomparability between studies. Crucial results such as the overall WMH extent may differ in significant ways depending on the methods used for WMH segmentation.
As a relevant example of how to address cross-study heterogeneity, the NeuroCHARGE Consortium (70) used results of 7 different large-scale prospective cohort studies for a genome-wide association study (GWAS). Before conducting their analysis, they assessed the results for comparability, encompassing WMH segmentation and visual rating scale data, by examining their quality individually via comparison with a reference standard. In addition, utilized visual scoring and volumetric methods were performed on standard image data sets to test agreement.
Automated segmentation was primarily based on machine learning algorithms: for instance, k-nearest neighbors, naive Bayesian classifiers, artificial neural networks and support vector machines were successfully employed to serve the problem of quantitative WMH delineation. Since deep learning, namely convolutional neural networks (CNN), proved themselves for computer vision tasks they are also a hot contender in the WMH segmentation problem. First studies and the WMH segmentation challenge at MICCAI 2017 (http://wmh.isi.uu.nl/) delivered promising results (128–130).
In the publications analyzed in our review, some validated their segmentation results against a gold-standard—usually manual segmentation. This “gold standard,” however, has a lot of inherent limitations, resulting in a significant degree of subjectivity in the validation process. This, again, contributes to incomparability between different methods due to the fact they have been validated on hardly comparable gold standards. Moreover, the methods used for validation, also show some heterogeneity. Many studies use different parameters than the most common metrics like the Dice similarity index and thereby contribute to the overall heterogeneity and lead to aggravated comparison. Again, standardization might provide a solution. The study field could consent, just in the manner of the STRIVE, to specific parameters for validation measures including guidelines of subset selection for specific segmentation tasks (131).
Regardless of the already discussed problems, there are further contributors to variation in WMH quantification. In the end, the quality of the segmentation process depends strongly on the quality of the underlying MRI-images. Especially clinical scans are often very heterogeneous in terms of available MRI-sequences, manufacturer, field strength, signal-to-noise ratio, additional pathologies visible in the scan like stroke lesions or tumors, overall quality assurance protocols and sequence parameters like voxel dimensions, slice gaps, contrast and automated distortion correction. Therefore, the application of the discussed algorithms in the clinical routine might be only possible to a limited extent.
In conclusion, the vast number of large-scale studies reporting the results of segmentation and quantification of WHM reflects the fact that cerebral small vessel disease is a research topic of great interest, especially within the context of epidemiological studies or large patient cohorts. Both, risk factors associated with the presence and extent of WMH and possible behavioral or clinical sequelae are in the focus of research. Approaches to WMH segmentation used in these studies with large samples rely on semi-automated or fully automated algorithms. A multiplicity of methods is used, and clear definitions of WMH are only provided in a minority of studies, which limits comparability and reproducibility of results. New technical developments in segmentation methods may further improve automated lesion segmentation in the near future. In addition to technical advancements, there is a clear need for creating and adhering to reporting guidelines covering both definition of WMH and description of segmentation approach.
Data Availability
The datasets generated for this study are available on request to the corresponding author.
Author Contributions
BF, MP, and GT contributed to the conception and design of the review and to the writing of the manuscript. BF and MP performed the PubMed search and extracting of relevant studies. All authors contributed to the analysis of the results, to manuscript revision, read and approved the submitted version. GT supervised the project.
Funding
This work has been supported by DFG, SFB936/project C2. The funding sources were not involved in the organization and report of this work.
Conflict of Interest Statement
The authors declare that the research was conducted in the absence of any commercial or financial relationships that could be construed as a potential conflict of interest.
Supplementary Material
The Supplementary Material for this article can be found online at: https://www.frontiersin.org/articles/10.3389/fneur.2019.00238/full#supplementary-material
References
1. Feigin VL, Norrving B, Mensah GA. Global burden of stroke. Circ Res. (2017) 120:439–48. doi: 10.1161/CIRCRESAHA.116.308413
2. Fitzpatrick AL, Kuller LH, Ives DG, Lopez OL, Jagust W, Breitner JCS, et al. Incidence and prevalence of dementia in the cardiovascular health study. J Am Geriatr Soc. (2004) 52:195–204. doi: 10.1111/j.1532-5415.2004.52058.x
3. Gorelick PB, Counts SE, Nyenhuis D. Vascular cognitive impairment and dementia. Biochim Biophys Acta Mol Basis Dis. (2016) 1862:860–8. doi: 10.1016/j.bbadis.2015.12.015
4. Wardlaw JM, Smith EE, Biessels GJ, Cordonnier C, Fazekas F, Frayne R, et al. Neuroimaging standards for research into small vessel disease and its contribution to ageing and neurodegeneration. Lancet Neurol. (2013) 12:822–38. doi: 10.1016/S1474-4422(13)70124-8
5. Debette S, Markus HS. The clinical importance of white matter hyperintensities on brain magnetic resonance imaging: systematic review and meta-analysis. BMJ. (2010) 341:1–9. doi: 10.1136/bmj.c3666
6. Herrmann LL, Le Masurier M, Ebmeier KP. White matter hyperintensities in late life depression: a systematic review. J Neurol Neurosurg Psychiatry. (2008) 79:619–24. doi: 10.1136/jnnp.2007.124651
7. Murray A, McNeil C, Salarirad S, Deary I, Phillips L, Whalley L, et al. Brain hyperintensity location determines outcome in the triad of impaired cognition, physical health and depressive symptoms: a cohort study in late life. Arch Gerontol Geriatr. (2016) 63:49–54. doi: 10.1016/J.ARCHGER.2015.10.004
8. van der Holst HM, Tuladhar AM, Zerbi V, van Uden IWM, de Laat KF, van Leijsen EMC, et al. White matter changes and gait decline in cerebral small vessel disease. NeuroImage Clin. (2018) 17:731–8. doi: 10.1016/j.nicl.2017.12.007
9. Baezner H, Blahak C, Poggesi A, Pantoni L, Inzitari D, Chabriat H, et al. Association of gait and balance disorders with age-related white matter changes: the LADIS study. Neurology. (2008) 70:935–42. doi: 10.1212/01.wnl.0000305959.46197.e6
10. Poggesi A, Pracucci G, Chabriat H, Erkinjuntti T, Fazekas F, Verdelho A, et al. Urinary Complaints in nondisabled elderly people with age-related white matter changes: the leukoaraiosis and disability (LADIS) Study. J Am Geriatr Soc. (2008) 56:1638–43. doi: 10.1111/j.1532-5415.2008.01832.x
11. Longstreth WT, Manolio TA, Arnold A, Burke GL, Bryan N, Jungreis CA, et al. Clinical correlates of white matter findings on cranial magnetic resonance imaging of 3301 elderly people. Stroke. (1996) 27:1274–82. doi: 10.1161/01.STR.27.8.1274
12. Maillard P, Seshadri S, Beiser A, Himali JJ, Au R, Fletcher E, et al. Effects of systolic blood pressure on white-matter integrity in young adults in the Framingham Heart Study: a cross-sectional study. Lancet Neurol. (2012) 11:1039–47. doi: 10.1016/S1474-4422(12)70241-7
13. Gons RAR, van Norden AGW, de Laat KF, van Oudheusden LJB, van Uden IWM, Zwiers MP, et al. Cigarette smoking is associated with reduced microstructural integrity of cerebral white matter. Brain. (2011) 134:2116–24. doi: 10.1093/brain/awr145
14. Schneider ALC, Selvin E, Sharrett AR, Griswold M, Coresh J, Jack CR, et al. Diabetes, prediabetes, and brain volumes and subclinical cerebrovascular disease on MRI: the atherosclerosis risk in communities neurocognitive study (ARIC-NCS). Diab Care. (2017) 40:1514–21. doi: 10.2337/dc17-1185
15. Fazekas F, Chawluk J, Alavi A, Hurtig H, Zimmerman R. MR signal abnormalities at 1.5 T in Alzheimer's dementia and normal aging. Am. J. Roentgenol. (1987) 149:351–6. doi: 10.2214/ajr.149.2.351
16. Scheltens P, Barkhof F, Leys D, Pruvo J, Nauta JJP, Vermersch P, et al. A semiquantative rating scale for the assessment of signal hyperintensities on magnetic resonance imaging. J Neurol Sci. (1993) 114:7–12. doi: 10.1016/0022-510X(93)90041-V
17. Wardlaw JM, Ferguson KJ, Graham C. White matter hyperintensities and rating scales? observer reliability varies with lesion load. J. Neurol. (2004) 251:584–90. doi: 10.1007/s00415-004-0371-x
18. van Straaten ECW, Fazekas F, Rostrup E, Scheltens P, Schmidt R, Pantoni L, et al. Impact of white matter hyperintensities scoring method on correlations with clinical data. Stroke. (2006) 37:836–40. doi: 10.1161/01.STR.0000202585.26325.74
19. Prins ND, van Straaten ECW, van Dijk EJ, Simoni M, van Schijndel RA, Vrooman HA, et al. Measuring progression of cerebral white matter lesions on MRI: visual rating and volumetrics. Neurology. (2004) 62:1533–9. doi: 10.1212/01.WNL.0000123264.40498.B6
20. Valdés Hernández M, del C, Morris Z, Dickie DA, Royle NA, Muñoz Maniega S, et al. Close correlation between quantitative and qualitative assessments of white matter lesions. Neuroepidemiology. (2013) 40:13–22. doi: 10.1159/000341859
21. van den Heuvel DMJ, ten Dam VH, de Craen AJM, Admiraal-Behloul F, van Es ACGM, Palm WM, Spilt A, et al. Measuring longitudinal white matter changes: comparison of a visual rating scale with a volumetric measurement. Am J Neuroradiol. (2006) 27:875–78. doi: 10.1136/jnnp.2005.070193
22. Schmidt P, Gaser C, Arsic M, Buck D, Förschler A, Berthele A, et al. An automated tool for detection of FLAIR-hyperintense white-matter lesions in multiple sclerosis. Neuroimage. (2012) 59:3774–83. doi: 10.1016/j.neuroimage.2011.11.032
23. Griffanti L, Zamboni G, Khan A, Li L, Bonifacio G, Sundaresan V, et al. BIANCA (Brain Intensity AbNormality Classification Algorithm): a new tool for automated segmentation of white matter hyperintensities. Neuroimage. (2016) 141:191–205. doi: 10.1016/j.neuroimage.2016.07.018
24. Caligiuri ME, Perrotta P, Augimeri A, Rocca F, Quattrone A, Cherubini A. Automatic detection of white matter hyperintensities in healthy aging and pathology using magnetic resonance imaging: a review. Neuroinformatics. (2015) 13:261–76. doi: 10.1007/s12021-015-9260-y
25. Moher D, Liberati A, Tetzlaff J, Altman DG. preferred reporting items for systematic reviews and meta-analyses: the PRISMA statement. PLoS Med. (2009) 6:e1000097. doi: 10.1371/journal.pmed.1000097
26. Gurol ME, Irizarry MC, Smith EE, Raju S, Diaz-Arrastia R, Bottiglieri T, et al. Plasma -amyloid and white matter lesions in AD, MCI, and cerebral amyloid angiopathy. Neurology. (2006) 66:23–9. doi: 10.1212/01.wnl.0000191403.95453.6a
27. Maillard P, Delcroix N, Crivello F, Dufouil C, Gicquel S, Joliot M, et al. An automated procedure for the assessment of white matter hyperintensities by multispectral (T1, T2, PD) MRI and an evaluation of its between-centre reproducibility based on two large community databases. Neuroradiology. (2008) 50:31–42. doi: 10.1007/s00234-007-0312-3
28. Schwarz C, Fletcher E, DeCarli C, Carmichael O. Fully-automated white matter hyperintensity detection with anatomical prior knowledge and without FLAIR. Inf Process Med Imag. (2009) 21:239–51.
29. Zijdenbos AP, Forghani R, Evans AC. Automatic “pipeline” analysis of 3-D MRI data for clinical trials: application to multiple sclerosis. IEEE Trans Med Imag. (2002) 21:1280–91. doi: 10.1109/TMI.2002.806283
30. Jack CR, O'Brien PC, Rettman DW, Shiung MM, Xu Y, Muthupillai R, et al. FLAIR histogram segmentation for measurement of leukoaraiosis volume. J Magn Reson Imag. (2001) 14:668–76. doi: 10.1002/jmri.10011
31. Beare R, Srikanth V, Chen J, Phan TG, Stapleton J, Lipshut R, et al. Development and validation of morphological segmentation of age-related cerebral white matter hyperintensities. Neuroimage. (2009) 47:199–203. doi: 10.1016/j.neuroimage.2009.03.055
32. DeCarli C, Massaro J, Harvey D, Hald J, Tullberg M, Au R, et al. Measures of brain morphology and infarction in the framingham heart study: establishing what is normal. Neurobiol Aging. (2005) 26:491–510. doi: 10.1016/j.neurobiolaging.2004.05.004
33. DeCarli C, Miller BL, Swan GE, Reed T, Wolf PA, Garner J, et al. Predictors of brain morphology for the men of the NHLBI twin study. Stroke. (1999) 30:529–36. doi: 10.1161/01.STR.30.3.529
34. Valdés Hernández M, del C, Ferguson KJ, Chappell FM, Wardlaw JM. New multispectral MRI data fusion technique for white matter lesion segmentation: method and comparison with thresholding in FLAIR images. Eur Radiol. (2010) 20:1684–91. doi: 10.1007/s00330-010-1718-6
35. Raz L, Jayachandran M, Tosakulwong N, Lesnick TG, Wille SM, Murphy MC, et al. Thrombogenic microvesicles and white matter hyperintensities in postmenopausal women. Neurology. (2013) 80:911–8. doi: 10.1212/WNL.0b013e3182840c9f
36. Grimaud J, Lai M, Thorpe J, Adeleine P, Wang L, Barker GJ, et al. Quantification of MRI lesion load in multiple sclerosis: a comparison of three computer-assisted techniques. Magn Reson Imag. (1996) 14:495–505.
37. Rost NS, Rahman RM, Biffi A, Smith EE, Kanakis A, Fitzpatrick K, et al. White matter hyperintensity volume is increased in small vessel stroke subtypes. Neurology. (2010) 75:1670–7. doi: 10.1212/WNL.0b013e3181fc279a
38. Ramirez J, Gibson E, Quddus A, Lobaugh NJ, Feinstein A, Levine B, et al. Lesion Explorer: A comprehensive segmentation and parcellation package to obtain regional volumetrics for subcortical hyperintensities and intracranial tissue. Neuroimage. (2011) 54:963–73. doi: 10.1016/J.NEUROIMAGE.2010.09.013
39. van der Flier WM, Middelkoop HAM, Weverling-Rijnsburger AWE, Admiraal-Behloul F, Spilt A, Bollen ELEM, et al. Interaction of medial temporal lobe atrophy and white matter hyperintensities in AD. Neurology. (2004) 62:1862–4. doi: 10.1212/01.WNL.0000125337.65553.8A
40. de Boer R, Vrooman HA, van der Lijn F, Vernooij MW, Ikram MA, van der Lugt A, et al. White matter lesion extension to automatic brain tissue segmentation on MRI. Neuroimage. (2009) 45:1151–61. doi: 10.1016/j.neuroimage.2009.01.011
41. Vrooman HA, Cocosco CA, van der Lijn F, Stokking R, Ikram MA, Vernooij MW, et al. Multi-spectral brain tissue segmentation using automatically trained k-Nearest-Neighbor classification. Neuroimage. (2007) 37:71–81. doi: 10.1016/j.neuroimage.2007.05.018
42. Lao Z, Shen D, Liu D, Jawad AF, Melhem ER, Launer LJ, et al. Computer-Assisted Segmentation of white matter lesions in 3d mr images using support vector machine. Acad Radiol. (2008) 15:300–13. doi: 10.1016/j.acra.2007.10.012
43. Anbeek P, Vincken KL, van Bochove GS, van Osch MJP, van der Grond J. Probabilistic segmentation of brain tissue in MR imaging. Neuroimage. (2005) 27:795–804. doi: 10.1016/j.neuroimage.2005.05.046
44. Anbeek P, Vincken KL, van Osch MJP, Bisschops RHC, van der Grond J. Probabilistic segmentation of white matter lesions in MR imaging. Neuroimage. (2004) 21:1037–44. doi: 10.1016/j.neuroimage.2003.10.012
45. Wen W, Sachdev P. The topography of white matter hyperintensities on brain MRI in healthy 60- to 64-year-old individuals. Neuroimage. (2004) 22:144–54. doi: 10.1016/j.neuroimage.2003.12.027
46. Admiraal-Behloul F, van den Heuvel DMJ, Olofsen H, van Osch MJP, van der Grond J, van Buchem MA, et al. Fully automatic segmentation of white matter hyperintensities in MR images of the elderly. Neuroimage. (2005) 28:607–17. doi: 10.1016/J.NEUROIMAGE.2005.06.061
47. Brickman AM, Sneed JR, Provenzano FA, Garcon E, Johnert L, Muraskin J, et al. Quantitative approaches for assessment of white matter hyperintensities in elderly populations. Psychiatry Res Neuroimag. (2011) 193:101–6. doi: 10.1016/j.pscychresns.2011.03.007
48. DeCarli C, Fletcher E, Ramey V, Harvey D, Jagust WJ. Anatomical Mapping of White Matter Hyperintensities (WMH). Stroke. (2005) 36:50–5. doi: 10.1161/01.STR.0000150668.58689.f2
49. Geerlings MI, Appelman APA, Vincken KL, Algra A, Witkamp TD, Mali WPTM, et al. Brain volumes and cerebrovascular lesions on MRI in patients with atherosclerotic disease. The SMART-MR study Atherosclerosis. (2010) 210:130–6. doi: 10.1016/J.ATHEROSCLEROSIS.2009.10.039
50. Simons PCG, Algra A., Eikelboom BC, Grobbee DE, van der Graaf Y SMART study group. Carotid artery stenosis in patients with peripheral arterial disease: the SMART study. J Vasc Surgery. (1999) 30:519–25. doi: 10.1016/S.0741-5214(99)70079-0
51. 3C Study Group M, Pugliatti M, Hubbard R, Britton J, Sotgiu S, Sadovnick AD, et al. Vascular factors and risk of dementia: design of the three-city study and baseline characteristics of the study population. Neuroepidemiology. (2003) 22:316–25. doi: 10.1159/000072920
52. Feinleib M, Kannel WB, Garrison RJ, McNamara PM, Castelli WP. The framingham offspring study. Design and preliminary data. Prev Med. (1975) 4:518–25. doi: 10.1016/0091-7435(75)90037-7
53. Kannel WB, Feinleib M, McNamara PM, Garrison RJ, Castelli WP. An investigation of coronary heart disease in families. The Framingham offspring study. Am J Epidemiol. (1979) 110:281–90. doi: 10.1093/oxfordjournals.aje.a112813
54. Tang MX, Cross P, Andrews H, Jacobs DM, Small S, Bell K, et al. Incidence of AD in African-Americans, Caribbean Hispanics, and Caucasians in northern Manhattan. Neurology. (2001) 56:49–56. doi: 10.1212/WNL.56.1.49
55. Hofman A, Grobbee DE, de Jong PT, van den Ouweland FA. Determinants of disease and disability in the elderly: the Rotterdam Elderly Study. Eur J Epidemiol. (1991) 7:403–22. doi: 10.1007/BF00145007
56. Habes M, Sotiras A, Erus G, Toledo JB, Janowitz D, Wolk DA, et al. White matter lesions Spatial heterogeneity, links to risk factors, cognition, genetics, and atrophy. Neurol. (2018) 91:964–75. doi: 10.1212/WNL.0000000000006116
57. Windham BG, Wilkening SR, Lirette ST, Kullo IJ, Turner ST, Griswold ME, et al. Associations between inflammation and physical function in african americans and european americans with prevalent cardiovascular risk factors. J Am Geriatr Soc. (2016) 64:1448–55. doi: 10.1111/jgs.14229
58. Zade D, Beiser A, McGlinchey R, Au R, Seshadri S, Palumbo C, et al. Apolipoprotein Epsilon 4 allele modifies waist-to-hip ratio effects on cognition and brain structure. J Stroke Cerebrovasc Dis. (2013) 22:119–25. doi: 10.1016/j.jstrokecerebrovasdis.2011.06.020
59. Jochemsen HM, Geerlings MI, Grool AM, Vincken KL, Mali WP, van der Graaf Y, et al. Angiotensin-converting enzyme and progression of white matter lesions and brain atrophy – the SMART-MR Study. J Alzheimer's Dis. (2012) 29:39–49. doi: 10.3233/JAD-2012-111772
60. Godin O, Tzourio C, Maillard P, Mazoyer B, Dufouil C. Antihypertensive treatment and change in blood pressure are associated with the progression of white matter lesion volumes. Circulation. (2011) 123:266–73. doi: 10.1161/CIRCULATIONAHA.110.961052
61. Pase MP, Himali JJ, Mitchell GF, Beiser A, Maillard P, Tsao C, et al. Association of aortic stiffness with cognition and brain aging in young and middle-aged adults. Hypertension. (2016) 67:513–9. doi: 10.1161/HYPERTENSIONAHA.115.06610
62. Tully PJ, Debette S, Tzourio C. The association between systolic blood pressure variability with depression, cognitive decline and white matter hyperintensities: the 3C Dijon MRI study. Psychol Med. (2018) 48:1444–53. doi: 10.1017/S0033291717002756
63. Andersson C, Preis SR, Beiser A, DeCarli C, Wollert KC, Wang TJ, et al. Associations of circulating growth differentiation factor-15 and ST2 concentrations with subclinical vascular brain injury and incident Stroke. (2015) 46:2568–75. doi: 10.1161/STROKEAHA.115.009026
64. Coker LH, Espeland MA, Hogan PE, Resnick SM, Bryan RN, Robinson JG, et al. Change in brain and lesion volumes after CEE therapies: the WHIMS-MRI studies. Neurology. (2014) 82:427–34. doi: 10.1212/WNL.0000000000000079
65. Reitz C, Guzman VA, Narkhede A, DeCarli C, Brickman AM, Luchsinger JA. Relation of Dysglycemia to structural brain changes in a multiethnic elderly cohort. J Am Geriatr Soc. (2017) 65:277–85. doi: 10.1111/jgs.14551
66. Nayor M, Enserro DM, Beiser AS, Cheng S, DeCarli C, Vasan RS, et al. Association of exhaled carbon monoxide with stroke. incidence and subclinical vascular brain injury. Stroke. (2016) 47:383–9. doi: 10.1161/STROKEAHA.115.010405
67. Kanhai DA, de Kleijn DPV, Kappelle LJ, Uiterwaal CSPM, van der Graaf Y, Pasterkamp G, et al. Extracellular vesicle protein levels are related to brain atrophy and cerebral white matter lesions in patients with manifest vascular disease: the SMART-MR study. BMJ Open. (2014) 4:e003824. doi: 10.1136/bmjopen-2013-003824
68. Wright CB, Shah NH, Mendez AJ, DeRosa JT, Yoshita M, Elkind MSV, et al. Fibroblast growth factor 23 is associated with subclinical cerebrovascular damage. Stroke. (2016) 47:923–8. doi: 10.1161/STROKEAHA.115.012379
69. Hooshmand B, Mangialasche F, Kalpouzos G, Solomon A, Kåreholt I, Smith AD, et al. Association of vitamin b 12, folate, and sulfur amino acids with brain magnetic resonance imaging measures in older adults. JAMA Psychiatry. (2016) 73:606. doi: 10.1001/jamapsychiatry.2016.0274
70. Fornage M, Debette S, Bis JC, Schmidt H, Ikram MA, Dufouil C, et al. Genome-wide association studies of cerebral white matter lesion burden: the CHARGE consortium. Ann Neurol. (2011) 69:928–39. doi: 10.1002/ana.22403
71. Traylor M, Zhang CR, Adib-Samii P, Devan WJ, Parsons OE, Lanfranconi S, et al. Genome-wide meta-analysis of cerebral white matter hyperintensities in patients with stroke. Neurology. (2016) 86:146–53. doi: 10.1212/WNL.0000000000002263
72. Cloonan L, Fitzpatrick KM, Kanakis AS, Furie KL, Rosand J, Rost NS. Metabolic determinants of white matter hyperintensity burden in patients with ischemic stroke. Atherosclerosis. (2015) 240:149–53. doi: 10.1016/j.atherosclerosis.2015.02.052
73. Jimenez-Conde J, Biffi A, Rahman R, Kanakis A, Butler C, Sonni S, et al. Hyperlipidemia and reduced white matter hyperintensity volume in patients with ischemic Stroke. (2010) 41:437–42. doi: 10.1161/STROKEAHA.109.563502
74. Hafsteinsdottir SH, Eiriksdottir G, Sigurdsson S, Aspelund T, Harris TB, Launer LJ, et al. Brain tissue volumes by APOE genotype and leisure activity—the AGES-Reykjavik Study. Neurobiol Aging. (2012) 33:829.e1–829.e8. doi: 10.1016/j.neurobiolaging.2011.06.028
75. Chung C-P, Chou K-H, Peng L-N, Liu L-K, Lee W-J, Chen L-K, et al. Associations between low circulatory low-density lipoprotein cholesterol level and brain health in non-stroke non-demented subjects. Neuroimage. (2018) 181:627–34. doi: 10.1016/j.neuroimage.2018.07.049
76. Tiehuis AM, van der Graaf Y, Mali WPTM, Vincken K, Muller M, Geerlings MI. Metabolic syndrome, prediabetes, and brain abnormalities on mri in patients with manifest arterial disease: The SMART-MR Study. Diab Care. (2014) 37:2515–21. doi: 10.2337/dc14-0154
77. Romero JR, Vasan RS, Beiser AS, Au R, Benjamin EJ, DeCarli C, et al. Association of matrix metalloproteinases with MRI indices of brain ischemia and aging. Neurobiol Aging. (2010) 31:2128–35. doi: 10.1016/j.neurobiolaging.2008.11.004
78. Debette S, Beiser A, Hoffmann U, DeCarli C, O'Donnell CJ, Massaro JM, et al. Visceral fat is associated with lower brain volume in healthy middle-aged adults. Ann. Neurol. (2010) 68:136–44. doi: 10.1002/ana.22062
79. Yano Y, Butler KR, Hall ME, Schwartz GL, Knopman DS, Lirette ST, et al. Associations of nocturnal blood pressure with cognition by self-identified race in middle-aged and older adults: the GENOA (Genetic Epidemiology Network of Arteriopathy) Study. J Am Heart Assoc. (2017) 6:e007022. doi: 10.1161/JAHA.117.007022
80. Korada SKC, Zhao D, Gottesman RF, Guallar E, Lutsey PL, Alonso A, et al. Parathyroid hormone and subclinical cerebrovascular disease: the atherosclerosis risk in communities brain magnetic resonance imaging study. J Stroke Cerebrovasc Dis. (2016) 25:883–93. doi: 10.1016/j.jstrokecerebrovasdis.2015.12.029
81. Murabito JM, Beiser AS, DeCarli C, Seshadri S, Wolf PA, Au R. Parental longevity is associated with cognition and brain ageing in middle-aged offspring. Age Ageing. (2014) 43:358–63. doi: 10.1093/ageing/aft175
82. Weinstein G, Beiser AS, Au R, DeCarli C, Wolf PA, Seshadri S. Association of Parental Stroke with brain injury and cognitive measures in offspring. Stroke. (2013) 44:812–5. doi: 10.1161/STROKEAHA.112.680520
83. Aggarwal NT, Clark CJ, Beck TL, Mendes de Leon CF, DeCarli C, Evans DA, et al. Perceived stress is associated with subclinical cerebrovascular disease in older adults. Am J Geriatr Psychiatry. (2014) 22:53–62. doi: 10.1016/J.JAGP.2012.06.001
84. Kooistra M, Boss HM, van der Graaf Y, Kappelle LJ, Biessels GJ, Geerlings MI. Physical activity, structural brain changes and cognitive decline. SMART-MR study. Atherosclerosis. (2014) 234:47–53. doi: 10.1016/j.atherosclerosis.2014.02.003
85. Hilal S, Ikram MA, Verbeek MM, Franco OH, Stoops E, Vanderstichele H, et al. C-Reactive protein, plasma amyloid-β levels, and their interaction with magnetic resonance imaging markers. Stroke. (2018) 49:2692–8. doi: 10.1161/STROKEAHA.118.022317
86. Kaffashian S, Tzourio C, Soumare A, Dufouil C, Zhu Y, Crivello F, et al. Plasma -amyloid and MRI markers of cerebral small vessel disease: Three-City Dijon Study. Neurology. (2014) 83:2038–45. doi: 10.1212/WNL.0000000000001038
87. Tan ZS, Harris WS, Beiser AS, Au R, Himali JJ, Debette S, et al. Red blood cell omega-3 fatty acid levels and markers of accelerated brain aging. Neurology. (2012) 78:658–64. doi: 10.1212/WNL.0b013e318249f6a9
88. Cox SR, Allerhand M, Ritchie SJ, Muñoz Maniega S, Valdés Hernández M, Harris SE, et al. Longitudinal serum S100β and brain aging in the Lothian Birth Cohort 1936. Neurobiol Aging. (2018) 69:274–82. doi: 10.1016/j.neurobiolaging.(2018).05.029
89. Ramos AR, Dong C, Rundek T, Elkind MSV, Boden-Albala B, Sacco RL, et al. Sleep duration is associated with white matter hyperintensity volume in older adults: the Northern Manhattan Study. J Sleep Res. (2014) 23:524–30. doi: 10.1111/jsr.12177
90. Chaker L, Wolters FJ, Bos D, Korevaar TIM, Hofman A, van der Lugt A, et al. Thyroid function and the risk of dementia. Neurology. (2016) 87:1688–95. doi: 10.1212/WNL.0000000000003227
91. Lyall DM, Muñoz Maniega S, Harris SE, Bastin ME, Murray C, Lutz MW, et al. APOE/TOMM40 Genetic Loci, white matter hyperintensities, and cerebral microbleeds. Int J Stroke. (2015) 10:1297–300. doi: 10.1111/ijs.12615
92. Latourte A, Soumaré A, Bardin T, Perez-Ruiz F, Debette S, Richette P. Uric acid and incident dementia over 12 years of follow-up: a population-based cohort study. Ann Rheum Dis. (2018) 77:328–35. doi: 10.1136/annrheumdis-2016-210767
93. Rutten-Jacobs LCA, Tozer DJ, Duering M, Malik R, Dichgans M, Markus HS, et al. Genetic Study of white matter integrity in uk biobank (n = 8448) and the overlap with stroke. depression, and dementia. Stroke. (2018) 49:1340–7. doi: 10.1161/STROKEAHA.118.020811
94. Michos ED, Carson KA, Schneider ALC, Lutsey PL, Xing L, Sharrett AR, et al. Vitamin D and Subclinical cerebrovascular disease. JAMA Neurol. (2014) 71:863. doi: 10.1001/jamaneurol.2014.755
95. Freudenberger P, Petrovic K, Sen A, Töglhofer AM, Fixa A, Hofer E, et al. Fitness and cognition in the elderly. Neurology. (2016) 86:418–24. doi: 10.1212/WNL.0000000000002329
96. Brickman AM, Tosto G, Gutierrez J, Andrews H, Gu Y, Narkhede A, et al. An MRI measure of degenerative and cerebrovascular pathology in Alzheimer disease. Neurology. (2018) 91:e1402–12. doi: 10.1212/WNL.000(0000)000006310
97. Brickman AM, Zahodne LB, Guzman VA, Narkhede A, Meier IB, Griffith EY, et al. Reconsidering harbingers of dementia: progression of parietal lobe white matter hyperintensities predicts Alzheimer's disease incidence. Neurobiol Aging. (2015) 36:27–32. doi: 10.1016/j.neurobiolaging.2014.07.019
98. Burke SL, Hu T, Fava NM, Li T, Rodriguez MJ, Schuldiner KL, et al. Sex differences in the development of mild cognitive impairment and probable Alzheimer's disease as predicted by hippocampal volume or white matter hyperintensities. J Women Aging. (2018) 31:140–64. doi: 10.1080/08952841.2018.1419476
99. Lo RY, Jagust WJ. Vascular burden and Alzheimer disease pathologic progression. Neurology. (2012) 79:1349–55. doi: 10.1212/WNL.0b013e31826c1b9d
100. Geerlings MI, Brickman AM, Schupf N, Devanand DP, Luchsinger JA, Mayeux R, et al. Depressive symptoms, antidepressant use, and brain volumes on mri in a population-based cohort of old persons without dementia. J Alzheimer's Dis. (2012) 30:75–82. doi: 10.3233/JAD-2012-112009
101. Grool AM, van der Graaf Y, Mali WPTM, Geerlings MI. Location of cerebrovascular and degenerative changes, depressive symptoms and cognitive functioning in later life: the SMART-Medea study. J Neurol Neurosurg Psychiatry. (2011) 82:1093–100. doi: 10.1136/jnnp.2010.232413
102. Grool AM, Geerlings MI, Sigurdsson S, Eiriksdottir G, Jonsson PV, Garcia ME, et al. Structural MRI correlates of apathy symptoms in older persons without dementia: AGES-Reykjavik Study. Neurology. (2014) 82:1628–35. doi: 10.1212/WNL.0000000000000378
103. Ryberg C, Rostrup E, Sjöstrand K, Paulson OB, Barkhof F, Scheltens P, et al. White Matter Changes Contribute to Corpus Callosum Atrophy in the Elderly: The LADIS Study. Am J Neuroradiol. (2008) 29:1498–504. doi: 10.3174/ajnr.A1169
104. Ryberg C, Rostrup E, Stegmann MB, Barkhof F, Scheltens P, van Straaten ECW, et al. Clinical significance of corpus callosum atrophy in a mixed elderly population. Neurobiol Aging. (2007) 28:955–63. doi: 10.1016/j.neurobiolaging.2006.04.008
105. van der Veen PH, Muller M, Vincken KL, Hendrikse J, Mali WPTM, van der Graaf Y, et al. Longitudinal relationship between cerebral small-vessel disease and cerebral blood flow: the second manifestations of arterial disease-magnetic resonance study. Stroke. (2015) 46:1233–8. doi: 10.1161/STROKEAHA.114.008030
106. Wiegman AF, Meier IB, Provenzano FA, Schupf N, Manly JJ, Stern Y, et al. Regional white matter hyperintensity volume and cognition predict death in a multiethnic community cohort of older adults. J Am Geriatr Soc. (2013) 61:2246–8. doi: 10.1111/jgs.12568
107. Callisaya ML, Srikanth VK, Lord SR, Close JC, Brodaty H, Sachdev PS, et al. Sub-Cortical Infarcts and the risk of falls in older people: combined results of TASCOG and Sydney MAS Studies. Int J Stroke. (2014) 9:55–60. doi: 10.1111/ijs.12279
108. Dhamoon MS, Cheung Y-K, Moon Y, DeRosa J, Sacco R, Elkind MSV, et al. Cerebral white matter disease and functional decline in older adults from the Northern Manhattan Study: A longitudinal cohort study. PLoS Med. (2018) 15:e1002529. doi: 10.1371/journal.pmed.1002529
109. Saavedra Pérez HC, Ikram MA, Direk N, Prigerson HG, Freak-Poli R, Verhaaren BFJ, et al. Cognition, structural brain changes and complicated grief. A population-based study. Psychol Med. (2015) 45:1389–99. doi: 10.1017/S0033291714002499
110. Hamedani AG, Rose KM, Peterlin BL, Mosley TH, Coker LH, Jack CR, et al. Migraine and white matter hyperintensities: The ARIC MRI study. Neurology. (2013) 81:1308–13. doi: 10.1212/WNL.0b013e3182a8235b
111. Honningsvåg L-M, Håberg AK, Hagen K, Kvistad KA, Stovner LJ, Linde M. White matter hyperintensities and headache: A population-based imaging study (HUNT MRI). Cephalalgia. (2018) 38:1927–39. doi: 10.1177/0333102418764891
112. Kurth T, Mohamed S, Maillard P, Zhu Y-C, Chabriat H, Mazoyer B, et al. Headache, migraine, and structural brain lesions and function: population based Epidemiology of Vascular Ageing-MRI study. BMJ. (2011) 342:c7357–c7357. doi: 10.1136/bmj.c7357
113. Nyquist PA, Yanek LR, Bilgel M, Cuzzocreo JL, Becker LC, Chevalier-Davis K, et al. Effect of white matter lesions on manual dexterity in healthy middle-aged persons. Neurology. (2015) 84:1920–6. doi: 10.1212/WNL.0000000000001557
114. Devanand DP, Tabert MH, Cuasay K, Manly JJ, Schupf N, Brickman AM, et al. Olfactory identification deficits and MCI in a multi-ethnic elderly community sample. Neurobiol Aging. (2010) 31:1593–1600. doi: 10.1016/j.neurobiolaging.2008.09.008
115. Aribisala BS, Wiseman S, Morris Z, Valdés-Hernández MC, Royle NA, Maniega SM, et al. Circulating inflammatory markers are associated with magnetic resonance imaging-visible perivascular spaces but not directly with white matter hyperintensities. Stroke. (2014) 45:605–7. doi: 10.1161/STROKEAHA.113.004059
116. Rist PM, Tzourio C, Elbaz A, Soumaré A, Dufouil C, Mazoyer B, et al. Structural brain lesions and restless legs syndrome: a cross-sectional population-based study. BMJ Open. (2014) 4:e005938. doi: 10.1136/bmjopen-2014-005938
117. Mutlu U, Cremers LGM, de Groot M, Hofman A, Niessen WJ, van der Lugt A, et al. Retinal microvasculature and white matter microstructure. Neurology. (2016) 87:1003–10. doi: 10.1212/WNL.0000000000003080
118. Glymour MM, Chene G, Tzourio C, Dufouil C. Brain MRI markers and dropout in a longitudinal study of cognitive aging: The Three-City Dijon Study. Neurology. (2012) 79:1340–8. doi: 10.1212/WNL.0b013e31826cd62a
119. Stewart R, Godin O, Crivello F, Maillard P, Mazoyer B, Tzourio C, et al. Longitudinal neuroimaging correlates of subjective memory impairment: 4-year prospective community study. Br J Psychiatry. (2011) 198:199–205. doi: 10.1192/bjp.bp.110.078683
120. Seiler S, Fletcher E, Hassan-Ali K, Weinstein M, Beiser A, Himali JJ, et al. Cerebral tract integrity relates to white matter hyperintensities, cortex volume, and cognition. Neurobiol Aging. (2018) 72:14–22. doi: 10.1016/j.neurobiolaging.2018.08.005
121. Maniega SM, Valdés Hernández MC, Clayden JD, Royle NA, Murray C, Morris Z, et al. White matter hyperintensities and normal-appearing white matter integrity in the aging brain. Neurobiol Aging. (2015) 36:909–18. doi: 10.1016/j.neurobiolaging.2014.07.048
122. Vernooij MW, Ikram MA, Tanghe HL, Vincent AJPE, Hofman A, Krestin GP, et al. Incidental findings on brain MRI in the general population. N Engl J Med. (2007) 357:1821–8. doi: 10.1056/NEJMoa070972
123. Weber C, Noels H. Atherosclerosis: current pathogenesis and therapeutic options. Nat Med. (2011) 17:1410–22. doi: 10.1038/nm.2538
124. Aizenstein HJ, Baskys A, Boldrini M, Butters MA, Diniz BS, Jaiswal MK, et al. Vascular depression consensus report – a critical update. BMC Med. (2016) 14:161. doi: 10.1186/s12916-016-0720-5
125. Taylor WD, Aizenstein HJ, Alexopoulos GS. The vascular depression hypothesis: mechanisms linking vascular disease with depression. Mol Psychiatry. (2013) 18:963–74. doi: 10.1038/mp.2013.20
126. Kaffashian S, Tzourio C, Zhu Y-C, Mazoyer B, Debette S. Differential effect of white-matter lesions and covert brain infarcts on the risk of ischemic stroke. and intracerebral Hemorrhage. Stroke. (2016) 47:1923–5. doi: 10.1161/STROKEAHA.116.012734
127. Damangir S, Westman E, Simmons A, Vrenken H, Wahlund L-O, Spulber G. Reproducible segmentation of white matter hyperintensities using a new statistical definition. Magn Reson Mater Phys Biol Med. (2017) 30:227–37. doi: 10.1007/s10334-016-0599-3
128. Ghafoorian M, Karssemeijer N, Heskes T, van Uden IWM, Sanchez CI, Litjens G, et al. Location sensitive deep convolutional neural networks for segmentation of white matter hyperintensities. Sci Rep. (2017) 7:5110. doi: 10.1038/s41598-017-05300-5
129. Guerrero R, Qin C, Oktay O, Bowles C, Chen L, Joules R, et al. White matter hyperintensity and stroke lesion segmentation and differentiation using convolutional neural networks. NeuroImage Clin. (2018) 17:918–34. doi: 10.1016/j.nicl.2017.12.022
130. Moeskops P, de Bresser J, Kuijf HJ, Mendrik AM, Biessels GJ, Pluim JPW, et al. Evaluation of a deep learning approach for the segmentation of brain tissues and white matter hyperintensities of presumed vascular origin in MRI. NeuroImage Clin. (2018) 17:251–62. doi: 10.1016/j.nicl.2017.10.007
131. Taha AA, Hanbury A. Metrics for evaluating 3D medical image segmentation: analysis, selection, and tool. BMC Med Imag. (2015) 15:29. doi: 10.1186/s12880-015-0068-x
132. Poels MMF, Zaccai K, Verwoert GC, Vernooij MW, Hofman A, van der Lugt A, et al. Arterial stiffness and cerebral small vessel disease. Stroke. (2012) 43:2637–42. doi: 10.1161/STROKEAHA.111.642264
133. Grool AM, Graaf Y, Vincken KL, Witkamp TD, Mali WPTM, Geerlings MI. Antidepressant use is related to larger white matter lesion volume in patients with symptomatic atherosclerotic disease: the SMART-MR study. J Neurol. (2013) 260:197–206. doi: 10.1007/s00415-012-6616-1
134. Glazer H, Dong C, Yoshita M, Rundek T, Elkind MSV, Sacco RL, et al. Subclinical cerebrovascular disease inversely associates with learning ability: The NOMAS. Neurology. (2015) 84:2362–7. doi: 10.1212/WNL.0000000000001657
135. Nyquist PA, Bilgel MS, Gottesman R, Yanek LR, Moy TF, Becker LC, et al. Extreme deep white matter hyperintensity volumes are associated with african american race. Cerebrovasc Dis. (2014) 37:244–50. doi: 10.1159/000358117
136. Kooistra M, Geerlings MI, Mali WPTM, Vincken KL, van der Graaf Y, Biessels GJ. Diabetes mellitus and progression of vascular brain lesions and brain atrophy in patients with symptomatic atherosclerotic disease. SMART-MR study. J Neurol Sci. (2013) 332:69–74. doi: 10.1016/j.jns.2013.06.019
137. Huey ED, Manly JJ, Tang M-X, Schupf N, Brickman AM, Manoochehri M, et al. Course and etiology of dysexecutive MCI in a community sample. Alzheimer's Dement. (2013) 9:632–9. doi: 10.1016/J.JALZ.2012.10.014
138. Stefansdottir H, Arnar DO, Aspelund T, Sigurdsson S, Jonsdottir MK, Hjaltason H, et al. Atrial fibrillation is associated with reduced brain volume and cognitive function independent of cerebral infarcts. Stroke. (2013) 44:1020–5. doi: 10.1161/STROKEAHA.12.679381
139. Versluis CE, van der Mast RC, van Buchem MA, Bollen ELEM, Blauw GJ, Eekhof JAH, et al. Progression of cerebral white matter lesions is not associated with development of depressive symptoms in elderly subjects at risk of cardiovascular disease. The PROSPER Study Int J Geriatr Psychiatry. (2006) 21:375–81. doi: 10.1002/gps.1477
140. Swardfager W, Cogo-Moreira H, Masellis M, Ramirez J, Herrmann N, Edwards JD, et al. The effect of white matter hyperintensities on verbal memory. Neurology. (2018) 90:e673–e682. doi: 10.1212/WNL.0000000000004983
141. Tsao CW, Himali JJ, Beiser AS, Larson MG, DeCarli C, Vasan RS, et al. Association of arterial stiffness with progression of subclinical brain and cognitive disease. Neurology. (2016) 86:619–26. doi: 10.1212/WNL.0000000000002368
142. Kloppenborg RP, Geerlings MI, Visseren FL, Mali WPTM, Vermeulen M, van der Graaf Y, et al. Homocysteine and progression of generalized small-vessel disease: The SMART-MR Study. Neurology. (2014) 82:777–83. doi: 10.1212/WNL.0000000000000168
143. Carmichael O, Schwarz C, Drucker D, Fletcher E, Harvey D, Beckett L, et al. Longitudinal changes in white matter disease and cognition in the first year of the alzheimer disease neuroimaging initiative. Arch Neurol. (2010) 67:1370. doi: 10.1001/archneurol.2010.284
144. Tiehuis AM, van der Graaf Y, Visseren FL, Vincken KL, Biessels GJ, Appelman APA, et al. Diabetes increases atrophy and vascular lesions on brain mri in patients with symptomatic arterial disease. Stroke. (2008) 39:1600–3. doi: 10.1161/STROKEAHA.107.506089
145. Kloppenborg RP, Nederkoorn PJ, Grool AM, Vincken KL, Mali WPTM, Vermeulen M, et al. Cerebral small-vessel disease and progression of brain atrophy: The SMART-MR study. Neurology. (2012) 79:2029–36. doi: 10.1212/WNL.0b013e3182749f02
146. Vlek A, Visseren F, Kappelle L, Witkamp T, Vincken K, Mali W, et al. Blood Pressure and white matter lesions in patients with vascular disease: The SMART-MR Study. Curr Neurovasc Res. (2009) 6:155–62. doi: 10.2174/156720209788970027
147. Tully PJ, Debette S, Mazoyer B, Tzourio C. White matter lesions are associated with specific depressive symptom trajectories among incident depression and dementia populations: three-city dijon MRI Study. Am J Geriatr Psychiatry. (2017) 25:1311–21. doi: 10.1016/j.jagp.2017.06.003
148. Brickman AM, Schupf N, Manly JJ, Stern Y, Luchsinger JA, Provenzano FA, et al. APOE ε4 and risk for alzheimer's disease: do regionally distributed white matter hyperintensities play a role? Alzheimer's Dement. (2014) 10:619–29. doi: 10.1016/j.jalz.2014.07.155
149. Schwartz GL, Bailey KR, Mosley T, Knopman DS, Jack CR, Canzanello VJ, et al. Association of ambulatory blood pressure with ischemic brain injury. Hypertension. (2007) 49:1228–34. doi: 10.1161/HYPERTENSIONAHA.106.078691
150. Gardener H, Scarmeas N, Gu Y, Boden-Albala B, Elkind MSV, Sacco RL, et al. Mediterranean diet and white matter hyperintensity volume in the northern manhattan study. Arch Neurol. (2012) 69:251. doi: 10.1001/archneurol.2011.548
151. Teodorczuk A, O'Brien JT, Firbank MJ, Pantoni L, Poggesi A, Erkinjuntti T, et al. White matter changes and late-life depressive symptoms. Br J Psychiatry. (2007) 191:212–7. doi: 10.1192/bjp.bp.107.036756
152. Brickman AM, Schupf N, Manly JJ, Luchsinger JA, Andrews H, Tang MX, et al. Brain morphology in older african americans, caribbean hispanics, and whites from northern manhattan. Arch Neurol. (2008) 65:1053. doi: 10.1001/archneur.65.8.1053
153. Tiehuis AM, Mali WPTM, van Raamt AF, Visseren FLJ, Biessels GJ, van Zandvoort MJE, et al. Cognitive dysfunction and its clinical and radiological determinants in patients with symptomatic arterial disease and diabetes. J Neurol Sci. (2009) 283:170–4. doi: 10.1016/J.JNS.2009.02.337
154. Godin O, Dufouil C, Maillard P, Delcroix N, Mazoyer B, Crivello F, et al. White Matter Lesions as a predictor of depression in the elderly: the 3c-dijon study. Biol Psychiatry. (2008) 63:663–9. doi: 10.1016/J.BIOPSYCH.2007.09.006
155. Aggarwal NT, Wilson RS, Bienias JL, De Jager PL, Bennett DA, Evans DA, et al. The association of magnetic resonance imaging measures with cognitive function in a biracial population sample. Arch Neurol. (2010) 67:475–82. doi: 10.1001/archneurol.2010.42
156. Vernooij MW, Ikram MA, Vrooman HA, Wielopolski PA, Krestin GP, Hofman A, et al. White Matter Microstructural Integrity and Cognitive Function in a General Elderly Population. Arch Gen Psychiatry. (2009) 66:545. doi: 10.1001/archgenpsychiatry.2009.5
157. Ritchie K, Ancelin M-L, Beaino E, Portet F, Brickman AM, Dartigues J-F, et al. Retrospective Identification and characterization of mild cognitive impairment from a prospective population cohort. Am J Geriatr Psychiatry. (2010) 18:692–700. doi: 10.1097/JGP.0b013e3181df4897
158. Debette S, Seshadri S, Beiser A, Au R, Himali JJ, Palumbo C, et al. Midlife vascular risk factor exposure accelerates structural brain aging and cognitive decline. Neurology. (2011) 77:461–8. doi: 10.1212/WNL.0b013e318227b227
159. Jefferson AL, Massaro JM, Wolf PA, Seshadri S, Au R, Vasan RS, et al. Inflammatory biomarkers are associated with total brain volume: The Framingham Heart Study. Neurology. (2007) 68:1032–8. doi: 10.1212/01.wnl.0000257815.20548.df
160. Lorius N, Locascio JJ, Rentz DM, Johnson KA, Sperling RA, Viswanathan A, et al. Vascular disease and risk factors are associated with cognitive decline in the alzheimer disease spectrum. Alzheimer Dis Assoc Disord. (2015) 29:18–25. doi: 10.1097/WAD.0000000000000043
161. Kloppenborg RP, Nederkoorn PJ, van der Graaf Y, Geerlings MI. Homocysteine and cerebral small vessel disease in patients with symptomatic atherosclerotic disease. The SMART-MR study. Atherosclerosis. (2011) 216:461–6. doi: 10.1016/J.ATHEROSCLEROSIS.2011.02.027
162. Sigurdsson S, Aspelund T, Forsberg L, Fredriksson J, Kjartansson O, Oskarsdottir B, et al. Brain tissue volumes in the general population of the elderly. Neuroimage. (2012) 59:3862–70. doi: 10.1016/j.neuroimage.2011.11.024
163. Graff-Radford J, Madhavan M, Vemuri P, Rabinstein AA, Cha RH, Mielke MM, et al. Atrial fibrillation, cognitive impairment, and neuroimaging. Alzheimer's Dement. (2016) 12:391–8. doi: 10.1016/J.JALZ.2015.08.164
164. Biesbroek JM, Kuijf HJ, van der Graaf Y, Vincken KL, Postma A, Mali WPTM, et al. Association between subcortical vascular lesion location and cognition: a voxel-based and tract-based lesion-symptom mapping study. The SMART-MR Study. PLoS ONE. (2013) 8:e60541. doi: 10.1371/journal.pone.0060541
165. Liu J, Rutten-Jacobs L, Liu M, Markus HS, Traylor M. Causal impact of type 2 diabetes mellitus on cerebral small vessel disease: a mendelian randomization analysis. Stroke. (2018) 49:1325–31. doi: 10.1161/STROKEAHA.117.020536
166. Duering M, Gesierich B, Seiler S, Pirpamer L, Gonik M, Hofer E, et al. Strategic white matter tracts for processing speed deficits in age-related small vessel disease. Neurology. (2014) 82:1946–50. doi: 10.1212/WNL.0000000000000475
167. Croll PH, Voortman T, Ikram MA, Franco OH, Schoufour JD, Bos D, et al. Better diet quality relates to larger brain tissue volumes. Neurology. (2018) 90:e2166–73. doi: 10.1212/WNL.0000000000005691
168. Shoamanesh A, Preis SR, Beiser AS, Vasan RS, Benjamin EJ, Kase CS, et al. Inflammatory biomarkers, cerebral microbleeds, and small vessel disease: framingham heart study. Neurology. (2015) 84:825–32. doi: 10.1212/WNL.0000000000001279
169. Zahodne L, Manly J, Narkhede A, Griffith E, DeCarli C, Schupf N, et al. Structural MRI predictors of late-life cognition differ across african americans, hispanics, and whites. Curr Alzheimer Res. (2015) 12:632–9. doi: 10.2174/1567205012666150530203214
170. Godin O, Tzourio C, Rouaud O, Zhu Y, Maillard P, Pasquier F, et al. Joint effect of white matter lesions and hippocampal volumes on severity of cognitive decline: The 3C-Dijon MRI Study. J Alzheimer's Dis. (2010) 20:453–63. doi: 10.3233/JAD-2010-1389
171. Rundek T, Della-Morte D, Gardener H, Dong C, Markert MS, Gutierrez J, et al. Relationship between carotid arterial properties and cerebral white matter hyperintensities. Neurology. (2017) 88:2036–42. doi: 10.1212/WNL.0000000000003951
172. Habes M, Erus G, Toledo JB, Zhang T, Bryan N, Launer LJ, et al. White matter hyperintensities and imaging patterns of brain ageing in the general population. Brain. (2016) 139:1164–79. doi: 10.1093/brain/aww008
173. Maillard P, Crivello F, Dufouil C, Tzourio-Mazoyer N, Tzourio C, Mazoyer B. Longitudinal follow-up of individual white matter hyperintensities in a large cohort of elderly. Neuroradiology. (2009) 51:209–20. doi: 10.1007/s00234-008-0489-0
174. Verhaaren BFJ, Vernooij MW, de Boer R, Hofman A, Niessen WJ, van der Lugt A, et al. High blood pressure and cerebral white matter lesion progression in the general population. Hypertension. (2013) 61:1354–9. doi: 10.1161/HYPERTENSIONAHA.111.00430
175. Saczynski JS, Siggurdsson S, Jonsson PV, Eiriksdottir G, Olafsdottir E, Kjartansson O, et al. Glycemic status and brain injury in older individuals: the age gene/environment susceptibility-reykjavik study. Diab Care. (2009) 32:1608–13. doi: 10.2337/dc08-2300
176. Ritchie SJ, Tucker-Drob EM, Cox SR, Dickie DA, del C, Valdés Hernández M, et al. Risk and protective factors for structural brain ageing in the eighth decade of life. Brain Struct. Funct. (2017) 222:3477–90. doi: 10.1007/s00429-017-1414-2
177. van Sloten TT, Sigurdsson S, van Buchem MA, Phillips CL, Jonsson PV, Ding J, et al. Cerebral small vessel disease and association with higher incidence of depressive symptoms in a general elderly population: the ages-reykjavik study. Am J Psychiatry. (2015) 172:570–8. doi: 10.1176/appi.ajp.2014.14050578
178. Godin O, Tzourio C, Maillard P, Alpérovitch A, Mazoyer B, Dufouil C. Apolipoprotein E genotype is related to progression of white matter lesion load. Stroke. (2009) 40:3186–90. doi: 10.1161/STROKEAHA.109.555839
179. Vidal J-S, Sigurdsson S, Jonsdottir MK, Eiriksdottir G, Thorgeirsson G, Kjartansson O, et al. Coronary artery calcium, brain function and structure. Stroke. (2010) 41:891–7. doi: 10.1161/STROKEAHA.110.579581
180. Knopman DS, Griswold ME, Lirette ST, Gottesman RF, Kantarci K, Sharrett AR, et al. Vascular imaging abnormalities and cognition. Stroke. (2015) 46:433–40. doi: 10.1161/STROKEAHA.114.007847
181. Appelman AP, van der Graaf Y, Vincken KL, Tiehuis AM, Witkamp TD, Mali WP, et al. Total cerebral blood flow, white matter lesions and brain atrophy: the SMART-MR Study. J Cereb Blood Flow Metab. (2008) 28:633–9. doi: 10.(1038)/sj.jcbfm.(9600)563
182. Godin O, Maillard P, Crivello F, Rovitch A, Mazoyer B, Tzourio C, et al. Association of white-matter lesions with brain atrophy markers: the three-city dijon MRI study. Cerebrovasc Dis. (2009) 28:177–84. doi: 10.1159/000226117
183. Aparicio HJ, Petrea RE, Massaro JM, Manning WJ, Oyama-Manabe N, Beiser AS, et al. Association of descending thoracic aortic plaque with brain atrophy and white matter hyperintensities: the framingham heart study. Atherosclerosis. (2017) 265:305–11. doi: 10.1016/j.atherosclerosis.2017.06.919
184. Appelman APA, Vincken KL, van der Graaf Y, Vlek ALM, Witkamp TD, Mali WPTM, et al. White matter lesions and lacunar infarcts are independently and differently associated with brain atrophy: the SMART-MR study. Cerebrovasc Dis. (2010) 29:28–35. doi: 10.1159/000255971
185. Luchsinger JA, Brickman AM, Reitz C, Cho SJ, Schupf N, Manly JJ, et al. Subclinical cerebrovascular disease in mild cognitive impairment. Neurology. (2009) 73:450–6. doi: 10.1212/WNL.0b013e3181b1636a
186. Satizabal CL, Zhu YC, Mazoyer B, Dufouil C, Tzourio C. Circulating IL-6 and CRP are associated with MRI findings in the elderly: The 3C-Dijon Study. Neurology. (2012) 78:720–7. doi: 10.1212/WNL.0b013e318248e50f
187. Marcus J, Gardener H, Rundek T, Elkind MSV, Sacco RL, DeCarli C, et al. Baseline and Longitudinal Increases in diastolic blood pressure are associated with greater white matter hyperintensity volume. Stroke. (2011) 42:2639–41. doi: 10.1161/STROKEAHA.111.617571
188. Muller M, Appelman APA, van der Graaf Y, Vincken KL, Mali WPTM, Geerlings MI. Brain atrophy and cognition: interaction with cerebrovascular pathology? Neurobiol Aging. (2011) 32:885–93. doi: 10.1016/j.neurobiolaging.2009.05.005
189. Ritchie SJ, Dickie DA, Cox SR, Valdes Hernandez M, del C, Corley J, et al. Brain volumetric changes and cognitive ageing during the eighth decade of life. Hum Brain Mapp. (2015) 36:4910–25. doi: 10.1002/hbm.22959
190. Vibha D, Tiemeier H, Mirza SS, Adams HHH, Niessen WJ, Hofman A, et al. Brain volumes and longitudinal cognitive change. Alzheimer Dis Assoc Disord. (2018) 32:43–9. doi: 10.1097/WAD.0000000000000235
191. Jochemsen HM, Muller M, Bots ML, Scheltens P, Vincken KL, Mali WPTM, et al. Arterial stiffness and progression of structural brain changes: the SMART-MR study. Neurology. (2015) 84:448–55. doi: 10.1212/WNL.0000000000001201
192. Moran C, Phan TG, Chen J, Blizzard L, Beare R, Venn A, et al. Brain Atrophy in Type 2 Diabetes: Regional distribution and influence on cognition. Diab Care. (2013) 36:4036–42. doi: 10.2337/dc13-0143
193. van der Veen PH, Muller M, Vincken KL, Witkamp TD, Mali WPTM, van der Graaf Y, et al. Longitudinal changes in brain volumes and cerebrovascular lesions on MRI in patients with manifest arterial disease: the SMART-MR study. J Neurol Sci. (2014) 337:112–8. doi: 10.1016/j.jns.2013.11.029
194. Jokinen H, Melkas S, Madureira S, Verdelho A, Ferro JM, Fazekas F, et al. Cognitive reserve moderates long-term cognitive and functional outcome in cerebral small vessel disease. J Neurol Neurosurg Psychiatry. (2016) 87:1296–302. doi: 10.1136/jnnp-2016-313914
Keywords: white matter hyperintensities, white matter lesions, systematic review, large-scale studies, white matter hyperintensity segmentation, segmentation, cerebral small vessel disease
Citation: Frey BM, Petersen M, Mayer C, Schulz M, Cheng B and Thomalla G (2019) Characterization of White Matter Hyperintensities in Large-Scale MRI-Studies. Front. Neurol. 10:238. doi: 10.3389/fneur.2019.00238
Received: 26 October 2018; Accepted: 22 February 2019;
Published: 26 March 2019.
Edited by:
Andreas Charidimou, Massachusetts General Hospital and Harvard Medical School, United StatesReviewed by:
Anne-Katrin Giese, Massachusetts General Hospital and Harvard Medical School, United StatesLoes C. A. Rutten-Jacobs, University of Cambridge, United Kingdom
Copyright © 2019 Frey, Petersen, Mayer, Schulz, Cheng and Thomalla. This is an open-access article distributed under the terms of the Creative Commons Attribution License (CC BY). The use, distribution or reproduction in other forums is permitted, provided the original author(s) and the copyright owner(s) are credited and that the original publication in this journal is cited, in accordance with accepted academic practice. No use, distribution or reproduction is permitted which does not comply with these terms.
*Correspondence: Benedikt M. Frey, b.frey@uke.de
†These authors have contributed equally to this work