- 1Computational Neuroimaging Group, Academic Unit of Neurology, Trinity College Dublin, Dublin, Ireland
- 2Laboratoire d'Imagerie Biomédicale, Sorbonne University, CNRS, INSERM, Paris, France
- 3Department of Neurology, St James's Hospital, Dublin, Ireland
- 4Centre for Advanced Medical Imaging (CAMI), St James's Hospital, Dublin, Ireland
- 5School of Medicine, Trinity College Dublin, Dublin, Ireland
Background: Magnetic resonance imaging is a key diagnostic and monitoring tool in multiple Sclerosis (MS). While the substrates of motor and neuropsychological symptoms in MS have been extensively investigated, nystagmus-associated imaging signatures are relatively under studied. Accordingly, the objective of this study is the comprehensive characterisation of cortical, subcortical, and brainstem involvement in a cohort of MS patients with gaze-evoked nystagmus.
Methods: Patients were recruited from a specialist MS clinic and underwent multimodal neuroimaging including high-resolution structural and diffusion tensor data acquisitions. Morphometric analyses were carried out to evaluate patterns of cortical, subcortical, brainstem, and cerebellar gray matter pathology. Volumetric analyses were also performed to further characterize subcortical gray matter degeneration. White matter integrity was evaluated using axial-, mean-, and radial diffusivity as well as fractional anisotropy.
Results: Whole-brain morphometry highlighted considerable brainstem and cerebellar gray matter atrophy, and the tract-wise evaluation of white matter metrics revealed widespread pathology in frontotemporal and parietal regions. Nystagmus-associated gray matter degeneration was identified in medial cerebellar, posterior medullar, central pontine, and superior collicular regions. Volume reductions were identified in the putamen, thalamus and hippocampus.
Conclusions: Multiple sclerosis is associated with widespread gray matter pathology which is not limited to cortical regions but involves striatal, thalamic, cerebellar, and hippocampal foci. The imaging signature of gaze-evoked nystagmus in MS confirms the degeneration of key structures of the neural integrator network.
Introduction
Magnetic resonance (MR) imaging plays a central role in the diagnosis and monitoring of multiple sclerosis (MS) and is a key outcome measure of phase III clinical trials. While clinical radiology continues to focus on the estimation of white matter (WM) lesion load, recent imaging studies have been increasingly successful in capturing progressive cortical gray matter (GM) degeneration (1). Cortical pathology in MS has been evaluated by a variety of imaging techniques, including quantitative susceptibility mapping, (2) perfusion MR, (3, 4) magnetisation transfer ratio imaging, (5) proton spectroscopy (6) and functional network analyses (7). The drawback of these techniques is that they require carefully optimized sequences, time consuming data acquisitions and complex post-processing pipelines which make them better suited for research purposes than everyday clinical applications. While subcortical gray matter degeneration in MS has been associated with a number of non-motor symptoms, such as fatigue, (8–10) memory impairment, (11, 12) and depression, (13) these structures are difficult to evaluate qualitatively in a clinical setting. Cerebellar imaging in MS has also overwhelmingly focused on white matter metrics, even though cerebellar gray matter degeneration have been linked to motor disability, (14) impaired information processing, (15), and falls (16).
The pathological substrate of non-motor features of MS, such as pseudobulbar affect, fatigue, apathy, eye-movement disturbances, and neuropsychological deficits are relatively poorly characterized in contrast to the plethora of imaging studies focusing on motor disability and gait impairment. It is widely recognized that white matter pathology alone does not account for the heterogeneity of clinical manifestations in MS and that cortical and subcortical gray matter pathology contribute substantially to extra-motor deficits (17). While gaze-evoked nystagmus (GEN) is a common manifestation of MS, it is seldom evaluated specifically in dedicated imaging studies (18, 19). Nystagmus has considerable quality of life implications, it impacts on rehabilitation efforts, ability to work, reading, using computers, watching television, driving, and operating machinery (20). Additionally, nystagmus in MS has been linked to headaches, difficulties with concentration, and distractibility. GEN is widely regarded as a specific manifestation of neuronal integrator dysfunction, which provides insufficient discharge to fixate at an eccentric position, resulting in a drift to the primary gaze position and triggering a corrective saccade (21). Traditionally, an upper, middle and lower saccade pathway is distinguished, where the upper pathway contributes to saccade initiation and target setting (22, 23). The middle saccade pathway originates from the superior colliculus (SC) and innervates the lower saccade pathway which triggers the excitatory burst neurons (EBNs) in the paramedian pontine reticular formation (PPRF). The horizontal neural integrator is primarily located in the nucleus prepositus hypoglossi (NPH), and to a lesser extent in the medial vestibular nucleus (MVN) (22, 24). Pharmacological treatment options for GEN are limited (25). Baclofen is thought to increase the action potentials of Purkinje cells, thus enhancing neural step integrator firing (26, 27). Other drugs, such as Clonazepam, Gabapentin and memantine are often tried with varying efficacy (28, 29). Despite its high incidence, limited therapeutic options and quality of life implications, GEN in MS is surprisingly under investigated in MRI studies. Accordingly, we have undertaken a prospective imaging study of a cohort of MS patients who all had horizontal gaze-evoked nystagmus to assess cerebellar, brainstem, and subcortical gray matter degeneration in addition to white matter alterations.
Methods
Participants
A prospective multimodal neuroimaging study was undertaken with the participation of 31 multiple sclerosis patients and 20 age-matched healthy controls. Patients with multiple sclerosis were recruited from a specialist MS clinic in a tertiary referral center in St James's Hospital Dublin. Inclusion criteria included an established diagnosis of MS and persistent horizontal gaze-evoked nystagmus on clinical assessment. Patients were only included if they had had horizontal gaze-evoked nystagmus (GEN), (30) sustained, large amplitude, non-pendular, jerk nystagmus while attempting to maintain an eccentric eye position (30, 31). GEN and is easily distinguishable from physiological end-point nystagmus (EPN) and to ensure assessment uniformity all patients were assessed by the same experienced neurologist. Exclusion criteria included internuclear ophthalmoplegia, intracranial pathology other than MS, previous cerebrovascular events, prior neurosurgical intervention, uncontrolled hypertension, in addition to standard MR safety exclusion criteria. Based on the above criteria one healthy control was excluded due to an incidental intracranial finding; a meningioma, and one control's T1-weighted structural data could not be included due to movement artifacts. Nine MS patients have been excluded from diffusion tensor analyses due to poor quality raw data. All participants provided informed written consent in accordance with the ethics (IRB) approval of this study. The clinical and demographic profile of participants is summarized in Table 1.
MRI Data Acquisition
Magnetic resonance (MR) data were acquired on a 3 Tesla Philips Achieva system using an 8-channel receive-only head coil. T1-weighted images were acquired using a 3D volumetric fast gradient echo sequence with, spatial resolution = 1 × 1 × 1 mm3, field-of-view (FOV) of 240 × 240 × 163 mm, TR/TE = 25/2.1 ms, flip angle = 30°, SENSE factor = 2. Following file conversions and quality assessments, T1-weighted structural data were analyzed for 31 MS patients and 18 healthy controls. The parameters for fluid attenuated inversion recovery (FLAIR) imaging were the following: TR/TE, 11000/125 ms; TI: 2,800 ms; turbo factor: 31; refocusing angle; 120°; spatial resolution: 0.5 × 0.5 × 4 mm; SENSE: no. Diffusion tensor images (DTI) were acquired using a spin-echo planar imaging (SE-EPI) sequence with a 32-direction Stejskal-Tanner diffusion encoding scheme: FOV = 140 × 244 × 244 mm, 70 slices with no inter-slice gap, spatial resolution = 2 × 2 × 2 mm3, TR/TE = 12285/55 ms, SENSE factor = 2, b-values = 0, 1000 s/mm2, with SPIR fat suppression and dynamic stabilization in an acquisition time of 8 min 16 s. Following file conversions and quality assessments, diffusion tensor data was pre-processed for 22 MS patients and 19 healthy controls.
Gray Matter Analyses
In order to reduce the effect of white matter (WM) lesions on tissue-type segmentation (32–34) and subsequent volumetric and morphometric analyses, lesion mapping and lesion filling was implemented on each participant's imaging data. White matter lesions were segmented by a single rater on FLAIR images and individual lesion masks were created for each participant using FMRIB's FSLeyes. Each subject's FLAIR image was co-registered onto their 3D T1-weighted structural image using FMRIB's Linear Image Registration Tool (FLIRT) (35, 36) and the resulting transformation matrix was then applied to the lesion map for registration onto the 3D T1-weighted image. The FSL lesion filling tool (34) was used to reduce intensity contrast within known lesion areas and thereby improve the accuracy of brain segmentation. This method uses the original T1-weighted structural image and the co-registered lesion mask to fill lesions with intensities matching the surrounding normal-appearing WM and has been shown to reduce tissue-type misclassification and improve the accuracy of subsequent brain volume measurements (34).
A dual approach was undertaken to comprehensively evaluate patterns of gray matter pathology; surface-based morphometry was carried out to identify focal cortical thinning and voxel-based morphometry (VBM) was performed to explore regional density alterations. For cortical thickness measurements, the FreeSurfer image analysis suite was used (37). The pre-processing pipeline included the removal of non-brain tissue, segmentation of the subcortical white matter and deep gray matter structures, intensity normalization, tessellation of the gray matter-white matter boundary, and automated topology correction (38). False Discovery Rate (FDR) corrections were used for group comparisons, statistical threshold set at p < 0.05 and contrasts were adjusted for both age and gender. The FMRIB's software library (FSL) (39) was used to perform VBM (40). Subsequent to brain extraction and tissue-type segmentation, gray-matter partial volume images were aligned to the MNI152 standard space using affine registration. The gray matter partial volume estimates were non-linearly co-registered to a study-specific template, modulated by a Jacobian field warp and smoothed with an isotropic Gaussian kernel with a sigma of 3 mm. The threshold-free cluster-enhancement (TFCE) method (41) and permutation-based nonparametric inference were used for the comparison of MS patients and healthy controls controlling for age and gender (42, 43). The statistical significance was set at p < 0.05 family-wise error (FWE). Total intracranial volume (TIV) was estimated for each participant by linearly aligning the subject's skull-stripped brain to the MNI152 space, computing the inverse of the determinant of the affine matrix and multiplying it by the size of the template. Following registration to template with FSL-FLIRT (35, 36), tissue type segmentation was undertaken using FSL-FAST (44).
Subcortical Gray Matter Analyses
In order to comprehensively evaluate subcortical gray matter degeneration, structures which exhibited density reductions on VBM were further evaluated by volumetric analyses using subcortical segmentation. Following standard pre-processing steps, the subcortical segmentation and registration tool FIRST (45) of the FMRIB's Software Library was used to estimate volumes of the thalamus, hippocampus, putamen, and brainstem. Pipelines for subcortical segmentation and volume estimations were previously described (46). Briefly, FSL-FIRST uses a two-stage affine registration algorithm to register input T1 data sets to the Montreal Neurological Institute 152 (MNI152) standard space and a model-based approach is then implemented for the segmentation of subcortical structures. Subcortical mesh and volumetric outputs are generated following automatic boundary corrections. Exploratory comparative statistics were carried out with IBM's SPSS Statistics version 22. Analyses of covariance (ANCOVA) were conducted to compare volumes of subcortical structures between study groups. Assumptions of normality, linearity and homogeneity of variances were verified. Volumes of subcortical gray matter structures were included as dependent variables, and study group allocation as the categorical independent variable. Age at the time of MRI scan, gender, and total intracranial volume (TIV) were used as covariates. Following Bonferroni corrections, a p < 0.0125 was considered significant. Effect-sizes were calculated using partial Eta squares (η2). For illustrative purposes, boxplots of volumes were generated to highlight inter-group volumetric differences for each structure.
White Matter Analyses
Total lesion load volume was estimated based on skull-striped FLAIR images using the pipeline described by Wetter et al. (47) in FMRIB's FSL environment. Pre-processing of raw diffusion tensor imaging (DTI) datasets included eddy current corrections, motion corrections and brain-tissue extraction using FSL (48). Subsequent to fitting a diffusion tensor model, maps of axial diffusivity (AD), radial diffusivity (RD) mean diffusivity (MD), and fractional anisotropy (FA) were generated. Tract-based spatial statistics (TBSS) and permutation-based nonparametric inference was used to compare the white matter profile of controls and patients applying the threshold-free cluster enhancement (TFCE) method (41, 49). Voxels from spatially co-registered binary lesion masks were excluded from the TBSS analyses. Design matrices included age and gender as covariates, and statistical significance was set at p < 0.0125 FWE to correct for testing for four diffusivity parameters.
In addition to the above quantitative analyses and whole-brain lesion load estimation, infratentorial lesion patterns were also evaluated visually based on FLAIR hyperintensities and the percentage of patients with lesions in the mesencephalon, pons, medulla, cerebellar peduncles, cerebellar hemispheres were documented.
Results
Gray Matter Analyses
While surface-based morphometry did not identify statistically significant cortical thickness alterations in our MS cohort, voxel-based morphometry highlighted a pattern of gray matter degeneration involving bilateral cerebellar, brainstem, and medial thalamic regions. Additional subcortical gray matter pathology was identified in the left putamen and left hippocampus. At p < 0.05 FWE, no frontal, parietal, or temporal cortical changes have been captured (Figure 1).
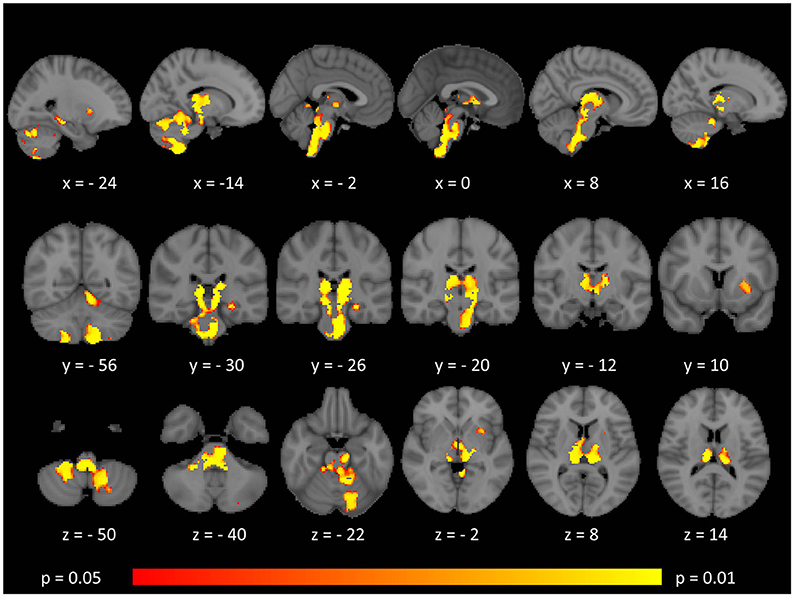
Figure 1. Patterns of gray matter atrophy in multiple sclerosis patients with gave-evoked nystagmus compared to healthy controls identified by voxel-based morphometry at p < 0.05 FWE adjusted for age and gender. Representative axial, coronal, and sagittal views are presented with corresponding MNI coordinates.
White Matter Analyses
The mean lesion load volume was 0.86 ± 0.34% of brain tissue volume (9.78 ± 4.07 mL) in the 31 MS patients who had structural data and 0.81 ± 0.32% of brain tissue volume (9.31 ± 4.04 mL) in the 22 MS patients who had diffusion tensor imaging data.
Tract-based spatial statistics confirmed widespread multi-lobar white matter degeneration for all four diffusivity metrics at p < 0.0125 FWE, defining the statistical threshold based on Bonferroni adjustments (Figures 2–5). The most widespread diffusivity changes were identified by axial diffusivity (AD) analyses (Figure 2). Statistical maps of fractional anisotropy (FA) only identified relatively focal changes confined to the body of the corpus callosum, left superior corticospinal tract, and the occipital lobe at this threshold (Figure 3). Maps of mean diffusivity (MD) highlighted symmetric frontal, parietal, temporal, and occipital white matter alterations (Figure 4) which were more widespread than those observed on radial diffusivity (RD) maps (Figure 5). Interestingly, none of the four diffusivity metrics captured infratentorial white matter changes in the brain stem or cerebellum at the above statistical thresholds.
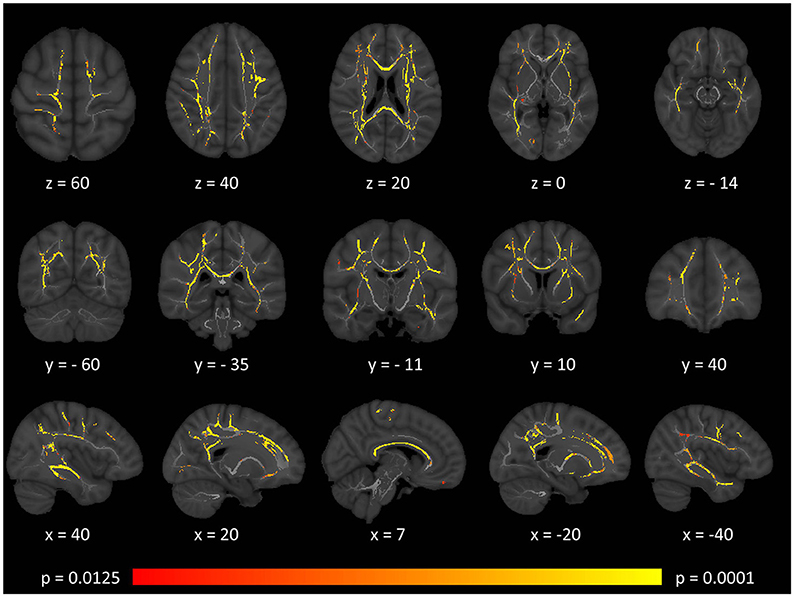
Figure 2. Patterns of increased axial diffusivity (AD) in multiple sclerosis patients with gave-evoked nystagmus compared to healthy controls at p < 0.0125 FWE adjusted for age and gender following tract-based spatial statistics. Representative axial, coronal, and sagittal views are presented with corresponding MNI coordinates.
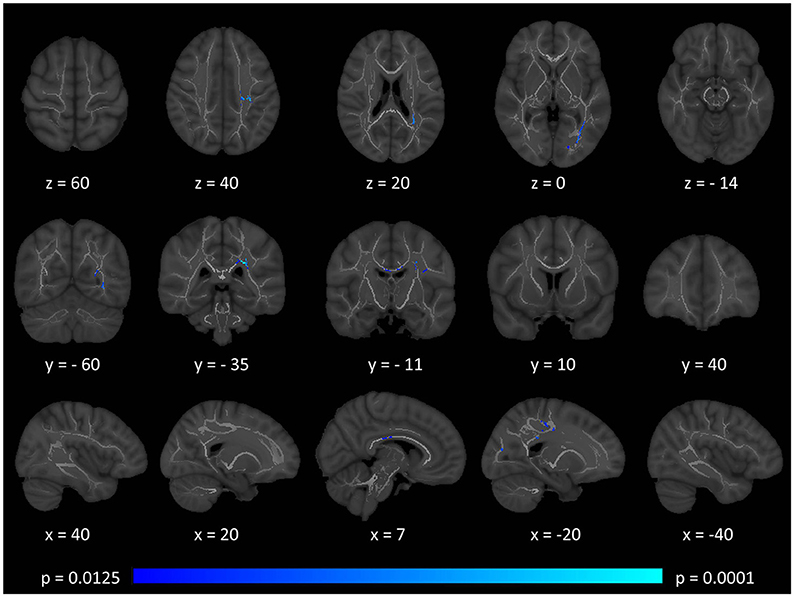
Figure 3. Patterns of decreased fractional anisotropy (FA) in multiple sclerosis patients with gave-evoked nystagmus compared to healthy controls at p < 0.0125 FWE adjusted for age and gender following tract-based spatial statistics. Representative axial, coronal, and sagittal views are presented with corresponding MNI coordinates.
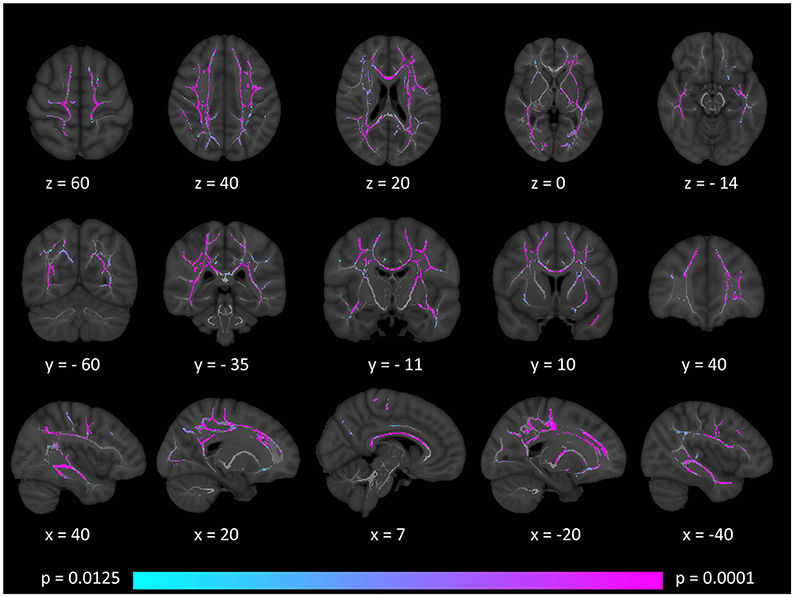
Figure 4. Patterns of increased mean diffusivity (MD) in multiple sclerosis patients with gave-evoked nystagmus compared to healthy controls at p < 0.0125 FWE adjusted for age and gender following tract-based spatial statistics. Representative axial, coronal, and sagittal views are presented with corresponding MNI coordinates.
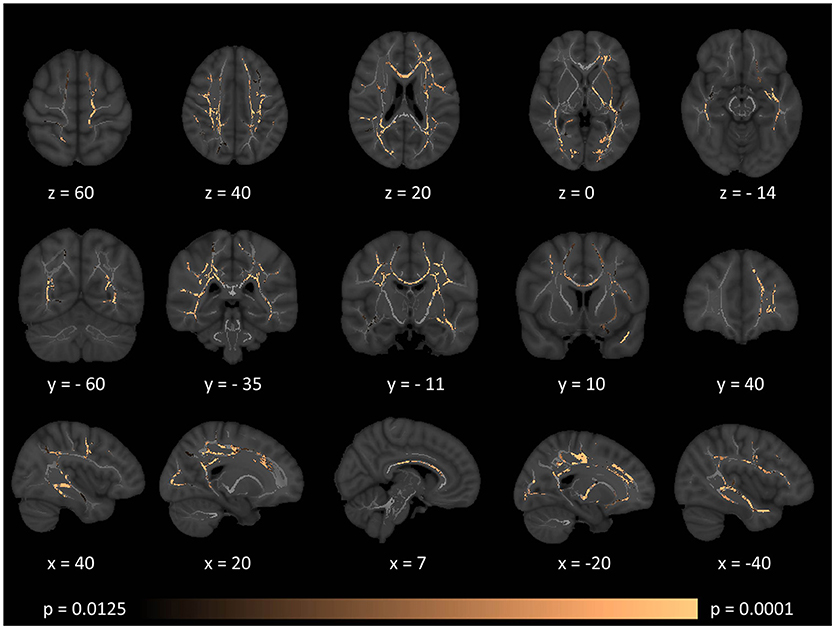
Figure 5. Patterns of increased radial diffusivity (RD) in multiple sclerosis patients with gave-evoked nystagmus compared to healthy controls at p < 0.0125 FWE adjusted for age and gender following tract-based spatial statistics. Representative axial, coronal, and sagittal views are presented with corresponding MNI coordinates.
FLAIR hyperintensities were detected in the mesencephalon in 22.5% of the patients, in the pons in 25.8% of the patients, in the medulla in 19.3% of the patients, in the cerebellar peduncles in 29% of the patients, in the cerebellar hemispheres in 35.4% of the patients. No obvious infratentorial FLAIR hyperintensities were visible in 54.8% of the patients.
Subcortical Gray Matter Volumes
Subcortical structures highlighted by voxel-based morphometry were further evaluated by volume estimations. Post-hoc comparisons of subcortical gray matter volumes (ANCOVA) highlighted significant putamen volume reductions in MS patients compared to controls. Considering the Bonferroni corrected threshold of p < 0.0125 a trend of thalamic (p = 0.017) and hippocampal (p = 0.014) atrophy was also identified. Table 2. The volumetric profiles of the four subcortical gray matter regions are further illustrated by box plots in Figure 6.
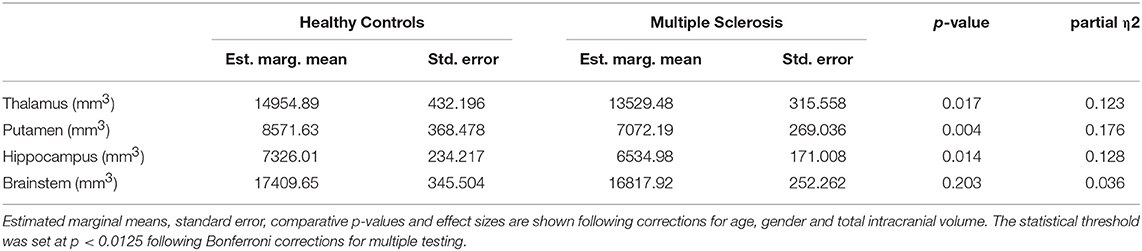
Table 2. The volumetric profile of subcortical gray matter structures and the brainstem in MS patients and controls.
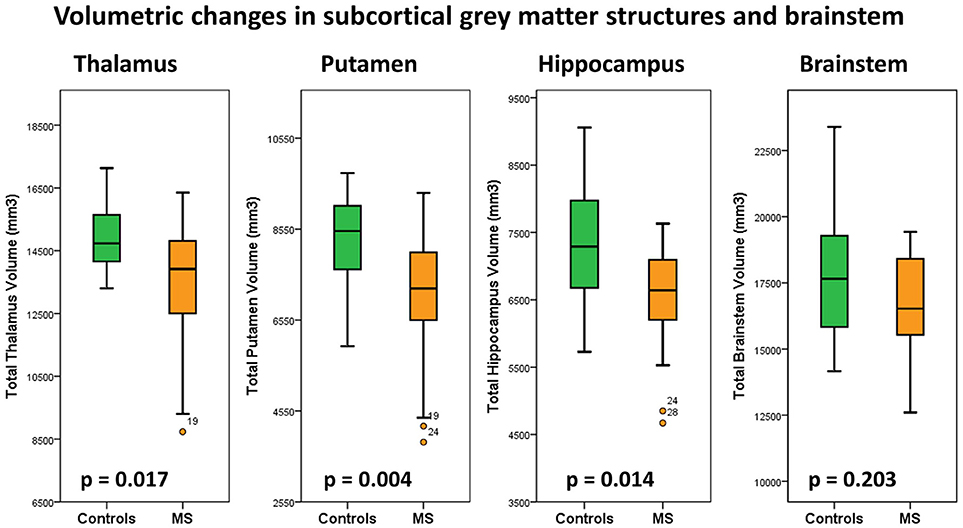
Figure 6. Total thalamus, putamen, hippocampus and brain stem volumes in healthy controls (green) and multiple sclerosis patients with gaze-evoked nystagmus (orange). P-values are corrected for age, gender and total intracranial volume. A statistical threshold of p < 0.0125 is considered significant following Bonferroni corrections for multiple testing.
Discussion
Our findings highlight extensive cerebellar, brainstem and subcortical gray matter degeneration in a cohort of MS patients with gaze-evoked nystagmus. We also show extensive supratentorial white matter degeneration using four diffusivity measures. Cerebellar and brain stem degeneration in our cohort is dominated by gray matter pathology which was readily identified by standard, whole-brain analysis pipelines. Our supplementary volumetric analyses have captured thalamic, putaminal, and hippocampal pathology.
Gray Matter Findings
Our standard morphometry analyses revealed bi-thalamic, bi-cerebellar and brainstem density reductions consistent with established nystagmus-associated anatomical foci. Additional gray matter degeneration was identified in the left putamen and left hippocampus. “Whole-brain” morphometry did not identify additional cortical pathology in either hemisphere. Similarly, surface-based morphometry did not identify cortical thickness alterations over the cerebral convexities.
Brainstem density reductions in the mesencephalon included the superior colliculus, a key structure in horizontal saccade control. The pontine changes incorporated the paramedian pontine reticular formation. The posterior medullary changes are consistent with the location of the nucleus prepositus hypoglossi and vestibular nuclei including the medial vestibular nucleus. The lack of significant cortical pathology suggests that the superior saccade pathway in our cohort is relatively intact and that the observed clinical signs are primarily driven by neural integrator network pathology in the brainstem (23, 50). The extensive brainstem gray matter pathology detected by VBM was not associated with volume reductions, (Table 2) tract-wise diffusivity alterations, (Figures 2–5) or widespread infratentorial lesion load on FLAIR.
Our gray matter analyses also map nystagmus-associated foci to medial cerebellar gray matter structures with relative sparing of the lateral and posterior aspects of the cerebellum. Pathology of specific cerebellar regions has been associated with specific types of nystagmus. Pathology of the flocculus and paraflocculus is traditionally linked to impaired suppression of the horizontal vestibulo-ocular reflex (VOR) during combined eye-head tracking (51). Nodulus and uvula pathology can result in positional nystagmus, periodic alternating nystagmus, or downbeat nystagmus. Gaze-evoked nystagmus is widely regarded as a manifestation of neural integrator network dysfunction (52, 53). In saccadic control a “pulse” and a “step” command is typically distinguished for neuronal discharge (54). The nucleus prepositus hypoglossi and to a lesser extent the medial vestibular nucleus contribute the “step” phase of neuronal activity. The superior colliculus in the midbrain controls saccade generation through pontine and midbrain pulse–step generator circuits and receives feedback from the brainstem burst generators (54). The role of midline cerebellar circuits is also well established in providing feed-back to burst generation (54). The etiology of GEN is linked to the inadequate “step” component, resulting in the eyes drifting back to their primary position and triggering a corrective saccade (22).
White Matter Findings
Tract-based spatial statistics (TBSS) demonstrated extensive bilateral supratentorial white matter degeneration in our cohort of patients. The divergent patterns of FA, AD, MD, and RD alterations highlight the value of multiparametric diffusion tensor imaging in contrast to relying on a single diffusivity metric. In our cohort, FA analyses highlighted anatomically limited white matter degeneration compared to the more extensive patterns identified by AD, MD, and RD. FA and MD are composite proxies of white matter integrity defined based on the three eigenvalues. While axial diffusivity is often regarded as an axonal marker, (55, 56) and radial diffusivity as a myelin related measure, (57, 58) this may be a simplistic interpretation especially in the absence of spatially matched histopathological data (59). The identification of the principle eigenvector and the accurate estimation of the three eigenvalues are particularly challenging in crossing fibers, low signal-to-noise sequences and in the presence of focal pathology (60, 61). Therefore, while AD and RD adds to the characterisation of GEN-associated white matter changes, the categorical interpretation of AD and RD alterations as axonal- vs. myelin-related pathology is not justified.
Our standard, “whole-brain” TBSS analyses have not captured cerebellar or brainstem white matter changes (Figures 2–5). Given the sensitivity of multiparametric (AD, MD, RD, FA) white matter imaging to detect white matter alterations, this may suggest that in our cohort of patients, infratentorial pathology is dominated by gray matter degeneration. (Figure 1) The qualitative appraisal of lesion patterns also revealed that 54.8% percent of our patients had no visible infratentorial FLAIR hyperintensities and only 22.5% of the patients had mesencephalic and 25.8% pontine lesions. The discrepancy between the limited infratentorial pathology seen on visual inspection and the considerable gray matter degeneration detected by VBM highlights the limitation of qualitative assessments. In our sample, we cannot attribute eye-movement abnormalities to gray matter pathology alone, as the infratentorial white matter lesions undoubtedly contribute to the clinical profile of our patients. The contribution of infratentorial white matter lesion patterns to specific eye-movement abnormalities has been studied across multiple conditions, including stroke, Arnold-Chiari malformations and multiple sclerosis (9, 62).
Subcortical Gray Matter Findings
We identified a trend of thalamic volume reduction in our MS cohort and VBM enabled the localisation of thalamic pathology to bilateral posterior-medial foci. There is accruing radiological, clinical and pathological evidence that thalamic changes can be detected early in the course of MS and may be associated with a range of clinical manifestations including fatigue, pain syndromes, cognitive, oculomotor, and motor disturbances (63–65). Whereas volumetric analyses only highlight global atrophy, our VBM analysis revealed focal medial thalamus, posterior hippocampus and lateral putamen pathology.
The involvement of putamen and hippocampus illustrates the spectrum of extra-motor degeneration in MS and signals two candidate regions-of-interest which could be specifically explored in longitudinal studies and clinical trials. Hippocampal atrophy has been previously linked to cornu ammonis degeneration and associated with learning and memory encoding difficulties (11, 12, 66). Putamen pathology is relatively rarely reported in imaging studies of MS (67) and extrapyramidal symptoms may be particularly difficult to ascertain clinically in the presence of extensive upper motor neuron signs. Despite extensive brainstem density alterations on VBM, no brainstem volume reductions were detected by our segmentation approach, which highlights the benefit of using multiple complementary imaging modalities.
Implications for Clinical Applications and Pharmacological Trials
In a clinical setting, scans of a given patient are often only qualitatively reviewed and lesion load is mostly visually estimated. The addition of 3D structural sequences without slice gaps and diffusion tensor sequences to clinical protocols may enable quantitative assessments and the accurate measurement of longitudinal change in the same patient. Magnetic resonance imaging is a key outcome measure in pharmaceutical trials, (68) and lesion load estimation based on T2-weighted imaging traditionally serves as a secondary endpoint for phase III clinical trials (69). In accordance with consensus guidelines, (70) recent clinical trials increasingly include cortical gray matter measures (71, 72). With very few exceptions however, (73) gray matter metrics of subcortical gray matter structures and the cerebellum are seldom utilized in clinical trials. The considerable cerebellar and deep gray matter degeneration highlighted by our study suggest that measures of these regions should also be evaluated as candidate biomarkers in MS. Cerebellar pathology is often exclusively linked to deficits in coordination and nystagmus, but its role in pseudobulbar affect and a range of cognitive functions is increasingly acknowledged (74, 75). An additional benefit of using quantitative metrics stems from their potential use in deep-learning and machine-learning applications which rely on advanced pattern recognition algorithms to aid diagnosis, patient stratification, and prognostication (76).
Limitations
The main limitations of this proof-of-concept study lie in its small cohort size and the lack of eye movement recordings which would have allowed more detailed eye movement analyses and clinico-radiological correlations. Despite the stringent recruitment criteria, our patients inherently exhibit a degree of clinical heterogeneity as evidenced by their EDSS and disease-duration profile. While we cannot claim clinical homogeneity in our sample, the “common denominator” from a symptoms-perspective is the presence of gaze-evoked nystagmus. Accordingly, an important expansion of this study would be the inclusion of an MS cohort without nystagmus as a “disease-control” group to confirm the specificity of our infratentorial findings as a GEN-associated neuroimaging signature. Another obvious expansion of this study would be the longitudinal follow-up of the study participants to characterize patterns of progressive gray matter degeneration, response to therapy and rate of decline.
Conclusions
Gaze-evoked nystagmus in multiple sclerosis is associated with multifocal gray matter pathology involving cerebellar, subcortical, and brainstem regions. White matter alterations in MS patients with gaze-evoked nystagmus are not limited to commissural tracts but involve widespread bi-hemispheric supratentorial regions. The imaging signature of gaze-evoked nystagmus in MS includes key structures of the oculomotor neural integrator network.
Ethics Statement
All procedures were performed in accordance with the ethical standards of the institutional ethics committee (SJH/AMNCH – St James's Hospital, Dublin, Ireland) and with the 1964 Helsinki declaration and its later amendments.
Author Contributions
PB, EF, RC, SLHS: study design, drafting the manuscript, data processing, statistical analyses, generation of figures and tables; JL: data processing, recruitment of participants, revision of the manuscript; JM: conceptualization and design of the study, data interpretation, revision of the manuscript; JR: conceptualization and design of the study, drafting and revision of the manuscript, supervision of all clinical aspects of the study, principle investigator.
Funding
PB is supported by the Health Research Board (HRB – Ireland; HRB EIA-2017-019), the Irish Institute of Clinical Neuroscience IICN – Novartis Ireland Research Grant, the Iris O'Brien Foundation, and the Perrigo Clinician-Scientist Research Fellowship.
Conflict of Interest Statement
The authors declare that the research was conducted in the absence of any commercial or financial relationships that could be construed as a potential conflict of interest.
Acknowleadgments
We gratefully acknowledge the generosity and kindness of our patients, their caregivers, and the healthy controls for participating in this research study.
Abbreviations
AD, Axial diffusivity; BG, Basal ganglia; CST, Corticospinal tract; DTI, Diffusion tensor imaging; EBN, Excitatory burst neurons; EDSS, Expanded Disability Status Scale; EPI, Echo-planar imaging; EPN, End-point nystagmus; FA, Fractional anisotropy; FAST, FMRIB’s Automated Segmentation Tool; FDR, False discovery rate; FLAIR, Fluid-attenuated inversion recovery; FLIRT, FMRIB’s Linear Image Registration Tool; FOV, Field-of-view; FEW, Familywise error; GEN, Gaze-evoked nystagmus; GM, gray matter; HC, Healthy control; MD, mean diffusivity; MLF, Medial longitudinal fasciculus; MNI152, Montreal Neurological Institute standard space; MS, Multiple sclerosis; MVN, Medial vestibular nucleus; NIN, Neural integrator network; NPH, Nucleus prepositus hypoglossi; PPMS, Primary progressive MS; PPRF, Paramedian pontine reticular formation; RD, Radial diffusivity; ROI, Region-of-interest; RRMS, Relapsing-remitting MS; SBM, Surface-based morphometry; SC, Superior colliculus; SPMS, Secondary progressive MS; TBSS, Tract-based spatial statistics; TE, Echo time; TFCE, Threshold-free cluster enhancement; TI, Inversion time; TR, Repetition time; T1WI, T1-weighted imaging; VBM, Voxel-based morphometry; WM, White matter.
References
1. Calabrese M, Castellaro M. Cortical gray matter MR imaging in multiple sclerosis. Neuroimaging Clin N Am. (2017) 27:301–12. doi: 10.1016/j.nic.2016.12.009
2. Castellaro M, Magliozzi R, Palombit A, Pitteri M, Silvestri E, Camera V, et al. Heterogeneity of cortical lesion susceptibility mapping in multiple sclerosis. AJNR Am J Neuroradiol. (2017) 38:1087–95. doi: 10.3174/ajnr.A5150
3. Debernard L, Melzer TR, Van Stockum S, Graham C, Wheeler-Kingshott CA, Dalrymple-Alford JC, et al. Reduced grey matter perfusion without volume loss in early relapsing-remitting multiple sclerosis. J Neurol Neurosurg Psychiatry (2014) 85:544–51. doi: 10.1136/jnnp-2013-305612
4. Peruzzo D, Castellaro M, Calabrese M, Veronese E, Rinaldi F, Bernardi V, et al. Heterogeneity of cortical lesions in multiple sclerosis: an MRI perfusion study. J Cereb Blood Flow Metab. (2013) 33:457–63. doi: 10.1038/jcbfm.2012.192
5. Pardini M, Sudre CH, Prados F, Yaldizli O, Sethi V, Muhlert N, et al. Relationship of grey and white matter abnormalities with distance from the surface of the brain in multiple sclerosis. J Neurol Neurosurg Psychiatry (2016) 87:1212–17. doi: 10.1136/jnnp-2016-313979
6. Chard DT, Griffin CM, McLean MA, Kapeller P, Kapoor R, Thompson AJ, et al. Brain metabolite changes in cortical grey and normal-appearing white matter in clinically early relapsing-remitting multiple sclerosis. Brain (2002) 125(Pt 10):2342–52. doi: 10.1093/brain/awf240
7. Liu Y, Wang H, Duan Y, Huang J, Ren Z, Ye J, et al. Functional brain network alterations in clinically isolated syndrome and multiple sclerosis: a graph-based connectome study. Radiology (2017) 282:534–41. doi: 10.1148/radiol.2016152843
8. Finke C, Schlichting J, Papazoglou S, Scheel M, Freing A, Soemmer C, et al. Altered basal ganglia functional connectivity in multiple sclerosis patients with fatigue. Mult Scler. (2015) 21:925–34. doi: 10.1177/1352458514555784
9. Nourbakhsh B, Azevedo C, Nunan-Saah J, Maghzi AH, Spain R, Pelletier D, et al. Longitudinal associations between brain structural changes and fatigue in early MS. Mult Scler Relat Dis. (2016) 5:29–33. doi: 10.1016/j.msard.2015.10.006
10. Damasceno A, Damasceno BP, Cendes F. Atrophy of reward-related striatal structures in fatigued MS patients is independent of physical disability. Mult Scler. (2016) 22:822–29. doi: 10.1177/1352458515599451
11. Gonzalez Torre JA, Cruz-Gomez AJ, Belenguer A, Sanchis-Segura C, Avila C, Forn C. Hippocampal dysfunction is associated with memory impairment in multiple sclerosis: a volumetric and functional connectivity study. Mult Scler. (2017) 23:1854–63. doi: 10.1177/1352458516688349
12. Planche V, Ruet A, Coupe P, Lamargue-Hamel D, Deloire M, Pereira B, et al. Hippocampal microstructural damage correlates with memory impairment in clinically isolated syndrome suggestive of multiple sclerosis. Mult Scler. (2017) 23:1214–24. doi: 10.1177/1352458516675750
13. Stuke H, Hanken K, Hirsch J, Klein J, Wittig F, Kastrup A, et al. Cross-sectional and longitudinal relationships between depressive symptoms and brain atrophy in ms patients. Front Hum Neurosci. (2016) 10:622. doi: 10.3389/fnhum.2016.00622
14. Cocozza S, Petracca M, Mormina E, Buyukturkoglu K, Podranski K, Heinig MM, et al. Cerebellar lobule atrophy and disability in progressive MS. J Neurol Neurosurg Psychiatry (2017) 88:1065–72. doi: 10.1136/jnnp-2017-316448
15. Moroso A, Ruet A, Lamargue-Hamel D, Munsch F, Deloire M, Coupe P, et al. Posterior lobules of the cerebellum and information processing speed at various stages of multiple sclerosis. J Neurol Neurosurg Psychiatry (2017) 88:146–51. doi: 10.1136/jnnp-2016-313867
16. Kalron A, Allali G, Achiron A. Cerebellum and cognition in multiple sclerosis: the fall status matters. J Neurol. (2018) 265:809–16. doi: 10.1007/s00415-018-8774-2
17. O'Callaghan C, Bertoux M, Hornberger M. Beyond and below the cortex: the contribution of striatal dysfunction to cognition and behaviour in neurodegeneration. J Neurol Neurosurg Psychiatry (2013) 85:371–78. doi: 10.1136/jnnp-2012-304558
18. Nakamagoe K, Fujizuka N, Koganezawa T, Yamaguchi T, Tamaoka A. Downbeat nystagmus associated with damage to the medial longitudinal fasciculus of the pons: a vestibular balance control mechanism via the lower brainstem paramedian tract neurons. J Neurol Sci. (2013) 328:98–101. doi: 10.1016/j.jns.2013.02.017
19. Lopez LI, Bronstein AM, Gresty MA, Du Boulay EP, Rudge P. Clinical and MRI correlates in 27 patients with acquired pendular nystagmus. Brain (1996) 119(Pt 2):465–72. doi: 10.1093/brain/119.2.465
20. Francis CE. Visual issues in multiple sclerosis. Phys Med Rehabil Clin North Am. (2013) 24:687–702. doi: 10.1016/j.pmr.2013.06.002
21. Suzuki Y, Kase M, Hashimoto M, Ohtsuka K. Leaky neural integration observed in square-wave jerks. Jpn J Ophthalmol. (2003) 47:535–36. doi: 10.1016/S0021-5155(03)00144-8
22. Godaux E, Cheron G. The hypothesis of the uniqueness of the oculomotor neural integrator: direct experimental evidence in the cat. J Physiol. (1996) 492(Pt 2):517–27. doi: 10.1113/jphysiol.1996.sp021326
23. Basso MA, Sommer MA. Exploring the role of the substantia nigra pars reticulata in eye movements. Neuroscience (2011) 198:205–12. doi: 10.1016/j.neuroscience.2011.08.026
24. Sparks DL. The brainstem control of saccadic eye movements. Nat Rev Neurosci. (2002) 3:952–64. doi: 10.1038/nrn986
25. Beh SC, Tehrani AS, Kheradmand A, Zee DS. Damping of monocular pendular nystagmus with vibration in a patient with multiple sclerosis. Neurology (2014) 82:1380–1. doi: 10.1212/WNL.0000000000000324
26. Averbuch-Heller L, Tusa RJ, Fuhry L, Rottach KG, Ganser GL, Heide W, et al. A double-blind controlled study of gabapentin and baclofen as treatment for acquired nystagmus. Ann Neurol. (1997) 41:818–25. doi: 10.1002/ana.410410620
27. Strupp M, Thurtell MJ, Shaikh AG, Brandt T, Zee DS, Leigh RJ. Pharmacotherapy of vestibular and ocular motor disorders, including nystagmus. J Neurol. (2011) 258:1207–22. doi: 10.1007/s00415-011-5999-8
28. Rucker JC. Current treatment of nystagmus. Curr Treat Options Neurol. (2005) 7:69–77. doi: 10.1007/s11940-005-0008-0
29. Thurtell MJ, Joshi AC, Leone AC, Tomsak RL, Kosmorsky GS, Stahl JS, et al. Crossover trial of gabapentin and memantine as treatment for acquired nystagmus. Ann Neurol. (2010) 67:676–80. doi: 10.1002/ana.21991
30. Stahl JS, Averbuch-Heller L, Leigh RJ. Acquired nystagmus. Arch Ophthalmol. (2000) 118:544–9. doi: 10.1001/archopht.118.4.544
31. Cannon SC, Robinson DA. Loss of the neural integrator of the oculomotor system from brain stem lesions in monkey. J Neurophysiol. (1987) 57:1383–409. doi: 10.1152/jn.1987.57.5.1383
32. Gelineau-Morel R, Tomassini V, Jenkinson M, Johansen-Berg H, Matthews PM, Palace J. The effect of hypointense white matter lesions on automated gray matter segmentation in multiple sclerosis. Hum Brain Mapp. (2012) 33:2802–14. doi: 10.1002/hbm.21402
33. Chard DT, Jackson JS, Miller DH, Wheeler-Kingshott CA. Reducing the impact of white matter lesions on automated measures of brain gray and white matter volumes. J Magn Reson Imaging (2010) 32:223-8. doi: 10.1002/jmri.22214
34. Battaglini M, Jenkinson M, De Stefano N. Evaluating and reducing the impact of white matter lesions on brain volume measurements. Hum Brain Mapp. (2012) 33:2062–71. doi: 10.1002/hbm.21344
35. Jenkinson M, Smith S. A global optimisation method for robust affine registration of brain images. Med Image Anal. (2001) 5:143–56. doi: 10.1016/S1361-8415(01)00036-6
36. Jenkinson M, Bannister P, Brady M, Smith S. Improved optimization for the robust and accurate linear registration and motion correction of brain images. Neuroimage (2002) 17:825–41. doi: 10.1006/nimg.2002.1132
38. Fischl B, Dale AM. Measuring the thickness of the human cerebral cortex from magnetic resonance images. Proc Nat Acad Sci USA. (2000) 97:11050–5. doi: 10.1073/pnas.200033797
39. Douaud G, Smith S, Jenkinson M, Behrens T, Johansen-Berg H, Vickers J, et al. Anatomically related grey and white matter abnormalities in adolescent-onset schizophrenia. Brain (2007) 130(Pt 9):2375–86. doi: 10.1093/brain/awm184
40. Good CD, Johnsrude IS, Ashburner J, Henson RN, Friston KJ, Frackowiak RS. A voxel-based morphometric study of ageing in 465 normal adult human brains. Neuroimage (2001) 14(1 Pt 1):21–36. doi: 10.1006/nimg.2001.0786
41. Smith SM, Nichols TE. Threshold-free cluster enhancement: addressing problems of smoothing, threshold dependence and localisation in cluster inference. Neuroimage (2009) 44:83–98. doi: 10.1016/j.neuroimage.2008.03.061
42. Winkler AM, Ridgway GR, Webster MA, Smith SM, Nichols TE. Permutation inference for the general linear model. Neuroimage (2014) 92:381–97. doi: 10.1016/j.neuroimage.2014.01.060
43. Nichols TE, Holmes AP. Nonparametric permutation tests for functional neuroimaging: a primer with examples. Hum Brain Mapp. (2002) 15:1–25. doi: 10.1002/hbm.1058
44. Zhang Y, Brady M, Smith S. Segmentation of brain MR images through a hidden Markov random field model and the expectation-maximization algorithm. IEEE Trans Med Imaging (2001) 20:45–57. doi: 10.1109/42.906424
45. Patenaude B, Smith SM, Kennedy DN, Jenkinson M. A Bayesian model of shape and appearance for subcortical brain segmentation. Neuroimage. (2011) 56:907–22. doi: 10.1016/j.neuroimage.2011.02.046
46. Bede P, Elamin M, Byrne S, McLaughlin RL, Kenna K, Vajda A, et al. Basal ganglia involvement in amyotrophic lateral sclerosis. Neurology (2013) 81:2107–15. doi: 10.1212/01.wnl.0000437313.80913.2c
47. Wetter NC, Hubbard EA, Motl RW, Sutton BP. Fully automated open-source lesion mapping of T2-FLAIR images with FSL correlates with clinical disability in MS. Brain Behav. (2016) 6:e00440. doi: 10.1002/brb3.440
48. Smith SM, Jenkinson M, Woolrich MW, Beckmann CF, Behrens TEJ, Johansen-Berg H, et al. Advances in functional and structural MR image analysis and implementation as FSL. Neuroimage (2004) 23(Suppl. 1):208–19. doi: 10.1016/j.neuroimage.2004.07.051
49. Smith SM, Jenkinson M, Johansen-Berg H, Rueckert D, Nichols TE, Mackay CE, et al. Tract-based spatial statistics: voxelwise analysis of multi-subject diffusion data. Neuroimage (2006) 31:1487–505. doi: 10.1016/j.neuroimage.2006.02.024
50. Shires J, Joshi S, Basso MA. Shedding new light on the role of the basal ganglia-superior colliculus pathway in eye movements. Curr Opin Neurobiol. (2010) 20:717–25. doi: 10.1016/j.conb.2010.08.008
51. Sharpe JA, Goldberg HJ, Lo AW, Herishanu YO. Visual-vestibular interaction in multiple sclerosis. Neurology (1981) 31:427–33. doi: 10.1212/WNL.31.4_Part_2.427
52. Arnold DB, Robinson DA. The oculomotor integrator: testing of a neural network model. Exp. Brain Res. (1997) 113:57–74. doi: 10.1007/BF02454142
53. Cannon SC, Robinson DA. An improved neural-network model for the neural integrator of the oculomotor system: more realistic neuron behavior. Biol Cybernet. (1985) 53:93–108. doi: 10.1007/BF00337026
54. Scudder CA, Kaneko CS, Fuchs AF. The brainstem burst generator for saccadic eye movements: a modern synthesis. Exp Brain Res. (2002) 142:439–62. doi: 10.1007/s00221-001-0912-9
55. Budde MD, Xie M, Cross AH, Song SK. Axial diffusivity is the primary correlate of axonal injury in the experimental autoimmune encephalomyelitis spinal cord: a quantitative pixelwise analysis. J Neurosci. (2009) 29:2805–13. doi: 10.1523/JNEUROSCI.4605-08.2009
56. Sun SW, Liang HF, Trinkaus K, Cross AH, Armstrong RC, Song SK. Noninvasive detection of cuprizone induced axonal damage and demyelination in the mouse corpus callosum. Magn Reson Med. (2006) 55:302–8. doi: 10.1002/mrm.20774
57. Song SK, Sun SW, Ramsbottom MJ, Chang C, Russell J, Cross AH. Dysmyelination revealed through MRI as increased radial (but unchanged axial) diffusion of water. Neuroimage (2002) 17:1429–36. doi: 10.1006/nimg.2002.1267
58. Song SK, Yoshino J, Le TQ, Lin SJ, Sun SW, Cross AH, et al. Demyelination increases radial diffusivity in corpus callosum of mouse brain. Neuroimage (2005) 26:132–40. doi: 10.1016/j.neuroimage.2005.01.028
59. Wheeler-Kingshott CA, Cercignani M. About “axial” and “radial” diffusivities. Magn Reson Med. (2009) 61:1255–60. doi: 10.1002/mrm.21965
60. Wheeler-Kingshott CA, Ciccarelli O, Schneider T, Alexander DC, Cercignani M. A new approach to structural integrity assessment based on axial and radial diffusivities. Funct Neurol. (2012) 27:85–90.
61. Field AS, Alexander AL, Wu YC, Hasan KM, Witwer B, Badie B. Diffusion tensor eigenvector directional color imaging patterns in the evaluation of cerebral white matter tracts altered by tumor. J Magn Reson Imaging (2004) 20:555–62. doi: 10.1002/jmri.20169
62. Bronstein AM, Miller DH, Rudge P, Kendall BE. Down beating nystagmus: magnetic resonance imaging and neuro-otological findings. J Neurol Sci. (1987) 81:173–84. doi: 10.1016/0022-510X(87)90094-3
63. Minagar A, Barnett MH, Benedict RH, Pelletier D, Pirko I, Sahraian MA, et al. The thalamus and multiple sclerosis: modern views on pathologic, imaging, and clinical aspects. Neurology (2013) 80:210–9. doi: 10.1212/WNL.0b013e31827b910b
64. Bisecco A, Rocca MA, Pagani E, Mancini L, Enzinger C, Gallo A, et al. Connectivity-based parcellation of the thalamus in multiple sclerosis and its implications for cognitive impairment: a multicenter study. Hum Brain Mapp. (2015) 36:2809–25. doi: 10.1002/hbm.22809
65. Fujiwara E, Kmech JA. Cognitive implications of deep gray matter iron in multiple sclerosis. AJNR Am J Neuroradiol. (2017) 38:942–8. doi: 10.3174/ajnr.A5109
66. Sicotte NL, Kern KC, Giesser BS, Arshanapalli A, Schultz A, Montag M, et al. Regional hippocampal atrophy in multiple sclerosis. Brain (2008) 131(Pt 4):1134–41. doi: 10.1093/brain/awn030
67. Schmalbrock P, Prakash RS, Schirda B, Janssen A, Yang GK, Russell M, et al. Basal ganglia iron in patients with multiple sclerosis measured with 7t quantitative susceptibility mapping correlates with inhibitory control. Am J Neuroradiol. (2016) 37:439–46. doi: 10.3174/ajnr.A4599
68. Bermel RA, Fisher E, Cohen JA. The use of MR imaging as an outcome measure in multiple sclerosis clinical trials. Neuroimaging clinics of North America. (2008) 18:687–701 xi. doi: 10.1016/j.nic.2008.06.008
69. Filippi M, Horsfield MA, Ader HJ, Barkhof F, Bruzzi P, Evans A, et al. Guidelines for using quantitative measures of brain magnetic resonance imaging abnormalities in monitoring the treatment of multiple sclerosis. Ann Neurol. (1998) 43:499–506. doi: 10.1002/ana.410430414
70. Geurts JJ, Roosendaal SD, Calabrese M, Ciccarelli O, Agosta F, Chard DT, et al. Consensus recommendations for MS cortical lesion scoring using double inversion recovery MRI. Neurology (2011) 76:418–24. doi: 10.1212/WNL.0b013e31820a0cc4
71. Miller DH, Soon D, Fernando KT, MacManus DG, Barker GJ, Yousry TA, et al. MRI outcomes in a placebo-controlled trial of natalizumab in relapsing MS. Neurology (2007) 68:1390–401. doi: 10.1212/01.wnl.0000260064.77700.fd
72. Radue EW, O'Connor P, Polman CH, Hohlfeld R, Calabresi P, Selmaj K, et al. Impact of fingolimod therapy on magnetic resonance imaging outcomes in patients with multiple sclerosis. Arch Neurol. (2012) 69:1259–69. doi: 10.1001/archneurol.2012.1051
73. Filippi M, Rocca MA, Pagani E, De Stefano N, Jeffery D, Kappos L, et al. Placebo-controlled trial of oral laquinimod in multiple sclerosis: MRI evidence of an effect on brain tissue damage. J Neurol Neurosurg Psychiatry (2014) 85:851–8. doi: 10.1136/jnnp-2013-306132
74. Bede P, Finegan E. Revisiting the pathoanatomy of pseudobulbar affect: mechanisms beyond corticobulbar dysfunction. Amyotroph Lateral Scler Frontotemporal Degener. (2017) 19:4–6. doi: 10.1080/21678421.2017.1392578
75. Guell X, Gabrieli JDE, Schmahmann JD. Triple representation of language, working memory, social and emotion processing in the cerebellum: convergent evidence from task and seed-based resting-state fMRI analyses in a single large cohort. Neuroimage (2018) 172:437–49. doi: 10.1016/j.neuroimage.2018.01.082
Keywords: multiple sclerosis, nystagmus, brainstem, cerebellum, striatum, thalamus, hippocampus
Citation: Bede P, Finegan E, Chipika RH, Li Hi Shing S, Lambe J, Meaney J and Redmond J (2018) Occulomotor Neural Integrator Dysfunction in Multiple Sclerosis: Insights From Neuroimaging. Front. Neurol. 9:691. doi: 10.3389/fneur.2018.00691
Received: 10 May 2018; Accepted: 31 July 2018;
Published: 23 August 2018.
Edited by:
Maria Assunta Rocca, San Raffaele Hospital (IRCCS), ItalyReviewed by:
Niels Bergsland, University at Buffalo, United StatesCris S. Constantinescu, University of Nottingham, United Kingdom
Copyright © 2018 Bede, Finegan, Chipika, Li Hi Shing, Lambe, Meaney and Redmond. This is an open-access article distributed under the terms of the Creative Commons Attribution License (CC BY). The use, distribution or reproduction in other forums is permitted, provided the original author(s) and the copyright owner(s) are credited and that the original publication in this journal is cited, in accordance with accepted academic practice. No use, distribution or reproduction is permitted which does not comply with these terms.
*Correspondence: Peter Bede, YmVkZXBAdGNkLmll