- 1 Department of Sleep and Cognition, Netherlands Institute for Neuroscience, an Institute of the Royal Netherlands Academy of Arts and Sciences, Amsterdam, Netherlands
- 2 BCN NeuroImaging Center, University Medical Center Groningen, Groningen, Netherlands
- 3 Leibniz Institute for Neurobiology, Otto von Guericke University, Magdeburg, Germany
- 4 Department of Psychiatry, VU University Medical Center, Amsterdam, Netherlands
- 5 Department of Anatomy and Neurosciences, VU University Medical Center, Amsterdam, Netherlands
- 6 Department of Psychiatry, Leiden University Medical Center Leiden, Netherlands
- 7 Leiden Institute for Brain and Cognition, Leiden University, Leiden, Netherlands
- 8 Departments of Integrative Neurophysiology and Medical Psychology, Center for Neurogenomics and Cognitive Research, VU University and Medical Center, Amsterdam, Netherlands
Sleep complaints increase profoundly with age; prevalence estimates of insomnia in the elderly reach up to 37%. The three major types of nocturnal complaints are difficulties initiating (DIS) and maintaining (DMS) sleep and early morning awakening (EMA), of which the latter appears most characteristic for aging. The neural correlates associated with these complaints have hardly been investigated, hampering the development of rational treatment and prevention. A recent study on structural brain correlates of insomnia showed that overall severity, but not duration, of insomnia complaints is associated with lower gray matter (GM) density in part of the left orbitofrontal cortex (OFC). Following up on this, we investigated, in an independent sample of people not diagnosed with insomnia, whether individual differences in GM density are associated with differences in DIS, DMS, and EMA. Sixty five healthy participants (mean age = 41 years, range 18–56) filled out questionnaires and underwent structural magnetic resonance imaging. Three compound Z-scores were computed for questionnaire items relating to DIS, DMS, and EMA. Whole-brain voxel-based morphometry was used to investigate their association with GM density. Results show that participants with lower GM density in a region where the left inferior OFC borders the insula report more EMA, but not DIS or DMS. This is the first study to investigate structural brain correlates of specific sleep characteristics that can translate into complaints in insomniacs. The selective association of EMA with orbitofrontal GM density makes our findings particularly relevant to elderly people, where EMA represents the most characteristic complaint. It is hypothesized that low GM density in aforementioned orbitofrontal area affects its role in sensing comfort. An intact ability to evaluate comfort may be crucial to maintain sleep, especially at the end of the night when sleep is vulnerable because homeostatic sleep propensity has dissipated.
Introduction
With prevalence estimates of 4–11%, chronic insomnia belongs to the most common disorders. The most important risk factor for insomnia is age; estimates of the prevalence in elderly people reach up to about 40% (Foley et al., 1995; Morphy et al., 2007). Individual sleep complaints are much more frequent, with an estimated one third of the population experiencing at least one DSM-IV insomnia symptom (Ohayon, 2002). Three relatively independent major sleep complaints can be discriminated, which can be present with variable severity; difficulties initiating sleep (DIS), difficulties maintaining sleep (DMS), and early morning awakening (EMA; American Psychiatric Association, 1994; American Academy of Sleep Medicine, 2005). Of these three, complaints of EMA most clearly increase with age, while DIS may actually decrease with age; both in clinically diagnosed insomniacs and in the general population of elderly people (Abe and Suzuki, 1985; National Sleep Foundation, 2003a,b). Additional daytime complaints of insomniacs concern non-restorative sleep and problems with daytime functioning.
In spite of the high prevalence and strong impact on well-being, quality of life and society (Leger and Bayon, 2010), the brain mechanisms underlying insomnia have hardly been explored. Several psychological models have been proposed, mostly addressing three factors; predisposing personality traits like the tendency to worry, precipitating events like stress, and perpetuating attitudes and practices like misconceptions about required sleep (Spielman, 1986; Perlis et al., 1997). Physiological observations have identified a “hyperaroused” central and autonomic nervous system profile; not only in insomniacs (Bonnet and Arand, 1997; Riemann et al., 2010), but also in individuals that are at risk of developing insomnia (Fernandez-Mendoza et al., 2010). Nevertheless, the brain mechanisms that underlie predisposing traits and a hyperaroused profile have hardly been explored.
Behavioral genetics studies in cohorts of twins and families indicate a considerable heritability of insomnia (Beaulieu-Bonneau et al., 2007) as well as sleep complaints in general (Boomsma et al., 2008). Heritable structural brain characteristics that might be associated with insomnia complaints are thus of particular interest. One of the most heritable structural brain characteristics is the topography of cerebral cortical gray matter (GM) density, particularly so for prefrontal GM where heritability estimates reach up to 95% (Thompson et al., 2001). A recent study demonstrated that elderly insomniacs have a significantly reduced GM density in part of the left orbitofrontal cortex (OFC; Altena et al., 2010). The reduction was found in a region compatible with the border of Brodmann areas 47/12M and 13L of the “orbital network” (Ongur et al., 2003), which is proposed to be essential for the hedonic evaluation of somatosensory input (Kringelbach, 2005), including the evaluation of thermal comfort (Dunn et al., 2010). The observation of compromised thermal comfort-evaluation abilities in elderly insomniacs (Raymann and Van Someren, 2008) supports the possible functional relevance of a reduction in GM density in this relatively small orbitofrontal area. Furthermore, individual differences in GM density in the aforementioned region were strongly correlated with differences in the overall severity of complaints, rendering it the first candidate for a structural brain correlate of insomnia (Altena et al., 2010). In striking contrast, individual differences in GM density were not associated with differences in the duration of insomnia. This dissociation suggests that a low GM density in a region at the border of areas 47/12M and 13L of the OFC is more likely to represent a pre-existing vulnerability to insomnia complaints, rather than a consequence of having experienced a period of insomnia.
The present study aimed to follow up on the promising suggestion of a neural correlate of insomnia by Altena et al. (2010), by investigating, in an independent sample of participants not diagnosed with insomnia, whether individual differences in GM density in aforementioned orbitofrontal region of interest (ROI) are associated with individual differences in the three major types of sleep characteristics: DIS, DMS, and EMA.
Materials and Methods
Participants and Procedures
Good quality magnetic resonance imaging (MRI) scans and completed questionnaires could be obtained from 65 self-declared healthy controls participating in the Netherlands Study of Depression and Anxiety (NESDA) neuroimaging study, which is part of a large-scale multisite, longitudinal, observational cohort study on the development and course of anxiety and depression (Penninx et al., 2008). Participants were between 18 and 57 years of age and had never been diagnosed with any of the DSM-IV disorders (American Psychiatric Association, 1994). Further exclusion criteria were the presence or history of major internal or neurological disorder, dependence on or recent abuse (past year) of alcohol and/or drugs, hypertension, any suspicion of current subclinical depression (see van Tol et al., 2010), and contraindications for participating in an MRI study. Diagnoses according to DSM-IV criteria were established using the structured Composite International Diagnostic Interview, lifetime version 2.1 (Robins et al., 1988), assessed by a trained interviewer. Table 1 provides an overview of participant characteristics, including data on circadian preference (mid-sleep time on free days corrected for sleep debt, computed as described in the supplemental data of; Roenneberg et al., 2004) and overall severity of insomnia complaints (total score on the Women’s Health Initiative Insomnia Rating Scale; WHIIRS, see below). The ethical review boards of each center approved this study and all participants provided consent after receiving written information.
Assessment of Sleep Complaints
Participants filled out a range of self-rating questionnaires, which included the WHIIRS (Levine et al., 2003a,b) and an early version of the Munich Chronotype Questionnaire (MCTQ, Zavada et al., 2005). Although the WHIIRS has only been validated in women, it contains clearly defined items pertaining to all the core symptoms of insomnia and can thus readily be used to investigate structural brain correlates of specific sleep characteristics in both women and men. Compound Z-scores were computed for questionnaire items relating to the three main sleep characteristics: DIS, DMS, and EMA. An estimate of DIS was obtained by calculating the average of standardized ratings on an item of the WHIIRS that asked about trouble falling asleep, and three items of the MCTQ, which ask for the time needed to fall asleep on workdays, on days off, and in general, respectively. An estimate of DMS was obtained by calculating the average of standardized ratings on three items of the WHIIRS that ask about waking up several times at night, about having trouble getting back to sleep, and about restlessness of one’s typical night’s sleep. An estimate of EMA was obtained by calculating the average of standardized ratings on an item of the WHIIRS asking about waking up earlier than planned, and two items of the MCTQ (Zavada et al., 2005) that ask for the time in the morning one is wide awake on workdays and days off.
Magnetic Resonance Imaging Acquisition
Imaging data were acquired using a Philips 3T MRI system (Best, The Netherlands) located at the Leiden University Medical Center (LUMC), Amsterdam Medical Center (AMC) of the University of Amsterdam or the University Medical Center Groningen (UMCG), equipped with a SENSE-8 (LUMC and UMCG) or SENSE-6 (AMC) channel head coil. For each subject, anatomical images were obtained using a sagittal three-dimensional gradient-echo T1-weighted sequence (repetition time = 9 ms; echo time = 3.5 ms; matrix = 256 × 256; voxel size = 1 mm isotropic; 170 slices).
Voxel-Based Morphometry-Processing
Imaging data were analyzed using voxel-based morphometry (VBM: Ashburner and Friston, 2000) with Diffeomorphic Anatomical Registration using Exponentiated Lie algebra (DARTEL: Ashburner, 2007) in the Statistical Parametric Mapping (SPM version 5; http://www.fil.ion.ucl.ac.uk/spm) suite of tools. DARTEL is a fully deformable registration and normalization method that is effectively unconstrained by number of degrees of freedom. It has proven good registration accuracy and has been recommended in favor of standard SPM normalization or the SPM unified segmentation approaches for whole-brain and regional analysis without segmenting regions of interest (Yassa and Stark, 2009). Pre-processing of the images has been described in detail previously (van Tol et al., 2010) and included (1) manual reorientation; (2) segmentation; (3) registration, normalization, and modulation (leaving the images in DARTEL template space at a resolution of 1.5 mm isotropic); and (4) smoothing at 8 mm full-width at half-maximum. Total (native) GM volume for each participant was computed by summing all the voxels in their GM image resulting from segmentation (step 2).
Voxel-Based Morphometry Analysis
Using the peak voxel from the voxel-wise regression analysis against severity of insomnia as reported in Altena et al. (2010), we created a spherical ROI with a 16 mm radius in aforementioned DARTEL template space. First, a matrix describing registration from Montreal Neurological Institute (MNI) space to DARTEL template space was computed using fully affine registration. Next, the obtained transformation matrix was used to transform MNI coordinates (x, y, z = −20, 22, −14) to the present DARTEL template space. Finally, a sphere with a 16 mm radius was centered on these coordinates using the WFU Pickatlas tools (Maldjian et al., 2003, 2004). Visual inspection showed that the resulting ROI predominantly contained parts of the left OFC as well as smaller parts of the left medial frontal, anterior insular and subcallosal cortex, and left caudate. All voxel-wise analyses were performed using the general linear model framework of SPM (Friston et al., 1995). To test for associations with DIS, DMS, and EMA, a whole-brain voxel-wise regression analysis was performed. Age, sex, total GM volume, and imaging site (by means of two dummy variables) were entered as covariates in each analysis. To achieve maximal sensitivity, optimize voxel residual smoothness estimation, and exclude false positives outside GM tissue, voxel-wise analyses were masked using a explicit optimal threshold GM mask created using the Masking toolbox (Ridgway et al., 2009). To preserve optimal normalization accuracy, we left the normalized, modulated, and smoothed images in DARTEL space. Therefore, coordinates are not equivalent to MNI coordinates. Regions are identified using the detailed brain atlas of Talairach and Tournoux (1988). Additionally, we registered the DARTEL template to MNI space and applied the resulting transformation matrix to the coordinates of the peak voxel in DARTEL space, resulting in an approximation of the location in standard space.
Statistical thresholding was performed roughly analogous to the original publication by van Tol et al. (2010); i.e., when performing whole-brain voxel-wise analyses we applied different statistical thresholds for analysis within and analysis outside a ROI. For analyses within the 16 mm radius spherical ROI centered on the peak voxel reported by Altena et al. (2010), we used a two-step approach to reduce the likelihood of Type I errors. Initially, a whole-brain voxel-wise height-threshold of Z > 3.09 (P < 0.001) and cluster size threshold of 50 contiguous voxels was set. If this initial analysis showed a significant cluster within our ROI, it was followed by a conclusive analysis within our ROI at a threshold of P < 0.05, family wise error voxel-corrected. For analysis outside our ROI, a whole-brain threshold of P < 0.05 family wise error cluster-corrected was set.
To gain more insight into effect size as well as for illustrative purposes, we computed mean GM density within significant clusters by averaging of the GM probabilities for each voxel in a cluster. To investigate the amount of explained variance in mean GM density within a significant cluster by DIS, DMS, and EMA, multiple regression analysis was performed twice; once with and once without DIS, DMS, or EMA, using age, sex, total GM volume, and imaging site (by means of two dummy variables) as predictors of no interest in each analysis.
Analysis of Sleep Measures
The general linear model framework of Statistical Package for the Social Sciences (SPSS version 17.0, Chicago, IL, USA) was used for the analyses of compound Z-scores. Reliability (internal consistency) of the DIS, DMS, and EMA measures was evaluated by calculating Cronbach’s alpha.
Missing Data
In case of missing values on any of the questionnaire items in a participant, compound Z-scores were computed over the remaining items for that specific sleep complaint. For DMS, compound Z-scores could not be computed in three participants because scores were not available for any of the three items that make up DMS, leaving 62 participants for the analysis of the association of GM density and DMS.
Results
Voxel-Based Morphometry Analysis
Voxel-wise analysis showed that the EMA compound Z-score significantly predicted GM density in a 66 voxel, 0.223 cc cluster at P < 0.001 uncorrected. The complete cluster was located within our 16 mm radius spherical ROI and the peak voxel (approximate MNI coordinates = −30, 13, −13) survived correction for multiple comparisons (P = 0.029, family wise error voxel-corrected). An auxiliary analysis, using mid-sleep time on free days (corrected for sleep debt) instead of EMA to predict GM density, showed no significant voxels within our ROI at P < 0.001 uncorrected. The cluster for EMA encompassed the region where the left inferior OFC borders with the most anterior aspect of the insular cortex (Figure 1). The association of EMA with the average GM density within this cluster (i.e., an average of the GM probabilities for each voxel in the cluster) is visualized in the scatterplot of Figure 2; EMA explained an additional 6% of variance on top of the other predictors age, sex, total GM volume, and imaging site. Voxel-wise analysis at P < 0.001 uncorrected within our ROI showed no significant association of GM density with either DIS or DMS.
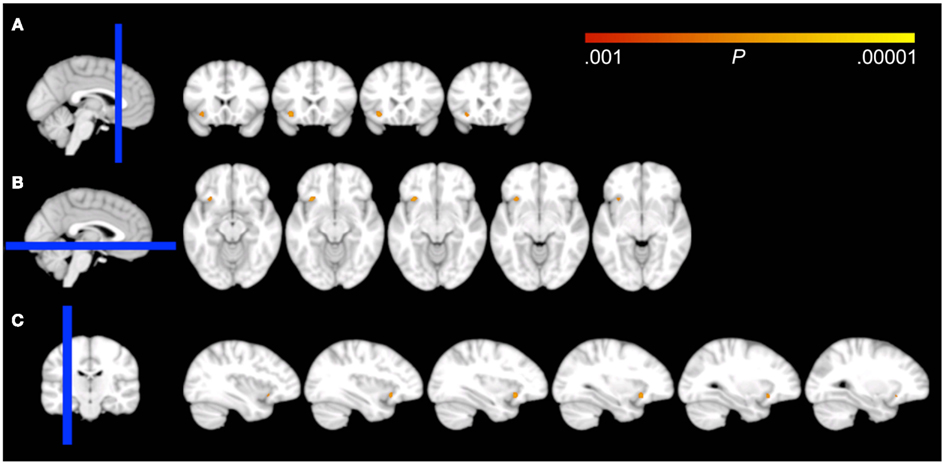
Figure 1. Effects of voxel-based regressions. A cluster of voxels is shown in which GM probability was a significant predictor for the early morning awakening compound Z-score, overlaid on all coronal (A), axial (B), and sagittal (C) 1.5 mm slices that contain the cluster from the DARTEL template, according to neurological convention (left = left). The blue bar in the localizer on the left side of the panels represents the slab of which slices are shown on the right. Note that the cluster overlaps the border of the inferior part of the orbitofrontal cortex and the most anterior aspect of the insular cortex.
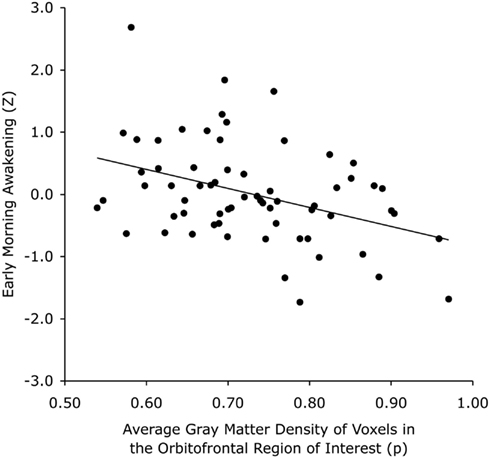
Figure 2. Scatterplot showing that individuals (represented by a dot) with less GM in a cluster compatible with the border of areas 13L and 47/12M of the left orbitofrontal cortex are more likely to experience earlier morning awakening. The solid line represents the linear regression fit.
No effects were observed when testing at the conservative threshold of P < 0.05 family wise error cluster-corrected. At the liberal threshold of P < 0.001 uncorrected, only a single cluster was found outside our ROI for EMA (in the posterior division of the right superior temporal gyrus), which speaks to the specificity of the observed effect in our voxel-wise ROI analysis.
Sleep Complaints
Although Cronbach’s alpha underestimates reliability when computed for a limited number of items, it demonstrated at least a minimally acceptable to even good internal consistency for the compound Z-scores when compared to commonly used standards for larger item pools (Cronbach’s alpha DIS: α = 0.85, DMS: α = 0.69, and EMA: α = 0.59). As to independence of the three compound scores, correlation analysis demonstrated that DIS was relatively independent of both DMS [r(62) = 0.20, P = 0.12] and EMA [r(65) = 0.06, P = 0.66], while DMS and EMA showed a significant correlation [r(62) = 0.52, P < 0.001].
Discussion
We aimed to investigate whether individual differences in GM density in a ROI compatible with the border of Brodmann areas 13L and 47/12M of the left OFC are associated with individual differences in the self-reported degree by which they experienced DIS, DMS, and EMA. This ROI was chosen because previous work from our group showed a strong correlation between GM density in this area and the severity of complaints in people diagnosed with chronic primary insomnia (Altena et al., 2010), thus indicating it to be a neural correlate of insomnia severity. The present study also supports an involvement of this area in sleep characteristics of people that have not been diagnosed with insomnia. Moreover, findings indicate that the association is specific for EMA; it was absent for difficulties initiating and maintaining sleep. This selectivity makes our findings particularly relevant to elderly people; EMA represents the complaint that most characteristically increases with age (Abe and Suzuki, 1985) and prefrontal GM volume is especially vulnerable to age-related decline (Raz et al., 1997, 2005; Good et al., 2001).
The present study has a considerable limitation with respect to the quantification of DIS, DMS, and EMA. We calculated summary Z-scores over relevant items derived from different self-rating questionnaires designed for different purposes, for which data was available in the NESDA study. DIS was computed over four items, while DMS and EMA were both calculated over three items. With very few items, it is unlikely to obtain high alphas unless the items are nearly identical to one another. Considering the small number of items used and their inevitable heterogeneity, even reliabilities above four are often considered reasonable. We can at present only conclude that orbitofrontal GM density shows a significant negative association with earlier awakening in the morning, which may or may not result from different underlying causes and may not be independent of DMS. It would be interesting to investigate, using large item pools, whether DIS, DMS, and EMA are each unidimensional, or rather multidimensional, constructs. Presently, we are not aware of sleep questionnaires that evaluated and validated unidimensional multi-item subscales for DIS, DMS, and EMA.
For the current study, imaging and questionnaire data was available for a sample of individuals spanning a wide age range (18–56 years). As both sleep complaints and brain atrophy increase with age, the observed correlations could in theory merely be due to common age affects. However, both native total GM volume and age were included as confound regressors in all our analyses, thus correcting for intra-individual differences in global brain atrophy as well as age-related changes in local GM volume. Consequently, we are confident that common aging effects cannot explain the observed relation between GM density and EMA.
The orbitofrontal area we focused on in the present study may represent a “hot-spot” for the hedonic evaluation of somatosensory input since it activates with pleasant stimuli (Kringelbach, 2005). It is important to note that there does not appear to be a unidimensional monotonic deactivation of this area from pleasure to aversion; the latter activating a more lateral orbitofrontal area instead (O’Doherty et al., 2001). A recent human brain imaging study elegantly showed that an orbitofrontal region compatible with the border of areas 13L and 47/12M is key to the evaluation of thermal comfort, irrespective of the evaluation of warmth or coolness itself (Dunn et al., 2010). This finding is of interest, because insomniacs have a compromised ability to judge thermal comfort (Raymann and Van Someren, 2008) and even small deviations from comfortable temperature have a strong impact on sleep (Van Someren, 2004, 2006; Raymann et al., 2008). It is tempting to suggest that a low GM volume in the orbitofrontal region at the border of areas 13L and 47/12M could predispose people to suboptimal sensing of comfort. An intact ability to evaluate comfort may be crucial to maintain sleep especially at the end of the night, when sleep is vulnerable because homeostatic sleep propensity has dissipated.
The cluster where the present study found EMA to account for 6% of the interindividual variability in GM density is not limited to the OFC, but extends toward the border of the insula. The insula is an interesting area with respect to the slow oscillations that characterize deep non-REM sleep. High-density sleep-EEG studies have demonstrated that slow oscillations tend to travel over the cortex mostly in an anteroposterior direction (Massimini et al., 2004). Although they can emerge at any part of the cortex, source estimates showed they are most likely to first appear in the insula and then propagate along the anteroposterior axis through the brain midline (Murphy et al., 2009). It is tempting to speculate that a relatively low GM volume in part of the insula may predispose people to a less firm generation of slow oscillations. Slight interindividual differences in ability to ignite slow oscillations may become noticeable only at the end of the night, when sleep is vulnerable because homeostatic sleep propensity has dissipated.
From the perspective of present-day models on sleep regulation, involvement of the OFC and comfort sensing in sleep EMA may be surprising at first glance. The consensus model of sleep-wake regulation consists of a circadian process and a homeostatic process and is aptly referred to as the two-process model of sleep regulation (Borbely, 1982; Daan et al., 1984; Dijk and Czeisler, 1995). The circadian process refers to the timing of sleep and wakefulness driven by the clock of the brain, located in the hypothalamic suprachiasmatic nucleus (Mistlberger, 2005). The homeostatic component refers to the hourglass of the brain, that keeps track of the time we are awake and gradually increases the pressure for sleep. This pressure dissipates as soon as sleep sets in. The decrease follows an exponential trajectory, so that most of the sleep pressure is dissipated within a few hours of sleep (Achermann et al., 1993; Leemburg et al., 2010; Van Someren, 2010). The neurobiological mechanisms underlying this hourglass mechanism are not as well characterized as the mechanisms of the clock, but are likely to involve adenosine (Porkka-Heiskanen et al., 1997), cytokines (Krueger et al., 2011), and an increase in synaptic density during wakefulness (Tononi and Cirelli, 2006).
Recently, it has been argued that the two-process model of sleep regulation falls short of recognizing that the ability to sleep also crucially depends on whether sleep-permissive conditions are met (Romeijn et al., 2012). Trivial as it may seem, one is unlikely to sleep without feeling comfortable – one of the sleep-permissive conditions – unless the circadian and homeostatic sleep drives are so high that they overrule the obstacle of being uncomfortable. Along this line of reasoning, a hypothesis can be forwarded on why a low GM density in an area that is essential for comfort sensing would be associated with EMA, but not with difficulties initiating or maintaining sleep. The circadian and homeostatic sleep processes concertedly provide a very strong drive to sleep during the initial part of the night, enforcing sleep even if sleep-permissive conditions, like comfort, are suboptimal. During the final part of the night, however, the homeostatic sleep pressure has dissipated virtually completely and the circadian clock is ready to switch to the promotion of wakefulness. It is in this final part of the night that one can presume suboptimal comfort signaling to interfere most strongly with the continuation of sleep. Whatever the experienced impact and reason of EMA – and subjects could well differ here –, it may require optimal comfort sensing and signaling by the OFC to continue sleep after the arousals that normally occur more frequently in the morning. This situation may be compared to someone being able to sleep in an uncomfortable upright sitting position when exhausted, while it would require the comfort of lying supine on a bed to catch any sleep if sleep propensity is low. Although admittedly highly speculative, this line of reasoning is amenable to hypothesis-driven experimental studies, into the possible involvement of OFC, insula, comfort sensing, and slow wave generation in sleep complaints. Innovative hypothesis-driven studies are direly needed to advance our understanding and treatment of insomnia; still one of the least understood disorders that chronically impacts the lives of so many, especially in the elderly population.
Conflict of Interest Statement
The authors declare that the research was conducted in the absence of any commercial or financial relationships that could be construed as a potential conflict of interest.
Acknowledgments
The authors thank Prof. Dr. André Aleman for kindly providing MRI data from controls scanned at the UMCG. This work was supported by the Netherlands Organization for Scientific Research (NWO), The Hague, The Netherlands (VICI innovation grant number 453-07-001) and by the FP7-PEOPLE-ITN-2008 Marie Curie Actions Networks for Initial Training (ITN) funding scheme, grant number 238665, project Neuroendocrine Immune Networks in Ageing (NINA). The infrastructure for the Netherlands Study of Depression and Anxiety study (http://www.nesda.nl/nl/) was supported by the Geestkracht program of the Netherlands Organization for Health Research and Development (Health Care Research the Netherlands, Medical Science, grant No. 10-000-1002), VU University Medical Center, Geestelijke GezondheidsZorg (GGS) in Geest, Arkin, Leiden University Medical Center, GGZ Rivierduinen, University Medical Center Groningen, Lentis, GGZ Friesland, GGZ Drenthe, Scientific Institute for Quality of Healthcare, Netherlands Institute for Health Services Research and Netherlands Institute of Mental Health and Addiction (Trimbos Institute).
Abbreviations
DIS, difficulties initiating sleep; DMS, difficulties maintaining sleep; EMA, early morning awakening; GM, gray matter; MRI, magnetic resonance imaging; OFC, orbitofrontal cortex; ROI, region of interest; VBM, voxel-based morphometry.
References
Abe, K., and Suzuki, T. (1985). Age trends of early awakening and feeling worse in the morning than in the evening in apparently normal people. J. Nerv. Ment. Dis. 173, 495–498.
Achermann, P., Dijk, D. J., Brunner, D. P., and Borbely, A. A. (1993). A model of human sleep homeostasis based on EEG slow-wave activity: quantitative comparison of data and simulations. Brain Res. Bull. 31, 97–113.
Altena, E., Vrenken, H., Van Der Werf, Y. D., van den Heuvel, O. A., and Van Someren, E. J. (2010). Reduced orbitofrontal and parietal gray matter in chronic insomnia: a voxel-based morphometric study. Biol. Psychiatry 67, 182–185.
American Academy of Sleep Medicine. (2005). ICSD2 – International Classification of Sleep Disorders: Diagnostic and Coding Manual. Rochester, MN: American Sleep Disorders Association.
American Psychiatric Association. (1994). Diagnostic and Statistical Manual of Mental Disorders DSM-IV. Washington, DC: American Psychiatric Association.
Ashburner, J., and Friston, K. J. (2000). Voxel-based morphometry – the methods. Neuroimage 11, 805–821.
Beaulieu-Bonneau, S., LeBlanc, M., Merette, C., Dauvilliers, Y., and Morin, C. M. (2007). Family history of insomnia in a population-based sample. Sleep 30, 1739–1745.
Boomsma, D. I., van Someren, E. J., Beem, A. L., de Geus, E. J., and Willemsen, G. (2008). Sleep during a regular week night: a twin-sibling study. Twin Res. Hum. Genet. 11, 538–545.
Daan, S., Beersma, D. G., and Borbely, A. A. (1984). Timing of human sleep: recovery process gated by a circadian pacemaker. Am. J. Physiol. 246, R161–R183.
Dijk, D. J., and Czeisler, C. A. (1995). Contribution of the circadian pacemaker and the sleep homeostat to sleep propensity, sleep structure, electroencephalographic slow waves, and sleep spindle activity in humans. J. Neurosci. 15, 3526–3538.
Dunn, B. J., Conover, K., Plourde, G., Munro, D., Kilgour, R., and Shizgal, P. (2010). “Hedonic valuation during thermal alliesthesia.” in Abstracts of the 16th Annual Meeting of the Organization for Human Brain Mapping, Minneapolis.
Fernandez-Mendoza, J., Vela-Bueno, A., Vgontzas, A. N., Ramos-Platon, M. J., Olavarrieta-Bernardino, S., Bixler, E. O., and De la Cruz-Troca, J. J. (2010). Cognitive-emotional hyperarousal as a premorbid characteristic of individuals vulnerable to insomnia. Psychosom. Med. 72, 397–403.
Foley, D. J., Monjan, A. A., Brown, S. L., Simonsick, E. M., Wallace, R. B., and Blazer, D. G. (1995). Sleep complaints among elderly persons: an epidemiologic study of three communities. Sleep 18, 425–432.
Friston, K. J., Holmes, A. P., Poline, J. B., Grasby, P. J., Williams, S. C., Frackowiak, R. S., and Turner, R. (1995). Analysis of fMRI time-series revisited. Neuroimage 2, 45–53.
Good, C. D., Johnsrude, I. S., Ashburner, J., Henson, R. N., Friston, K. J., and Frackowiak, R. S. (2001). A voxel-based morphometric study of ageing in 465 normal adult human brains. Neuroimage 14, 21–36.
Kringelbach, M. L. (2005). The human orbitofrontal cortex: linking reward to hedonic experience. Nat. Rev. Neurosci. 6, 691–702.
Krueger, J. M., Clinton, J. M., Winters, B. D., Zielinski, M. R., Taishi, P., Jewett, K. A., and Davis, C. J. (2011). Involvement of cytokines in slow wave sleep. Prog. Brain Res. 193, 39–47.
Leemburg, S., Vyazovskiy, V. V., Olcese, U., Bassetti, C. L., Tononi, G., and Cirelli, C. (2010). Sleep homeostasis in the rat is preserved during chronic sleep restriction. Proc. Natl. Acad. Sci. U.S.A. 107, 15939–15944.
Levine, D. W., Kaplan, R. M., Kripke, D. F., Bowen, D. J., Naughton, M. J., and Shumaker, S. A. (2003a). Factor structure and measurement invariance of the Women’s Health Initiative Insomnia Rating Scale. Psychol. Assess. 15, 123–136.
Levine, D. W., Kripke, D. F., Kaplan, R. M., Lewis, M. A., Naughton, M. J., Bowen, D. J., and Shumaker, S. A. (2003b). Reliability and validity of the Women’s Health Initiative Insomnia Rating Scale. Psychol. Assess. 15, 137–148.
Maldjian, J. A., Laurienti, P. J., and Burdette, J. H. (2004). Precentral gyrus discrepancy in electronic versions of the Talairach atlas. Neuroimage 21, 450–455.
Maldjian, J. A., Laurienti, P. J., Kraft, R. A., and Burdette, J. H. (2003). An automated method for neuroanatomic and cytoarchitectonic atlas-based interrogation of fMRI data sets. Neuroimage 19, 1233–1239.
Massimini, M., Huber, R., Ferrarelli, F., Hill, S., and Tononi, G. (2004). The sleep slow oscillation as a traveling wave. J. Neurosci. 24, 6862–6870.
Mistlberger, R. E. (2005). Circadian regulation of sleep in mammals: role of the suprachiasmatic nucleus. Brain Res. Brain Res. Rev. 49, 429–454.
Morphy, H., Dunn, K. M., Lewis, M., Boardman, H. F., and Croft, P. R. (2007). Epidemiology of insomnia: a longitudinal study in a UK population. Sleep 30, 274–280.
Murphy, M., Riedner, B. A., Huber, R., Massimini, M., Ferrarelli, F., and Tononi, G. (2009). Source modeling sleep slow waves. Proc. Natl. Acad. Sci. U.S.A. 106, 1608–1613.
National Sleep Foundation. (2003a). 2003 Sleep in America Poll: Adult Sleep Habits. Available at: http://www.sleepfoundation.org
National Sleep Foundation. (2003b). 2003 Sleep in America Poll: Sleep and Aging. Available at: http://www.sleepfoundation.org
O’Doherty, J., Rolls, E. T., Francis, S., Bowtell, R., and McGlone, F. (2001). Representation of pleasant and aversive taste in the human brain. J. Neurophysiol. 85, 1315–1321.
Ohayon, M. M. (2002). Epidemiology of insomnia: what we know and what we still need to learn. Sleep Med. Rev. 6, 97–111.
Ongur, D., Ferry, A. T., and Price, J. L. (2003). Architectonic subdivision of the human orbital and medial prefrontal cortex. J. Comp. Neurol. 460, 425–449.
Penninx, B. W., Beekman, A. T., Smit, J. H., Zitman, F. G., Nolen, W. A., Spinhoven, P., Cuijpers, P., De Jong, P. J., Van Marwijk, H. W., Assendelft, W. J., Van Der Meer, K., Verhaak, P., Wensing, M., De Graaf, R., Hoogendijk, W. J., Ormel, J., Van Dyck, R., and Consortium, N. R. (2008). The Netherlands Study of Depression and Anxiety (NESDA): rationale, objectives and methods. Int. J. Methods Psychiatr. Res. 17, 121–140.
Perlis, M. L., Giles, D. E., Mendelson, W. B., Bootzin, R. R., and Wyatt, J. K. (1997). Psychophysiological insomnia: the behavioural model and a neurocognitive perspective. J. Sleep Res. 6, 179–188.
Porkka-Heiskanen, T., Strecker, R. E., Thakkar, M., Bjorkum, A. A., Greene, R. W., and McCarley, R. W. (1997). Adenosine: a mediator of the sleep-inducing effects of prolonged wakefulness. Science 276, 1265–1268.
Raymann, R. J., and Van Someren, E. J. (2008). Diminished capability to recognize the optimal temperature for sleep initiation may contribute to poor sleep in elderly people. Sleep 31, 1301–1309.
Raymann, R. J. E. M., Swaab, D. F., and Van Someren, E. J. W. (2008). Skin deep: cutaneous temperature determines sleep depth. Brain 131, 500–513.
Raz, N., Gunning, F. M., Head, D., Dupuis, J. H., McQuain, J., Briggs, S. D., Loken, W. J., Thornton, A. E., and Acker, J. D. (1997). Selective aging of the human cerebral cortex observed in vivo: differential vulnerability of the prefrontal gray matter. Cereb. Cortex 7, 268–282.
Raz, N., Lindenberger, U., Rodrigue, K. M., Kennedy, K. M., Head, D., Williamson, A., Dahle, C., Gerstorf, D., and Acker, J. D. (2005). Regional brain changes in aging healthy adults: general trends, individual differences and modifiers. Cereb. Cortex 15, 1676–1689.
Ridgway, G. R., Omar, R., Ourselin, S., Hill, D. L., Warren, J. D., and Fox, N. C. (2009). Issues with threshold masking in voxel-based morphometry of atrophied brains. Neuroimage 44, 99–111.
Riemann, D., Spiegelhalder, K., Feige, B., Voderholzer, U., Berger, M., Perlis, M., and Nissen, C. (2010). The hyperarousal model of insomnia: a review of the concept and its evidence. Sleep Med. Rev. 14, 19–31.
Robins, L. N., Wing, J., Wittchen, H. U., Helzer, J. E., Babor, T. F., Burke, J., Farmer, A., Jablenski, A., Pickens, R., Regier, D. A., Sartorius, N., and Towle, L. H. (1988). The Composite International Diagnostic Interview. An epidemiologic Instrument suitable for use in conjunction with different diagnostic systems and in different cultures. Arch. Gen. Psychiatry 45, 1069–1077.
Roenneberg, T., Kuehnle, T., Pramstaller, P. P., Ricken, J., Havel, M., Guth, A., and Merrow, M. (2004). A marker for the end of adolescence. Curr. Biol. 14, R1038–R1039.
Romeijn, N., Raymann, R. J., Most, E., Te Lindert, B., Van Der Meijden, W. P., Fronczek, R., Gomez-Herrero, G., and Van Someren, E. J. (2012). Sleep, vigilance, and thermosensitivity. Pflugers Arch. 463, 169–176.
Talairach, J., and Tournoux, P. (1988). Co-Planar Stereotaxic Atlas of the Human Brain: 3-Dimensional Proportional System: An Approach to Cerebral Imaging. New York, NY: Thieme Medical Publishers.
Thompson, P. M., Cannon, T. D., Narr, K. L., van Erp, T., Poutanen, V. P., Huttunen, M., Lonnqvist, J., Standertskjold-Nordenstam, C. G., Kaprio, J., Khaledy, M., Dail, R., Zoumalan, C. I., and Toga, A. W. (2001). Genetic influences on brain structure. Nat. Neurosci. 4, 1253–1258.
Tononi, G., and Cirelli, C. (2006). Sleep function and synaptic homeostasis. Sleep Med. Rev. 10, 49–62.
Van Someren, E. J. (2010). Doing with less sleep remains a dream. Proc. Natl. Acad. Sci. U.S.A. 107, 16003–16004.
Van Someren, E. J. W. (2004). Sleep propensity is modulated by circadian and behavior-induced changes in cutaneous temperature. J. Therm. Biol. 29, 437–444.
Van Someren, E. J. W. (2006). Mechanisms and functions of coupling between sleep and temperature rhythms. Prog. Brain Res. 153, 309–324.
van Tol, M. J., van der Wee, N. J., van den Heuvel, O. A., Nielen, M. M., Demenescu, L. R., Aleman, A., Renken, R., van Buchem, M. A., Zitman, F. G., and Veltman, D. J. (2010). Regional brain volume in depression and anxiety disorders. Arch. Gen. Psychiatry 67, 1002–1011.
Yassa, M. A., and Stark, C. E. (2009). A quantitative evaluation of cross-participant registration techniques for MRI studies of the medial temporal lobe. Neuroimage 44, 319–327.
Keywords: insomnia, aging, early morning awakening, orbitofrontal cortex, voxel-based morphometry, structural magnetic resonance imaging
Citation: Stoffers D, Moens S, Benjamins J, van Tol M-J, Penninx BWJH, Veltman DJ, Van der Wee NJA and Van Someren EJW (2012) Orbitofrontal gray matter relates to early morning awakening: a neural correlate of insomnia complaints? Front. Neur. 3:105. doi: 10.3389/fneur.2012.00105
Received: 08 March 2012; Paper pending published: 13 April 2012;
Accepted: 12 June 2012; Published online: 28 June 2012.
Edited by:
Julie Carrier, Université de Montréal, CanadaReviewed by:
Renate Wehrle, University Clinic Regensburg, GermanyJessica Massicotte-Marquez, Université de Montréal, Canada
Copyright: © 2012 Stoffers, Moens, Benjamins, van Tol, Penninx, Veltman, Van der Wee and Van Someren. This is an open-access article distributed under the terms of the Creative Commons Attribution Non Commercial License, which permits non-commercial use, distribution, and reproduction in other forums, provided the original authors and source are credited.
*Correspondence: Eus J. W. Van Someren, Department of Sleep and Cognition, Netherlands Institute for Neuroscience, an Institute of the Royal Netherlands Academy of Arts and Sciences, Meibergdreef 47, 1105 BA Amsterdam, Netherlands. e-mail: e.van.someren@nin.knaw.nl