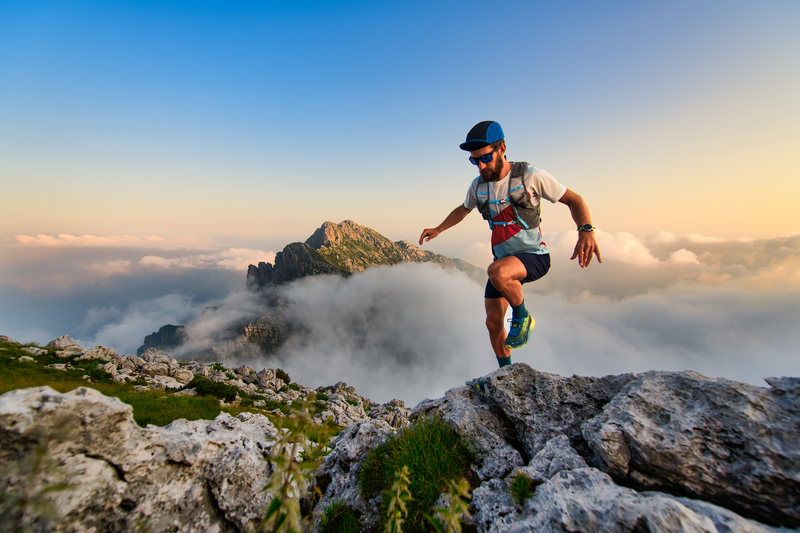
94% of researchers rate our articles as excellent or good
Learn more about the work of our research integrity team to safeguard the quality of each article we publish.
Find out more
REVIEW article
Front. Neuroinform.
Volume 19 - 2025 | doi: 10.3389/fninf.2025.1557177
The final, formatted version of the article will be published soon.
You have multiple emails registered with Frontiers:
Please enter your email address:
If you already have an account, please login
You don't have a Frontiers account ? You can register here
Introduction:Alzheimer's disease is a progressive neurodegenerative disorder challenging early diagnosis and treatment. Recent advancements in deep learning algorithms applied to multimodal brain imaging offer promising solutions for improving diagnostic accuracy and predicting disease progression. Method:This narrative review synthesizes current literature on deep learning applications in Alzheimer's disease diagnosis using multimodal neuroimaging. The review process involved a comprehensive search of relevant databases (PubMed, Embase, Google Scholar and ClinicalTrials.gov), selection of pertinent studies, and critical analysis of findings. We employed a best-evidence approach, prioritizing high-quality studies and identifying consistent patterns across the literature. Results:Deep learning architectures, including convolutional neural networks, recurrent neural networks, and transformer-based models, have shown remarkable potential in analyzing multimodal neuroimaging data. These models can effectively process structural and functional imaging modalities, extracting relevant features and patterns associated with Alzheimer's pathology. Integration of multiple imaging modalities has demonstrated improved diagnostic accuracy compared to single-modality approaches. Deep learning models have also shown promise in predictive modeling, identifying potential biomarkers and forecasting disease progression. Discussion:While deep learning approaches show great potential, several challenges remain. Data heterogeneity, small sample sizes, and limited generalizability across diverse populations are significant hurdles. The clinical translation of these models requires careful consideration of interpretability, transparency, and ethical implications. The future of AI in neurodiagnostics for Alzheimer's disease looks promising, with potential applications in personalized treatment strategies.
Keywords: Alzheimer's disease, deep learning, multimodal neuroimaging, Disease progression prediction, brain imaging Analysis
Received: 08 Jan 2025; Accepted: 17 Mar 2025.
Copyright: © 2025 Raza, Hassan, Jamil, Hyder, Batool, Walji and Abbas. This is an open-access article distributed under the terms of the Creative Commons Attribution License (CC BY). The use, distribution or reproduction in other forums is permitted, provided the original author(s) or licensor are credited and that the original publication in this journal is cited, in accordance with accepted academic practice. No use, distribution or reproduction is permitted which does not comply with these terms.
* Correspondence:
Muhammad Liaquat Raza, King Abdulaziz Medical City, Riyadh, 14611, Saudi Arabia
Disclaimer: All claims expressed in this article are solely those of the authors and do not necessarily represent those of their affiliated organizations, or those of the publisher, the editors and the reviewers. Any product that may be evaluated in this article or claim that may be made by its manufacturer is not guaranteed or endorsed by the publisher.
Research integrity at Frontiers
Learn more about the work of our research integrity team to safeguard the quality of each article we publish.