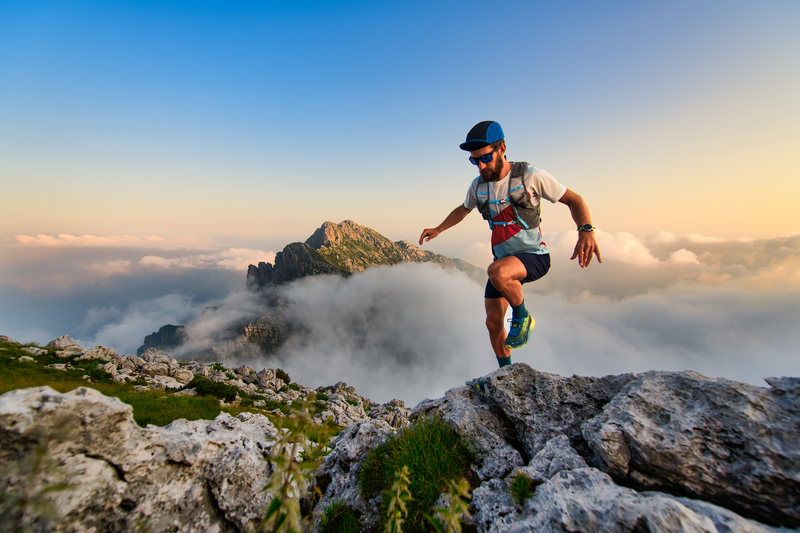
95% of researchers rate our articles as excellent or good
Learn more about the work of our research integrity team to safeguard the quality of each article we publish.
Find out more
EDITORIAL article
Front. Neuroinform. , 16 January 2025
Volume 19 - 2025 | https://doi.org/10.3389/fninf.2025.1543103
This article is part of the Research Topic Recent Applications of Noninvasive Physiological Signals and Artificial Intelligence View all 7 articles
Editorial on the Research Topic
Recent applications of noninvasive physiological signals and artificial intelligence
Artificial intelligence (AI) is currently transforming diverse fields (Qasmi and Fatima, 2024; Alyabroodi et al., 2023), by enabling the personalization of the user experience or expected outcomes, by monitoring and detecting pathological conditions, among other benefits. The use of non-invasive biomedical signals can enhance the performance of AI applications by providing complimentary objective information about the traits of a person that can be otherwise difficult to evaluate or by delivering physiological information that can contribute to the advancement of biomedical signal processing to improve medical attention (Tseng et al., 2023; Kumar et al., 2024).
Several applications of AI on non-invasive physiological signals for neuroscience are explored in this collection. In the pioneer field of neuro-humanities, Blanco-Ríos et al. propose a real-time system for emotion recognition using EEG signals and Extra-Trees, which achieved a very high accuracy with the goal of enhancing learning experience in the field of humanities. Health applications are also explored, such as in Fernandez Rojas et al. where the accuracy of deep learning models is compared with functional near-infrared spectroscopy (fNIRS) and baseline models for the assessment of pain. The best model (CNN-LSTM) could be used as a possible method for effective pain assessment, which could lead to a more precise tool for clinicians for the care for patients with communication limitations.
Other novel studies in this collection are focused on the research of brain-computer interfaces (BCI). Juan et al. combined spectro-temporal and spectro-spatial feature extraction methods and deep learning models (CNN) to enhance the decoding accuracy of motor imagery from EEG signals during pedaling tasks, obtaining an accuracy of up to 80% despite higher instability. In a similar topic, Dillen et al. evaluated the usability of a BCI that relied on motor imagery detection and augmented reality for different motor tasks. This was achieved through an assessing protocol that consisted in validating technical robustness, evaluating the control system and comparing it with a non-BCI alternative, including user evaluations. These contributions are important for assuring BCIs are practical and effective in different scenarios.
Regarding wearable devices, biomedical signals need to be compressed and reconstructed for their transmission while reducing noise. Zhang et al. present an improvement in electrocardiographic (ECG) signal reconstruction based on weighted nuclear norm minimization (WNNM) and denoising-based approximate message passing algorithms (AMP). Within the same topic of signal quality, Cisotto et al. present an innovative deep learning model called hvEEGNet, which is based on a hierarchical variational autoencoder and trained with a new loss function. It is designed for the reconstruction of multi-channel EEG signals. Unlike previous works, the model is capable of performing high-fidelity reconstruction of multi-channel EEG datasets, with high consistency across subjects.
The presented articles in this Research Topic provide insights into the recent applications of artificial intelligence and biomedical signals, mainly with a neuroscience perspective. These studies show some of the future trends that may be developed to improve personal experience and accuracy, especially in the healthcare services.
IA: Writing – original draft, Writing – review & editing. EI: Writing – original draft, Writing – review & editing. AU: Writing – original draft, Writing – review & editing.
The authors declare that the research was conducted in the absence of any commercial or financial relationships that could be construed as a potential conflict of interest.
All claims expressed in this article are solely those of the authors and do not necessarily represent those of their affiliated organizations, or those of the publisher, the editors and the reviewers. Any product that may be evaluated in this article, or claim that may be made by its manufacturer, is not guaranteed or endorsed by the publisher.
Alyabroodi, Z., Abuasal, S., Alamareen, A. A. B., Al-mashagbeh, M. H., and Hakami, H. (2023). The impact of artificial intelligence on healthcare area—a systematic review. Artif. Intell. Internet Things Soc. 5.0, 485–494. doi: 10.1007/978-3-031-43300-9_40
Kumar, A., Koch, N., Imran, S., and Yadav, J. (2024). “Artificial intelligence and machine learning in biomedical signal processing,” in Evolution of Machine Learning and Internet of Things Applications in Biomedical Engineering, eds. A. K. Rana, V. Sharma, and S. K. Rana (Boca Raton, FL: CRC Press), 145–168. doi: 10.1201/9781003476207-10
Qasmi, F., and Fatima, S. K. (2024). “Enhancing education and well-being through artificial intelligence: opportunities and challenges,” in International Conference on Breaking Barriers with Generative Intelligence (Cham: Springer), 154–164. doi: 10.1007/978-3-031-65996-6_13
Keywords: biomedical signal processing, machine learning, brain-computer interface, affective computer, user experience
Citation: Angulo IN, Iáñez E and Ubeda A (2025) Editorial: Recent applications of noninvasive physiological signals and artificial intelligence. Front. Neuroinform. 19:1543103. doi: 10.3389/fninf.2025.1543103
Received: 10 December 2024; Accepted: 06 January 2025;
Published: 16 January 2025.
Edited and reviewed by: Michael Denker, Jülich Research Centre, Germany
Copyright © 2025 Angulo, Iáñez and Ubeda. This is an open-access article distributed under the terms of the Creative Commons Attribution License (CC BY). The use, distribution or reproduction in other forums is permitted, provided the original author(s) and the copyright owner(s) are credited and that the original publication in this journal is cited, in accordance with accepted academic practice. No use, distribution or reproduction is permitted which does not comply with these terms.
*Correspondence: Andres Ubeda, YW5kcmVzLnViZWRhQHVhLmVz
Disclaimer: All claims expressed in this article are solely those of the authors and do not necessarily represent those of their affiliated organizations, or those of the publisher, the editors and the reviewers. Any product that may be evaluated in this article or claim that may be made by its manufacturer is not guaranteed or endorsed by the publisher.
Research integrity at Frontiers
Learn more about the work of our research integrity team to safeguard the quality of each article we publish.