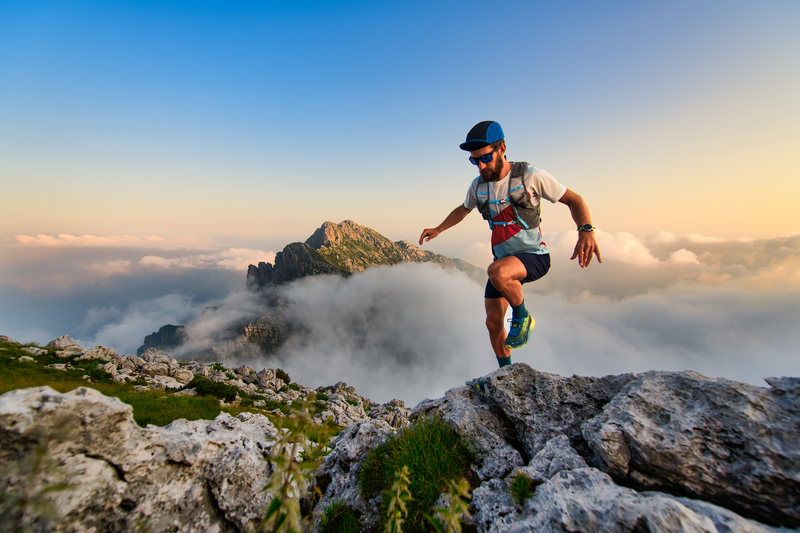
94% of researchers rate our articles as excellent or good
Learn more about the work of our research integrity team to safeguard the quality of each article we publish.
Find out more
ORIGINAL RESEARCH article
Front. Neuroinform.
Volume 19 - 2025 | doi: 10.3389/fninf.2025.1530445
This article is part of the Research Topic Open and FAIR Data in Neuroscience View all articles
The final, formatted version of the article will be published soon.
You have multiple emails registered with Frontiers:
Please enter your email address:
If you already have an account, please login
You don't have a Frontiers account ? You can register here
The effectiveness of research and innovation often relies on the diversity or heterogeneity of datasets that are Findable, Accessible, Interoperable and Reusable (FAIR). However, the global landscape of brain data is yet to achieve desired levels of diversity that can facilitate generalisable outputs. Brain datasets from low-and middle-income countries of Africa are still missing in the global open science ecosystem. This can mean that decades of brain research and innovation may not be generalisable to populations in Africa.This research combined experiential learning or experiential research with a survey questionnaire. The experiential research involved deriving insights from direct, hands-on experiences of collecting African Brain data in view of making it FAIR. This was a critical process of action, reflection, and learning from doing data collection. A questionnaire was then used to validate the findings from the experiential research and provide wider contexts for these findings.The experiential research revealed major challenges to FAIR African brain data that can be categorised as socio-cultural, economic, technical, ethical and legal challenges. It also highlighted opportunities for growth that include capacity development, development of technical infrastructure, funding as well as policy and regulatory changes. The questionnaire then showed that the wider African neuroscience community believes that these challenges can be ranked in order of priority as follows: Technical, economic, socio-cultural and ethical and legal challenges.We conclude that African researchers need to work together as a community to address these challenges in a way to maximise efforts and to build a thriving FAIR brain data ecosystem that is socially acceptable, ethically responsible, technically robust and legally compliant.
Keywords: FAIR, African Neuroscience, Brain data, Neuroimaging, neuroinformatics
Received: 18 Nov 2024; Accepted: 12 Feb 2025.
Copyright: © 2025 Wogu, Ogoh, Filima, Nsaanee, Caron, Pestilli and Eke. This is an open-access article distributed under the terms of the Creative Commons Attribution License (CC BY). The use, distribution or reproduction in other forums is permitted, provided the original author(s) or licensor are credited and that the original publication in this journal is cited, in accordance with accepted academic practice. No use, distribution or reproduction is permitted which does not comply with these terms.
* Correspondence:
Damian Eke, University of Nottingham, Nottingham, United Kingdom
Disclaimer: All claims expressed in this article are solely those of the authors and do not necessarily represent those of their affiliated organizations, or those of the publisher, the editors and the reviewers. Any product that may be evaluated in this article or claim that may be made by its manufacturer is not guaranteed or endorsed by the publisher.
Research integrity at Frontiers
Learn more about the work of our research integrity team to safeguard the quality of each article we publish.