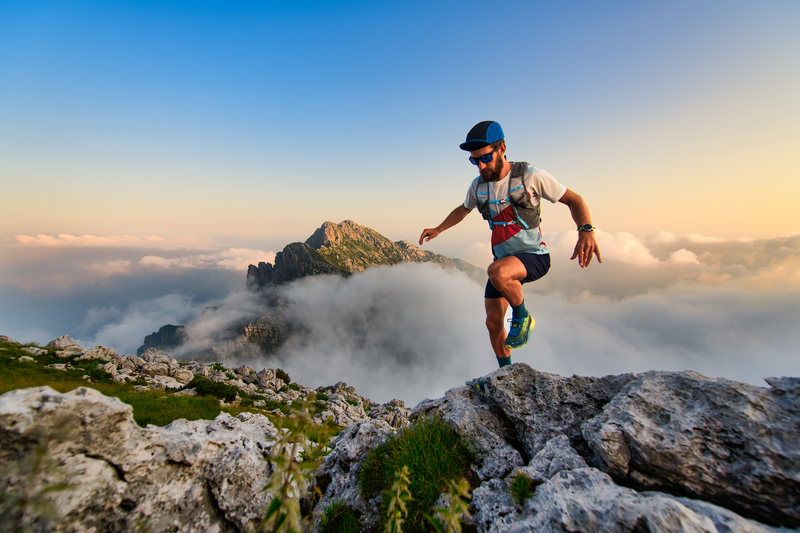
94% of researchers rate our articles as excellent or good
Learn more about the work of our research integrity team to safeguard the quality of each article we publish.
Find out more
ORIGINAL RESEARCH article
Front. Neuroinform.
Volume 19 - 2025 | doi: 10.3389/fninf.2025.1521805
This article is part of the Research Topic Advanced EEG Analysis Techniques for Neurological Disorders View all 6 articles
The final, formatted version of the article will be published soon.
You have multiple emails registered with Frontiers:
Please enter your email address:
If you already have an account, please login
You don't have a Frontiers account ? You can register here
Analysis of electroencephalography (EEG) signals gathered by brain-computer interface (BCI) recently demonstrated that deep neural networks (DNNs) can be effectively used for investigation of time sequences for physical actions (PA) classification. In this work the relatively simple DNN with fully-connected network (FCN) components and convolutional neural network (CNN) components was considered to classify finger-palm-hand manipulations each from the graspand-lift (GAL) dataset. The main aim of this work was to imitate and investigate environmental influence by the proposed noise data augmentation (NDA) of two kinds: (i) natural NDA by inclusion of noise EEG data from neighboring regions by increasing the sampling size N and the different offset values for sample labeling, (ii) synthetic NDA by adding the generated Gaussian noise. The natural NDA by increasing N leads to the higher micro and macro area under the curve (AUC) for receiver operating curve values for the bigger N values than usage of synthetic NDA.The detrended fluctuation analysis (DFA) was applied to investigate the fluctuation properties and calculate the correspondent Hurst exponents H for the quantitative characterization of the fluctuation variability. H values for the low time window scales (< 2 seconds) are higher in comparison to ones for the bigger time window scales. For example, H more than 2-3 times higher for some PAs, i.e. it means that the shorter EEG fragments (< 2 seconds) demonstrate the scaling behavior of the higher complexity than the longer fragments. As far as these results were obtained by the relatively small DNN with the low resource requirements, this approach can be promising for porting such models to Edge Computing infrastructures on devices with the very limited computational resources.
Keywords: Deep neural network, Brain-computer interface, Grasp-and-lift, Physical action, Data augmentation, Noise, noise data augmentation, detrended fluctuation analysis
Received: 02 Nov 2024; Accepted: 13 Feb 2025.
Copyright: © 2025 Gordienko, Gordienko, Taran, Rojbi, Telenyk and Stirenko. This is an open-access article distributed under the terms of the Creative Commons Attribution License (CC BY). The use, distribution or reproduction in other forums is permitted, provided the original author(s) or licensor are credited and that the original publication in this journal is cited, in accordance with accepted academic practice. No use, distribution or reproduction is permitted which does not comply with these terms.
* Correspondence:
Yuri Gordienko, National Technical University of Ukraine “Igor Sikorsky Kyiv Polytechnic Institute”, Kyiv, Ukraine
Nikita Gordienko, National Technical University of Ukraine “Igor Sikorsky Kyiv Polytechnic Institute”, Kyiv, Ukraine
Vladyslav Taran, National Technical University of Ukraine “Igor Sikorsky Kyiv Polytechnic Institute”, Kyiv, Ukraine
Sergii Telenyk, Cracow University of Technology, Kraków, 31155, Lesser Poland, Poland
Sergii Stirenko, National Technical University of Ukraine “Igor Sikorsky Kyiv Polytechnic Institute”, Kyiv, Ukraine
Disclaimer: All claims expressed in this article are solely those of the authors and do not necessarily represent those of their affiliated organizations, or those of the publisher, the editors and the reviewers. Any product that may be evaluated in this article or claim that may be made by its manufacturer is not guaranteed or endorsed by the publisher.
Research integrity at Frontiers
Learn more about the work of our research integrity team to safeguard the quality of each article we publish.