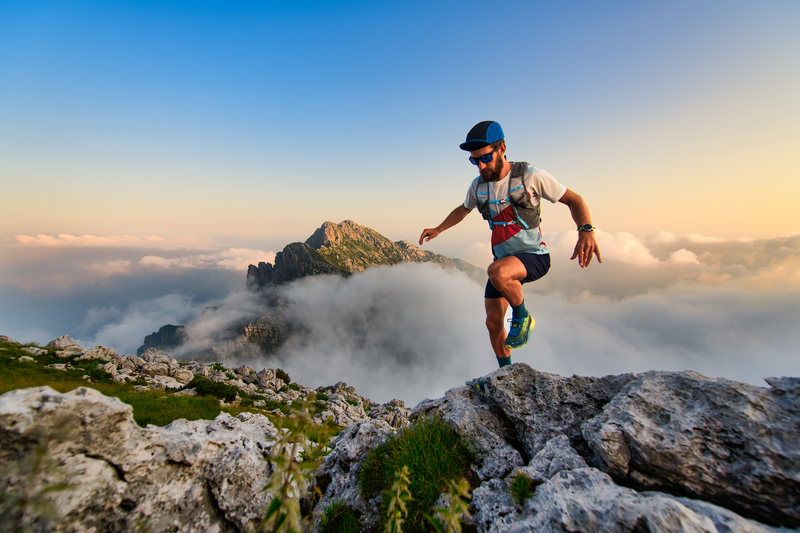
95% of researchers rate our articles as excellent or good
Learn more about the work of our research integrity team to safeguard the quality of each article we publish.
Find out more
TECHNOLOGY AND CODE article
Front. Neuroinform.
Volume 19 - 2025 | doi: 10.3389/fninf.2025.1515873
This article is part of the Research Topic Clinical Neuroinformatics: Advancing Integration and Analysis in Diagnostic Neuroscience View all 3 articles
The final, formatted version of the article will be published soon.
You have multiple emails registered with Frontiers:
Please enter your email address:
If you already have an account, please login
You don't have a Frontiers account ? You can register here
In cognitive neuroscience, the integration of deep neural networks (DNNs) with traditional neuroscientific analyses has significantly advanced our understanding of both biological neural processes and the functioning of DNNs. However, challenges remain in effectively comparing the representational spaces of artificial models and brain data, particularly due to the growing variety of models and the specific demands of neuroimaging research. To address these challenges, we present Net2Brain, a Python-based toolbox that provides an end-to-end pipeline for incorporating DNNs into neuroscience research, encompassing dataset download, a large selection of models, feature extraction, evaluation, and visualization. Net2Brain provides functionalities in four key areas. First, it offers access to over 600 DNNs trained on diverse tasks across multiple modalities, including vision, language, audio, and multimodal data, organized through a carefully structured taxonomy. Second, it provides a streamlined API for downloading and handling popular neuroscience datasets, such as the NSD and THINGS dataset, allowing researchers to easily access corresponding brain data. Third, Net2Brain facilitates a wide range of analysis options, including feature extraction, representational similarity analysis (RSA), and linear encoding, while also supporting advanced techniques like variance partitioning and searchlight analysis. Finally, the toolbox integrates seamlessly with other established open source libraries, enhancing interoperability and promoting collaborative research. By simplifying model selection, data processing, and evaluation, Net2Brain empowers researchers to conduct more robust, flexible, and reproducible investigations of the relationships between artificial and biological neural representations.
Keywords: Cognitive neuroscience, deep neural networks, Neuroimaging Data Analysis, Artificial Intelligence in Neuroscience, Toolbox, Multimodal Neural Models
Received: 23 Oct 2024; Accepted: 07 Apr 2025.
Copyright: © 2025 Bersch, Vilas, Saba-Sadiya, Schaumlöffel, Dwivedi, Sartzetaki, Cichy and Roig. This is an open-access article distributed under the terms of the Creative Commons Attribution License (CC BY). The use, distribution or reproduction in other forums is permitted, provided the original author(s) or licensor are credited and that the original publication in this journal is cited, in accordance with accepted academic practice. No use, distribution or reproduction is permitted which does not comply with these terms.
* Correspondence:
Domenic Bersch, Department of Computer Science, Goethe University, Frankfurt, Hesse, Germany
Disclaimer: All claims expressed in this article are solely those of the authors and do not necessarily represent those of their affiliated organizations, or those of the publisher, the editors and the reviewers. Any product that may be evaluated in this article or claim that may be made by its manufacturer is not guaranteed or endorsed by the publisher.
Research integrity at Frontiers
Learn more about the work of our research integrity team to safeguard the quality of each article we publish.