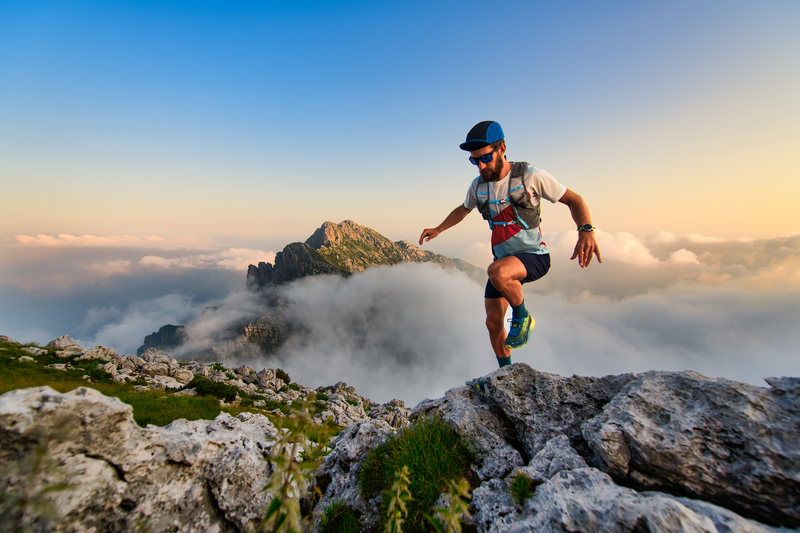
94% of researchers rate our articles as excellent or good
Learn more about the work of our research integrity team to safeguard the quality of each article we publish.
Find out more
EDITORIAL article
Front. Neuroinform. , 07 January 2025
Volume 18 - 2024 | https://doi.org/10.3389/fninf.2024.1543121
This article is part of the Research Topic Protecting Privacy in Neuroimaging Analysis: Balancing Data Sharing and Privacy Preservation View all 6 articles
Editorial on the Research Topic
Protecting privacy in neuroimaging analysis: balancing data sharing and privacy preservation
Neuroimaging is an indispensable tool in neuroscience and medical research, enabling precise investigations into brain structure and function (Yen et al., 2023; Yan et al., 2022; Shoeibi et al., 2023; Botvinik-Nezer and Wager, 2023; Leite et al., 2024; Wager and Smith, 2003). Techniques such as Magnetic Resonance Imaging (MRI) generate vast amounts of sensitive data, rich in insights yet fraught with privacy challenges (Saponaro et al., 2022; Cali et al., 2023; Li et al., 2020; Zou et al., 2024; Acar et al., 2023). As scientific progress depends on data sharing and collaboration (Martone, 2023), balancing these needs with robust privacy preservation has become a critical concern (Zhang et al., 2020). This Research Topic addresses this challenge by exploring innovative methodologies, frameworks, and technologies that advance the field while safeguarding individual privacy.
The Research Topic aims to promote interdisciplinary research into privacy-preserving solutions for neuroimaging analysis, ensuring compliance with ethical and legal standards (Li et al., 2020). It seeks to balance data utility with privacy protections by fostering methods for anonymization, leveraging AI tools such as federated learning and differential privacy, and aligning technologies with global governance frameworks (Zou et al., 2024; Jeon et al., 2020; Dwork, 2006; Abadi et al., 2016). This Research Topic serves as a roadmap for ethical neuroimaging research and a platform for dialogue among neuroscientists, AI researchers, ethicists, and policymakers.
This Research Topic features five papers that exemplify the breadth and depth of research at the intersection of privacy, neuroimaging, and artificial intelligence. Each contribution highlights a unique facet of the privacy-preserving landscape, collectively offering a comprehensive exploration of the field's current state and future potential.
The first paper (Cao et al.) tackles the pervasive issue of inflated effect sizes in small-sample neuroimaging studies, a challenge that undermines reproducibility and generalizability. By employing hierarchical Bayesian models, the authors demonstrate how statistical recalibration can improve the reliability of findings while enabling collaborative meta-analyses across studies. This methodological advance sets a foundation for ensuring that shared neuroimaging data is not only secure but also statistically robust.
The second paper (Spahr et al.) explores AI-driven segmentation methods for intracranial hemorrhage detection in CT scans. Leveraging self-supervised and weakly-supervised learning, the study addresses the need for label-efficient solutions that minimize reliance on large, annotated datasets. This work showcases how AI innovations can enhance efficiency and maintain privacy, particularly in resource-constrained environments where data annotation is a bottleneck.
Federated learning takes center stage in the third and fourth papers, both of which highlight its potential for decentralized neuroimaging analysis. The third paper (Mitrovska et al.) introduces a secure federated learning framework for Alzheimer's disease detection, incorporating secure aggregation techniques to protect sensitive data during model training. Similarly, the fourth paper (Thapaliya et al.) presents Sparse Federated Learning for Neuroimaging (NeuroSFL), which optimizes communication efficiency by focusing on sparse sub-networks. Together, these studies underscore the adaptability and scalability of federated learning as a cornerstone of privacy-preserving neuroimaging research.
The final paper (Alsaigh et al.) adopts a broader lens, examining the alignment of AI governance frameworks with neuroinformatics practices. By identifying gaps in existing regulations and proposing strategies for harmonization, the authors provide a roadmap for integrating privacy-preserving technologies within the complex landscape of global governance. This contribution emphasizes the importance of aligning technical advancements with ethical principles, ensuring trust and transparency in neuroimaging research.
Artificial intelligence serves as a driving force behind many of the contributions in this Research Topic, offering powerful tools for balancing data sharing and privacy. AI-driven methodologies such as federated learning, differential privacy, and explainable AI not only enable secure data analysis but also enhance transparency and trustworthiness (Yuste, 2023; White et al., 2022; Yang et al., 2022). These technologies address critical challenges, such as mitigating privacy risks in decentralized environments and ensuring that sensitive neuroimaging data remains private without compromising utility.
Federated learning, in particular, emerges as a transformative approach, allowing researchers to train models collaboratively without sharing raw data. The secure aggregation and sparsity-focused innovations presented in this Research Topic demonstrate how federated learning can scale to meet the demands of large, heterogeneous neuroimaging datasets. Complementary technologies such as differential privacy and blockchain also hold promise for further enhancing data security and accountability, though their integration into routine neuroimaging workflows remains a challenge.
Despite these advancements, significant challenges persist. Balancing data utility with privacy remains a fundamental tension, as techniques that protect privacy often introduce trade-offs in model performance or scalability (Yuste, 2023; Mitrovska et al.). For instance, federated learning frameworks are susceptible to performance degradation in non-IID (non-independent and identically distributed) data settings, a common scenario in neuroimaging. Similarly, the computational demands of privacy-preserving technologies may limit their accessibility to smaller research institutions, exacerbating inequities in the field.
Ethical and societal challenges add another layer of complexity (Aboy et al., 2024; van Kolfschooten and van Oirschot, 2024). Cognitive privacy, informed consent, and equitable access to the benefits of neuroimaging research are ongoing concerns (Bublitz et al., 2024). The rapid evolution of AI often outpaces the development of regulatory frameworks, creating misalignments between technological capabilities and ethical oversight (Ratto Trabucco, 2023; Ienca and Ignatiadis, 2020; Wajnerman Paz, 2022; Jwa and Martinez-Martin, 2024; Yuste et al., 2017; Genser et al., 2024). Addressing these gaps will require sustained dialogue among stakeholders, including neuroscientists, technologists, ethicists, and policymakers (Ligthart et al., 2023; Bublitz et al., 2024).
This Research Topic highlights the transformative potential of privacy-preserving technologies in neuroimaging, emphasizing the critical balance between advancing data sharing and maintaining individual privacy. By presenting cutting-edge methodologies, practical frameworks, and real-world applications, the contributions collectively offer a comprehensive roadmap for ethical and innovative neuroimaging research. These works demonstrate that privacy and progress can coexist, fostering collaboration and trust across disciplines.
The ubiquity of AI further amplifies the need for dynamic and adaptive regulatory frameworks that evolve alongside technological advancements. As neuroimaging intersects with AI-driven innovation, static and siloed regulations are insufficient to address the complexity of overlapping challenges in data governance and privacy. Instead, flexible approaches that align AI's transformative capabilities with ethical oversight are essential to ensuring responsible progress.
This Research Topic is a testament to the potential of interdisciplinary collaboration in tackling complex challenges at the intersection of technology, ethics, and neuroscience. By fostering dialogue and innovation, it lays the groundwork for a future where neuroimaging research thrives in an environment of trust, transparency, and shared progress. We invite readers to engage with these contributions, advancing the conversation and shaping a more ethical and innovative future for neuroimaging and beyond.
This Research Topic would not have been possible without the dedication of the authors, whose innovative research forms its foundation, the reviewers, whose constructive feedback ensured its rigor, and the neuroimaging and AI communities, whose contributions drive progress in privacy preservation and scientific discovery.
RM: Writing – original draft, Writing – review & editing. ML: Writing – original draft, Writing – review & editing. XL: Writing – original draft, Writing – review & editing. JC: Writing – original draft, Writing – review & editing. SS: Writing – original draft, Writing – review & editing.
The author(s) declare financial support was received for the research, authorship, and/or publication of this article. This article was derived from a research grant funded by the Research, Development, and Innovation Authority (RDIA), Kingdom of Saudi Arabia, with grant number 12615-iu-2023-IU-R-2-1-EI-.
SS was employed by NVIDIA Corporation.
The remaining authors declare that the research was conducted in the absence of any commercial or financial relationships that could be construed as a potential conflict of interest.
The author(s) declared that they were an editorial board member of Frontiers, at the time of submission. This had no impact on the peer review process and the final decision.
All claims expressed in this article are solely those of the authors and do not necessarily represent those of their affiliated organizations, or those of the publisher, the editors and the reviewers. Any product that may be evaluated in this article, or claim that may be made by its manufacturer, is not guaranteed or endorsed by the publisher.
Abadi, M., H. McMahan, B., Chu, A., Mironov, I., Zhang, L., Goodfellow, I., et al. (2016). “Deep learning with differential privacy,” in Proceedings of the ACM Conference on Computer and Communications Security, 308–18. doi: 10.1145/2976749.2978318
Aboy, M., Minssen, T., and Vayena, E. (2024). Navigating the EU AI act: implications for regulated digital medical products. NPJ Digit. Med. 7, 1–6. doi: 10.1038/s41746-024-01232-3
Acar, F., Maumet, C., Heuten, T., Vervoort, M., Bossier, H., Seurinck, R., et al. (2023). Review paper: reporting practices for task FMRI studies. Neuroinformatics 21, 221–42. doi: 10.1007/s12021-022-09606-2
Botvinik-Nezer, R., and Wager, T. D. (2023). Reproducibility in neuroimaging analysis: challenges and solutions. Biol. Psychiat. 8, 780–88. doi: 10.1016/j.bpsc.2022.12.006
Bublitz, C., Molnár-Gábor, F., and Soekadar, S. R. (2024). Implications of the novel EU AI act for neurotechnologies. Neuron 112, 3013–16. doi: 10.1016/j.neuron.2024.08.011
Cali, R. J., Bhatt, R. R., Thomopoulos, S. I., Gadewar, S., Ba Gari, I., Chattopadhyay, T., et al. (2023). The influence of brain MRI defacing algorithms on brain-age predictions via 3D convolutional neural networks. BioRxiv. doi: 10.1109/EMBC40787.2023.10340740
Dwork, C. (2006). “Differential privacy,” in Lecture Notes in Computer Science (Including Subseries Lecture Notes in Artificial Intelligence and Lecture Notes in Bioinformatics), 1–12.
Genser, J., Damianos, S., and Yuste, R. (2024). Safeguarding Brain Data: Assessing the Privacy Practices of Consumer Neurotechnology Companies.
Ienca, M., and Ignatiadis, K. (2020). Artificial intelligence in clinical neuroscience: methodological and ethical challenges. AJOB Neurosci. 11, 77–87. doi: 10.1080/21507740.2020.1740352
Jeon, B., Ferdous, S. M., Rahman, M. R., and Walid, A. (2020). Privacy-Preserving Decentralized Aggregation for Federated Learning, December.
Jwa, A. S., and Martinez-Martin, N. (2024). Rationales and approaches to protecting brain data: a scoping review. Neuroethics 17, 1–15. doi: 10.1007/s12152-023-09534-1
Leite, J., Nhoatto, F., Jacob, A., Santana, R., and Lobato, F. (2024). Computational tools for neuronal morphometric analysis: a systematic search and review. Neuroinformatics 22, 353–77. doi: 10.1007/s12021-024-09674-6
Li, X., Gu, Y., Dvornek, N., Staib, L. H., Ventola, P., and Duncan, J. S. (2020). Multi-site FMRI analysis using privacy-preserving federated learning and domain adaptation: ABIDE results. Med. Image Analy. 65:101765. doi: 10.1016/j.media.2020.101765
Ligthart, S., Ienca, M., Meynen, G., Molnar-Gabor, F., Andorno, R., Bublitz, C., et al. (2023). Minding rights: mapping ethical and legal foundations of ‘neurorights.' Cambridge Quart. Healthcare Ethics 32, 461–81. doi: 10.1017/S0963180123000245
Martone, M. E. (2023). The past, present and future of neuroscience data sharing: a perspective on the state of practices and infrastructure for FAIR. Front. Neuroinform. 17:1276407. doi: 10.3389/fninf.2023.1276407
Ratto Trabucco, F. (2023). Neurorights between ethical and legal implications. Cuadernos de Derecho Transnacional 15, 750–57. doi: 10.20318/cdt.2023.7561
Saponaro, S., Giuliano, A., Bellotti, R., Lombardi, A., Tangaro, S., Oliva, P., et al. (2022). Multi-site harmonization of MRI data uncovers machine-learning discrimination capability in barely separable populations: an example from the ABIDE dataset. NeuroImage. Clini. 35:103082. doi: 10.1016/j.nicl.2022.103082
Shoeibi, A., Khodatars, M., Jafari, M., Ghassemi, N., Moridian, P., Alizadehsani, R., et al. (2023). Diagnosis of brain diseases in fusion of neuroimaging modalities using deep learning: a review. Inform. Fusion 93, 85–117. doi: 10.1016/j.inffus.2022.12.010
van Kolfschooten, H., and van Oirschot, J. (2024). The EU artificial intelligence act (2024): implications for healthcare. Health Policy 149:105152. doi: 10.1016/j.healthpol.2024.105152
Wager, T. D., and Smith, E. E. (2003). Neuroimaging studies of working memory: a meta-analysis. Cognitive, Affect. Behav. Neurosci. 3, 255–74. doi: 10.3758/CABN.3.4.255
Wajnerman Paz, A. (2022). Is your neural data part of your mind? exploring the conceptual basis of mental privacy. Minds Mach. 32, 395–415. doi: 10.1007/s11023-021-09574-7
White, T., Blok, E., and Calhoun, V. D. (2022). Data sharing and privacy issues in neuroimaging research: opportunities, obstacles, challenges, and monsters under the bed. Human Brain Mapp. 43:278. doi: 10.1002/hbm.25120
Yan, W., Qu, G., Hu, W., Abrol, A., Cai, B., Qiao, C., et al. (2022). Deep learning in neuroimaging: promises and challenges. IEEE Signal Proc. Magaz. 39, 87–98. doi: 10.1109/MSP.2021.3128348
Yang, Z., Shi, Y., Zhou, Y., Wang, Z., and Yang, K. (2022). Trustworthy federated learning via blockchain. IEEE Intern. Things J. 2022, 1–1. doi: 10.1109/JIOT.2022.3201117
Yen, C., Lin, C., and Chiang, M. C. (2023). Exploring the frontiers of neuroimaging: a review of recent advances in understanding brain functioning and disorders. Life 13:1472. doi: 10.3390/life13071472
Yuste, R. (2023). Advocating for neurodata privacy and neurotechnology regulation. Nature Protoc. 18, 2869–75. doi: 10.1038/s41596-023-00873-0
Yuste, R., Goering, S., Agüeray Arcas, P., Bi, G., Carmena, J. M., Carter, A., et al. (2017). Four ethical priorities for neurotechnologies and AI. Nature 551, 159–163. doi: 10.1038/551159a
Zhang, Y. D., Dong, Z., Wang, S. H., Yu, X., Yao, X., Zhou, Q., et al. (2020). Advances in multimodal data fusion in neuroimaging: overview, challenges, and novel orientation. Inform. Fusion 64, 149–187. doi: 10.1016/j.inffus.2020.07.006
Keywords: neuroimaging analysis, privacy-preserving, data sharing, neuroinformatics, artificial intelligence, machine learning, AI explainability, AI governance
Citation: Mehmood R, Lazar M, Liang X, Corchado JM and See S (2025) Editorial: Protecting privacy in neuroimaging analysis: balancing data sharing and privacy preservation. Front. Neuroinform. 18:1543121. doi: 10.3389/fninf.2024.1543121
Received: 10 December 2024; Accepted: 17 December 2024;
Published: 07 January 2025.
Edited and reviewed by: Michael Denker, Jülich Research Centre, Germany
Copyright © 2025 Mehmood, Lazar, Liang, Corchado and See. This is an open-access article distributed under the terms of the Creative Commons Attribution License (CC BY). The use, distribution or reproduction in other forums is permitted, provided the original author(s) and the copyright owner(s) are credited and that the original publication in this journal is cited, in accordance with accepted academic practice. No use, distribution or reproduction is permitted which does not comply with these terms.
*Correspondence: Rashid Mehmood, Ui5NZWhtb29kQGdtYWlsLmNvbQ==; Ui5NZWhtb29kQGl1LmVkdS5zYQ==
Disclaimer: All claims expressed in this article are solely those of the authors and do not necessarily represent those of their affiliated organizations, or those of the publisher, the editors and the reviewers. Any product that may be evaluated in this article or claim that may be made by its manufacturer is not guaranteed or endorsed by the publisher.
Research integrity at Frontiers
Learn more about the work of our research integrity team to safeguard the quality of each article we publish.