- 1School of Biomedical Engineering, Health Science Center, Shenzhen University, Shenzhen, China
- 2Guangdong Key Laboratory for Biomedical Measurements and Ultrasound Imaging, Shenzhen University, Shenzhen, China
- 3National-Regional Key Technology Engineering Laboratory for Medical Ultrasound, Shenzhen University, Shenzhen, China
- 4Guangdong Key Laboratory of Mental Health and Cognitive Science, South China Normal University, Guangzhou, China
- 5Center for Studies of Psychological Application, School of Psychology, South China Normal University, Guangzhou, China
- 6Peng Cheng Laboratory, Shenzhen, China
- 7Department of Child Psychiatry, Shenzhen Kangning Hospital, Shenzhen University School of Medicine, Shenzhen, China
Both the Pearson correlation and partial correlation methods have been widely used in the resting-state functional MRI (rs-fMRI) studies. However, they can only measure linear relationship, although partial correlation excludes some indirect effects. Recent distance correlation can discover both the linear and non-linear dependencies. Our goal was to use the multivariate pattern analysis to compare the ability of such three correlation methods to distinguish between the patients with obsessive-compulsive disorder (OCD) and healthy control subjects (HCSs), so as to find optimal correlation method. The main process includes four steps. First, the regions of interest are defined by automated anatomical labeling (AAL). Second, functional connectivity (FC) matrices are constructed by the three correlation methods. Third, the best discriminative features are selected by support vector machine recursive feature elimination (SVM-RFE) with a stratified N-fold cross-validation strategy. Finally, these discriminative features are used to train a classifier. We had a total of 128 subjects out of which 61 subjects had OCD and 67 subjects were normal. All the three correlation methods with SVM have achieved good results, among which distance correlation is the best [accuracy = 93.01%, specificity = 89.71%, sensitivity = 95.08%, and area under the receiver-operating characteristic curve (AUC) = 0.94], followed by Pearson correlation and partial correlation is the last. The most discriminative regions of the brain for distance correlation are right dorsolateral superior frontal gyrus, orbital part of left superior frontal gyrus, orbital part of right middle frontal gyrus, right anterior cingulate and paracingulate gyri, left the supplementary motor area, and right precuneus, which are the promising biomarkers of OCD.
Introduction
Obsessive-compulsive disorder (OCD) is a mental disorder that causes repeated and unwanted thoughts and/or obsessive feelings and compulsive actions and it can limit the ability of the patient to take part in relationships, the workplace, and in society (Piacentini et al., 2003; Abramowitz et al., 2009). Its prevalence is about 1–3% lifetime (Ruscio et al., 2010; Rapinesi et al., 2019). In clinical practice, no diagnostic biomarkers are available for OCD and its diagnosis is always based on some symptom-oriented criteria according to the International Classification of Diseases (ICD; Stein et al., 2016) and the Diagnostic and Statistical Manual of Mental Disorders (DSM; Battle, 2013). However, these criteria may have several problems over the conditions of an individual. For example, the patients with OCD often co-occur with depression and anxiety or another psychiatric comorbidity, which can contribute to misdiagnosis.
With the development of medical imaging, researchers can explore the pathogenesis of OCD. Currently, the pathogenesis of OCD has been confirmed to be caused by the cortico-striato-thalamo-cortical (CSTC) circuit dysfunction, but emerging evidence indicates that broader brain regions, such as the left supplementary motor area (SMA) and right precuneus, are involved in this disorder (Saxena et al., 1998; Rehn et al., 2018; Thorsen et al., 2018; Hazari et al., 2019). These changes in the brain are due to the diversity of tasks in the investigation of OCD. Therefore, task-based functional MRI (task-fMRI) has been studied for detecting the functional changes in the brain in patients with OCD and their relatives (Menzies et al., 2008a). However, task-fMRI studies can only focus on some specific regions of the brain and may have missed important information existing in regions of the brain not related to the task. Without specific design in task-fMRI, resting-state functional MRI (rs-fMRI) provides an effective and noninvasive approach to assess the neural activation and functional connectivity (FC) of the human brain without any hypothesis. It can also provide a reliable measure of baseline brain activity and may complement and extend findings from task-based studies (Biswal et al., 1995; Hou et al., 2014; de Vries et al., 2019; Yang et al., 2019).
Recently, the multivariate pattern analysis based on a machine learning (ML) algorithm has been introduced for neuroimaging analysis of a variety of diseases such as autism, depression, and schizophrenia (Sajda, 2006; Anderson et al., 2011; Zeng et al., 2012; Liu et al., 2014, 2015a, 2017; Mueller et al., 2015; Rathore et al., 2017; Lamothe et al., 2018; Zhou et al., 2018; Bu et al., 2019; Rapinesi et al., 2019). It has the advantage of being able to inference individual level over the univariate analysis used at the group level (Orrù et al., 2012; Goodman et al., 2014). In comparison to other traditional methods of analysis, its ability to use inter-regional correlations, such as the Pearson correlation, to detect subtle and spatially distributed effects (Menzies et al., 2008b; Bruin et al., 2020; Zhan et al., 2021). Therefore, it seems particularly well-suited for the neuroimaging analyses in OCD, as abnormalities are typically distributed across the brain (Klöppel et al., 2008; Arbabshirani et al., 2017).
In this study, we employed the multivariate pattern analysis via the three correlation methods to distinguish the patients with OCD from a healthy control subject (HCS). A general flowchart of rs-fMRI based on the FC matrix for diagnosis is shown in Figure 1. In this framework, there are four main steps: (1) defining the region of interests (ROIs) from the rs-fMRI images or by using the anatomically and functionally defined reference atlases of the brain, (2) extracting rs-fMRI time series based on the ROIs and calculating the FC matrices, (3) using feature selection method to get the optimal features from the FC matrices, and (4) training a classifier.

Figure 1. Classification of flow chart with four main steps: (1) defining the ROIs from the rs-fMRI images or by using anatomical templates that have been defined, (2) extracting rs-fMRI time series based on the ROIs and calculating the FC matrices, (3) using feature selection method to define the optimal features from the FC matrices, and (4) training a classifier. ROIs, region of interests; rs-fMRI, resting-state functional MRI; FC, functional connectivity.
Currently, this study mainly focused on the second and third parts. In the second part, with rs-fMRI time series data, the FC matrix can be extracted for characterizing the network structure of the brain. One way is to calculate the Pearson correlation between rs-fMRI time series over the ROIs predefined as automated anatomical labeling (AAL) with 116 structural regions (Tzourio-Mazoyer et al., 2002). For example (Shenas et al., 2013; Gruner et al., 2014; Sen et al., 2016; Takagi et al., 2017), the authors use Pearson correlation as the network features. In addition, the partial correlation was also used for measuring the FC (Varoquaux et al., 2010; Smith et al., 2011; Dadi et al., 2019). However, the Pearson correlation and partial correlation only discover the linear dependency, although the partial correlation excludes the indirect influence of the correlation structure. To overcome this limitation, the distance correlation was proposed to measure both linear and non-linear associations between the two ROIs (Szekely et al., 2007; Yoo et al., 2019).
In the third part, because of high-dimensional features from the FC matrix, we need a feature selection algorithm to reduce the dimensionality. In literature, the recursive feature elimination (RFE) algorithm is a very excellent feature selection technique that has been widely used in many fields (Guyon et al., 2002; Ding et al., 2015; Liu et al., 2015b; Lin et al., 2017; Wang et al., 2019), but it needs a specific classifier. Currently, studies on the diagnosis of OCD disease are usually limited to a small data set, so the researchers tend to use traditional ML methods to complete the task. Among them, support vector machine (SVM) provides excellent performance (Shenas et al., 2013; Gruner et al., 2014; Sen et al., 2016; Takagi et al., 2017; Wang et al., 2019). Therefore, we applied the SVM-RFE algorithm to filter the features.
In the fourth part, these selected features were entered into the seven classifiers. According to the final classification performance, we can explore the optimal FC method and classifier and investigate the regions of the brain, which may be potential biomarkers. Finally, our aims were 2-fold: one is to investigate which correlation method achieves the best discrimination between OCD and HCS and the other is to explore some potential biomarkers according to the above results.
Materials and Methods
Participants
This study was approved by the Ethics Committee of Shenzhen Kangning Hospital and the written informed consent was obtained from each participant. A total of 128 subjects were enrolled from Shenzhen Kangning Hospital and Guangzhou Brain Hospital, including 67 HCS and 61 patients with OCD, aged from 13 to 63 years old. The demographic information and clinical characteristics information are shown in Table 1. The independent sample t-test was carried out on the age (p = 0.45). For the Yale-Brown Obsessive Compulsive Scale (Y-BOCS) total score, the Y-BOCS obsessions score, and the Y-BCOS compulsions score (p < 0.001), we used the independent sample Kruskal–Wallis test.
Imaging Data Acquisition
A 3.0-Tesla MR system (Philips Medical Systems, Best, the Netherlands) equipped with an eight-channel phased-array head coil was used for the data acquisition. Functional data were collected by using gradient echo-planar imaging (EPI) sequences [time repetition (TR) = 2,000 ms, echo time (TE) = 60 ms, flip angle = 90°, 33 slices, field of view (FOV) = 240 mm2 × 240 mm2, matrix = 64 × 64, slice thickness = 4 mm, and voxel size = 3.75 mm3 × 3.75 mm3 × 4 mm3]. For each participant, the fMRI scanning lasted for 480 s and 240 volumes were obtained. For spatial normalization and localization, a high-resolution T1-weighted anatomical image was also acquired by using a magnetization prepared gradient echo sequence (TR = 8 ms, TE = 3.7 ms, flip angle = 7°, FOV = 240 mm2 × 240 mm2, matrix = 256 × 256, slice thickness = 1 mm, and voxel size = 0.94 mm3 × 0.94 mm3 × 1 mm3). During the scanning, the participants were instructed to relax with their eyes closed and stay awake without moving.
Data Preprocessing
The data were preprocessed by using the Statistical Parametric Mapping toolbox (SPM12, https://www.fil.ion.ucl.ac.uk/spm) and the Data Processing Assistant for Resting-State fMRI (DPARSF version 4.4, http://rfmri.org/dpabi; Shenas et al., 2013, 2014). Image preprocessing consisted of: (1) removing first the 10-time points; (2) slicing timing correction; (3) realigning the time series of the images for each subject; (4) T1-weighted individual structural images by coregistered to the mean functional image; (5) the transformed structural images by segmented into gray matter, white matter, and cerebrospinal fluid; (6) based on these segmented images, using diffeomorphic anatomical registration through exponentiated lie algebra (DARTEL) (Ashburner, 2007) tool to estimate the normalization parameters from individual native space to the Montreal Neurological Institute (MNI) space (Xue et al., 2020); (7) the functional imaging data normalized to the MNI space by using these normalization parameters and resampling at 3 mm3 × 3 mm3 × 3 mm3; (8) nuisance covariate regression (head motion parameters, white matter signal, and cerebrospinal fluid signal); (9) spatial smoothing with a 4-mm full-width half-maximum isotropic Gaussian kernel; (10) band-pass filtering (0.01–0.08 Hz); and (11) micro-head-motion correction according to framewise displacement (FD) by replacing the rs-fMRI volume with FD > 0.5 mm (nearest neighbor interpolation).
Definition of ROIs and Calculation of the FC Matrix
In this study, we employed an AAL atlas to define the ROIs. For the calculation of the FC matrix, the Pearson correlation, partial correlation, and distance correlation methods will be used in this study. For each subject, the mean of time series over all voxels in each region was extracted. The FC matrices were calculated between these average time courses with the three correlation methods implemented with Nilearn software (http://nilearn.github.io/). Considering that the matrix was symmetric, we only needed to take the lower triangle of the matrix. Finally, we flattened the lower trig matrix to get a feature vector with a length of (116 × 116 – 116)/2 = 6,670. In our following experiment, each feature was normalized by Fisher's z-transformation (Fisher, 1915; Vergun et al., 2013; Kassraian-Fard et al., 2016).
Feature Selection and Classification
In this study, to reduce the dimension of the data and find the most discriminative subset of feature, we applied SVM-RFE with a stratified N-fold cross-validation strategy for feature selection (SVM-RFE-NCV). It is a sequential backward selection algorithm based on the maximum margin principle of SVM under the N-fold cross-validation. The process contains five steps: (1) training the model with the samples, (2) sorting the scores of each feature, (3) removing the features with the minimum scores, (4) training the model again with the remaining features and repeating the process, and (5) selecting the required features (Ding et al., 2015; Wang et al., 2019). With the selected features, seven classifiers are compared: SVM with linear kernel, multilayer perceptron (MLP), extreme gradient boosting (XGBoost), gradient boosting decision tree (GBDT), graph convolution network (GCN), and sparse L1 and non-sparse L2 regularization for the logistic regression classifiers (LR-L1 and LR-L2; Friedman et al., 2001; Chen and Guestrin, 2016; Kipf, 2017). The SVM-RFE-NCV process was embedded in a classification framework with 10-fold cross-validation (10-CV).
Performance Evaluation
The performance of the proposed classifiers is assessed by using the four performance measures: specificity, sensitivity, accuracy, and area under the receiver-operating characteristic curve (AUC). To test whether these classification scores are significant, we performed a permutation test: we first randomly reassigned the subject labels and then performed the 10-CV classification. This procedure was repeated by 1,000 times. The p-value was then calculated by dividing the number of times that showed a higher value than the derived from the non-permuted model by the total number of permutations (Plitt et al., 2015).
Results
In this study, some qualitative and quantitative comparison results are provided. At first, we qualitatively compared the three methods via the scatter plot and correlation visualization. Then, the classification results of the OCD and HCS are evaluated according to the pipelines composed of the three correlation measures, the SVM-RFE-NCV, and the seven classifiers, to select correlation measure and classifier to obtain the best discrimination between the OCD and HCS. In addition, we will use the SVM-RFE-NCV to find the regions of the brain corresponding to the most discriminative features. The SVM-RFE-NCV and classification algorithms are implemented by using Scikit-learn (Pedregosa et al., 2012).
Scatter Plot and Correlation Visualization
To explore the differences among the Pearson, partial, and distance correlations, we calculated the average functional matrices of the patients and HCS, respectively. Since the distance correlation coefficient ranges from 0 to 1 while the other two range from−1 to 1, we used the unsigned versions of the Pearson and partial correlation coefficients (for example, taking the absolute value of them). The results are shown in Figure 2 and we can see that both the distance and Pearson correlations give similar structures of the functional matrix, while the structure of partial correlation is greatly different from them. To further reflect the similarities and differences among the Pearson, distance and partial correlations, we draw their scatter plots as shown in Figure 3. The values of three coefficients mainly lie in the different intervals: (-0.1, 0.9) for the Pearson correlation coefficients, (-0.1, 0.1) for the partial correlation coefficients, and (0.1, 0.8) for the distance correlation coefficients.
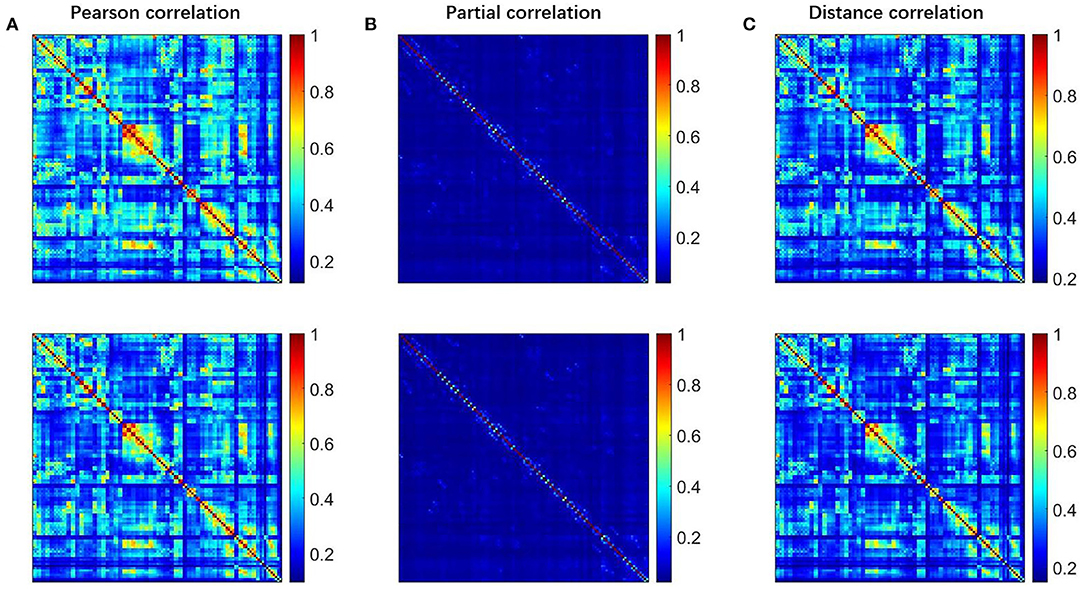
Figure 2. The FC matrices for HCS and the patients with OCD. The first row is for HCS and the second row is for the patients with OCD. (A) Pearson correlation, (B) partial correlation, and (C) distance correlation. FC, functional connectivity; OCD, obsessive-compulsive disorder; HCS, healthy control subject.
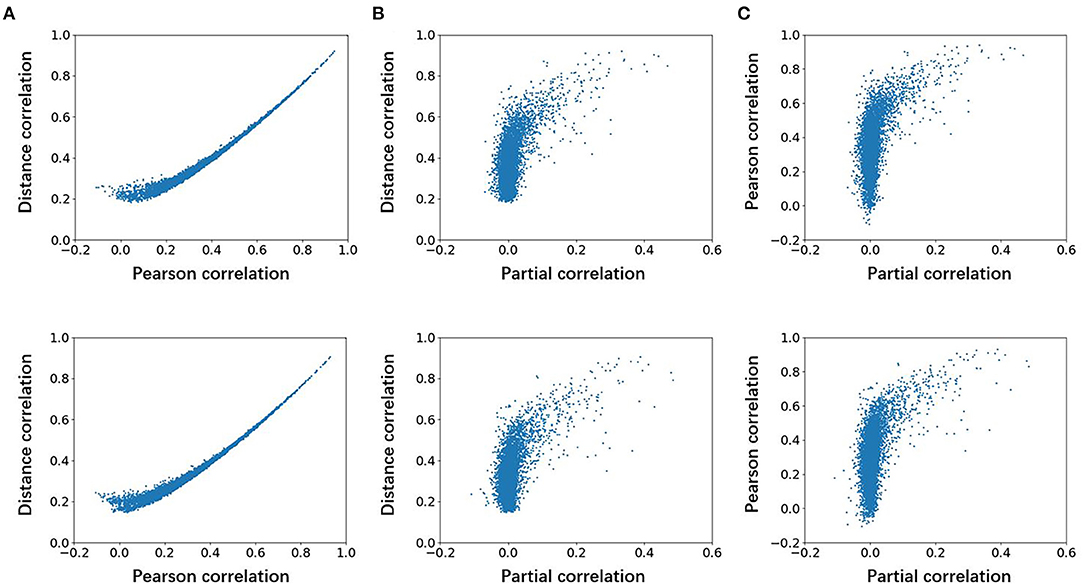
Figure 3. Scatter plots of association among the Pearson, partial, and distance correlations for average functional connectivity across the participants. The first row is for HCS and the second row is for the patients with OCD. (A) Distance correlation vs. Pearson correlation, (B) distance correlation vs. partial correlation, and (C) pearson correlation vs. partial correlation. OCD, obsessive-compulsive disorder; HCS, healthy control subject.
Choice of FC Method
To find the optimal correlation method, we proceeded in two steps. First, for each correlation method, we used the SVM-RFE-NCV to find the best feature subset that gave the prediction. Second, we compared the performance of each correlation method on the best feature subset.
Best Feature Subset
For the SVM-RFE-NCV, the number of optimal features (NOFs) varies with N. Table 2 summarizes the changes of accuracy and NOF under the different N conditions. For the Pearson correlation, partial correlation, and distance correlation, the performance is the highest when N is equal to 5, 8, and 5, respectively. Therefore, the best feature subset of the Pearson correlation and distance correlation was obtained by the SVM-RFE-5CV algorithm. The best feature subset of partial correlation was achieved by the SVM-RFE-8CV algorithm.
Best Correlation Method
Three correlation methods produced a good performance in the classification. Their ROC curves are shown in Figure 4, from which we can see that they exhibit good performance, with AUC values range from 0.87 to 0.94 (p < 0.01). The other classification results of the three correlation methods for the patients with OCD and HCS are summarized in Table 3. The distance correlation and Pearson correlation are slightly lower than partial correlation in sensitivity, but distance correlation was the best in accuracy, specificity, and AUC followed by the Pearson correlation and partial correlation. Therefore, in the classification of the OCD and HCS, distance correlation comprehensive performance is the best. Its accuracy, sensitivity, and specificity are 93.01, 89.71, and 95.08% (p < 0.01), respectively. The second is Pearson correlation (accuracy = 89.74%, sensitivity = 89.71%, and specificity = 86.62%, p < 0.01). For partial correlation, accuracy is 84.87%, sensitivity is 96.21%, and specificity is 75.90% (p < 0.01).
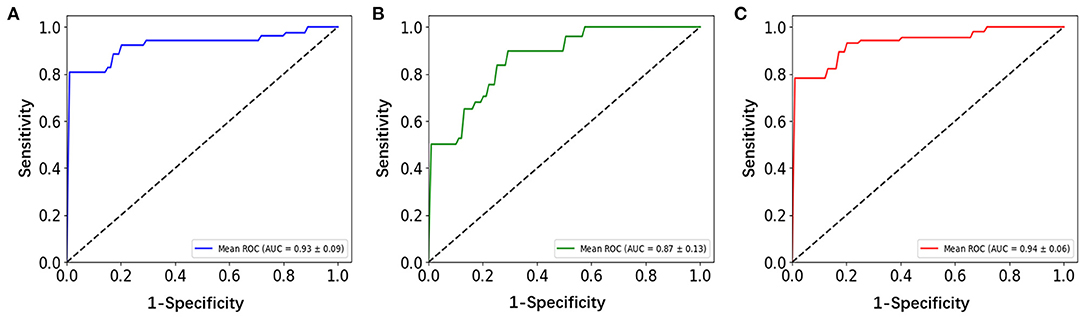
Figure 4. ROC curves assessing Pearson correlation, partial correlation, and distance correlation performance by using SVM. (A) Pearson correlation, (B) partial correlation, and (C) distance correlation. ROC, receiver-operating characteristic; SVM, support vector machine.
Results of Different Classifiers
Through the above analysis, the best discriminative features can be obtained by using the distance correlation and the SVM-RFE-5CV. As stated earlier, the seven classifiers including SVM with linear kernel, MLP, XGBoost, GBDT, GCN, LR-L1, and LR-L2 classifiers were applied to identify these features separately. For SVM, the penalty parameter was set to 1. For LR-L1 and LR-L2, the penalty parameter was set to 0.01. For XGBoost, the learning rate was set to 0.01, the number of gradients boosted trees (n_estimators) to 200, maximum depth of the tree (max_depth) to 5, subsample ratio of the training instance (subsample) to 0.85, the minimum sum of instance weight needed in a child to 2, subsample ratio of the columns when constructing each tree to 0.7, and the other parameters to the default values. For GBDT, the learning rate was set to 0.01, n_estimators to 600, max_depth to 3, subsample ratio to 0.7, the minimum number of samples required to be at a leaf node to 10, the minimum weighted fraction of the total of weights required to be a leaf node to 0.1, and other parameters to the default values. For the GCN and MLP, dropout was set to 0.1, weight decay to 1 × 10−3, learning rates to 0.02 and 0.05, number of epochs to 1,000, number of layers to 2, and the numbers of neurons per layer to 128 and 256. The results of classification by 10-CV are given in Table 4. The optimal classification result is achieved via SVM for accuracy, sensitivity, specificity, and AUC with values as high as 93.01, 89.71, 95.08%, 0.94 (p < 0.01), respectively.
Potential Biomarkers From Connectivity Patterns
To find the regions of the brain that strongly contributed to the discrimination between the patients with OCD and HCS, we selected the top 10 most discriminative features according to the SVM-RFE-NCV method. Specific regions of the brain were then located based on these features. The spatial maps of the regions of the brain (Xia et al., 2013) are shown in Figure 5 and the detailed information is listed in Table 5.
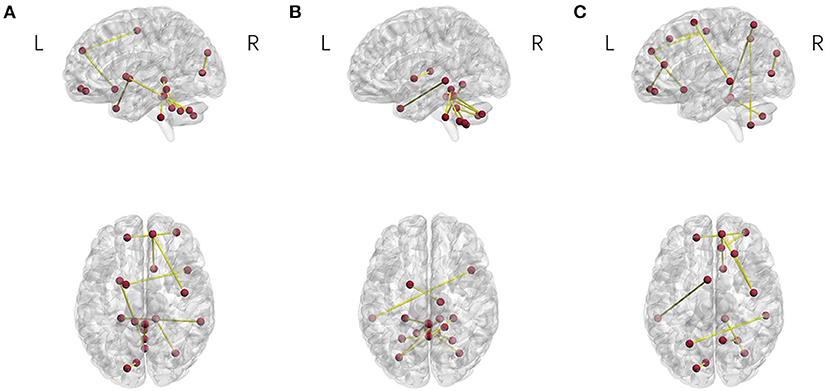
Figure 5. Brain connectivity patterns for the three correlation methods. (A) Pearson correlation, (B) partial correlation, and (C) distance correlation.
For Pearson correlation, the most discriminative regions included the right precentral gyrus, orbital part of left superior frontal, orbital part of right middle frontal gyrus, right olfactory cortex, the medial part of right superior frontal gyrus, left calcarine fissure and surrounding cortex, left superior occipital gyrus, left putamen, left globus pallidus, right middle temporal gyrus, right middle temporal pole, right crus II of cerebellar hemisphere, left lobule III of cerebellar hemisphere, right lobule III of cerebellar hemisphere, left lobule X of cerebellar hemisphere, lobule III of the vermis, lobule VIII of the vermis, lobule IX of the vermis, and lobule X of the vermis.
For partial correlation, the most discriminative regions for OCD were composed of the left globus pallidus, right thalamus, left middle temporal gyrus, right middle temporal pole, left crus II of cerebellar hemisphere, right crus II of cerebellar hemisphere, right lobule III of cerebellar hemisphere, right lobule IV of cerebellar hemisphere, right lobule V of cerebellar hemisphere, left lobule VIII of cerebellar hemisphere, right lobule VIII of cerebellar hemisphere, left lobule VIII of cerebellar hemisphere, right lobule IX of cerebellar hemisphere, left lobule X of cerebellar hemisphere, and right lobule X of the cerebellar hemisphere.
For distance correlation, the discriminative regions for OCD primarily consisted of the right percental gyrus, right dorsolateral superior frontal gyrus, orbital part of left superior frontal gyrus, orbital part of right middle frontal gyrus, left SMA, right olfactory cortex, the medial part of right superior frontal gyrus, right anterior cingulate and paracingulate gyri, left calcarine fissure and surrounding cortex, left superior occipital gyrus, left superior parietal gyrus, right precuneus, right superior temporal pole, right inferior temporal gyrus, left crus II of cerebellar hemisphere, left lobule III of cerebellar hemisphere, and right lobule VIII of the cerebellar hemisphere.
Discussion
The goal of this study is to investigate the potential diagnostic value of the different correlation methods in patients with OCD. We systematically compare the FC matrix-based prediction methods. Our results show that the distance correlation method is optimally followed by the Pearson correlation and partial correlation methods. Besides, a suitable classifier can effectively improve classification performance and it is vital to choose a suitable one. For this reason, we perform many experiments on the multiple classifiers (e.g., LR-L1, SVM). By comparing the different classification results, we found that SVM is the most suitable one in terms of the quantitative results.
We explored the important nodes and connectivity patterns in the network of the brain constructed by the three correlation methods. In these networks, many abnormal areas of the brain and connectivity mentioned in the previous studies about OCD were found including areas in and out of the classical CSTC circuit such as the precentral gyrus and SMA (Ku et al., 2020). These results provide preliminary support for the use of the three correlation methods, especially distance correlation, as promising classification markers for patients with OCD.
Of the three FC methods, distance correlation showed the greatest diagnostic accuracy for discriminating the patients with OCD from HCS. It has been shown that distance correlation directly reflects linear and non-linear correlation in the ROIs. Therefore, the location of the regions of the brain based on distance correlation also showed a considerable research value. For example, the SMA is involved in the planning of the movement. It has been found to involve the compulsion and repetitive behavior of OCD (Gillan et al., 2016). This effect could make the distance correlation method more sensitive to detect dysfunctional neural activity than the other two FC methods. In addition, we can find that the FC matrix based on distance correlation calculation has some intergroup differences. These intergroup differences for the FC matrix may underlie the excellent classification achieved in the current study. Therefore, these ML algorithms were able to identify the patients with OCD and HCS through the FC matrix based on distance correlation calculation. This also provides support for the FC matrix composed of distance correlation calculation as a promising classification marker for OCD.
Pearson correlation was a widely used correlation method, which was generally used to measure the linear relationship between the ROIs. Therefore, its classification performance was lower than distance correlation. In addition, they showed the similarities and differences in the regions of the brain of the Pearson and distance correlation localization. These same regions of the brain had the right precentral gyrus, orbital part of the left superior frontal, and medial part of the right superior frontal gyrus, etc. In this study, they played a critical role in exploring the pathogenesis of OCD. The medial part of the right superior frontal gyrus, corresponding to the left ventral medial prefrontal cortex (vmPFC), has also been found in the disrupted emotion and cognition induced by the symptoms of OCD (Becker et al., 2014; Apergis-Schoute et al., 2017). These different regions of the brain included left putamen, right anterior cingulate, and paracingulate gyri, etc. Among them, the regions of brain (e.g., globus pellidus, putamen.) located by Pearson correlation have great research value (Hibar et al., 2018; Calzà et al., 2019). However, due to the limitation of Pearson correlation measuring linear dependency, some crucial regions of the brain will be ignored. The brain regions located (e.g., anterior cingulate and paracingulate gyri, SMA) by distance correlation can complement and extend it (Ku et al., 2020).
Partial correlation shows good classification performance, although it is lower than the Pearson and distance correlations. It was generally used to exclude the indirect influence of the correlation structure. In other words, it can measure the degree of linear correlation between two regions of the brain without indirect influence from other regions of the brain. Therefore, we can infer that more consideration should be given to the synergies between the multiple regions of the brain in OCD. In addition, we can see that they are mainly distributed in the cerebellum in the regions of the brain defined by partial correlation. In previous studies, the cerebellum also played an important role in the exploration of the pathogenesis of OCD (Zhang et al., 2019). Therefore, we believe that partial correlation has a high potential to explore the influence of the cerebellum on OCD.
In this study, the discriminative regions of the brain are in and out of the CSTC circuit. Previous studies have reported that the orbitofrontal cortex (OFC) play crucial roles in processing reward, negative effect, and, specifically, fear and anxiety in OCD (Kringelbach and Rolls, 2004; Milad and Rauch, 2007). A recent meta-analysis of the voxel-based morphometry (VBM) studies showed decreased gray matter in the bilateral OFCs (de Wit et al., 2014). In fMRI studies, the researchers revealed the white matter abnormalities in OFC (Piras et al., 2013). Furthermore, the anterior cingulate cortex (ACC), putamen, and thalamus have been suggested to play important roles in the previous studies of OCD (Yoo et al., 2007; Zhu et al., 2015; Fan et al., 2017; Hazari et al., 2019). The OCD severity associations have been reported with hypermetabolism in the ACC (Swedo et al., 1989). In this study, consistent with these findings, the OFC, the ACC, putamen, and thalamus displayed a high degree of discriminative ability between the patients with OCD and HCS. These results provide further support for dysfunction in the CSTC circuit in patients with OCD.
In addition, some researchers found that OCD is related to the sensorimotor network (i.e., precentral gyrus/SMA) (Cui et al., 2020). Morein-Zamir et al. (2016) reported that the activation from the regions of the brain within the sensorimotor network in the inhibitory control processes may explain the essence of inhibitory control deficits of OCD. Meanwhile, one recent study indicated that OCD was associated with increased activity in the SMA. With repetitive transcranial magnetic stimulation (rTMS) treatment in SMA, the researcher can observe a reduction in the Y-BOCS score at the 4th week. The reduction in compulsion contributed to the reduction of the global Y-BOCS (Lee et al., 2017). Therefore, these previous results further support this study.
Finally, this study also showed that the cerebellum contributed to distinguish between the patients with OCD and HCS. For example, Miquel et al. (2019) suggested that inhibiting activity in the cerebellar cortex would increase impulsive and compulsive symptomatology. On the other hand, the stimulation of the cerebellar cortex should improve behavioral inhibitory control. Meanwhile, other previous studies reported the existence of disconnectedness in the fronto-striato-limbic community and connectedness between the cerebellar and visual areas in the patients with OCD, which was also related to the clinical symptomatology of OCD (Kashyap et al., 2021).
Despite the encouraging performance achieved, there are still two major limitations in the current research. First, the current study is only evaluated in a small database, which will make the results difficult to generalize. Second, the proposed method uses only single image modality data. Using a variety of modalities can obtain comprehensive features and improve the classification performance of the model. However, the subjects with the multimodal image data in the database are limited. In the future, we will explore the multimodal image data to discriminate the patients with OCD from HCS.
Conclusion
To conclude, the current experimental results show that it is promising to apply distance correlation for measuring the FC between the ROIs of the brain with contrast to both the traditional Pearson correlation and partial correlation. Besides improving the discrimination performance between the patients with OCD and HCS, the selected biomarkers via the SVM-RFE-NCV strategy may provide the potential clinical values for the patients with OCD.
Data Availability Statement
The raw data supporting the conclusions of this article will be made available by the authors, without undue reservation.
Ethics Statement
The studies involving human participants were reviewed and approved by the Shenzhen Kangning Hospital. The patients/participants provided their written informed consent to participate in this study.
Author Contributions
WL, ZP, and CC designed the experiment. CC obtained the funding. LJ and CC collected and preprocessed rs-fMRI data. QL performed the multivariate pattern analysis and completed the first draft of this article. WL, ZP, CC, and QL have finished the final version of this article. All the authors contributed and approved the final version of the article.
Funding
This study was partly supported by grants from the National Natural Science Foundation of China (61971289 and 31871113), the Shenzhen Fundamental Research Project (JCYJ20170412111316339 and JCYJ20160422113119640), and the Shenzhen-Hong Kong Institute of Brain Science—Shenzhen Fundamental Research Institutions.
Conflict of Interest
The authors declare that the research was conducted in the absence of any commercial or financial relationships that could be construed as a potential conflict of interest.
Publisher's Note
All claims expressed in this article are solely those of the authors and do not necessarily represent those of their affiliated organizations, or those of the publisher, the editors and the reviewers. Any product that may be evaluated in this article, or claim that may be made by its manufacturer, is not guaranteed or endorsed by the publisher.
Acknowledgments
We sincerely thank the patients and their families for participating in the experiment and we also thank the doctors, nurses, and technicians who assisted the patients in the experiment. We also deeply appreciate the reviewers and editors for their helpful comments and suggestions for improving our work.
References
Abramowitz, J. S., Taylor, S., and McKay, D. (2009). Obsessive-compulsive disorder. Lancet 374, 491–499. doi: 10.1016/S0140-6736(09)60240-3
Anderson, J. S., Nielsen, J. A., Froehlich, A. L., DuBray, M. B., Druzgal, T. J., Cariello, A. N., et al. (2011). Functional connectivity magnetic resonance imaging classification of autism. Brain J. Neurolgy. 134, 3742–3754. doi: 10.1093/brain/awr263
Apergis-Schoute, A. M., Gillan, C. M., Fineberg, N. A., Fernandez-Egea, E., Sahakian, B. J., and Robbins, T. W. (2017). Neural basis of impaired safety signaling in obsessive compulsive disorder. Proc. Natl. Acad. Sci. U. S. A. 114, 3216–3221. doi: 10.1073/pnas.1609194114
Arbabshirani, M. R., Plis, S., Sui, J., and Calhoun, V. D. (2017). Single subject prediction of brain disorders in neuroimaging: promises and pitfalls. NeuroImage 145, 137–165. doi: 10.1016/j.neuroimage.2016.02.079
Ashburner, J. (2007). A fast diffeomorphic image registration algorithm. NeuroImage 38, 95–113. doi: 10.1016/j.neuroimage.2007.07.007
Battle, D. E. (2013). Diagnostic and statistical manual of mental disorders (DSM). CoDAS 25, 191–192. doi: 10.1590/s2317-17822013000200017
Becker, M. P. I., Nitsch, A. M., Schlosser, R., Koch, K., Schachtzabel, C., Wagner, G., et al. (2014). Altered emotional and BOLD responses to negative, positive and ambiguous performance feedback in OCD. Soc. Cogn. Affect. Neur. 9, 1127–1133. doi: 10.1093/scan/nst095
Biswal, B., Yetkin, F. Z., Haughton, V. M., and Hyde, J. S. (1995). Functional connectivity in the motor cortex of resting human brain using echo-planar mri. Magnet. Reson. Med. 34, 537–541. doi: 10.1002/mrm.1910340409
Bruin, W. B., Taylor, L., Thomas, R. M., Shock, J. P., Zhutovsky, P., Abe, Y., et al. (2020). Structural neuroimaging biomarkers for obsessive-compulsive disorder in the ENIGMA-OCD consortium: medication matters. Transl. Psychiatr. 10:342. doi: 10.1038/s41398-020-01013-y
Bu, X., Hu, X. Y., Zhang, L. Q., Li, B., Zhou, M., Lu, L., et al. (2019). Investigating the predictive value of different resting-state functional MRI parameters in obsessive-compulsive disorder. Transl. Psychiat. 9:17. doi: 10.1038/s41398-018-0362-9
Calzà, J., Gürsel, D. A., Schmitz-Koep, B., Bremer, B., Reinholz, L., Berberich, G., et al. (2019). Altered cortico-striatal functional connectivity during resting state in obsessive-compulsive disorder. Front. psychiat. 10:319. doi: 10.3389/fpsyt.2019.00319
Chen, T., and Guestrin, C. (2016). “Xgboost: a scalable tree boosting system,” in Proceedings of the 22nd ACM Sigkdd International Conference on Knowledge Discovery and Data Mining (San Francisco, CA). doi: 10.1145/2939672.2939785
Cui, G., Ou, Y., Chen, Y., Lv, D., Jia, C., Zhong, Z., et al. (2020). Altered global brain functional connectivity in drug-naive patients with obsessive-compulsive disorder. Front. Psychiatr. 11:98. doi: 10.3389/fpsyt.2020.00098
Dadi, K., Rahim, M., Abraham, A., Chyzhyk, D., Milham, M., Thirion, B., et al. (2019). Benchmarking functional connectome-based predictive models for resting-state fMRI. NeuroImage 192, 115–134. doi: 10.1016/j.neuroimage.2019.02.062
de Vries, F. E., de Wit, S. J., van den Heuvel, O. A., Veltman, D. J., Cath, D. C., van Balkom, A. J. L. M., et al. (2019). Cognitive control networks in OCD: a resting-state connectivity study in unmedicated patients with obsessive-compulsive disorder and their unaffected relatives. World J. Biol. Psychia. 20, 230–242. doi: 10.1080/15622975.2017.1353132
de Wit, S. J., Alonso, P., Schweren, L., Mataix-Cols, D., Lochner, C., Menchón, J. M., et al. (2014). Multicenter voxel-based morphometry mega-analysis of structural brain scans in obsessive-compulsive disorder. Am. J. Psychiatr. 171, 340–349. doi: 10.1176/appi.ajp.2013.13040574
Ding, X., Yang, Y., Stein, E. A., and Ross, T. J. (2015). Multivariate classification of smokers and nonsmokers using SVM-RFE on structural MRI images. Hum. Brain Map. 36, 4869–4879. doi: 10.1002/hbm.22956
Fan, S., Cath, D. C., van den Heuvel, O. A., van der Werf, Y. D., Schöls, C., Veltman, D. J., et al. (2017). Abnormalities in metabolite concentrations in tourette's disorder and obsessive-compulsive disorder-a proton magnetic resonance spectroscopy study. Psychoneuroendocrinology 77, 211–217. doi: 10.1016/j.psyneuen.2016.12.007
Fisher, R. A. (1915). Frequency distribution of the values of the correlation coefficient in samples from an indefinitely large population. Biometrika 10, 507–521. doi: 10.2307/2331838
Friedman, J., Hastie, T., and Tibshirani, R. (2001). The Elements of Statistical Learning: Springer Series in Statistics. New York, NY: Springer.
Gillan, C. M., Robbins, T. W., Sahakian, B. J., van den Heuvel, O. A., and van Wingen, G. (2016). The role of habit in compulsivity. Eur. Neuropsychopharm. 26, 828–840. doi: 10.1016/j.euroneuro.2015.12.033
Goodman, W. K., Grice, D. E., Lapidus, K. A., and Coffey, B. J. (2014). Obsessive-compulsive disorder. Psychiatr. Clin. North Am. 37, 257–267. doi: 10.1016/j.psc.2014.06.004
Gruner, P., Vo, A., Argyelan, M., Ikuta, T., Degnan, A. J., John, M., et al. (2014). Independent component analysis of resting state activity in pediatric obsessive-compulsive disorder. Hum. Brain Map. 35, 5306–5315. doi: 10.1002/hbm.22551
Guyon, I., Weston, J., Barnhill, S., and Vapnik, V. (2002). Gene selection for cancer classification using support vector machines. Machine Learn. 46, 389–422. doi: 10.1023/A:1012487302797
Hazari, N., Narayanaswamy, J. C., and Venkatasubramanian, G. (2019). Neuroimaging findings in obsessive-compulsive disorder: a narrative review to elucidate neurobiological underpinnings. Ind. J. Psychiat. 61, S9–S29. doi: 10.4103/psychiatry.IndianJPsychiatry_525_18
Hibar, D. P., Cheung, J. W., Medland, S. E., Mufford, M. S., Jahanshad, N., Dalvie, S., et al. (2018). Significant concordance of genetic variation that increases both the risk for obsessive-compulsive disorder and the volumes of the nucleus accumbens and putamen. British J. Psychiat. 213, 430–436. doi: 10.1192/bjp.2018.62
Hou, J. M., Zhao, M., Zhang, W., Song, L. H., Wu, W. J., Wang, J., et al. (2014). Resting-state functional connectivity abnormalities in patients with obsessive-compulsive disorder and their healthy first-degree relatives. J. Psychiatr. Neurosci. 39, 304–311. doi: 10.1503/jpn.130220
Kashyap, R., Eng, G. K., Bhattacharjee, S., Gupta, B., Ho, R., Ho, C. S. H., et al. (2021). Individual-fMRI-approaches reveal cerebellum and visual communities to be functionally connected in obsessive compulsive disorder. Sci. Rep. 11:1354. doi: 10.1038/s41598-020-80346-6
Kassraian-Fard, P., Matthis, C., Balsters, J. H., Maathuis, M. H., and Wenderoth, N. (2016). Promises, pitfalls, and basic guidelines for applying machine learning classifiers to psychiatric imaging data, with autism as an example. Front. Psychiatry 7:177. doi: 10.3389/fpsyt.2016.00177
Klöppel, S., Stonnington, C. M., Chu, C., Draganski, B., Scahill, R. I., Rohrer, J. D., et al. (2008). Automatic classification of MR scans in Alzheimer's disease. Brain J. Neurol. 131, 681–689. doi: 10.1093/brain/awm319
Kringelbach, M. L., and Rolls, E. T. (2004). The functional neuroanatomy of the human orbitofrontal cortex: evidence from neuroimaging and neuropsychology. Progr. Neurobiol. 72, 341–372. doi: 10.1016/j.pneurobio.2004.03.006
Ku, J., Kim, S. J., Lee, H., Jhung, K., An, S. K., Namkoong, K., et al. (2020). Deactivation of anterior cingulate cortex during virtual social interaction in obsessive-compulsive disorder. Psychiatr. Res. Neuroimag. 304:111154. doi: 10.1016/j.pscychresns.2020.111154
Lamothe, H., Baleyte, J. M., Smith, P., Pelissolo, A., and Mallet, L. (2018). Individualized immunological data for precise classification of OCD patients. Brain Sci. 8:80149. doi: 10.3390/brainsci8080149
Lee, Y. J., Koo, B. H., Seo, W. S., Kim, H. G., Kim, J. Y., and Cheon, E. J. (2017). Repetitive transcranial magnetic stimulation of the supplementary motor area in treatment-resistant obsessive-compulsive disorder: an open-label pilot study. J. Clin. Neurosci. 44, 264–268. doi: 10.1016/j.jocn.2017.06.057
Lin, X., Li, C., Zhang, Y., Su, B., Fan, M., and Wei, H. (2017). Selecting feature subsets based on SVM-RFE and the overlapping ratio with applications in bioinformatics. Molecules. 23:10052. doi: 10.3390/molecules23010052
Liu, F., Guo, W., Fouche, J. P., Wang, Y., Wang, W., Ding, J., et al. (2015a). Multivariate classification of social anxiety disorder using whole brain functional connectivity. Brain Struct. Funct. 220, 101–115. doi: 10.1007/s00429-013-0641-4
Liu, F., Wang, Y., Li, M., Wang, W., Li, R., Zhang, Z., et al. (2017). Dynamic functional network connectivity in idiopathic generalized epilepsy with generalized tonic-clonic seizure. Hum. Brain Map. 38, 957–973. doi: 10.1002/hbm.23430
Liu, F., Wee, C. Y., Chen, H., and Shen, D. (2014). Inter-modality relationship constrained multi-modality multi-task feature selection for Alzheimer's Disease and mild cognitive impairment identification. NeuroImage 84, 466–475. doi: 10.1016/j.neuroimage.2013.09.015
Liu, F., Xie, B., Wang, Y., Guo, W., Fouche, J. P., Long, Z., et al. (2015b). Characterization of post-traumatic stress disorder using resting-state fMRI with a multi-level parametric classification approach. Brain Topogr. 28, 221–237. doi: 10.1007/s10548-014-0386-2
Menzies, L., Chamberlain, S. R., Laird, A. R., Thelen, S. M., Sahakian, B. J., and Bullmore, E. T. (2008b). Integrating evidence from neuroimaging and neuropsychological studies of obsessive-compulsive disorder: the orbitofronto-striatal model revisited. Neurosci. Biobehav. Rev. 32, 525–549. doi: 10.1016/j.neubiorev.2007.09.005
Menzies, L., Williams, G. B., Chamberlain, S. R., Ooi, C., Fineberg, N., Suckling, J., et al. (2008a). White matter abnormalities in patients with obsessive-compulsive disorder and their first-degree relatives. Am. J. Psychiatr. 165, 1308–1315. doi: 10.1176/appi.ajp.2008.07101677
Milad, M. R., and Rauch, S. L. (2007). The role of the orbitofrontal cortex in anxiety disorders. Ann. N. Y. Acad. Sci. 1121, 546–561. doi: 10.1196/annals.1401.006
Miquel, M., Nicola, S. M., Gil-Miravet, I., Guarque-Chabrera, J., and Sanchez-Hernandez, A. (2019). A working hypothesis for the role of the cerebellum in impulsivity and compulsivity. Front. Behav. Neurosci. 13:99. doi: 10.3389/fnbeh.2019.00099
Morein-Zamir, S., Voon, V., Dodds, C. M., Sule, A., van Niekerk, J., Sahakian, B. J., et al. (2016). Divergent subcortical activity for distinct executive functions: stopping and shifting in obsessive compulsive disorder. Psychol. Med. 46, 829–840. doi: 10.1017/S0033291715002330
Mueller, S., Wang, D., Pan, R., Holt, D. J., and Liu, H. (2015). Abnormalities in hemispheric specialization of caudate nucleus connectivity in schizophrenia. J. Am. Med. Assoc. Psychiatr. 72, 552–560. doi: 10.1001/jamapsychiatry.2014.3176
Orrù, G., Pettersson-Yeo, W., Marquand, A. F., Sartori, G., and Mechelli, A. (2012). Using Support Vector Machine to identify imaging biomarkers of neurological and psychiatric disease: a critical review. Neurosci. Biobehav. Rev. 36, 1140–1152. doi: 10.1016/j.neubiorev.2012.01.004
Pedregosa, F., Varoquaux, G., Gramfort, A., Michel, V., Thirion, B., Grisel, O., et al. (2012). Scikit-learn: machine learning in python. J. Mac. Learn. Res. 12, 2825–2830.
Piacentini, J., Bergman, R. L., Keller, M., and McCracken, J. (2003). Functional impairment in children and adolescents with obsessive-compulsive disorder. J. Child Adolesc. Psychopharmacol. 13(Suppl.1), S61–S69. doi: 10.1089/104454603322126359
Piras, F., Piras, F., Caltagirone, C., and Spalletta, G. (2013). Brain circuitries of obsessive compulsive disorder: a systematic review and meta-analysis of diffusion tensor imaging studies. Neurosci. Biobehav. Rev. 37, 2856–2877. doi: 10.1016/j.neubiorev.2013.10.008
Plitt, M., Barnes, K. A., Wallace, G. L., Kenworthy, L., and Martin, A. (2015). Resting-state functional connectivity predicts longitudinal change in autistic traits and adaptive functioning in autism. Proc. Natl. Acad. Sci. U. S. A. 112, E6699–E6706. doi: 10.1073/pnas.1510098112
Rapinesi, C., Kotzalidis, G. D., Ferracuti, S., Sani, G., Girardi, P., and Del Casale, A. (2019). Brain stimulation in obsessive-compulsive disorder (OCD): a systematic review. Curr. Neuropharmacol. 17, 787–807. doi: 10.2174/1570159X17666190409142555
Rathore, S., Habes, M., Iftikhar, M. A., Shacklett, A., and Davatzikos, C. (2017). A review on neuroimaging-based classification studies and associated feature extraction methods for Alzheimer's disease and its prodromal stages. NeuroImage 155, 530–548. doi: 10.1016/j.neuroimage.2017.03.057
Rehn, S., Eslick, G. D., and Brakoulias, V. (2018). A meta-analysis of the effectiveness of different cortical targets used in repetitive transcranial magnetic stimulation (rTMS) for the treatment of obsessive-compulsive disorder (OCD). Psychiatr. Quart. 89, 645–665. doi: 10.1007/s11126-018-9566-7
Ruscio, A. M., Stein, D. J., Chiu, W. T., and Kessler, R. C. (2010). The epidemiology of obsessive-compulsive disorder in the National Comorbidity Survey Replication. Mol. Psychiatr. 15, 53–63. doi: 10.1038/mp.2008.94
Sajda, P. (2006). Machine learning for detection and diagnosis of disease. Ann. Rev. Biomed. Eng. 8, 537–565. doi: 10.1146/annurev.bioeng.8.061505.095802
Saxena, S., Brody, A. L., Schwartz, J. M., and Baxter, L. R. (1998). Neuroimaging and frontal-subcortical circuitry in obsessive-compulsive disorder. Br. J. Psychiat. 173, 26–37. doi: 10.1192/S0007125000297870
Sen, B., Bernstein, G. A., Tingting, X., Mueller, B. A., Schreiner, M. W., Cullen, K. R., et al. (2016). “Classification of obsessive-compulsive disorder from resting-state fMRI,” in 2016 38th Annual International Conference of the IEEE Engineering in Medicine and Biology Society (Orlando), 3606–3609. doi: 10.1109/EMBC.2016.7591508
Shenas, S. K., Halici, U., and Cicek, M. (2013). “Detection of obsessive compulsive disorder using resting-state functional connectivity data,” in Proceedings of the 2013 6th International Conference on Biomedical Engineering and Informatics, eds J. X. Gao, D. Xu, X. Sun, and Y. Wu (Chicago, IL), 132–136. doi: 10.1109/BMEI.2013.6746921
Shenas, S. K., Halici, U., and Çiçek, M. (2014). “A comparative analysis of functional connectivity data in resting and task-related conditions of the brain for disease signature of OCD,” in 2014 36th Annual International Conference of the IEEE Engineering in Medicine and Biology Society (Hangzhou), 978–981. doi: 10.1109/EMBC.2014.6943756
Smith, S. M., Miller, K. L., Salimi-Khorshidi, G., Webster, M., Beckmann, C. F., Nichols, T. E., et al. (2011). Network modelling methods for FMRI. NeuroImage 54, 875–891. doi: 10.1016/j.neuroimage.2010.08.063
Stein, D. J., Kogan, C. S., Atmaca, M., Fineberg, N. A., Fontenelle, L. F., Grant, J. E., et al. (2016). The classification of obsessive-compulsive and related disorders in the ICD-11. J. Affect. Disord. 190, 663–674. doi: 10.1016/j.jad.2015.10.061
Swedo, S. E., Schapiro, M. B., Grady, C. L., Cheslow, D. L., Leonard, H. L., Kumar, A., et al. (1989). Cerebral glucose metabolism in childhood-onset obsessive-compulsive disorder. Arch. Gen. Psychiatr. 46, 518–523. doi: 10.1001/archpsyc.1989.01810060038007
Szekely, G. J., Rizzo, M. L., and Bakirov, N. K. (2007). Measuring and testing dependence by correlation of distances. Ann. Stat. 35, 2769–2794. doi: 10.1214/009053607000000505
Takagi, Y., Sakai, Y., Lisi, G., Yahata, N., Abe, Y., Nishida, S., et al. (2017). A neural marker of obsessive-compulsive disorder from whole-brain functional connectivity. Sci. Rep. 7:7538. doi: 10.1038/s41598-017-07792-7
Thorsen, A. L., Hagland, P., Radua, J., Mataix-Cols, D., Kvale, G., Hansen, B., et al. (2018). Emotional processing in obsessive-compulsive disorder: a systematic review and meta-analysis of 25 functional neuroimaging studies. Biol. Psychiatr. Cogn. Neurosci. Neuroimag. 3, 563–571. doi: 10.1016/j.bpsc.2018.01.009
Tzourio-Mazoyer, N., Landeau, B., Papathanassiou, D., Crivello, F., Etard, O., Delcroix, N., et al. (2002). Automated anatomical labeling of activations in SPM using a macroscopic anatomical parcellation of the MNI MRI single-subject brain. NeuroImage 15, 273–289. doi: 10.1006/nimg.2001.0978
Varoquaux, G., Gramfort, A., Poline, J. B., and Thirion, B. (2010). Brain Covariance Selection: Better Individual Functional Connectivity Models Using Population Prior. Advances in Neural Information Processing Systems; 2010 2010-12-07; Vancouver, Canada. Available online at: https://hal.inria.fr/inria-00512451v4/document; https://hal.inria.fr/inria-00512451v4/file/paper.pdf (accessed September 27, 2021).
Vergun, S., Deshpande, A., Meier, T., Song, J., Tudorascu, D., Nair, V., et al. (2013). Characterizing functional connectivity differences in aging adults using machine learning on resting state fMRI data. Front. Comput. Neurosci. 7:38. doi: 10.3389/fncom.2013.00038
Wang, C., Xiao, Z., and Wu, J. (2019). Functional connectivity-based classification of autism and control using SVM-RFECV on rs-fMRI data. Phys. Med. 65, 99–105. doi: 10.1016/j.ejmp.2019.08.010
Xia, M., Wang, J., and He, Y. (2013). BrainNet Viewer: a network visualization tool for human brain connectomics. PLoS ONE 8:e68910. doi: 10.1371/journal.pone.0068910
Xue, K., Liang, S., Yang, B., Zhu, D., Xie, Y., Qin, W., et al. (2020). Local dynamic spontaneous brain activity changes in first-episode, treatment-naïve patients with major depressive disorder and their associated gene expression profiles. Psychol. Med. 2020, 1–10. doi: 10.1017/S0033291720003876
Yang, X., Luo, J., Zhong, Z., Yang, X., Yao, S., Wang, P., et al. (2019). Abnormal regional homogeneity in patients with obsessive-compulsive disorder and their unaffected siblings: a resting-state fMRI study. Front. Psychiatr. 10:452. doi: 10.3389/fpsyt.2019.00452
Yoo, K., Rosenberg, M. D., Noble, S., Scheinost, D., Constable, R. T., and Chun, M. M. (2019). Multivariate approaches improve the reliability and validity of functional connectivity and prediction of individual behaviors. NeuroImage 197, 212–223. doi: 10.1016/j.neuroimage.2019.04.060
Yoo, S. Y., Jang, J. H., Shin, Y. W., Kim, D. J., Park, H. J., Moon, W. J., et al. (2007). White matter abnormalities in drug-naïve patients with obsessive-compulsive disorder: a diffusion tensor study before and after citalopram treatment. Acta Psychiatr. Scand. 116, 211–219. doi: 10.1111/j.1600-0447.2007.01046.x
Zeng, L. L., Shen, H., Liu, L., Wang, L., Li, B., Fang, P., et al. (2012). Identifying major depression using whole-brain functional connectivity: a multivariate pattern analysis. Brain J. Neurol. 135, 1498–1507. doi: 10.1093/brain/aws059
Zhan, Y., Wei, J., Liang, J., Xu, X., He, R., Robbins, T. W., et al. (2021). Diagnostic classification for human autism and obsessive-compulsive disorder based on machine learning from a primate genemodel. Am. J. Psychiatr. 178, 65–76. doi: 10.1176/appi.ajp.2020.19101091
Zhang, H., Wang, B., Li, K., Wang, X., Li, X., Zhu, J., et al. (2019). Altered functional connectivity between the cerebellum and the cortico-striato-thalamo-cortical circuit in obsessive-compulsive disorder. Front. Psychiatr. 10:522. doi: 10.3389/fpsyt.2019.00522
Zhou, C., Cheng, Y., Ping, L., Xu, J., Shen, Z., Jiang, L., et al. (2018). Support vector machine classification of obsessive-compulsive disorder based on whole-brain volumetry and diffusion tensor imaging. Front. Psychiatr. 9:524. doi: 10.3389/fpsyt.2018.00524
Keywords: obsessive-compulsive disorder, functional connectivity, distance correlation, classification, rs-fMRI
Citation: Luo Q, Liu W, Jin L, Chang C and Peng Z (2021) Classification of Obsessive-Compulsive Disorder Using Distance Correlation on Resting-State Functional MRI Images. Front. Neuroinform. 15:676491. doi: 10.3389/fninf.2021.676491
Received: 05 March 2021; Accepted: 13 September 2021;
Published: 20 October 2021.
Edited by:
Sharlene D. Newman, University of Alabama, United StatesReviewed by:
Feng Liu, Tianjin Medical University General Hospital, ChinaShihui Ying, Shanghai University, China
Copyright © 2021 Luo, Liu, Jin, Chang and Peng. This is an open-access article distributed under the terms of the Creative Commons Attribution License (CC BY). The use, distribution or reproduction in other forums is permitted, provided the original author(s) and the copyright owner(s) are credited and that the original publication in this journal is cited, in accordance with accepted academic practice. No use, distribution or reproduction is permitted which does not comply with these terms.
*Correspondence: Chunqi Chang, Y3FjaGFuZyYjeDAwMDQwO3N6dS5lZHUuY24=; Ziwen Peng, cGVuZ3p3JiN4MDAwNDA7bS5zY251LmVkdS5jbg==
†These authors have contributed equally to this work