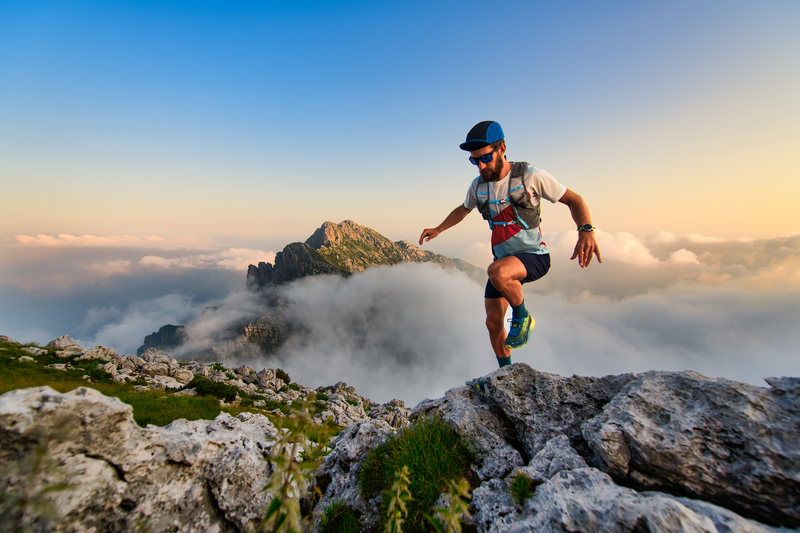
95% of researchers rate our articles as excellent or good
Learn more about the work of our research integrity team to safeguard the quality of each article we publish.
Find out more
ORIGINAL RESEARCH article
Front. Neuroimaging
Sec. Computational Neuroimaging
Volume 4 - 2025 | doi: 10.3389/fnimg.2025.1558759
This article is part of the Research Topic Multiscale Brain Modelling View all 4 articles
The final, formatted version of the article will be published soon.
You have multiple emails registered with Frontiers:
Please enter your email address:
If you already have an account, please login
You don't have a Frontiers account ? You can register here
Functional connectivity (FC) is a metric of how different brain regions interact with each other. Although there have been some studies correlating learning and memory with FC, there have not yet been, to date, studies that use machine learning (ML) to explain how FC changes can be used to explain behavior not only in healthy mice but also in mouse models of Alzheimer’s Disease (AD). Here, we investigated changes in FC and their relationship to learning and memory in controls and the APP/PS1 mouse model of AD at 3-, 6-, and 10-months of age. Using resting state functional Magnetic Resonance Imaging (rs-fMRI) in awake, unanesthetized mice, we assessed FC between 30 brain regions. In the APP/PS1 mice, we identified a pattern of hyperconnectivity across all three time points, with 47 hyperconnected regions at 3 months, 46 at 6 months, and 84 at 10 months. Notably, FC changes were also observed in the Default Mode Network (DMN), exhibiting a loss of hyperconnectivity over time. ML models were then used to define interactions between neuroimaging readouts and learning and memory performance, providing novel insights into how FC changes explain spatial learning and memory performance. These ML models show potential for early disease detection by identifying connectivity patterns associated with cognitive decline. Additionally, ML may provide a means to begin to understand how FC translates into learning and memory performance.
Keywords: Alzheimer's disease, RS-fMRI, functional connectivity, modeling, Behavior, mouse model
Received: 10 Jan 2025; Accepted: 04 Apr 2025.
Copyright: © 2025 Fadel, Hipskind, Pedersen, Romero, Ortiz, Shin, Samee and Pautler. This is an open-access article distributed under the terms of the Creative Commons Attribution License (CC BY). The use, distribution or reproduction in other forums is permitted, provided the original author(s) or licensor are credited and that the original publication in this journal is cited, in accordance with accepted academic practice. No use, distribution or reproduction is permitted which does not comply with these terms.
* Correspondence:
Hassan Samee, Baylor College of Medicine, Houston, 77030, Texas, United States
Robia G. Pautler, Baylor College of Medicine, Houston, 77030, Texas, United States
Disclaimer: All claims expressed in this article are solely those of the authors and do not necessarily represent those of their affiliated organizations, or those of the publisher, the editors and the reviewers. Any product that may be evaluated in this article or claim that may be made by its manufacturer is not guaranteed or endorsed by the publisher.
Research integrity at Frontiers
Learn more about the work of our research integrity team to safeguard the quality of each article we publish.