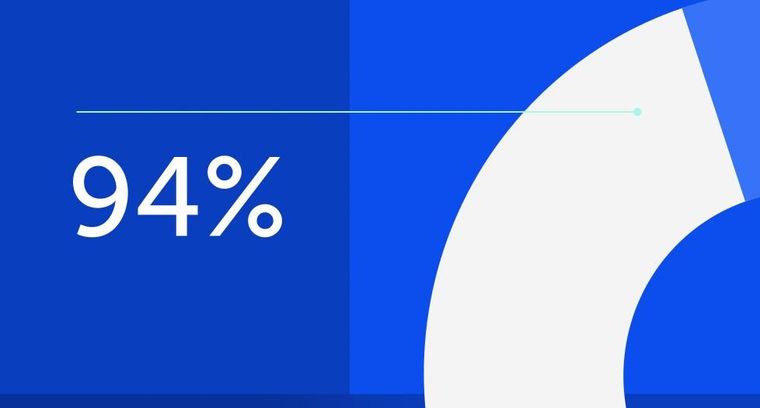
94% of researchers rate our articles as excellent or good
Learn more about the work of our research integrity team to safeguard the quality of each article we publish.
Find out more
ORIGINAL RESEARCH article
Front. Neuroimaging
Sec. Neuroimaging Analysis and Protocols
Volume 4 - 2025 | doi: 10.3389/fnimg.2025.1501801
This article is part of the Research TopicAutonomous Low-field Magnetic Resonance Imaging - Volume IIView all 3 articles
The final, formatted version of the article will be published soon.
Select one of your emails
You have multiple emails registered with Frontiers:
Notify me on publication
Please enter your email address:
If you already have an account, please login
You don't have a Frontiers account ? You can register here
Low-field magnetic resonance imaging (MRI ) experiences MRI is gaining interest, especially in low-resource settings, due to its low cost, portability, small footprint, and low power consumption.However, it suffers from significant noise, limiting its clinical utility. This study introduces native noise denoising (NND), which leverages the inherent noise characteristics of the acquired lowfield data. By obtaining the noise characteristics from corner patches of low-field images, we iteratively added similar noise to high-field images to create a paired noisy-clean dataset. A U-Net based denoising autoencoder was trained on this dataset and evaluated on three low-field datasets: the M4Raw dataset (0.3T), in vivo brain MRI (0.05T), and phantom images (0.05T).The NND approach demonstrated improvements in signal-to-noise ratio (SNR) of 67% , 3% , and 432.76% , 19.02% and 8.16% across the M4Raw, in vivo and phantom datasets, respectively.Qualitative assessments, including difference maps, line intensity plots, and effective receptive fields, suggested that NND preserves structural details and edges compared to random noise denoising (RND), indicating potential enhancements in visual quality. These findings imply that native noise modeling could be a promising strategy for improving This substantial improvement in low-field MRI image quality , warranting further investigation in clinical applicationsimaging quality addresses the fundamental challenge of diagnostic confidence in resource-constrained settings. By mitigating the primary technical limitation of these systems, our approach expands the clinical utility of low-field MRI scanners, potentially facilitating broader access to diagnostic imaging across resource-limited healthcare environments globally.
Keywords: Low-field MRI, MRI, denoising, native noise, machine learning (ML)
Received: 25 Sep 2024; Accepted: 10 Apr 2025.
Copyright: © 2025 Ssentamu, Kimbowa, Omoding, Atamba, Mukwaya, Jjuuko and Geethanath. This is an open-access article distributed under the terms of the Creative Commons Attribution License (CC BY). The use, distribution or reproduction in other forums is permitted, provided the original author(s) or licensor are credited and that the original publication in this journal is cited, in accordance with accepted academic practice. No use, distribution or reproduction is permitted which does not comply with these terms.
* Correspondence:
Tonny Ssentamu, King's College London, London, United Kingdom
Alvin Kimbowa, University of British Columbia, Vancouver, V6T 1Z2, British Columbia, Canada
Sairam Geethanath, Johns Hopkins University, Baltimore, 21218, Maryland, United States
Disclaimer: All claims expressed in this article are solely those of the authors and do not necessarily represent those of their affiliated organizations, or those of the publisher, the editors and the reviewers. Any product that may be evaluated in this article or claim that may be made by its manufacturer is not guaranteed or endorsed by the publisher.
Supplementary Material
Research integrity at Frontiers
Learn more about the work of our research integrity team to safeguard the quality of each article we publish.