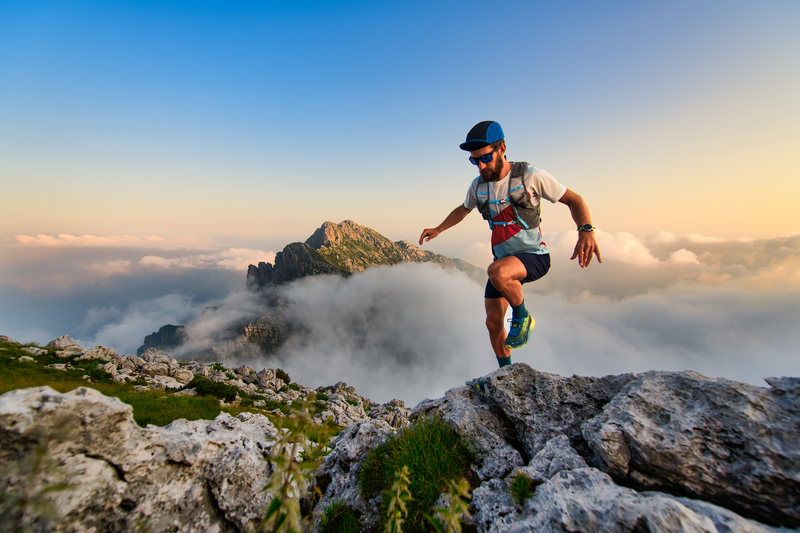
94% of researchers rate our articles as excellent or good
Learn more about the work of our research integrity team to safeguard the quality of each article we publish.
Find out more
SYSTEMATIC REVIEW article
Front. Neuroimaging , 17 December 2024
Sec. Clinical Neuroimaging
Volume 3 - 2024 | https://doi.org/10.3389/fnimg.2024.1481858
Objective: Resting-state functional MRI (rs-fMRI) may localize the seizure onset zone (SOZ) for epilepsy surgery, when compared to intracranial EEG and surgical outcomes, per a prior meta-analysis. Our goals were to further characterize this agreement, by broadening the queried rs-fMRI analysis subtypes, comparative modalities, and same-modality comparisons, hypothesizing SOZ-signal strength may overcome this heterogeneity.
Methods: PubMed, Embase, Scopus, Web of Science, and Google Scholar between April 2010 and April 2020 via PRISMA guidelines for SOZ-to-established-modalities were screened. Odd ratios measured agreement between SOZ and other modalities. Fixed- and random-effects analyses evaluated heterogeneity of odd ratios, with the former evaluating differences in agreement across modalities and same-modality studies.
Results: In total, 9,550 of 14,384 were non-duplicative articles and 25 met inclusion criteria. Comparative modalities were EEG 7, surgical outcome 6, intracranial EEG 5, anatomical MRI 4, EEG-fMRI 2, and magnetoencephalography 1. Independent component analysis 9 and seed-based analysis 8 were top rs-fMRI methods. Study-level odds ratio heterogeneity in both the fixed- and random-effects analysis was significant (p < 0.001). Marked cross-modality and same-modality systematic differences in agreement between rs-fMRI and the comparator were present (p = 0.005 and p = 0.002), respectively, with surgical outcomes having higher agreement than EEG (p = 0.002) and iEEG (p = 0.007). The estimated population mean sensitivity and specificity were 0.91 and 0.09, with predicted values across studies ranging from 0.44 to 0.96 and 0.02 to 0.67, respectively.
Significance: We evaluated centrality and heterogeneity in SOZ agreement between rs-fMRI and comparative modalities using a wider variety of rs-fMRI analyzing subtypes and comparative modalities, compared to prior. Strong evidence for between-study differences in the agreement odds ratio was shown by both the fixed- and the random-effects analyses, attributed to rs-fMRI analysis variability. Agreement with rs-fMRI differed by modality type, with surgical outcomes having higher agreement than EEG and iEEG. Overall, sensitivity was high, but specificity was low, which may be attributed in part to differences between other modalities.
• Heterogeneity in study-level rs-fMRI SOZ analysis methods and comparative SOZ-localizing modalities likely contributed to lack of study-level agreement odds ratios.
• Agreement in SOZ localization with rs-fMRI varied between modalities and within studies using the same modality, suggesting heterogeneity in agreement.
• The level of agreement in SOZ localization was higher for surgical outcome when compared to EEG and iEEG.
• Operationalizing resting-state fMRI (rs-fMRI) for epilepsy surgery is hindered by variability in analysis methods and validation benchmarks. Currently, only independent component analysis and accepted gold standards are validated at the meta-analysis level for seizure onset localization.
Epilepsy is a serious neurologic disorder affecting over 70 million people with a lifetime prevalence of 7.6 per 1,000 persons worldwide that is characterized by recurrent and spontaneous seizures (Fiest et al., 2014; Thijs et al., 2019). Epilepsy is associated with social stigma, multiple comorbidities, and a high economic burden (Fiest et al., 2014). Nearly 80% of people with this disorder live in low- and middle-income countries (Beghi, 2020), and many remain untreated (Fiest et al., 2014).
In 30% of drug-resistant epilepsy (DRE) cases, medications fail to achieve seizure control (Kalilani et al., 2018). Thus, surgical resection of the seizure onset zone (SOZ) is a potentially curative option, improving their quality of life (Kalilani et al., 2018; Engel et al., 2003; Jobst and Cascino, 2015). However, accurate localization of SOZ, the brain region of seizure origin, is vital for successful surgical outcomes (Fiest et al., 2014). Surgical intervention fails in 30–70%, depending on SOZ-localizing modalities used and epilepsy subtype (Bulacio et al., 2012; Engel, 2016; Gonzalez-Martinez et al., 2007; Laxer et al., 2014; Malmgren and Edelvik, 2017; McIntosh et al., 2004; Sillanpaa and Shinnar, 2010; Covidence systematic review software, n.d.). Despite the curative or palliative effects, surgery is an underutilized resource, potentially due to lack of or late provider referral, negative views on the likelihood of seizure freedom, and fears of associated risks (Thijs et al., 2019; Galan et al., 2021).
The non-invasive SOZ modalities include scalp electroencephalography (EEG), magnetoencephalography (MEG), positron emission tomography (PET), ictal single-photon emission computed tomography (SPECT), high-resolution magnetic resonance imaging (MRI), and resting-state functional MRI (rs-fMRI) (Thijs et al., 2019; Sheng et al., 2018). Contrastingly, invasive means of SOZ localization use electrodes on the surface or inserted into brain tissue, termed intracranial EEG (iEEG) or stereo encephalography (SEEG).
EEG is useful for localizing the SOZ based on the pattern and location of electrical signals; however, the most common form, scalp EEG, is limited by relatively poor spatial resolution and is needed to capture ictal activity. Simultaneous EEG-fMRI can provide improved spatial resolution compared to scalp EEG but also requires specialized equipment. Ictal SPECT studies utilize tracers to identify the SOZ as regions of hyper-perfusion and may be combined with interictal images and overlayed with MRI (Perissinotti et al., 2018). Similarly, combined acquisition of PET/MRI has been shown to improve identification of epileptogenic foci compared to PET alone (Boscolo Galazzo et al., 2016; Khalaf et al., 2022). One major limitation of both EEG and SPECT is that they require a patient to be actively seizing to effectively localize the SOZ. MEG detects the magnetic fields generated by neuronal electrical currents but is primarily limited in its ability to detect deeper brain structures frequently associated with DRE (Vivekananda et al., 2021). aMRI can identify structural abnormalities leading to epileptic activity but does not delineate the epileptic activity.
When non-invasive investigations fail or need verification to accurately identify the SOZ, invasive diagnostic modalities such as iEEG are often necessary. iEEG involves a subdural grid or strip electrodes to capture activity from relatively large surfaces of the brain or depth electrodes that can reach the deep brain structures (Burneo et al., 2006; Grande et al., 2020; Jayakar et al., 2016; Nagahama et al., 2018). iEEG is the current gold standard for presurgical SOZ localization; however, its invasive nature results in higher mortality and morbidity than non-invasive methods (Chakraborty et al., 2020). While morbidity rates have decreased over time, patients would benefit from a more reliable non-invasive method that could reduce the regions requiring iEEG confirmation or increase the likelihood of placing SEEG in the true SOZ.
Comparatively, rs-fMRI is both non-invasive and can be performed during the interictal period. Rs-fMRI is an emerging modality that can be used to detect the SOZ and map the surrounding eloquent brain areas. Due to its non-invasiveness and potential for whole brain network detection, rs-fMRI has delineated pathophysiology of various neurologic and psychiatric disorders (Cha et al., 2015; Gong et al., 2020; Ibrahim et al., 2021; Lau et al., 2019; Pini et al., 2020). Rs-fMRI assesses functional connectivity through the detection of variation of blood-oxygen-level-dependent (BOLD) signals that reflect differences in neural activity (Matthews and Jezzard, 2004).
There are various methods for assessing rs-fMRI, and it is unknown which are most useful for SOZ localization. For example, independent component analysis (ICA), which is a data-driven method, yields the whole brain network profile. Contrastingly, there are hypothesis-driven methods, such as seed-based correlation (SBC) maps, which evaluate only those regions pre-determined to be of interest (Metwali and Samii, 2019; Rosazza et al., 2014; Rosazza and Minati, 2011). Other commonly used methods for localizing activity include regional homogeneity (ReHo) and amplitude of low-frequency fluctuation (ALFF)/fractional ALFF (fALFF) (Zang et al., 2015). ReHo measures the synchronization of neighboring voxels, and ALFF/fALFF measures the amplitude of time series fluctuations from regions of interest (Zang et al., 2015; Zang et al., 2004; Zou et al., 2008). Further advantages and limitations in general of the various rs-fMRI signal processing methods are reported (Cole et al., 2010).
Armed with such methods, there has been an emergence of rs-fMRI in clinical settings (Anzellotti et al., 2010; Chen et al., 2017; Khoo et al., 2019; Reyes et al., 2016; Zhao et al., 2019). However, there are few large or meta-studies validating rs-fMRI findings in epilepsy compared to controls or other pathology (Zang et al., 2015). Furthermore, there is only one meta-analysis evaluating the localization of seizure networks for the purpose of epilepsy surgery evaluation by Chakraborty et al. (2020). Their study compared the SOZ localization by rs-fMRI with ICA and primary component analysis (PCA) to that by intracranial EEG or epilepsy surgery outcomes, finding non-inferiority of rs-fMRI. While positive agreement in SOZ localization between rs-fMRI and the comparative modalities was found, the study also found moderate heterogeneity in agreement between studies.
Thus, the first goal of this study is to evaluate the validity of rs-fMRI in localizing the SOZ, by comparing it to other established SOZ-localizing modalities across a diverse set of analysis approaches and validation standards. The second goal is to evaluate the clinical relevance of rs-fMRI for pre-surgical planning by evaluating its agreement with surgical outcomes. With these two goals, this study aims to clarify the role of rs-fMRI in epilepsy surgery and provide further insights into its potential as a valid, non-invasive modality for SOZ localization. We hypothesized that the underlying BOLD–epilepsy neuronal relationship may exhibit a sufficiently large signal to overcome the heterogeneity introduced by both the choice of rs-fMRI analysis method and the physiological properties of the validating SOZ modalities.
A literature review was conducted using the Preferred Reporting Items for Systematic Reviews and Meta-Analyses (PRISMA) guidelines. PubMed, Embase, Scopus, Web of Science, and the first 100 results of Google Scholar were evaluated up to 22 April 2020. The search was limited to publications after 2000. Terms queried consisted of variations of resting-state fMRI, epilepsy, seizure, irritative zone, network mapping, lateral, local, and connectivity network, resulting in n = 14,382. Two additional resources were added by hand searching. The articles were loaded into Covidence (Veritas Health Innovation),1 an online software designed to streamline literature reviews for meta-analyses. After duplicates were removed, 9,550 articles remained. The abstracts and full-text articles were each screened by two of ten independent reviewers, and discrepancies were reviewed by one of two independent reviewers blinded to the original votes as a tiebreaker. Within Covidence, each article was then randomly assigned to the independent interviewers.
Study inclusion criteria consisted of (1) primary research, (2) involving human patients with DRE, (3) patients who underwent rs-fMRI, and (4) the rs-fMRI results were compared to another SOZ-localizing modality. Exclusion criteria were non-peer-reviewed publication abstracts with n < 10 (if published then any number of subjects were allowed), studies written in a language other than English, unavailable subject level SOZ data, duplicate populations with another included article (with exception as detailed in the results that also have novel population), or studies that did not focus on localizing the SOZ.
Each study was analyzed for SOZ localization by a prior non-rs-fMRI established modality, SOZ localization by rs-fMRI, and surgical outcomes, if applicable. The comparative modalities include anatomical MRI, EEG, EEG-fMRI, MEG, iEEG, and surgical outcomes. When a study used multiple comparative modalities, only one primary modality was selected for analysis. The selected modality had to meet the following criteria: (1) It provided usable results for all study participants, and (2) it was described by the authors as the primary modality that influenced the surgical target location. In cases where no primary modality was specified, the comparative modalities were chosen in the following category order: (1) surgical outcomes, (2) iEEG, (3) EEG-fMRI, EEG, MEG, or anatomical MRI. In the case of the third category, the modality with the most detailed subject level data and the most specific brain location information was selected. Applying this process returned the following distribution of primary comparative modalities: iEEG/EEG (193 cases, 43%), surgical outcomes (154 cases, 34%), and anatomical MRI (80 cases, 18%).
True positives (TP) were defined as the SOZ, and rs-fMRI and comparative modality localized to the same place. False positives (FP) were defined as when the rs-fMRI localized a SOZ where a comparative modality did not detect a SOZ. False negatives (FN) were defined as when the comparative modality localized to a different location OR the comparative modality localized a SOZ while rs-fMRI did not detect any. True negatives (TN) were defined as when both rs-fMRI and the comparative modality did not localize a SOZ. For each study, the counts of the TP, FP, FN, and TN were extracted and tabulated into 2×2 tables, with rs-fMRI as the rows and the comparative as the columns (Table 1).
If surgical outcomes were available, which is considered the highest level of proof of validity of SOZ, then SOZ TP, FP, TN, and FN were defined according to Boerwinkle et al. (2017) as summarized in Table 2.
The Quality Assessment of Diagnostic Studies (QUADAS-2) checklist was used to assess the quality of included articles (Whiting et al., 2011). A 0 denotes low risk, a 1 denotes high risk, and a 2 denotes an uncertain risk. These findings are displayed in Table 3, with final expert review denoted in the caption.
The odds ratio was used to quantify the agreement between rs-fMRI and the comparative modalities. An odds ratio larger and less than 1 implies agreement and disagreement, respectively, while an odds ratio of 1 implies that the modalities are independent. Two types of models were used, namely, fixed-effects models and random-effects models (Borenstein et al., 2010). Differences in odd ratios between studies are viewed as systematic differences in fixed-effects models but as random variation in random-effects models.
Each study was summarized into a 2×2 table. In the fixed-effects analysis, odds ratios that quantify the agreement between rs-fMRI and the comparative, with exact confidence intervals (CIs), were estimated based on the conditional likelihood. Only 10 studies that had non-zero margins were used in the fixed-effects analysis. In five studies, one cell was zero, and median unbiased estimates were obtained. The heterogeneity of the odd ratios was tested using the likelihood ratio test. As this test requires a large sample in each study, it was restricted to the seven studies with at least 20 subjects and no zero margins (29 to 64 patients per study, 298 patients in total). Sensitivities and specificities from the individual studies were calculated with their respective 95% CI intervals.
Among the 7 studies with at least 20 subjects and no zero margins, differences in agreement with rs-fMRI across modalities were evaluated using a four-level categorization of modality: Anatomical MRI, EEG, iEEG, and surgical outcome. Differences in agreement with rs-fMRI between modalities (three comparisons) and between studies within modalities (three comparisons) were then evaluated. Differences between and within modalities were evaluated using a likelihood ratio test, and significance was evaluated using a p-value threshold of 0.05.
The random-effects model included all studies (n = 25) and was a generalized mixed model with three independent random effects per study: one for each marginal log odds (rs-fMRI and comparative) and one for the log odds ratio. The predicted study-specific odds ratios and their respective 95% prediction intervals (PI) were computed. The heterogeneity of odd ratios was tested using the likelihood ratio test. Sensitivities and specificities from the individual studies were calculated with their respective 95% PI intervals. SAS software version 9.4/15.2 was used. Data and code are available in an open repository.2
The systematic literature review resulted in 25 studies qualifying for inclusion (Figure 1). Reasons and quantities for study exclusion are delineated in Figure 1. Of the three Hunyadi et al. (2014, 2015a, 2015b) publications, there is potential for unconfirmed population overlap. However, there is clear evidence of unique participant data as the comparative modalities used in each were different (e.g., EEG, EEG-fMRI, and surgical outcome). Based on these distinctions, we concluded that the subjects were unique across studies.
Figure 1. PRISMA flow diagram for the current meta-analysis. *Animal studies, patients without epilepsy; **study design (n = 141), indication (n = 25), comparator (n = 12), publication type (n = 20), intervention (n = 9), outcomes (n = 9), patient population (n = 4), setting (n = 3), language (n = 4), manuscript unavailable (n = 11), duplicate paper (n = 8), duplicate population (n = 14), and unavailable subject level SOZ data (n = 52).
The inter-rater reliability data of the reviewers’ evaluation of the abstract of the publications are provided in Table 4. Inter-rater reliability of the publications reflected overall good agreement between raters, with proportionate agreement between 0.7 and 1 for all but one rater pairing at 0.33, and random agreement probability between 0.88–1.0 for all but two rater pairings at 0.55 (Table 4). Cohen’s Kappa coefficient range was −0.5 to 0.47, which is low. However, this ratio’s value was indeterminate in six rater pairings; thus, proportionate agreement was determined to be more informative of the study findings.
Of the 337 publications with full-text review, 312 were excluded, with the most common reason being study design (141) not matching with the study question, which required a known comparator on a 1:1 basis for the rs-fMRI SOZ.
The most common rs-fMRI analysis methods were ICA and SBC, utilized in nine and eight studies, respectively. For studies not meeting inclusion criteria, the analysis methods are in Table 5. The analyses were categorized as “data-driven” if the whole brain was investigated and “hypothesis-driven” if the study investigated a reduced set of regions of interest. The comparative modalities included aMRI, EEG, iEEG, EEG-fMRI, MEG, and surgical outcomes.
From the remaining 25 included studies, 452 rs-fMRI SOZ patient comparisons were evaluated. The age range was 18 months to 66 years old (Table 6). Six studies included children: Lee et al. (2014), Reyes et al. (2016), Boerwinkle et al. (2017), Chen et al. (2017), Boerwinkle et al. (2019), and Zhao et al. (2019).
Table 6. Demographics, rs-fMRI software, and comparative modality used in studies included in the meta-analysis.
Of the studies utilizing imaging modalities to define SOZ truth, two counted participants more than once (which is well accepted given the method explanation): Bettus et al. (2010) and Khoo et al. (2019). Bettus et al. (2010) evaluated the left and right hemisphere separately. Khoo et al. (2019) evaluated heterotopic nodular pairs within patients separately. Of the studies utilizing surgical outcomes to define ground truth, each surgery counted as a separate comparison event for corresponding pre-operative rs-fMRI (Boerwinkle et al., 2019). They investigated 64 surgical outcomes among 58 participants, four participants had two surgeries, and one participant had three surgeries.
Per the QUADAS-2 Bias Assessment Tool, there are four domains of bias: patient selection, index test, reference test, and flow and timing (Whiting et al., 2011), with results as delineated in Table 3. According to QUADAS-2 guidelines, to qualify for downgrading evidence, a publication must have two domains with high-risk bias. None of the included publications had bias meeting this level of concern. Further, of the areas rated as high risk, two were overturned by further expert review as described below.
For the patient selection domain, seven studies were rated as high risk due to selection of patients that was not well specified, such as being in consecutive order or provided reasoning otherwise. One had concern for population type (Whiting et al., 2011). This study validated rs-fMRI SOZ to that of SEEG in DRE due to multiple heterotopic nodules. Thus, the patient selection does match the study question by expert review, though the assigned reviewers rated it otherwise. The rs-fMRI connectivity between the heterotopias corresponded to that found by seizure and epileptiform activity by SEEG. Thus, while the two reviewers who evaluated this specific study in 28 consecutive patients were concerned if the comparison lined up with the study question, the expert review consensus is rated as low risk, although again the original finding is provided.
In the reference test domain, one study was of high risk (Whiting et al., 2011). In this study, six patients who underwent SEEG and rs-fMRI were analyzed by predefined metrics. Thus, it is not clear why our interpreters rated this study as possibly biased since the methods removed expert opinion from interpretation. Because of this, in retrospect, our expert overall rating is no concern, although the original results are provided in the table.
Of the 25 studies included in the study, 9 had a sample size of 20 or more subjects. While 10 had no zero margins, 6 had one zero margin, and 9 had two zero margins. Only 7 studies had a sample size over 20 and no zero margins. A single zero margin indicates that either one row or one column total is zero as presented in Table 1. The presence of two zero margins implies that only one of the four cell counts was not zero. For example, Anzellotti et al. (2010) had a sample size of one, resulting in only one non-zero cell. Thus, the number of studies with the respective comparative modalities was EEG 7, surgical outcome 6, iEEG 5, aMRI 4, EEG-fMRI 2, and MEG 1. Of the 9 studies with greater than 20 subjects, patients were more often in the adult than pediatric age range, and 5 studies used the SPM software for analysis, whereas 3 studies used FSL; and, of these, 5 took a hypothesis-based approach (meaning evaluating only certain regions of interest), whereas 4 were data-driven (meaning evaluating the entire brain).
In the fixed-effects analysis, the odds ratio estimates for the 10 studies with no zero margins ranged between 0.08 and 222 (Figure 2). Only three studies have 95% CI above 1. Only one study, Hunyadi et al. (2015a), had an interval below 1 indicating disagreement between rs-fMRI SOZ and the comparative modality. The heterogeneity of the odds ratios was significant indicating high evidence in the favor of heterogeneity (p < 0.001). The estimated common odds ratio is 8.0 with a 95% confidence interval of 4.31 and 14.90. However, the usefulness of this single estimate is highly questionable because there is strong evidence against the existence of a common odds ratio. The overall sensitivity was 0.87, and the study estimates varied between 0.36 to 1.00 (Figure 3). In comparison, the overall specificity was 0.58, and the study estimates varied between 0.00 and 0.92 among studies with no-zero margins. Estimated specificities were always lower than sensitivities. Boerwinkle et al. (2019) had both a high sensitivity (0.96) and specificity (0.93) estimate.
Figure 2. Fixed-effects analysis odd ratios. Each study was summarized into a 2×2 table, and conditional likelihood-based estimates were obtained if there are no zero cells. In five studies, one cell was zero, and median unbiased estimates were obtained (as marked by *). If any margin is zero, the study is non-informative. This left the 10 studies shown in this figure. The analysis presented very strong evidence against the existence of a common odds ratio. Red line indicates an odds ratio of 1 of no association.
Figure 3. Fixed-effects analysis sensitivities and specificities. Each study was summarized into a 2×2 table. Sensitivities and specificities were calculated, and exact 95% confidence intervals were obtained.
Among the seven studies with no-zero margins and sample size above 20, the counts of anatomical MRI, EEG, iEEG, and surgical outcome were 1, 2, 2, and 2, respectively (total: 298 subjects). Positive agreement with rs-fMRI was observed with anatomical rs-fMRI (p-val = 0.04), iEEG (p-val = 0.01), and surgical outcome (p-val < 0.001) (Table 7). The fixed-effects analysis showed marked differences in agreement with rs-fMRI between modalities (p = 0.005), as well as differences in agreement between studies using the same modality (p = 0.002). That is, there is strong evidence of differences in agreement with rs-fMRI across the four modalities and across studies within each modality. Moreover, the level of agreement between rs-fMRI and the comparative was highest for surgical outcome with an odds ratio of 48.0 (95% CI: 10.4–334), higher than agreement between rs-fMRI and EEG (p = 0.002) and between rs-fMRI and iEEG (p = 0.007), but not significantly different from agreement between rs-fMRI and anatomical MRI (p = 0.173). The considerable difference in estimated agreement between Boerwinkle et al. (2017) (OR 1.7) and Boerwinkle et al. (2019) (OR 222), two studies sharing the same modality, highlights the heterogeneity of agreement between studies within each modality (Table 7). This suggests the possibility of experience level increasing expert-derived SOZ and post-operative seizure outcome concordance.
Table 7. Fixed effects analysis of agreement heterogeneity within and between modalities (7 Studies, 298 subjects).
For the random-effects analysis, the population median odds ratio was estimated to be 1.007 (95% PI: 0.003–361.41), and the predicted odd ratio estimates for all 25 studies ranged from 0.04 to 147 (Figure 4). As in the fixed-effects analysis, the random-effects analysis identified the same three studies with a 95% PI above 1. In addition, it is estimated that, in the underlying population of studies, the log odds ratio is centered approximately 0.0069 (95% CI: −2.59 to 2.60) with a standard deviation of 3. This implies, for example, that the population quartiles of log odds ratio are −2 and + 2, a 54-fold increase in the agreement odds ratio going from the first to the third quartile which is indicative of considerable between-study heterogeneity. The null hypothesis of no population heterogeneity among the odds ratios is rejected (p = 0.001). These results suggest that the agreement between rs-fMRI and comparative modalities varies widely across studies. Most studies resulted in odds ratios close to 1 in both the fixed-effects and random-effects analyses. However, Boerwinkle et al. (2019) stood out with the largest estimated odds ratios, with values of 222 in the fixed-effects analysis and 148 in the random-effects analysis.
Figure 4. Random-effects analysis odd ratios. Random-effects model includes a random study-specific log odds ratio measuring the agreement between fs-MRI and the comparative. Predicted study-specific odds ratios and prediction intervals are depicted. The study-specific odds ratios, assumed normally distributed, are estimated to have a mean (and median) of 1.007 and with a standard deviation of 3. The analysis presented very strong evidence against the existence of a common odds ratio.
The median probability of a positive rs-fMRI is 0.91, and 95% of studies fall between 0.33 and 0.995. The analogous estimates for the comparative are 0.87 and 0.38 to 0.985. That is, rs-fMRI and the comparative have similar levels of positive detection. It is worth noting that this similarity has no bearing on agreement or disagreement. Moreover, the population mean sensitivity is 0.91 and the predicted sensitivities for all 25 studies ranged between 0.44 and 0.96. The population mean for specificity is lower at 0.09, and the predicted values varied, ranging between 0.02 to 0.67 (Figure 5). Most studies had large sensitivities and low specificity estimates with wide prediction intervals.
Figure 5. Random-effects sensitivities and specificities. Random-effects model includes a random study-specific log odds ratio measuring the agreement between fs-MRI and the comparative. Predicted study-specific odds ratios and prediction intervals are depicted. The study-specific sensitivity and specificity assumed normality are assumed to have a mean of 0.91 and 0.09, respectively.
We expanded upon the prior meta-analysis of rs-fMRI SOZ by ICA/PCA validated by surgical outcomes or iEEG that found a common odds ratio of 2.63 (95% CI: 0.66–10.6) (Nagahama et al., 2018), by removing all restrictions and characterizing the broader library of rs-fMRI SOZ analysis and extent of validation modality subtypes. We evaluated overall agreement and then investigated whether this agreement differed between modality groups and within studies under each modality. The overall evaluation was geared to help us understand what the effect size of rs-fMRI agreement was overall regardless of comparator, the between modality evaluation could help identify if and which types of modalities had the highest agreement with rs-fMRI, and the within modality evaluation would help us understand if significant differences in agreement between studies sharing the same modality were present. In addition, we tested the heterogeneity of odd ratios to evaluate the validity of a common odds ratio to describe overall agreement of SOZ localization between rs-fMRI and the comparators across studies.
The fixed-effects analysis presented some indicators of SOZ agreement. (1) The study-specific common odds ratio was estimated to be 8.00 (95% CI: 4.3–14.9). Nonetheless, the usefulness of this single estimate is highly questionable because there is strong evidence against the existence of a common odds ratio (p < 0.001). (2) Out of the 10 studies with no zero margins included in the fixed-effects analysis, 8 studies estimated positive agreement, and 2 studies estimated negative agreement. (3) In the 4 largest studies, those with greater than 40 subjects, the odd ratio estimates, and the respective 95% confidence intervals were above 1. (4) Only one of the 25 included studies showed poor agreement between rs-fMRI and a comparator. Hunyadi et al. (2015a) found that rs-fMRI localized the SOZ to a different location than their comparative modality, surgical outcome, more often than it localized the SOZ to the same location (Hunyadi et al., 2015a). This Hunyadi et al. (2015a) study required that participants have an EEG-fMRI negative for localizing information, so this is a potential explanation for the lack of helpful information from a similarly BOLD-dependent modality (Hunyadi et al., 2015a). Though with it being such a relatively small study, caution to any conclusion is warranted. (5) Finally, somewhat spuriously different than the other studies, but in the opposite direction, Boerwinkle et al. (2019) had a relatively high odds ratio. A few key implementation aspects may yield some insight into this difference. One is that this study came from a repeat first author in relatively larger studies, implying possible influence of learned expertise effect. However, there is also the possibility of bias, though this is contradicted by a separate lab validating subjects from the same dataset using fully automated SOZ detection method with deep learning and artificial intelligence (Banerjee et al., 2023).
The random-effects analysis estimated a population median odds ratio of 1.007 (95% PI: 0.003–361.41). The hypothesis of homogeneity was strongly rejected (p-value = 0.001), arguing against the existence of a true common odds ratio. The prior meta-analysis estimated positive agreement in SOZ localization between rs-fMRI and the comparators (Chakraborty et al., 2020) using a random-effects model and found moderate, yet not significant (p = 0.162), heterogeneity in odd ratios. The added scope of rs-fMRI SOZ analysis methods, validation modalities, and wide range of patient demographics across studies may have contributed to the increased evidence against a common odds ratio. One source of such study-level heterogeneity is evidenced by the relatively wide array of rs-fMRI SOZ analysis methods, and even within the same investigator over time a difference potentially due to increasing experience. Notably, there could be an early emerging trend of convergence as the two most frequently used in recent larger studies were the data-driven ICA and hypothesis-driven SBC. This is not to say there is evidence of superiority between the analysis methods. Furthermore, we posit that each method has its strengths and, when used appropriately in isolation or combination, may improve SOZ localization and surgical outcomes. For example, to verify whether comparative modalities’ SOZ candidates contain the true SOZ, ICA may be an ideal initial search approach since it may hone in on a smaller cadre of region(s). Then, hypothesis-driven techniques, such as SBC or effective connectivity, may further narrow the SOZ candidates (Grande et al., 2020; Jayakar et al., 2016; Tavares et al., 2017; Chassoux et al., 2018; Mullin et al., 2016).
There were indicators of differences in SOZ localization agreement between rs-fMRI and the modality groups. (1) The fixed-effects analysis demonstrated high evidence of systematic differences in agreement between rs-fMRI across modalities with the level of agreement being higher for surgical outcome when compared to EEG (p = 0.002) and iEEG (p = 0.007). (2) In addition, the analysis presented high evidence of systematic differences in agreement between rs-fMRI and the comparator between studies sharing the same modality. These results suggest that while an overall positive agreement was determined by the fixed-effects analysis, the level of agreement varied across modalities, and even between studies using the same modality.
The random-effects model estimated a population mean sensitivity of 0.91. These results indicate that there is a high probability of the rs-fMRI localizing the SOZ given that the comparative modality also localized the SOZ. In contrast, the estimated population mean specificity was 0.09 which indicates a low probability of rs-fMRI not localizing the SOZ given that the comparative modality also did not localize the SOZ. Similarly, the study-specific predicted sensitivities were always higher than the predicted specificities. Similar results were observed in the fixed-effects analysis.
Strengths of this study include the wide inclusion of rs-fMRI SOZ analysis methods and extent of validation modality subtypes. In addition, this study is the first meta-analysis to investigate the sensitivity and specificity of rs-fMRI for seizure onset localization. However, there are several limitations to consider.
First, while the comparative localizing modalities are established as standards of care, this study evaluated concurrent validity, which measures the level of agreement between two or more instruments in assessing the same outcome as opposed to other measures of validity (Higgins and Straub, 2006). Second, nearly half of the studies included in our analysis had small sample sizes (n < 10), which contributes to low precision and non-estimable odds ratios, and may limit the reliability of our pooled sensitivity and specificity estimates. The predominance of small case studies also raises the possibility of publication bias, as studies with positive or significant results are more likely to be published, potentially skewing the overall findings. This publication bias could result in an overrepresentation of positive outcomes, which may artificially inflate sensitivity and specificity values for rs-fMRI’s accuracy in SOZ localization.
Third, heterogeneity across studies represents a significant limitation. The heterogeneity test for study-level odds ratios in both random- and fixed-effects analyses yielded statistically significant results, suggesting that the studies included in the meta-analysis exhibit heterogeneity and provide strong evidence that a study-level common odds ratio does not exist. This could be due to multiple factors including the inclusion of multiple comparative modalities, different rs-fMRI software, seizure etiology, and patient populations. Furthermore, our QUADAS-2 analysis indicated that 12 out of 25 studies had an uncertain or high risk of bias in at least one domain; these studies were retained in our comparisons as excluding them could have further limited our dataset. However, these high-risk studies may have introduced additional bias, impacting the robustness of our meta-analysis.
Furthermore, this analysis included only studies published up to 2019, which excludes recent advancements in rs-fMRI methodologies. Since 2019, newer techniques such as improved data acquisition, processing algorithms, and network-targeted approaches have emerged, potentially enhancing the clinical applicability of rs-fMRI for SOZ localization. Future meta-analyses incorporating these newer methodologies will be critical to validate and extend our findings as rs-fMRI evolves as a tool for SOZ localization. Additionally, with time the ILAE surgical outcome classification system has evolved to become the most broadly recognized system to compare surgical outcomes. Our study is limited by retrospective collection of surgical outcomes classified under the Engel approach, which may be improved in the future by transition to the ILAE approach.
While this is the largest meta-analysis to date evaluating the agreement of SOZ localization between rs-fMRI with other localizing modalities, the overall number of studies remains limited. Therefore, it is important to interpret these results as guidance for future validation studies of rs-fMRI.
Studies are needed to validate the use of rs-fMRI in localizing SOZ with comparative modalities, with special consideration to modalities that have high accuracy such as iEEG and surgical outcomes. Evaluating the convergent validity of rs-fMRI against gold-standard modalities may allow clinicians to localize SOZ while minimizing the degree of invasive iEEG verification risks. In addition, rs-fMRI is an increasingly accessible imaging modality as more healthcare centers acquire three Tesla MRI machines and the images can be sent to experts for analysis, or possibly fully automated SOZ localization (Jayakar et al., 2016). This compares to methods such as iEEG, which requires on-site expertise for electrode placement. Rs-fMRI could benefit patients in resource-deprived communities by providing them with increased access to expertise. Unfortunately, studies evaluating the convergent validity of rs-fMRI with modalities such as iEEG are limited due to the invasive nature of gold-standard procedures, limited funding and resources such as insurance reimbursement (Young et al., 2023), access to the expertise of advanced modalities, and difficulties related to the recruitment of individuals willing to participate in these studies.
Accurate presurgical localization of SOZ is important as it is a determining factor in the success of treating epilepsy for patients undergoing surgical resection. Rs-fMRI is an emerging modality for localizing SOZ, but limited research exists evaluating its agreement with other established localizing modalities, especially when considering a wide variety of rs-fMRI analysis methods and comparative modalities. This meta-analysis considered 25 studies that compared agreement in SOZ localization between rs-fMRI and other localizing modalities, including a wider range of rs-fMRI analysis subtypes and verification modalities than previous meta-analysis studies. Using the odds ratio as a measure of within-study agreement, the data provide strong evidence for considerable variation in agreement between rs-fMRI and the other modalities across studies. Agreement with rs-fMRI was highest for surgical outcomes, followed by EEG and iEEG. In addition, there is significant variation in agreement between studies using the same modality. A high sensitivity and a low specificity of SOZ location for rs-fMRI when compared to the comparative were also found. While this is the largest meta-analysis evaluating the agreement of SOZ localization between rs-fMRI with other localizing modalities to date, the number of studies included in the analysis is small; therefore, it is important to use the results as guidance for future validation studies of rs-fMRI.
The datasets presented in this study can be found in online repositories. The names of the repository/repositories and accession number(s) can be found in the article/supplementary material.
VB: Conceptualization, Data curation, Formal analysis, Investigation, Methodology, Project administration, Resources, Supervision, Validation, Writing – original draft, Writing – review & editing. MN: Conceptualization, Data curation, Methodology, Supervision, Validation, Writing – original draft, Writing – review & editing. JV: Formal analysis, Writing – original draft. MA: Validation, Writing – original draft. WR: Project administration, Validation, Visualization, Writing – original draft, Writing – review & editing. EG: Validation, Writing – original draft. PM: Conceptualization, Methodology, Validation, Writing – original draft. IM: Conceptualization, Methodology, Validation, Writing – original draft. JJ: Conceptualization, Methodology, Validation, Writing – original draft. AG: Conceptualization, Methodology, Validation, Writing – original draft. KG: Validation, Writing – original draft. FN: Conceptualization, Methodology, Validation, Writing – original draft. AP: Conceptualization, Methodology, Validation, Writing – original draft. MK: Visualization, Writing – review & editing. BQ: Formal analysis, Resources, Writing – original draft.
The author(s) declare that no financial support was received for the research, authorship, and/or publication of this article.
We are thankful for the editorial assistance of the NC Translational and Clinical Sciences Institute, which is supported by the National Center for Advancing Translational Sciences (NCTRACS), National Institutes of Health, through Grant Award Number UM1TR004406 (JEV, BQ).
The authors declare that the research was conducted in the absence of any commercial or financial relationships that could be construed as a potential conflict of interest.
All claims expressed in this article are solely those of the authors and do not necessarily represent those of their affiliated organizations, or those of the publisher, the editors and the reviewers. Any product that may be evaluated in this article, or claim that may be made by its manufacturer, is not guaranteed or endorsed by the publisher.
Anzellotti, F., Franciotti, R., Bonanni, L., Tamburro, G., Perrucci, M. G., Thomas, A., et al. (2010). Persistent genital arousal disorder associated with functional hyperconnectivity of an epileptic focus. Neuroscience 167, 88–96. doi: 10.1016/j.neuroscience.2010.01.050
Banerjee, A., Kamboj, P., Wyckoff, S. N., Sussman, B. L., Gupta, S. K. S., and Boerwinkle, V. L. (2023). Automated seizure onset zone locator from resting-state functional MRI in drug-resistant epilepsy. Front. Neuroimag. 1:1007668. doi: 10.3389/fnimg.2022.1007668
Barron, D. S., Fox, P. T., Pardoe, H., Lancaster, J., Price, L. R., Blackmon, K., et al. (2015). Thalamic functional connectivity predicts seizure laterality in individual TLE patients: application of a biomarker development strategy. Neuroimage Clin. 7, 273–280. doi: 10.1016/j.nicl.2014.08.002
Beghi, E. (2020). The epidemiology of epilepsy. Neuroepidemiology 54, 185–191. doi: 10.1159/000503831
Bettus, G., Bartolomei, F., Confort-Gouny, S., Guedj, E., Chauvel, P., Cozzone, P. J., et al. (2010). Role of resting state functional connectivity MRI in presurgical investigation of mesial temporal lobe epilepsy. J. Neurol. Neurosurg. Psychiatry 81, 1147–1154. doi: 10.1136/jnnp.2009.191460
Boerwinkle, V. L., Cediel, E. G., Mirea, L., Williams, K., Kerrigan, J. F., Lam, S., et al. (2019). Network targeted approach and postoperative resting state functional MRI are associated with seizure outcome. Ann. Neurol. 86, 344–356. doi: 10.1002/ana.25547
Boerwinkle, V. L., Mohanty, D., Foldes, S. T., Guffey, D., Minard, C. G., Vedantam, A., et al. (2017). Correlating resting-state functional magnetic resonance imaging connectivity by independent component analysis-based epileptogenic zones with intracranial electroencephalogram localized seizure onset zones and surgical outcomes in prospective pediatric intractable epilepsy study. Brain Connect. 7, 424–442. doi: 10.1089/brain.2016.0479
Borenstein, M., Hedges, L. V., Higgins, J. P., and Rothstein, H. R. (2010). A basic introduction to fixed-effect and random-effects models for meta-analysis. Res Synth Methods 1, 97–111. doi: 10.1002/jrsm.12
Boscolo Galazzo, I., Mattoli, M. V., Pizzini, F. B., de Vita, E., Barnes, A., Duncan, J. S., et al. (2016). Cerebral metabolism and perfusion in MR-negative individuals with refractory focal epilepsy assessed by simultaneous acquisition of (18)F-FDG PET and arterial spin labeling. Neuroimage Clin. 11, 648–657. doi: 10.1016/j.nicl.2016.04.005
Bulacio, J. C., Jehi, L., Wong, C., Gonzalez-Martinez, J., Kotagal, P., Nair, D., et al. (2012). Long-term seizure outcome after resective surgery in patients evaluated with intracranial electrodes. Epilepsia 53, 1722–1730. doi: 10.1111/j.1528-1167.2012.03633.x
Burneo, J. G., Steven, D. A., McLachlan, R. S., and Parrent, A. G. (2006). Morbidity associated with the use of intracranial electrodes for epilepsy surgery. Can. J. Neurol. Sci. 33, 223–227. doi: 10.1017/s0317167100005023
Cha, J., Hwang, J. M., Jo, H. J., Seo, S. W., Na, D. L., and Lee, J. M. (2015). Assessment of functional characteristics of amnestic mild cognitive impairment and Alzheimer’s disease using various methods of resting-state FMRI analysis. Biomed. Res. Int. 2015:907464. doi: 10.1155/2015/907464
Chakraborty, A. R., Almeida, N. C., Prather, K. Y., O’Neal, C. M., Wells, A. A., Chen, S., et al. (2020). Resting-state functional magnetic resonance imaging with independent component analysis for presurgical seizure onset zone localization: a systematic review and meta-analysis. Epilepsia 61, 1958–1968. doi: 10.1111/epi.16637
Chassoux, F., Navarro, V., Catenoix, H., Valton, L., and Vignal, J. P. (2018). Planning and management of SEEG. Neurophysiol. Clin. 48, 25–37. doi: 10.1016/j.neucli.2017.11.007
Chen, Z., An, Y., Zhao, B., Yang, W., Yu, Q., Cai, L., et al. (2017). The value of resting-state functional magnetic resonance imaging for detecting epileptogenic zones in patients with focal epilepsy. PLoS One 12:e0172094. doi: 10.1371/journal.pone.0172094
Cole, D. M., Smith, S. M., and Beckmann, C. F. (2010). Advances and pitfalls in the analysis and interpretation of resting-state FMRI data. Front. Syst. Neurosci. 4:8. doi: 10.3389/fnsys.2010.00008
Covidence Systematic Review Software. (n.d.) Veritas health innovation. Available online at: http://www.covidence.org
Engel, J. Jr. (2016). What can we do for people with drug-resistant epilepsy? The 2016 Wartenberg lecture. Neurology 87, 2483–2489. doi: 10.1212/WNL.0000000000003407
Engel, J. Jr., Wiebe, S., French, J., Sperling, M., Williamson, P., Spencer, D., et al. (2003). Practice parameter: temporal lobe and localized neocortical resections for epilepsy. Epilepsia 44, 741–751. doi: 10.1046/j.1528-1157.2003.48202.x
Fiest, K. M., Sajobi, T. T., and Wiebe, S. (2014). Epilepsy surgery and meaningful improvements in quality of life: results from a randomized controlled trial. Epilepsia 55, 886–892. doi: 10.1111/epi.12625
Galan, F. N., Beier, A. D., and Sheth, R. D. (2021). Advances in epilepsy surgery. Pediatr. Neurol. 122, 89–97. doi: 10.1016/j.pediatrneurol.2021.06.004
Gnanadas, A. A., Sathishbabu, S., and Vijayakarthik, M. (2017). Identification of epileptic seizure using ReHo from Rs-fMRI. 2017 Second International Conference on Recent Trends and Challenges in Computational Models (ICRTCCM): 292–296.
Gong, J., Wang, J., Qiu, S., Chen, P., Luo, Z., Wang, J., et al. (2020). Common and distinct patterns of intrinsic brain activity alterations in major depression and bipolar disorder: voxel-based meta-analysis. Transl. Psychiatry 10:353. doi: 10.1038/s41398-020-01036-5
Gonzalez-Martinez, J. A., Srikijvilaikul, T., Nair, D., and Bingaman, W. E. (2007). Long-term seizure outcome in reoperation after failure of epilepsy surgery. Neurosurgery 60, 873–880. doi: 10.1227/01.NEU.0000255438.13871.FA
Grande, K. M., Ihnen, S. K. Z., and Arya, R. (2020). Electrical stimulation mapping of brain function: a comparison of subdural electrodes and stereo-EEG. Front. Hum. Neurosci. 14:611291. doi: 10.3389/fnhum.2020.611291
Higgins, P. A., and Straub, A. J. (2006). Understanding the error of our ways: mapping the concepts of validity and reliability. Nurs. Outlook 54, 23–29. doi: 10.1016/j.outlook.2004.12.004
Hunyadi, B., De Vos, M., Paesschen, W., and Huffel, S.. (2015a). Exploring the epileptic network with parallel ICA of interictal EEG-FMRI. European Signal Processing Conference (EUSIPCO), Nice, France.
Hunyadi, B., Tousseyn, S., Dupont, P., Van Huffel, S., De Vos, M., and Van Paesschen, W. (2015b). A prospective fMRI-based technique for localising the epileptogenic zone in presurgical evaluation of epilepsy. NeuroImage 113, 329–339. doi: 10.1016/j.neuroimage.2015.03.011
Hunyadi, B., Tousseyn, S., Dupont, P., Van Huffel, S., Van Paesschen, W., and De Vos, M. (2014). Automatic selection of epileptic independent fMRI components. Annu Int Conf IEEE Eng med. Biol. Soc., 3853–3856. doi: 10.1109/embc.2014.6944464
Ibrahim, B., Suppiah, S., Ibrahim, N., Mohamad, M., Hassan, H. A., Nasser, N. S., et al. (2021). Diagnostic power of resting-state fMRI for detection of network connectivity in Alzheimer’s disease and mild cognitive impairment: a systematic review. Hum. Brain Mapp. 42, 2941–2968. doi: 10.1002/hbm.25369
Jann, K., Wiest, R., Hauf, M., Meyer, K., Boesch, C., Mathis, J., et al. (2008). BOLD correlates of continuously fluctuating epileptic activity isolated by independent component analysis. NeuroImage 42, 635–648. doi: 10.1016/j.neuroimage.2008.05.001
Jayakar, P., Gotman, J., Harvey, A. S., Palmini, A., Tassi, L., Schomer, D., et al. (2016). Diagnostic utility of invasive EEG for epilepsy surgery: indications, modalities, and techniques. Epilepsia 57, 1735–1747. doi: 10.1111/epi.13515
Jobst, B. C., and Cascino, G. D. (2015). Resective epilepsy surgery for drug-resistant focal epilepsy: a review. JAMA 313, 285–293. doi: 10.1001/jama.2014.17426
Kalilani, L., Sun, X., Pelgrims, B., Noack-Rink, M., and Villanueva, V. (2018). The epidemiology of drug-resistant epilepsy: a systematic review and meta-analysis. Epilepsia 59, 2179–2193. doi: 10.1111/epi.14596
Kang, J. K., Bénar, C., Al-Asmi, A., Khani, Y. A., Pike, G. B., Dubeau, F., et al. (2003). Using patient-specific hemodynamic response functions in combined EEG-fMRI studies in epilepsy. NeuroImage 20, 1162–1170. doi: 10.1016/s1053-8119(03)00290-8
Khalaf, A. M., Nadel, H. R., and Dahmoush, H. M. (2022). Simultaneously acquired MRI arterial spin-labeling and Interictal FDG-PET improves diagnosis of pediatric temporal lobe epilepsy. AJNR Am. J. Neuroradiol. 43, 468–473. doi: 10.3174/ajnr.A7421
Khoo, H. M., von Ellenrieder, N., Zazubovits, N., Hall, J. A., Dubeau, F., and Gotman, J. (2019). Internodular functional connectivity in heterotopia-related epilepsy. Ann. Clin. Transl. Neurol. 6, 1010–1023. doi: 10.1002/acn3.769
Lau, W. K. W., Leung, M. K., and Lau, B. W. M. (2019). Resting-state abnormalities in autism Spectrum disorders: a meta-analysis. Sci. Rep. 9:3892. doi: 10.1038/s41598-019-40427-7
Laxer, K. D., Trinka, E., Hirsch, L. J., Cendes, F., Langfitt, J., Delanty, N., et al. (2014). The consequences of refractory epilepsy and its treatment. Epilepsy Behav. 37, 59–70. doi: 10.1016/j.yebeh.2014.05.031
Lee, H. W., Arora, J., Papademetris, X., Tokoglu, F., Negishi, M., Scheinost, D., et al. (2014). Altered functional connectivity in seizure onset zones revealed by fMRI intrinsic connectivity. Neurology 83, 2269–2277. doi: 10.1212/WNL.0000000000001068
Malmgren, K., and Edelvik, A. (2017). Long-term outcomes of surgical treatment for epilepsy in adults with regard to seizures, antiepileptic drug treatment and employment. Seizure 44, 217–224. doi: 10.1016/j.seizure.2016.10.015
Matthews, P. M., and Jezzard, P. (2004). Functional magnetic resonance imaging. J. Neurol. Neurosurg. Psychiatry 75:6. doi: 10.1142/9781860948961_0015
McIntosh, A. M., Kalnins, R. M., Mitchell, L. A., Fabinyi, G. C., Briellmann, R. S., and Berkovic, S. F. (2004). Temporal lobectomy: long-term seizure outcome, late recurrence and risks for seizure recurrence. Brain 127, 2018–2030. doi: 10.1093/brain/awh221
Metwali, H., and Samii, A. (2019). Seed-based connectivity analysis of resting-state fMRI in patients with brain tumors: a feasibility study. World Neurosurg. 128, e165–e176. doi: 10.1016/j.wneu.2019.04.073
Morgan, V. L., Price, R. R., Arain, A., Modur, P., and Abou-Khalil, B. (2004). Resting functional MRI with temporal clustering analysis for localization of epileptic activity without EEG. NeuroImage 21, 473–481. doi: 10.1016/j.neuroimage.2003.08.031
Mullin, J. P., Shriver, M., Alomar, S., Najm, I., Bulacio, J., Chauvel, P., et al. (2016). Is SEEG safe? A systematic review and meta-analysis of stereo-electroencephalography-related complications. Epilepsia 57, 386–401. doi: 10.1111/epi.13298
Nagahama, Y., Schmitt, A. J., Nakagawa, D., Vesole, A. S., Kamm, J., Kovach, C. K., et al. (2018). Intracranial EEG for seizure focus localization: evolving techniques, outcomes, complications, and utility of combining surface and depth electrodes. J. Neurosurg. 130, 1180–1192. doi: 10.3171/2018.1.jns171808
Perissinotti, A., Niñerola-Baizán, A., Rubí, S., Carreño, M., Marti-Fuster, B., Aparicio, J., et al. (2018). PISCOM: a new procedure for epilepsy combining ictal SPECT and interictal PET. Eur. J. Nucl. Med. Mol. Imaging 45, 2358–2367. doi: 10.1007/s00259-018-4080-6
Pini, L., Jacquemot, C., Cagnin, A., Meneghello, F., Semenza, C., Mantini, D., et al. (2020). Aberrant brain network connectivity in presymptomatic and manifest Huntington’s disease: a systematic review. Hum. Brain Mapp. 41, 256–269. doi: 10.1002/hbm.24790
Reyes, A., Thesen, T., Wang, X., Hahn, D., Yoo, D., Kuzniecky, R., et al. (2016). Resting-state functional MRI distinguishes temporal lobe epilepsy subtypes. Epilepsia 57, 1475–1484. doi: 10.1111/epi.13456
Rosazza, C., Aquino, D., D’Incerti, L., Cordella, R., Andronache, A., Zacà, D., et al. (2014). Preoperative mapping of the sensorimotor cortex: comparative assessment of task-based and resting-state FMRI. PLoS One 9:e98860. doi: 10.1371/journal.pone.0098860
Rosazza, C., and Minati, L. (2011). Resting-state brain networks: literature review and clinical applications. Neurol. Sci. 32, 773–785. doi: 10.1007/s10072-011-0636-y
Sheng, J., Liu, S., Qin, H., Li, B., and Zhang, X. (2018). Drug-resistant epilepsy and surgery. Curr. Neuropharmacol. 16, 17–28. doi: 10.2174/1570159x15666170504123316
Sillanpaa, M., and Shinnar, S. (2010). Long-term mortality in childhood-onset epilepsy. N. Engl. J. Med. 363, 2522–2529. doi: 10.1056/NEJMoa0911610
Song, Q., Yin, F., Chen, H., Zhang, Y., Hu, Q., and Yao, D. (2006). “A novel unified SPM-ICA-PCA method for detecting epileptic activities in resting-state fMRI” in Advances in natural computation. eds. L. Jiao, L. Wang, X. Gao, J. Liu, and F. Wu (Berlin, Heidelberg: Springer Berlin Heidelberg), 627–636.
Stufflebeam, S. M., Liu, H., Sepulcre, J., Tanaka, N., Buckner, R. L., and Madsen, J. R. (2011). Localization of focal epileptic discharges using functional connectivity magnetic resonance imaging. J. Neurosurg. 114, 1693–1697. doi: 10.3171/2011.1.Jns10482
Su, L., An, J., Ma, Q., Qiu, S., and Hu, D. (2015). Influence of resting-state network on lateralization of functional connectivity in mesial temporal lobe epilepsy. AJNR Am. J. Neuroradiol. 36, 1479–1487. doi: 10.3174/ajnr.A4346
Tavares, V., Ribeiro, A. S., Capela, C., Cerqueira, L., and Ferreira, H. A.. (2017). Epileptogenic focus localization using complexity analysis of BOLD signals. 2017 IEEE 5th Portuguese Meeting on Bioengineering (ENBENG). 1–4.
Thijs, R. D., Surges, R., O’Brien, T. J., and Sander, J. W. (2019). Epilepsy in adults. Lancet 393, 689–701. doi: 10.1016/s0140-6736(18)32596-0
van Houdt, P. J., Ossenblok, P. P., Colon, A. J., Hermans, K. H. M., Verdaasdonk, R. M., Boon, P. A. J. M., et al. (2015). Are epilepsy-related fMRI components dependent on the presence of Interictal epileptic discharges in scalp EEG? Brain Topogr. 28, 606–618. doi: 10.1007/s10548-014-0407-1
Vivekananda, U., Cao, C., Liu, W., Zhang, J., Rugg-Gunn, F., Walker, M. C., et al. (2021). The use of simultaneous stereo-electroencephalography and magnetoencephalography in localizing the epileptogenic focus in refractory focal epilepsy. Brain Commun 3:fcab072. doi: 10.1093/braincomms/fcab072
Wang, S., Zhang, Z., Lu, G., and Luo, L. (2007). Localization of brain activity by temporal anti-correlation with the posterior cingulate cortex. Annu. Int. Conf. IEEE Eng. Med. Biol. Soc., 5227–5230. doi: 10.1109/iembs.2007.4353520
Weaver, K. E., Chaovalitwongse, W. A., Novotny, E. J., Poliakov, A., Grabowski, T. G., and Ojemann, J. G. (2013). Local functional connectivity as a pre-surgical tool for seizure focus identification in non-lesion, focal epilepsy. Front. Neurol. 4:43. doi: 10.3389/fneur.2013.00043
Whiting, P. F., Rutjes, A. W., Westwood, M. E., Mallett, S., Deeks, J. J., Reitsma, J. B., et al. (2011). QUADAS-2: a revised tool for the quality assessment of diagnostic accuracy studies. Ann. Intern. Med. 155, 529–536. doi: 10.7326/0003-4819-155-8-201110180-00009
Yang, Z., Choupan, J., Reutens, D., and Hocking, J. (2015). Lateralization of temporal lobe epilepsy based on resting-state functional magnetic resonance imaging and machine learning. Front. Neurol. 6:184. doi: 10.3389/fneur.2015.00184
Young, M. J., Bodien, Y. G., Freeman, H. J., Fecchio, M., and Edlow, B. L. (2023). Toward uniform insurer coverage for functional MRI following severe brain injury. J. Head Trauma Rehabil. 38, 351–357. doi: 10.1097/htr.0000000000000864
Zang, Y., Jiang, T., Lu, Y., He, Y., and Tian, L. (2004). Regional homogeneity approach to fMRI data analysis. NeuroImage 22, 394–400. doi: 10.1016/j.neuroimage.2003.12.030
Zang, Y.-F., Zuo, X.-N., Milham, M., and Hallett, M. (2015). Toward a Meta-analytic synthesis of the resting-state fMRI literature for clinical populations. Biomed. Res. Int. 2015:435265, 1–3. doi: 10.1155/2015/435265
Zhao, T., Wang, H., Wang, K., Yang, X., Zhou, W., and Hong, B. (2019). Cross-modal consistency of epileptogenic network in SEEG and resting-state fMRI. 2019 9th International IEEE/EMBS Conference on Neural Engineering (NER): 953–956.
Zou, Q. H., Zhu, C. Z., Yang, Y., Zuo, X. N., Long, X. Y., Cao, Q. J., et al. (2008). An improved approach to detection of amplitude of low-frequency fluctuation (ALFF) for resting-state fMRI: fractional ALFF. J. Neurosci. Methods 172, 137–141. doi: 10.1016/j.jneumeth.2008.04.012
Keywords: meta-analysis, resting-state functional MRI, seizure network, epilepsy surgery, neuroimaging
Citation: Boerwinkle VL, Nowlen MA, Vazquez JE, Arhin MA, Reuther WR, Cediel EG, McCarty PJ, Manjón I, Jubran JH, Guest AC, Gillette KD, Nowlen FM, Pines AR, Kazemi MH and Qaqish BF (2024) Resting-state fMRI seizure onset localization meta-analysis: comparing rs-fMRI to other modalities including surgical outcomes. Front. Neuroimaging. 3:1481858. doi: 10.3389/fnimg.2024.1481858
Received: 16 August 2024; Accepted: 13 November 2024;
Published: 17 December 2024.
Edited by:
Daniel Tozer, University of Cambridge, United KingdomReviewed by:
Hisako Fujiwara, Cincinnati Children’s Hospital Medical Center, United StatesCopyright © 2024 Boerwinkle, Nowlen, Vazquez, Arhin, Reuther, Cediel, McCarty, Manjón, Jubran, Guest, Gillette, Nowlen, Pines, Kazemi and Qaqish. This is an open-access article distributed under the terms of the Creative Commons Attribution License (CC BY). The use, distribution or reproduction in other forums is permitted, provided the original author(s) and the copyright owner(s) are credited and that the original publication in this journal is cited, in accordance with accepted academic practice. No use, distribution or reproduction is permitted which does not comply with these terms.
*Correspondence: Varina L. Boerwinkle, VmFyaW5hX0JvZXJ3aW5rbGVAbWVkLnVuYy5lZHU=
†These authors have contributed equally to this work and share first authorship
Disclaimer: All claims expressed in this article are solely those of the authors and do not necessarily represent those of their affiliated organizations, or those of the publisher, the editors and the reviewers. Any product that may be evaluated in this article or claim that may be made by its manufacturer is not guaranteed or endorsed by the publisher.
Research integrity at Frontiers
Learn more about the work of our research integrity team to safeguard the quality of each article we publish.