- 1Department of Neurological Surgery, Vanderbilt University Medical Center, Nashville, TN, United States
- 2Department of Biomedical Engineering, Vanderbilt University, Nashville, TN, United States
- 3Department of Neurological Surgery, University of California San Diego, La Jolla, CA, United States
Neuroimaging plays a crucial role in enabling a surgeon's proficiency and achieving optimal outcomes across various subspecialties of neurosurgery. Since Wilhelm Roentgen's groundbreaking discovery of the radiograph in 1895, imaging capabilities have advanced astronomically, significantly benefiting the field of neurosurgery. In this review, we aim to provide a concise overview of neuroimaging in four specific subspecialties: neuro-oncology, cerebrovascular, spine, and functional neurosurgery. Although the diseases and procedures mentioned are not exhaustive, they are illustrative examples of how neuroimaging has contributed to advancements in neurosurgery. Our intention is to emphasize the critical role of neuroimaging in pre-operative, intra-operative, and post-operative settings, while also highlighting its potential to drive research to further enhance existing neurosurgical technologies and ultimately better patient outcomes.
1. Introduction
Neurological conditions evaluated by neurosurgeons affect over 22 million people globally each year, with nearly 14 million individuals requiring neurosurgical intervention (Dewan et al., 2018). Neurosurgeons' skills are complimented by neuroimaging before, during, and after surgery. In the last century there have been many technological advancements in neurosurgery, arguably none larger than those in neuroimaging. Notably, between 1960 and 2010 when measuring top performing technology by patent codes (Marcus et al., 2015), 37.9% of neurosurgery patents were associated with image guidance. Further, from 2005–2010 almost half of the patents in neurosurgery were related to image guidance 46.0% (Marcus et al., 2015).
The earliest neuroimaging began with skull radiographs when Wilhelm Roentgen discovered x-rays in 1895 (Enchev, 2009). In 1918 Walter Dandy discovered ventriculography and pneumoencephalography (Figure 1) when performing radiography in a patient with an open penetrating head injury (Dandy, 1918, 1919). Portuguese neurologist Egas Moniz debuted carotid artery imaging with sodium iodide contrast in 1927 (Enchev, 2009). The vast impact of early neuroimaging, though revolutionary at the time, pales in comparison to contemporary imaging techniques including computed tomography (CT), magnetic resonance imaging (MRI), single-photon emission computerized tomography (SPECT), and positron emission tomography (PET).
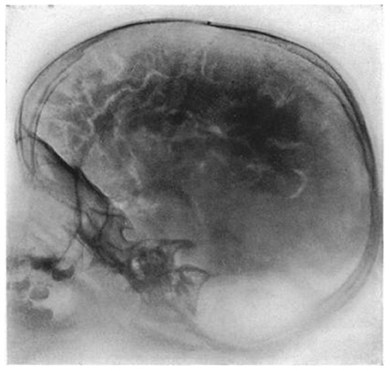
Figure 1. Pneumocephalogram, published by Dandy (1919) Open domain use.
This review examines neuroimaging applications in four neurosurgical domains: neuro-oncology (Barbaro et al., 2021), cerebrovascular (Heros and Morcos, 2000), spine (Walker et al., 2019), and functional neurosurgery (Ryvlin et al., 2014; Lewis et al., 2016). While providing examples of diseases and surgeries, it aims to demonstrate how neuroimaging contributes to diagnosis and treatment. These examples illustrate significant contributions of neuroimaging to neurosurgery, covering a range of diseases and surgeries without including every possibility. Furthermore, this review highlights the current impact of neuroimaging in neurosurgery and its future potential.
2. Neuro-oncology surgery
2.1. Current uses
Neuroimaging in neuro-oncology includes diagnosis, neuronavigation, and monitoring treatment response (Nandu et al., 2018). Preoperative MRI localizes tumor(s), neighboring anatomy, assists in tumor grading and prognosis by revealing tumor properties like edema (Figure 2C) (Nandu et al., 2018; Barbaro et al., 2021). For instance, low-grade gliomas are usually non-enhancing on T1 weighted MRI and hyperintense on T2 MRI (Barbaro et al., 2021). Another modality, 18-flurodeoxyglucose (18F-FDG) PET, may reveal metastases indicated by areas of elevated metabolic activity.
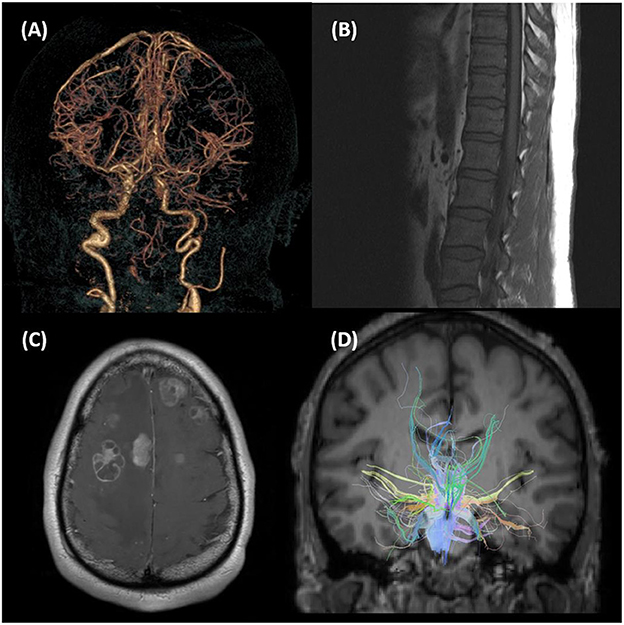
Figure 2. (A) 3D reconstruction of CT angiogram. (B) T1 sagittal MRI of lumbar spine. (C) Axial gadolinium contrast enhanced MRI of the brain showing multiple metastatic tumors. (D) Reconstructed diffusion tractography imaging superimposed upon T1 anatomical MRI.
Surgeons employ neuroimaging based surgical planning to maximize tumor removal while minimizing damage to healthy parenchyma. Preoperative imaging assists with intraoperative positioning, craniotomy size and location, and surgical trajectory (Yang et al., 2019). Beyond identifying tumor's location, neuroimaging can determine areas to avoid. For example, functional MRI (fMRI) can find eloquent areas, such as the motor cortex, and plan resection to limit/avoid postoperative deficits (Azad and Duffau, 2020).
Intraoperatively, neuroimaging is used for neuronavigation. Preoperative neuroimaging is incorporated to determine location in surgical space relative to patient's neuroimaging and anatomy. In an example tracking system, a fixed reference frame is attached to the operating bed and infrared cameras track surgical probes while displaying their location overlaid onto the patient's neuroimaging, allowing real-time intraoperative guidance (Orringer et al., 2012). Limitations of intraoperative neuronavigation include image registration techniques used to align imaging and patient space, and accuracy of the preoperative imaging relative to changes intraoperatively (Narasimhan et al., 2020a). Postoperatively neuroimaging is used to assess treatment response by monitoring tumor recurrence or identifying new tumors (Nandu et al., 2018). Continued follow up in patients with brain metastases is crucial; for example in patients who receive stereotactic radiosurgery alone for brain metastases exhibit rates as high as 40–70% of new intracranial metastases remote from original sites at 1 year (Shultz et al., 2015). Serial neuroimaging, for tumor recurrence and new tumors guides treatment response and planning.
2.2. Emerging research
A limitation to intraoperative guidance is brain shift which can invalidate intraoperative guidance systems (Sun et al., 2014). Causes of brain shift include swelling, tumor cavity collapse, and gravity (Narasimhan et al., 2020a). Ongoing research to minimize the impact of brain shift on neuronavigation includes biomechanical brain models to actively update preoperative neuroimaging into intraoperative space (Sun et al., 2014; Narasimhan et al., 2020a). These biomechanical models can correct for movements of brain tissue as tumor is resected and update intraoperative guidance in real time. Another novel solution to address intraoperative navigation mismatch is intraoperative MRI (iMRI) which enables active updating of intraoperative guidance (Albayrak et al., 2004).
There are also a variety of neuroimaging techniques with potential to improve patient care preoperatively. Diffusion-weighted MRI (DW-MRI) can be used to calculate apparent diffusion coefficient, enabling identification of tumor grades and subtypes, as well as distinguishing healthy tissue from tumor (Nandu et al., 2018). Brain tumors can alter vascularity based on tumor-induced angiogenesis, and this can be measured using imaging techniques including perfusion-weighted MRI, dynamic contrast enhanced MRI, and dynamic susceptibility contrast MRI (Nandu et al., 2018). Furthermore, computational model neuroimaging-based techniques can help perform non-invasive diagnostics (Narasimhan et al., 2019). Limitations in standardization across image acquisition and processing currently impair clinical implementation of these techniques (Nandu et al., 2018). Diffusion tensor imaging (DTI), allows for non-invasive identification neural tracts such as corticospinal tract or Meyer's loop to avoid postoperative motor and visual field deficits (Essayed et al., 2017; Henderson et al., 2020). Novel tractography algorithms allow for localization of eloquent white matter tracts despite traditionally confounding effects of localized tumor edema (Henderson et al., 2021). One randomized controlled trial using tractography for high grade glioma resection demonstrated improved resection extent and Karnofsky performance scale (Wu et al., 2007).
Functional neuroimaging guidance of tumor resection such as resting-state fMRI, is increasingly used for language mapping preoperatively (Sair et al., 2016; Gross et al., 2022). One study of 40 glioma patients showed task-based fMRI demonstrated a sensitivity of 37.1% and specificity of 83.4%. Other studies comparing fMRI for motor mapping vs. language mapping have shown higher sensitivity and specificity for motor mapping (Azad and Duffau, 2020). Generally, neuroimaging is essential in neuro-oncology and further incorporation of advanced neuroimaging into standard oncological neurosurgical care may improve resection extent and reduce risk to eloquent cortex.
3. Cerebrovascular surgery
3.1. Current uses
Cerebrovascular surgery includes treatment of arteriovenous malformations, intracranial hemorrhages (ICH), strokes, aneurysms, and other vascular lesions with high rates of mortality and morbidity (Michalak et al., 2016). Successful cerebrovascular neurosurgery is highly dependent upon timing, i.e., “time is brain” (Saver, 2006); therefore preoperatively, the most frequently used modality is CT. CT quickly allows physicians to evaluate size and location of ICH, presence of intraventricular hemorrhage, hydrocephalus, and edema. Clinical evaluation and ICH score (calculated from neuroimaging) are used for predicting 30-day mortality after hemorrhage (Hemphill et al., 2001). CT angiography (CTA) can localize pathology and may aid prognostication (Figure 2A). One study showed the spot sign score could predict hematoma expansion in ICH as it represents focal pooling or extravasation of contrast within a hematoma. Presence of spot sign increased in hospital mortality risk (55.6%) and poor outcome among survivors at 3-month follow-up (50.8%) (Delgado Almandoz et al., 2010). Three neuroimaging methods for preoperative subarachnoid hemorrhage (SAH) diagnosis are non-contrast computed tomography (NCCT) with lumbar puncture, NCCT followed by CTA, and magnetic resonance angiography (MRA) (de Oliveira Manoel et al., 2014). For SAH, NCCT is very sensitive in acute detection of bleed (< 6 h), however, lumbar puncture showing xanthocromia is the traditional gold standard for presence of blood in CSF (de Oliveira Manoel et al., 2014). Neuroimaging is employed postoperatively to assess treatment efficacy and determine next steps.
With emergence of the coronavirus pandemic, there has been an increase in acute cerebrovascular disease (Hernández-Fernández et al., 2020). A study performed in Spain over 50 days, showed 23 1,683 COVID admissions developed cerebrovascular disease (Hernández-Fernández et al., 2020). As the COVID-19 pandemic continues, neuroimaging may better characterization cerebrovascular sequelae in COVID.
3.2. Emerging research
Imaging modalities that traditionally treatment of cerebrovascular disease, evaluate the lumen of blood vessels. Cerebrovascular disease often begins as inflammatory changes in the vessel wall preceding luminal/perfusion changes, therefore intracranial vessel wall imaging may allow earlier detection and intervention for cerebrovascular disease by screening patients with established risk factors thereby allowing for early intervention (Young et al., 2019).
For SAH and other cerebrovascular diseases ongoing research includes use of structural MRI, DW-MRI, MRI morphometry, and fMRI to improve patient care (de Oliveira Manoel et al., 2014). ICH volume changes assessed with structural MRI correlate with patient performance on neuropsychological performances (de Oliveira Manoel et al., 2014). Additionally, DWI can categorize brain tissue at risk for ischemia and help predict poor outcomes (de Oliveira Manoel et al., 2014).
Intraoperative digital subtraction angiography (iDSA) is not frequently applied in open cerebrovascular surgery, but has been shown to alter treatment in 12% of patients (Fandino et al., 2013). iDSA may reduce excessive surgical steps/intraoperative time in cerebrovascular surgery. Combining iDSA with near-infrared indocyanine green video angiography helps assess vascular flow during resection of vascular lesions like cavernomas or arteriovenous malformations and improve patient outcomes (Fandino et al., 2013). Overall, imaging is fundamental for cerebrovascular surgery and improvements in neuroimaging techniques can facilitate improved outcomes.
4. Spine surgery
4.1. Current uses
Patient selection in spine surgery is primarily guided by clinical exams and imaging which are key factors in successful surgical outcomes (Ahn et al., 2016; Mundell et al., 2018; Letchuman et al., 2022). Preoperatively, plain radiograph (x-ray) is quick and inexpensive; and, despite low resolution, is the initial imaging modality to evaluate spinal pathologies (Harada et al., 2020). Furthermore, by obtaining multiple view x-rays spinal stability can be evaluated under different loading conditions (Harada et al., 2020). X-rays are fundamental to preoperative measurements including Cobb angles, lordosis, and kyphosis (Harada et al., 2020).
Advanced spinal imaging, including MRI and CT, provide additional preoperative information (Figure 2B). For example, preoperative CT allows assessment of pedicle anatomy for planning of screw placement during instrumented fusions (Harada et al., 2020). CT myelography evaluates spinal cord, nerve roots, and spinal lining by injecting contrast into the thecal sac. Although largely supplanted by MRI, it is still used in those who cannot undergo MRI, and some argue it is superior to MRI in evaluation of foraminal/lateral recess stenosis (Harada et al., 2020) [with MRI missing lateral recess nerve root compression in 30% of cases (Bartynski and Lin, 2003)]. Some have shown using advanced spinal imaging increases rates of spine surgery, however, their contributions to spine surgery are immeasurable (Lurie et al., 2003).
Intraoperatively visualizing anatomy through x-ray has decreased reliance on anatomic landmarks and allows surgeons to directly verify spinal level and decreased incidence of wrong-level surgery while improving pedicle screw placement accuracy (Mao et al., 2021). Most spinal surgeries now employ fluoroscopic imaging for level localization and hardware placement (Harada et al., 2020). Surgical image guidance was originally developed for intracranial surgery; however, these have recently been translated into systems for spine surgery (Mao et al., 2021). Systems like StealthStation (Medtronic, Memphis, TN, USA), use a reference arc attached to fixed spinous process to register 3D data with intraoperative patient position (Girardi et al., 1999). This allows surgeons to use preoperative image data fused with intraoperative 3D data for patient-specific surgical guidance. Intraoperative imaging, such as O-arm (Medtronic, Dublin, Ireland) and Airo (Brainlab, Feldkirchen, Germany), allow imaging in operative position, increasing safety and accuracy (Sembrano et al., 2012; Scarone et al., 2018). These modern intraoperative neuroimaging systems have revolutionized spine surgery and patient care.
4.2. Emerging research
Some surgeons argue future of spine surgery lies in combining image guidance technology and robotic surgery, including systems like augmented reality (Ahern et al., 2020; Pham et al., 2020). Image guided robotic surgery may mitigate surgeon fatigue and improve precision of movements in long procedures. A recent multicentre study comparing navigated and non-navigated robot-assisted spine surgery showed that combining image navigation with robotics reduced fluoroscopic radiation time per screw, decreased robot abandonment rate, and resulted in lower blood transfusion rate compared to system without navigation (Lee et al., 2021). Many believe that the next generation of image guided robotics will begin to include artificial intelligence and machine learning techniques to predict surgical movements and cut down on response time (D'Souza et al., 2019).
In efforts to improve upon qualitative assessments of cord change, a marker of injury, techniques like sodium MRI have been developed to characterize viability of tissues by indirectly assessing active membrane sodium-potassium pumps (Madelin et al., 2014). Negative charge of glycosaminoglycan side chains in intravertebral disk are balanced by sodium concentration in intervertebral disks, therefore sodium MRI has potential to help detect early degeneration of intravertebral disks by assessing concentration of sodium (Madelin et al., 2014). Generally, combining novel neuroimaging with advances in robotics may allow improved patient selection and improved surgical accuracy.
5. Functional surgery
The World Society for Stereotactic and Functional Neurosurgery (WSSFN) states that neuroimaging is inherent to the definition of this neurosurgical field: “Stereotactic and Functional Neurosurgery is a branch of neurosurgery that utilizes dedicated structural and functional neuroimaging to identify and target discrete areas of the brain and to perform specific interventions to relieve a variety of symptoms of neurological and other disorders and to improve function of both the structurally normal and abnormal nervous system.” This area of neurosurgery includes but is not limited to treatment of movement disorders, epilepsy, and psychiatric disorders (Ryvlin et al., 2014).
5.1. Current uses
5.1.1. Movement disorders
While diagnosis for movement disorders is often clinical, neuroimaging is vital in patient evaluation and diagnosis (Mascalchi et al., 2012). Characteristic patterns of atrophy visualized on T1-weighted MRI are pathognomonic for various forms of Parkinsonism, for example the hummingbird sign of progressive supranuclear palsy (PSP) or hot cross bun sign of multisystem atrophy (MSA) (Mascalchi et al., 2012). SPECT, using dopamine transporter (DAT) labeling, shows asymmetric decreases in uptake in putamen and caudate in even early Parkinson's disease (Mascalchi et al., 2012). In PSP and MSA, SPECT shows decreased uptake of tracers and dopamine D2 receptors in striata, not observed early in Parkinson's disease (Mascalchi et al., 2012).
Preoperative neuroimaging in movement disorders is most helpful in enhancing accuracy when planning surgical interventions (Ben-Haim et al., 2011). Traditionally interventions for movement disorders utilize stereotaxic techniques, such as Leksell frame with intraoperative x-ray, to pass an electrode to target (Zrinzo, 2010). Historically, DBS (deep brain stimulation) targeting was accomplished during awake surgery and accuracy/efficacy of implantation could be evaluated with microelectrode recordings, known neurophysiology, and test stimulations. Recently, systems like STarFix have combined preoperative neuroimaging and surgery planning software to 3-D print custom stereotactic frames for intraoperative use (Konrad et al., 2011; D'Haese et al., 2012). These “frameless” methods of DBS implantation are based on preoperative high-resolution imaging combined with minimally invasive fiducial markers placed before imaging acquisition.
5.1.2. Epilepsy
Epilepsy surgery, for individuals with drug-resistant epilepsy, includes neuromodulation, resection, and ablation (Kwan et al., 2010; Englot, 2018; Gonzalez et al., 2019a). Neuroimaging for epilepsy includes scalp EEG and high-quality 3T MRI, confident localization is key to surgical planning (Deblaere and Achten, 2008; Zijlmans et al., 2009). PET may show focal region(s) of hypometabolism indicating seizure onset zone. In patients without structural evidence, MRI-negative, FDG-PET may provide evidence to guide surgical planning (Wong et al., 2012; Capraz et al., 2015). In a study of 141 patients who underwent anterior temporal lobectomy for TLE 24 patients, with MRI negative but FDG-PET hypometabolism, were seizure free at a rate of 79.2%, while those with mesial temporal sclerosis on MRI were seizure free at a rate of 82% (Capraz et al., 2015; Shin et al., 2018). Conversely, hyperperfusion on ictal SPECT may correspond with focal seizure onset. Sensitivity of SPECT is enhanced when subtracting interictal SPECT from ictal SPECT and registering to anatomical MRI (SISCOM) (Desai et al., 2013; Sulc et al., 2014). Interictal magnetoencephalography (MEG) may help identify epileptogenic activity between seizures (Englot et al., 2015). Just as in neuro-oncological surgery, either fMRI or MEG can be used for non-invasive localization of eloquent cortex. This facilitates preoperative surgical planning, including quantifying risk to motor, sensory, and language cortices (Szaflarski et al., 2017). Other neuroimaging techniques, Wada (intracarotid sodium amobarbital) and fMRI, can be used to preoperatively lateralize verbal/visuospatial memory (Abou-Khalil, 2007).
5.2. Emerging research
Structural MRI is needed for accurate DBS implantation, but employing connectome framework with MRI can be used to evaluate and optimize efficacy of DBS. Horn and Fox outline how connectomics neuromodulation will soon provide opportunities to guide neuromodulation (Horn and Fox, 2020). Recent studies using fMRI have shown subcortical arousal structures have abnormal functional connectivity proportional to disease severity, and some of these connectivity abnormalities resolve after successful epilepsy surgery. These subcortical arousal structures may represent future targets for neuromodulation to treat consciousness impairment during seizures (Kundu et al., 2018; Gonzalez et al., 2019b, 2020, 2021). SEEG based connectomics studies have shown that seizure onset zone may be identified using directional functional connectivity without requiring ictal recordings (Narasimhan et al., 2020b; Paulo et al., 2022).
Novel neuroimaging techniques now allow for asleep DBS placement. The advent of asleep DBS has led to a contentious discussion of asleep vs. awake DBS surgery. Some argue that awake surgeries may present a greater risk to the patient due to multiple electrode(s) passes during intraoperative testing relative to asleep placement. The counter argument is in asleep patient efficacy of electrode placement cannot be evaluated until weeks later. Asleep targeting for DBS and other neuromodulation truly opens the possibility of treatment of non-motor diseases. For example, when treating depression or obsessive-compulsive disorder, targets do not always display an acute response to stimulation.
FMRI may soon be an invaluable tool for functional neurosurgery. A group used fMRI in patients with Parkinson's Disease to identify DBS stimulation frequency associated with clinically optimized settings (DiMarzio et al., 2021). In this preliminary study, 2 of 14 patients experienced sustained symptom improvement after fMRI-guided programming. As noted in their study, traditional programming techniques can take up to 6 months with multiple return neurologist visits, and neuroimaging guided DBS programming could decrease burden of system optimization. Overall, neuroimaging is already fundamental for functional neurosurgery and will play a growing role.
Directional leads in deep brain stimulation (DBS) offer selective stimulation of specific brain regions, providing more control over direction/spread of stimulation. Unlike conventional DBS electrodes, directional leads have multiple independent contacts that can be programmed individually. Computational modeling based on neuroimaging, such as tractography of deep brain white matter tracts (Figure 2D), can aid in planning and identifying DBS targets (Howell and McIntyre, 2016). Recent studies have shown that patient-specific white matter connectivity and electrical field modeling can help predict clinical benefits in Parkinson's disease (PD) (DiRisio et al., 2023).
Imaging-negative epilepsy and movement disorders are surgically challenging. For example, some patients with clinical features of Parkinson's disease have scans without evidence of dopaminergic deficit (SWEDD) (Nicastro et al., 2018). Recent advents such as quantitative and machine learning based processing of SPECT have identified cases of PD previously thought to be imaging SWEDD PD, suggesting others may be misdiagnosis of PD (Erro et al., 2016). MRI negative epilepsy, such as TLE traditionally has lower rates of successful surgical treatment. We know that up to 30% of patients with TLE have MRI negative scans (Muhlhofer et al., 2017). In patients with refractory unilateral TLE, there exist abnormal cortical and subcortical resting-state fMRI-based connectivity which lateralize ipsilateral to the epileptogenic zone (Vaughan et al., 2016; González et al., 2022). Additionally, modern neuroimaging techniques are beginning to guide surgical treatment of diseases for which we have no reliable structural correlates, such as neuropsychiatric disorders and pain. These connectivity abnormalities may be used clinically to help guide surgical plans.
6. Conclusion
Neuroimaging and neurosurgery go hand in hand and there are seemingly endless possibilities to better leverage current imaging techniques as well as incorporate emerging technologies. There is a large disparity in surgical care globally, with approximately 5 million people annually not receiving necessary surgery (Dewan et al., 2018). While neuroimaging cannot fully address this disparity, improvements in neuroimaging technology with a focus on accessibility have the potential to bridge the gap in patient care globally. Another emerging and rapidly growing area of research is the combination of machine learning with neuroimaging for neurosurgical care (Kamal et al., 2018; Senders et al., 2018; Davatzikos, 2019). For example, with respect to navigation, machine learning has been incorporated into guidance for autonomous surgical robots (Senders et al., 2018). However, incorporation of all novel neuroimaging techniques is limited by standardization of acquisition and processing techniques.
As evident here and in other existing material, there is an ever-increasing abundance of neuroimaging techniques and new methods to utilize it. Neuroimaging has developed boundlessly, and modern techniques have surpassed its humble beginnings (Figure 2). Both from the perspective of researchers and clinicians, it is crucial for the improvement of patient care in neurosurgery that scientist developing novel neuroimaging techniques and neurosurgeons employing clinical neuroimaging continue to collaborate to leverage our existing and emerging methodologies to further patient care.
Author contributions
SN and HG contributed to the design and conception of this review. All authors contributed to the manuscript revision and approved of the submitted version.
Conflict of interest
The authors declare that the research was conducted in the absence of any commercial or financial relationships that could be construed as a potential conflict of interest.
Publisher's note
All claims expressed in this article are solely those of the authors and do not necessarily represent those of their affiliated organizations, or those of the publisher, the editors and the reviewers. Any product that may be evaluated in this article, or claim that may be made by its manufacturer, is not guaranteed or endorsed by the publisher.
References
Abou-Khalil, B. (2007). An update on determination of language dominance in screening for epilepsy surgery: the Wada test and newer noninvasive alternatives. Epilepsia. 48, 442–455. doi: 10.1111/j.1528-1167.2007.01012.x
Ahern, D. P., Gibbons, D., Schroeder, G. D., Vaccaro, A. R., and Butler, J. S. (2020). Image-guidance, robotics, and the future of spine surgery. Clin. Spine Surg. 33, 179–184. doi: 10.1097/BSD.0000000000000809
Ahn, J., Bohl, D. D., Tabaraee, E., Basques, B. A., and Singh, K. (2016). Current trends in outpatient spine surgery. Clin. Spine Surg. 29, 384–386. doi: 10.1097/BSD.0000000000000417
Albayrak, B., Samdani, A., and Black, P. (2004). Intra-operative magnetic resonance imaging in neurosurgery. Acta Neurochir. 146, 543–557. doi: 10.1007/s00701-004-0229-0
Azad, T. D., and Duffau, H. (2020). Limitations of functional neuroimaging for patient selection and surgical planning in glioma surgery. Neurosurg. Focus. 48, E12. doi: 10.3171/2019.11.FOCUS19769
Barbaro, M., Fine, H. A., and Magge, R. S. (2021). Foundations of Neuro-Oncology: A Multidisciplinary Approach. World Neurosurg. 151, 392–401. doi: 10.1016/j.wneu.2021.02.059
Bartynski, W. S., and Lin, L. (2003). Lumbar root compression in the lateral recess: MR imaging, conventional myelography, and CT myelography comparison with surgical confirmation. Am. J. Neuroradiol. 24, 348–360.
Ben-Haim, S., Gologorsky, Y., Monahan, A., Weisz, D., and Alterman, R. L. (2011). Fiducial registration with spoiled gradient-echo magnetic resonance imaging enhances the accuracy of subthalamic nucleus targeting. Neurosurgery. 69, 870–875. doi: 10.1227/NEU.0b013e318222ae33
Capraz, I. Y., Kurt, G., Akdemir, Ö., Hirfanoglu, T., Oner, Y., Sengezer, T., et al. (2015). Surgical outcome in patients with MRI-negative, PET-positive temporal lobe epilepsy. Seizure. 29, 63–68. doi: 10.1016/j.seizure.2015.03.015
Dandy, W. E. (1918). Ventriculography following the injection of air into the cerebral ventricles. Ann. Surg. 68, 5. doi: 10.1097/00000658-191807000-00002
Dandy, W. E. (1919). Roentgenography of the brain after the injection of air into the spinal canal. Ann. Surg. 70, 397. doi: 10.1097/00000658-191910000-00004
Davatzikos, C. (2019). Machine learning in neuroimaging: progress and challenges. Neuroimage. 197, 652. doi: 10.1016/j.neuroimage.2018.10.003
de Oliveira Manoel, A. L., Mansur, A., Murphy, A., Turkel-Parrella, D., Macdonald, M., Macdonald, R. L., et al. (2014). Aneurysmal subarachnoid haemorrhage from a neuroimaging perspective. Crit. Care. 18, 1–13. doi: 10.1186/s13054-014-0557-2
Deblaere, K., and Achten, E. (2008). Structural magnetic resonance imaging in epilepsy. Eur Radiol. 18, 119–129. doi: 10.1007/s00330-007-0710-2
Delgado Almandoz, J. E., Yoo, A. J., Stone, M. J., Schaefer, P. W., Oleinik, A., Brouwers, H. B., et al. (2010). The spot sign score in primary intracerebral hemorrhage identifies patients at highest risk of in-hospital mortality and poor outcome among survivors. Stroke. 41, 54–60. doi: 10.1161/STROKEAHA.109.565382
Desai, A., Bekelis, K., Thadani, V. M., Roberts, D. W., Jobst, B. C., Duhaime, A. C., et al. (2013). Interictal PET and ictal subtraction SPECT: sensitivity in the detection of seizure foci in patients with medically intractable epilepsy. Epilepsia. 54, 341–350. doi: 10.1111/j.1528-1167.2012.03686.x
Dewan, M. C., Rattani, A., Fieggen, G., Arraez, M. A., Servadei, F., Boop, F. A., et al. (2018). Global neurosurgery: the current capacity and deficit in the provision of essential neurosurgical care. Executive summary of the global neurosurgery initiative at the program in global surgery and social change. J. Neurosurg. 130, 1055–1064. doi: 10.3171/2017.11.JNS171500
D'Haese, P. F., Pallavaram, S., Li, R., Remple, M. S., Kao, C., Neimat, J. S., et al. (2012). CranialVault and its CRAVE tools: a clinical computer assistance system for deep brain stimulation (DBS) therapy. Med. Image Anal. 16, 744–753. doi: 10.1016/j.media.2010.07.009
DiMarzio, M., Madhavan, R., Hancu, I., Fiveland, E., Prusik, J., Joel, S., et al. (2021). Use of functional MRI to assess effects of deep brain stimulation frequency changes on brain activation in Parkinson disease. Neurosurgery. 88, 356–365. doi: 10.1093/neuros/nyaa397
DiRisio, A. C., Avecillas-Chasin, J. M., Platt, S., Jimenez-Shahed, J., Figee, M., Mayberg, H. S., et al. (2023). White matter connectivity of subthalamic nucleus and globus pallidus interna targets for deep brain stimulation. J. Neurosurg. 1, 1–10. doi: 10.3171/2023.2.JNS222576
D'Souza, M., Gendreau, J., Feng, A., Kim, L. H., Ho, A. L., Veeravagu, A., et al. (2019). Robotic-assisted spine surgery: history, efficacy, cost, and future trends. Robot Surg. 6, 9. doi: 10.2147/RSRR.S190720
Enchev, Y. (2009). Neuronavigation: geneology, reality, and prospects. Neurosurg Focus. 27, E11. doi: 10.3171/2009.6.FOCUS09109
Englot, D. J. (2018). A modern epilepsy surgery treatment algorithm: incorporating traditional and emerging technologies. Epilepsy Behav. 80, 68–74. doi: 10.1016/j.yebeh.2017.12.041
Englot, D. J., Nagarajan, S. S., Imber, B. S., Raygor, K. P., Honma, S. M., Mizuiri, D., et al. (2015). Epileptogenic zone localization using magnetoencephalography predicts seizure freedom in epilepsy surgery. Epilepsia. 56, 949–958. doi: 10.1111/epi.13002
Erro, R., Schneider, S. A., Stamelou, M., Quinn, N. P., and Bhatia, K. P. (2016). What do patients with scans without evidence of dopaminergic deficit (SWEDD) have? New evidence and continuing controversies. J. Neurol. Neurosurg. Psychiat. 87, 319–323. doi: 10.1136/jnnp-2014-310256
Essayed, W. I., Zhang, F., Unadkat, P., Cosgrove, G. R., Golby, A. J., O'Donnell, L. J., et al. (2017). White matter tractography for neurosurgical planning: A topography-based review of the current state of the art. NeuroImage. 15, 659–672. doi: 10.1016/j.nicl.2017.06.011
Fandino, J., Taussky, P., Marbacher, S., Muroi, C., Diepers, M., Fathi, A. R., et al. (2013). The concept of a hybrid operating room: applications in cerebrovascular surgery. Acta Neurochir. Suppl. 115, 113–117. doi: 10.1007/978-3-7091-1192-5_24
Girardi, F., Cammisa Jr, F., Sandhu, H., and Alvarez, L. (1999). The placement of lumbar pedicle screws using computerised stereotactic guidance. J. Bone Joint Surg. Br. 81, 825–829. doi: 10.1302/0301-620X.81B5.0810825
González, H. F., Narasimhan, S., Goodale, S. E., Johnson, G. W., Doss, D. J., Paulo, D. L., et al. (2022). Arousal and salience network connectivity alterations in surgical temporal lobe epilepsy. J. Neurosurg. 1, 1–11. doi: 10.3171/2022.5.JNS22837
Gonzalez, H. F. J., Chakravorti, S., Goodale, S. E., Gupta, K., Claassen, D. O., Dawant, B., et al. (2019b). Thalamic arousal network disturbances in temporal lobe epilepsy and improvement after surgery. J. Neurol. Neurosurg. Psychiatry. 90, 1109–1116. doi: 10.1136/jnnp-2019-320748
Gonzalez, H. F. J., Goodale, S. E., Jacobs, M. L., Haas, K. F., Landman, B. A., Morgan, V. L., et al. (2020). Brainstem functional connectivity disturbances in epilepsy may recover after successful surgery. Neurosurgery. 86, 417–428. doi: 10.1093/neuros/nyz128
Gonzalez, H. F. J., Narasimhan, S., Johnson, G. W., Wills, K. E., Haas, K. F., Konrad, P. E., et al. (2021). Role of the nucleus basalis as a key network node in temporal lobe Epilepsy. Neurology. 96, e1334–e1346. doi: 10.1212/WNL.0000000000011523
Gonzalez, H. F. J., Yengo-Kahn, A., and Englot, D. J. (2019a). Vagus nerve stimulation for the treatment of Epilepsy. Neurosurg. Clin. N Am. 30, 219–230. doi: 10.1016/j.nec.2018.12.005
Gross, W. L., Helfand, A. I., Swanson, S. J., Conant, L. L., Humphries, C. J., Raghavan, M., et al. (2022). Prediction of naming outcome with fMRI language lateralization in left temporal epilepsy surgery. Neurology. 98, e2337–e46. doi: 10.1212/WNL.0000000000200552
Harada, G. K., Siyaji, Z. K., Younis, S., Louie, P. K., Samartzis, D., An, H. S., et al. Imaging in spine surgery: current concepts future directions. Spine, Surg. Relat. Res. (2020) 4, 99–110. doi: 10.22603/ssrr.2020-0011.
Hemphill, I. I. I. J. C., Bonovich, D. C., Besmertis, L., Manley, G. T., and Johnston, S. C. (2001). The ICH score: a simple, reliable grading scale for intracerebral hemorrhage. Stroke. 32, 891–897. doi: 10.1161/01.STR.32.4.891
Henderson, F., Abdullah, K. G., Verma, R., and Brem, S. (2020). Tractography and the connectome in neurosurgical treatment of gliomas: the premise, the progress, and the potential. Neurosurg. Focus. 48, E6. doi: 10.3171/2019.11.FOCUS19785
Henderson, F., Parker, D., Vijayakumari, A. A., Elliott, M., Lucas, T., McGarvey, M. L., et al. (2021). Enhanced fiber tractography using edema correction: application and evaluation in high-grade gliomas. Neurosurgery. 89, 246. doi: 10.1093/neuros/nyab129
Hernández-Fernández, F., Sandoval Valencia, H., Barbella-Aponte, R. A., Collado-Jiménez, R., Ayo-Martín, Ó., Barrena, C., et al. (2020). Cerebrovascular disease in patients with COVID-19: neuroimaging, histological and clinical description. Brain. 143, 3089–3103. doi: 10.1093/brain/awaa239
Heros, R. C., and Morcos, J. J. (2000). Cerebrovascular surgery: past, present, and future. Neurosurgery. 47, 1007–1033. doi: 10.1097/00006123-200011000-00001
Horn, A., and Fox, M. D. (2020). Opportunities of connectomic neuromodulation. Neuroimage. 221, 117180. doi: 10.1016/j.neuroimage.2020.117180
Howell, B., and McIntyre, C. C. (2016). Analyzing the tradeoff between electrical complexity and accuracy in patient-specific computational models of deep brain stimulation. J. Neural. Eng. 13, 036023. doi: 10.1088/1741-2560/13/3/036023
Kamal, H., Lopez, V., and Sheth, S. A. (2018). Machine learning in acute ischemic stroke neuroimaging. Front. Neurol. 9, 945. doi: 10.3389/fneur.2018.00945
Konrad, P. E., Neimat, J. S., Yu, H., Kao, C. C., Remple, M. S., D'Haese, P. F., et al. (2011). Customized, miniature rapid-prototype stereotactic frames for use in deep brain stimulator surgery: initial clinical methodology and experience from 263 patients from 2002 to 2008. Stereotact. Funct. Neurosurg. 89, 34–41. doi: 10.1159/000322276
Kundu, B., Brock, A. A., Englot, D. J., Butson, C. R., and Rolston, J. D. (2018). Deep brain stimulation for the treatment of disorders of consciousness and cognition in traumatic brain injury patients: a review. Neurosurg. Focus. 45, E14. doi: 10.3171/2018.5.FOCUS18168
Kwan, P., Arzimanoglou, A., Berg, A. T., Brodie, M. J., Allen Hauser, W., Mathern, G., et al. (2010). Definition of drug resistant epilepsy: consensus proposal by the ad hoc Task Force of the ILAE Commission on Therapeutic Strategies. Epilepsia. 51, 1069–1077. doi: 10.1111/j.1528-1167.2009.02397.x
Lee, N. J., Zuckerman, S. L., Buchanan, I. A., Boddapati, V., Mathew, J., Leung, E., et al. (2021). Is there a difference between navigated and non-navigated robot cohorts in robot-assisted spine surgery? A multicenter, propensity-matched analysis of 2,800 screws and 372 patients. Spine J. 21, 1504–1512. doi: 10.1016/j.spinee.2021.05.015
Letchuman, V., Agarwal, N., Mummaneni, V. P., Wang, M. Y., Shabani, S., Patel, A., et al. (2022). Pearls and pitfalls of awake spine surgery: a simplified patient-selection algorithm. World Neurosurg. 161, 154–155. doi: 10.1016/j.wneu.2022.02.085
Lewis, P. M., Thomson, R. H., Rosenfeld, J. V., and Fitzgerald, P. B. (2016). Brain neuromodulation techniques: a review. Neuroscientist. 22, 406–421. doi: 10.1177/1073858416646707
Lurie, J. D., Birkmeyer, N. J., and Weinstein, J. N. (2003). Rates of advanced spinal imaging and spine surgery. Spine. 28, 616–620. doi: 10.1097/01.BRS.0000049927.37696.DC
Madelin, G., Lee, J. S., Regatte, R. R., and Jerschow, A. (2014). Sodium MRI: methods and applications. Prog. Nucl. Magn. Reson. Spectrosc. 79, 14–47. doi: 10.1016/j.pnmrs.2014.02.001
Mao, J. Z., Agyei, J. O., Khan, A., Hess, R. M., Jowdy, P. K., Mullin, J. P., et al. (2021). Technologic evolution of navigation and robotics in spine surgery: a historical perspective. World Neurosurg. 145, 159–167. doi: 10.1016/j.wneu.2020.08.224
Marcus, H. J., Hughes-Hallett, A., Kwasnicki, R. M., Darzi, A., Yang, G. Z., Nandi, D., et al. (2015). Technological innovation in neurosurgery: a quantitative study. J. Neurosurg. 123, 174–181. doi: 10.3171/2014.12.JNS141422
Mascalchi, M., Vella, A., and Ceravolo, R. (2012). Movement disorders: role of imaging in diagnosis. J. Magn. Reson. Imag. 35, 239–256. doi: 10.1002/jmri.22825
Michalak, S. M., Rolston, J. D., and Lawton, M. T. (2016). Incidence and predictors of complications and mortality in cerebrovascular surgery: national trends from 2007 to 2012. Neurosurgery. 79, 182–193. doi: 10.1227/NEU.0000000000001251
Muhlhofer, W., Tan, Y. L., Mueller, S. G., and Knowlton, R. (2017). MRI-negative temporal lobe epilepsy—What do we know? Epilepsia. 58, 727–742. doi: 10.1111/epi.13699
Mundell, B. F., Gates, M. J., Kerezoudis, P., Alvi, M. A., Freedman, B. A., Nassr, A., et al. (2018). Does patient selection account for the perceived cost savings in outpatient spine surgery? A meta-analysis of current evidence and analysis from an administrative database: presented at the 2018 AANS/CNS Joint Section on Disorders of the Spine and Peripheral Nerves. J. Neurosurg. 29, 687–695. doi: 10.3171/2018.4.SPINE1864
Nandu, H., Wen, P. Y., and Huang, R. Y. (2018). Imaging in neuro-oncology. Ther. Adv. Neurol. Disord. 11, 1756286418759865. doi: 10.1177/1756286418759865
Narasimhan, S., Johnson, H. B., Nickles, T. M., Miga, M. I., Rana, N., Attia, A., et al. (2019). Biophysical model-based parameters to classify tumor recurrence from radiation-induced necrosis for brain metastases. Med. Phys. 46, 2487–2496. doi: 10.1002/mp.13461
Narasimhan, S., Kundassery, K. B., Gupta, K., Johnson, G. W., Wills, K. E., Goodale, S. E., et al. (2020b). Seizure-onset regions demonstrate high inward directed connectivity during resting-state: An SEEG study in focal epilepsy. Epilepsia. 61, 2534–2544. doi: 10.1111/epi.16686
Narasimhan, S., Weis, J. A., Luo, M., Simpson, A. L., Thompson, R. C., Miga, M. I., et al. (2020a). Accounting for intraoperative brain shift ascribable to cavity collapse during intracranial tumor resection. J. Med. Imag. 7, 031506. doi: 10.1117/1.JMI.7.3.031506
Nicastro, N., Burkhard, P. R., and Garibotto, V. (2018). Scan without evidence of dopaminergic deficit (SWEDD) in degenerative parkinsonism and dementia with Lewy bodies: a prospective study. J. Neurol. Sci. 385, 17–21. doi: 10.1016/j.jns.2017.11.039
Orringer, D. A., Golby, A., and Jolesz, F. (2012). Neuronavigation in the surgical management of brain tumors: current and future trends. Expert. Rev. Med. Devices. 9, 491–500. doi: 10.1586/erd.12.42
Paulo, D. L., Wills, K. E., Johnson, G. W., Gonzalez, H. F., Rolston, J. D., Naftel, R. P., et al. SEEG functional connectivity measures to identify epileptogenic zones: stability, medication influence, recording condition. Neurology. (2022) 98, e2060–e2072. doi: 10.1212/WNL.0000000000200386.
Pham, M. H., Osorio, J. A., and Lehman, R. A. (2020). Navigated spinal robotics in minimally invasive spine surgery, with preoperative and intraoperative workflows: 2-dimensional operative video. Oper. Neurosurg. 19, E422–E. doi: 10.1093/ons/opaa095
Ryvlin, P., Cross, J. H., and Rheims, S. (2014). Epilepsy surgery in children and adults. Lancet Neurol. 13, 1114–1126. doi: 10.1016/S1474-4422(14)70156-5
Sair, H. I., Yahyavi-Firouz-Abadi, N., Calhoun, V. D., Airan, R. D., Agarwal, S., Intrapiromkul, J., et al. (2016). Presurgical brain mapping of the language network in patients with brain tumors using resting-state f MRI: Comparison with task f MRI. Hum. Brain Mapp. 37, 913–923. doi: 10.1002/hbm.23075
Saver, J. L. (2006). Time is brain–quantified. Stroke. 37, 263–266. doi: 10.1161/01.STR.0000196957.55928.ab
Scarone, P., Vincenzo, G., Distefano, D., Del Grande, F., Cianfoni, A., Presilla, S., et al. (2018). Use of the Airo mobile intraoperative CT system versus the O-arm for transpedicular screw fixation in the thoracic and lumbar spine: a retrospective cohort study of 263 patients. J. Neurosurg. 29, 397–406. doi: 10.3171/2018.1.SPINE17927
Sembrano, J. N., Polly, D. W., Ledonio, C. G. T., and Santos, E. R. G. (2012). Intraoperative 3-dimensional imaging (O-arm) for assessment of pedicle screw position: does it prevent unacceptable screw placement? Int. J. Spine Surg. 6, 49–54. doi: 10.1016/j.ijsp.2011.11.002
Senders, J. T., Zaki, M. M., Karhade, A. V., Chang, B., Gormley, W. B., Broekman, M. L., et al. (2018). An introduction and overview of machine learning in neurosurgical care. Acta Neurochir. 160, 29–38. doi: 10.1007/s00701-017-3385-8
Shin, J. H., Joo, E. Y., Seo, D. W., Shon, Y. M., Hong, S. B., Hong, S. C., et al. (2018). Prognostic factors determining poor postsurgical outcomes of mesial temporal lobe epilepsy. PLoS ONE. 13, e0206095. doi: 10.1371/journal.pone.0206095
Shultz, D. B., Modlin, L. A., Jayachandran, P., Von Eyben, R., Gibbs, I. C., Choi, C. Y., et al. (2015). Repeat courses of stereotactic radiosurgery (SRS), deferring whole-brain irradiation, for new brain metastases after initial SRS. Int. J. Radiat. Oncol. Biol. Phys. 92, 993–999. doi: 10.1016/j.ijrobp.2015.04.036
Sulc, V., Stykel, S., Hanson, D. P., Brinkmann, B. H., Jones, D. T., Holmes, D. R., et al. (2014). Statistical SPECT processing in MRI-negative epilepsy surgery. Neurology. 82, 932–939. doi: 10.1212/WNL.0000000000000209
Sun, K., Pheiffer, T. S., Simpson, A. L., Weis, J. A., Thompson, R. C., Miga, M. I., et al. (2014). Near real-time computer assisted surgery for brain shift correction using biomechanical models. IEEE J. Transl. Eng. Health Med. 2, 1–13. doi: 10.1109/JTEHM.2014.2327628
Szaflarski, J. P., Gloss, D., Binder, J. R., Gaillard, W. D., Golby, A. J., Holland, S. K., et al. (2017). Practice guideline summary: Use of fMRI in the presurgical evaluation of patients with epilepsy: report of the guideline development, dissemination, and implementation subcommittee of the american academy of neurology. Neurology. 88, 395–402. doi: 10.1212/WNL.0000000000003532
Vaughan, D. N., Rayner, G., Tailby, C., and Jackson, G. D. (2016). MRI-negative temporal lobe epilepsy: a network disorder of neocortical connectivity. Neurology. 87, 1934–1942. doi: 10.1212/WNL.0000000000003289
Walker, C. T., Kakarla, U. K., Chang, S. W., and Sonntag, V. K. (2019). History and advances in spinal neurosurgery: JNSPG 75th anniversary invited review article. J. Neurosurg. 31, 775–785. doi: 10.3171/2019.9.SPINE181362
Wong, C. H., Bleasel, A., Wen, L., Eberl, S., Byth, K., Fulham, M., et al. (2012). Relationship between preoperative hypometabolism and surgical outcome in neocortical epilepsy surgery. Epilepsia. 53, 1333–1340. doi: 10.1111/j.1528-1167.2012.03547.x
Wu, J. S., Zhou, L. F., Tang, W. J., Mao, Y., Hu, J., Song, Y. Y., et al. (2007). Clinical evaluation and follow-up outcome of diffusion tensor imaging-based functional neuronavigation: a prospective, controlled study in patients with gliomas involving pyramidal tracts. Neurosurgery. 61, 935–949. doi: 10.1227/01.neu.0000303189.80049.ab
Yang, X., Narasimhan, S., Luo, M., Thompson, R. C., Chambless, L. B., Morone, P. J., et al. (2019). Development and evaluation of a “trackerless” surgical planning and guidance system based on 3D Slicer. J. Med. Imaging. 6, 035002. doi: 10.1117/1.JMI.6.3.035002
Young, C. C., Bonow, R. H., Barros, G., Mossa-Basha, M., Kim, L. J., Levitt, M. R., et al. (2019). Magnetic resonance vessel wall imaging in cerebrovascular diseases. Neurosurg. Focus. 47, E4. doi: 10.3171/2019.9.FOCUS19599
Zijlmans, M., de Kort, G. A., Witkamp, T. D., Huiskamp, G. M., Seppenwoolde, J. H., van Huffelen, A. C., et al. (2009). 3T versus 1.5 T phased-array MRI in the presurgical work-up of patients with partial epilepsy of uncertain focus. J. Magn. Reson. Imag. 30, 256–262. doi: 10.1002/jmri.21811
Keywords: neuroimaging, neuronavigation, neurosurgery, neuro-oncology, cerebrovascular, spine, functional, epilepsy
Citation: Narasimhan S and González HFJ (2023) Survey of neuroimaging in neurological surgery, current state, and emerging research. Front. Neuroimaging 2:1022680. doi: 10.3389/fnimg.2023.1022680
Received: 18 August 2022; Accepted: 30 May 2023;
Published: 16 June 2023.
Edited by:
Xiao Liu, The Pennsylvania State University (PSU), United StatesReviewed by:
Anupa A. Vijayakumari, Cleveland Clinic, United StatesCopyright © 2023 Narasimhan and González. This is an open-access article distributed under the terms of the Creative Commons Attribution License (CC BY). The use, distribution or reproduction in other forums is permitted, provided the original author(s) and the copyright owner(s) are credited and that the original publication in this journal is cited, in accordance with accepted academic practice. No use, distribution or reproduction is permitted which does not comply with these terms.
*Correspondence: Hernán F. J. González, aDFnb256YWxlekBoZWFsdGgudWNzZC5lZHU=
†These authors have contributed equally to this work