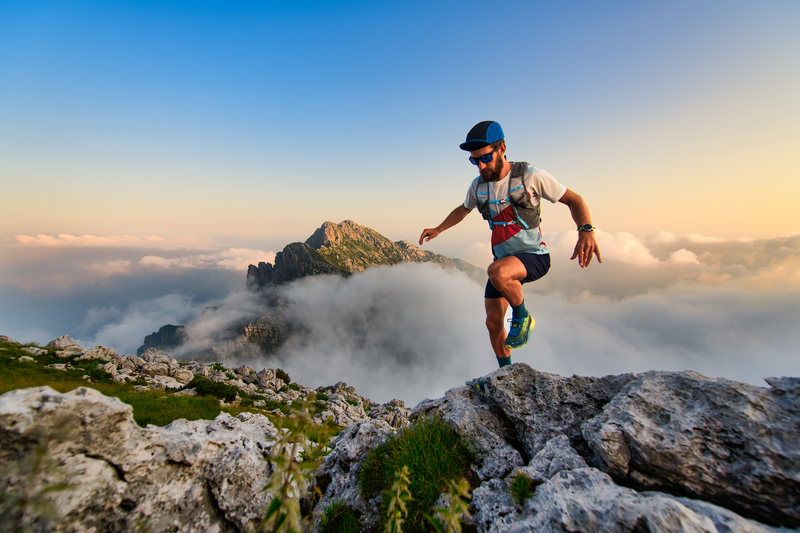
94% of researchers rate our articles as excellent or good
Learn more about the work of our research integrity team to safeguard the quality of each article we publish.
Find out more
ORIGINAL RESEARCH article
Front. Neuroergonomics
Sec. Cognitive Neuroergonomics
Volume 6 - 2025 | doi: 10.3389/fnrgo.2025.1472693
The final, formatted version of the article will be published soon.
You have multiple emails registered with Frontiers:
Please enter your email address:
If you already have an account, please login
You don't have a Frontiers account ? You can register here
Objective: This study aims to investigate the relationship between the subjective performance evaluations on pilot trainees' aircraft control abilities and their brainwave dynamics reflected in the results from EEG microstate analysis. Specifically, we seek to identify correlations between distinct microstate patterns and each dimension included in the subjective flight control evaluations, shedding light on the neurophysiological mechanisms underlying aviation expertise and possible directions for future improvements in pilot training.Background: Proficiency in aircraft control is crucial for aviation safety and modern aviation where pilots need to maneuver aircraft through an array of situations, ranging from routine takeoffs and landings to complex weather conditions and emergencies. However, the neurophysiological aspects of aviation expertise remain largely unexplored. This research bridges the gap by examining the relationship between pilot trainees' specific brainwave patterns and their subjective evaluations of flight control levels, offering insights into the cognitive underpinnings of pilot skill efficiency and development.Method: EEG microstate analysis was employed to examine the brainwave dynamics of pilot trainees while they performed aircraft control tasks under a flight simulator-based pilot training process. Trainees' control performance was evaluated by experienced instructors across five dimensions and their EEG data were analyzed to investigate the associations between the parameters of specific microstates with successful aircraft control.The experimental results revealed significant associations between aircraft control levels and the parameters of distinct EEG microstates. Notably, these associations varied 1 Zhao et al.across control dimensions, highlighting the multifaceted nature of control proficiency.G with aircraft control, emphasizing the role of attentional processes, perceptual integration, working memory, cognitive flexibility, decision-making, and executive control in aviation expertise. Conversely, negative correlations between microstate class C and class F with aircraft control indicated links between pilot trainees' cognitive control and their control performance on flight tasks.The findings underscore the multidimensional nature of aircraft control proficiency and emphasize the significance of attentional and cognitive processes in achieving aviation expertise. These neurophysiological markers offer a basis for designing targeted pilot training programs and interventions to enhance trainees' aircraft control skills.
Keywords: Aircraft control evaluations, Brain Dynamics, EEG microstate analysis, Correlation analysis, cognitive functions, Pilot training
Received: 16 Aug 2024; Accepted: 11 Feb 2025.
Copyright: © 2025 Zhao, Law, Su, Jennings, Bourgon, Jia, Larose, Bowness and Zeng. This is an open-access article distributed under the terms of the Creative Commons Attribution License (CC BY). The use, distribution or reproduction in other forums is permitted, provided the original author(s) or licensor are credited and that the original publication in this journal is cited, in accordance with accepted academic practice. No use, distribution or reproduction is permitted which does not comply with these terms.
* Correspondence:
Yong Zeng, Concordia University, Montreal, Canada
Disclaimer: All claims expressed in this article are solely those of the authors and do not necessarily represent those of their affiliated organizations, or those of the publisher, the editors and the reviewers. Any product that may be evaluated in this article or claim that may be made by its manufacturer is not guaranteed or endorsed by the publisher.
Research integrity at Frontiers
Learn more about the work of our research integrity team to safeguard the quality of each article we publish.