- 1CNRS, EPHE, INCIA, UMR5287, Université de Bordeaux, Bordeaux, France
- 2CLLE, Université de Toulouse, CNRS, Toulouse, France
- 3Université Paul Valéry Montpellier 3, EPSYLON EA 4556, Montpellier, France
- 4ToNIC, Université de Toulouse, INSERM, Toulouse, France
- 5Centre Hospitalier Universitaire Toulouse, Toulouse, France
Introduction: Strokes leave around 40% of survivors dependent in their activities of daily living, notably due to severe motor disabilities. Brain-computer interfaces (BCIs) have been shown to be efficiency for improving motor recovery after stroke, but this efficiency is still far from the level required to achieve the clinical breakthrough expected by both clinicians and patients. While technical levers of improvement have been identified (e.g., sensors and signal processing), fully optimized BCIs are pointless if patients and clinicians cannot or do not want to use them. We hypothesize that improving BCI acceptability will reduce patients' anxiety levels, while increasing their motivation and engagement in the procedure, thereby favoring learning, ultimately, and motor recovery. In other terms, acceptability could be used as a lever to improve BCI efficiency. Yet, studies on BCI based on acceptability/acceptance literature are missing. Thus, our goal was to model BCI acceptability in the context of motor rehabilitation after stroke, and to identify its determinants.
Methods: The main outcomes of this paper are the following: i) we designed the first model of acceptability of BCIs for motor rehabilitation after stroke, ii) we created a questionnaire to assess acceptability based on that model and distributed it on a sample representative of the general public in France (N = 753, this high response rate strengthens the reliability of our results), iii) we validated the structure of this model and iv) quantified the impact of the different factors on this population.
Results: Results show that BCIs are associated with high levels of acceptability in the context of motor rehabilitation after stroke and that the intention to use them in that context is mainly driven by the perceived usefulness of the system. In addition, providing people with clear information regarding BCI functioning and scientific relevance had a positive influence on acceptability factors and behavioral intention.
Discussion: With this paper we propose a basis (model) and a methodology that could be adapted in the future in order to study and compare the results obtained with: i) different stakeholders, i.e., patients and caregivers; ii) different populations of different cultures around the world; and iii) different targets, i.e., other clinical and non-clinical BCI applications.
1. Introduction
Brain-Computer Interfaces (BCIs) are technologies that enable users to control applications such as video games (Kerous et al., 2018) or wheelchairs (Li et al., 2013), solely through their brain activity. Beyond these control applications, BCIs can be used for neurofeedback (NF) training with the objective of learning how to modulate our own cerebral activity, not in order to control something, but to improve or restore cognitive or motor skills. BCI-based post-stroke motor rehabilitations are in this second category and have demonstrated their efficacy to improve patients' motor and cognitive abilities (Cervera et al., 2018; Bai et al., 2020; Nojima et al., 2022). In the coming years, they are expected to substantially improve post-stroke subjects' quality of life (Nojima et al., 2022).
In classical motor rehabilitation, when subjects have no residual movement, i.e., when they cannot move their affected limb at all, physical practice is impossible and both subjects and therapists must rely on mental practice alone. Here, in mental practice, we include motor imagery (MI) as well as attempted movements. In concrete terms, therapists usually ask the subjects to perform MI or to try to move their arm (attempted movements), and simultaneously stimulate the limb by mobilizing it or, for instance, by using functional electrical stimulation (FES-which consists in stimulating peripheral motor nerves in order to artificially generate movements). While associated to encouraging results (Sharma et al., 2006), the difficulty encountered when trying to demonstrate the efficiency of this procedure might be related to the impossibility to assess the patients' compliance when they are asked to perform MI tasks (Sharma et al., 2006). In addition, we believe pure mental practice-based rehabilitation procedures present two main limitations. The first one is due to the impossibility of the therapist to know when, exactly, the patient imagines moving or tries to move. Therefore, the feedback patients are provided with will most likely not be synchronized with their MI or movement attempts. A second limitation concerns the constant reminder that the patient gets when the therapist asks them to move their arm and that they are unable to do so. Post-stroke subjects experiencing high anxiety levels (Burton et al., 2013), this method might also have detrimental psychological effects, potentially resulting in the patient disengaging from the rehabilitation procedure, and in the therapy being less efficient.
In this context, BCIs are very relevant as they enable the detection of MI/attempted movements of the impaired limb, which are underlain by modulations of the so-called sensori-motor rhythms (SMRs)—as defined in the BCI field by a large band covering mu (μ) and beta (β) rhythms (8–30 Hz) (Pfurtscheller et al., 2000)—, and provide the patient with a synchronized NF, for instance using FES that triggers an arm muscle contraction, or visual feedback [movement of a virtual hand on a screen (Pichiorri et al., 2015)]. Such a NF training enables the participants to train to voluntarily self-regulate their SMRs in a closed loop process, which should favor synaptic plasticity and motor recovery (Jeunet et al., 2019).
While this is encouraging, BCI efficiency is still far from the level required to achieve the clinical breakthrough expected by both clinicians and patients. Thus, BCIs remain barely used in clinical practice, outside laboratories (Kübler et al., 2014). BCI efficiency is known to be modulated by several factors. Many researchers are working on improving this efficiency either from a “technical” point of view (e.g., signal processing Lotte et al., 2018), or—less often—from the human learning standpoint (Pillette et al., 2020; Roc et al., 2021). This is an important step forward: reaching high efficiency is a necessary condition for BCI adoption. Nonetheless, it might not be sufficient for those technologies to be actually used in a clinical setting: fully optimized BCIs (in terms of sensors, signal processing, and training procedures) are pointless if patients and clinicians are not able or do not want to use them, i.e., if BCIs are not accepted (Blain-Moraes et al., 2012). For instance, misconceptions that patients and their entourage have regarding BCIs may have a detrimental effect on the acceptance of these technologies. BCI acceptance could also be altered by the fact that most stroke patients experience depression, and therefore high anxiety levels (Burton et al., 2013) that have a detrimental effect on BCI acceptance and learning (Jeunet et al., 2016). Thus, BCI acceptance is likely to have a major impact on the patients' learning processes and therefore on the efficiency of BCI-based stroke rehabilitation procedures. We hypothesize that identifying acceptability and acceptance factors will help us overcome these misconceptions and personalize the procedures, which will in turn result in reduced anxiety, and increased motivation and engagement levels for the patients. This should favor their learning and, ultimately, motor recovery. In other words, we expect that improving the acceptance levels of BCIs will result in an increased efficiency of these technologies and therefore contribute to their democratization.
Thus, it is crucial, when designing stroke rehabilitation procedures, to consider technology acceptance as a lever to optimize BCI efficiency—in terms of motor recovery. Yet, BCI acceptance remains an aspect that has been little studied to date. To the best of our knowledge, only (Morone et al., 2015) assessed the relevance of a BCI-based stroke rehabilitation procedure of the upper limb using acceptability and usability measures as primary criteria (pilot study, N = 8 patients). The acceptability and usability were measured in terms of mood, motivation, satisfaction, and perceived workload. Indeed, in the BCI field, acceptability is mostly assessed as an attribute of the user's satisfaction, itself being a dimension of user experience (Kübler et al., 2014; Nijboer, 2015). Morone et al. (2015) concludes that the BCI training was “accepted with a good compliance/adherence.” The same conclusion was drawn in the context of a BCI dedicated to a gamified cognitive training for the elderly (Lee et al., 2013) as well as in different studies dedicated to the acceptance of BCIs by Amyotrophic Lateral Sclerosis (ALS) patients (Huggins et al., 2011; Blain-Moraes et al., 2012; Nijboer, 2015), which is the clinical condition for which acceptance has been the most investigated (Nijboer, 2015). Using a focus group approach, Blain-Moraes et al. (2012) have shown that both personal and relational factors impacted BCI acceptance. The personal factors included physical (pain, discomfort), physiological (fatigue, endurance) and psychological (anxiety, attitude toward the technology) concerns, and the relational factors included corporeal (electrode type), technological (relationship between BCI and other type of software and hardware) and social (appearance, training and support personnel) factors. The relational factors had a stronger impact than the personal ones. In the same line, Huggins et al. (2011, 2015) led qualitative studies to assess the influence that different factors (physical interface, setup and training, acceptable performance, task and feature priorities) had on BCI acceptance in ALS patients and patients who had undergone a spinal cord injury. The functions provided by the BCI were rated as the most important feature, together with the ease of use of the system and the availability of a stand-by mode. Finally, Geronimo et al. (2015) have shown that behavioral impairments such as apathy and mental rigidity had a negative impact on ALS patients' BCI usage behavior. Furthermore, the fact that they performed a pilot study during which patients appeared to have a low perceived control over the system altered the perceived usefulness of the BCI. This latter study is the only one in the field of BCIs that assessed acceptance in terms of usage behavior and perceived usefulness. These are concepts from the field of psychology and ergonomics, which we draw on in the next paragraph (Kaleshtari et al., 2016). As claimed by Kaleshtari et al. (2016), who designed the first Model of Rehabilitation Technology Acceptance and Usability (RTAU), in order to be effective, rehabilitation technologies have to be used and therefore accepted by the patients and their families. According to Kaleshtari et al. (2016), this acceptance depends both on personal features, technology features, and social influence. The domain-specific literature indeed suggests that BCI acceptability and acceptance seem to rely on “subjective technical confidence and positive attitudes toward the use of technologies” (Morone et al., 2015). The EEG cap characteristics (gel, montage, and time to set up) seem important both for patients and caregivers (Morone et al., 2015; Nijboer, 2015). Generally speaking, the simpler the better for them (Huggins et al., 2011). Nonetheless, the EEG technology used and the reliability/discomfort trade-off, together with all the BCI-related characteristics, need to be thought of in light of the characteristics and requirements of the application (e.g., level of reliability required) as well as in light of the profile of the patient. This is why BCI-based stroke rehabilitation procedures should be carefully adapted to the training context of each patient. In this spirit, Kübler et al. (2014) proposed an inspiring approach, suggesting to “shift from focusing on single aspects, such as accuracy and information transfer rate, to a more holistic user experience” using User-Centered Design. User-Centered Design has since then been shown to contribute to the acceptability and usability of a BCI-based stroke rehabilitation procedure (Morone et al., 2015) and, more broadly, improved user experience has been suggested to enhance user acceptance and increase the performance of BCI systems (Gürkök et al., 2011).
The concepts of acceptability and acceptance were introduced in order to understand what led users to adopt or not a new system (Alexandre et al., 2018). The adoption of a technology refers to a use that is maintained over time, i.e., without abandonment. In concrete terms, acceptability measure is an evaluation of the user's behavioral intention (BI) i.e., their intention to use the studied technology. The main determinants of BI are perceived usefulness (PU) and perceived ease of use (PEOU). PU is the personal feeling about utility of the system, and PEOU the degree of belief to which using the system will require little or no effort. Acceptability and acceptance differ by the moment they are measured at: acceptability concerns the user's standpoint before any interaction with the system, while acceptance comes after at least one first use.
To the best of our knowledge, there is no model of BCI acceptability yet. Thus, our goals were to (i) create a theoretical model of acceptability—based on the literature—including the factors influencing BI toward BCIs, especially in the context or motor rehabilitation after stroke; (ii) implement a questionnaire from this model to assess BCI acceptability in general public; (iii) validate the structure of our model and questionnaire; and (iv) quantify the impact of the different factors included in the model on acceptability.
With this study we targeted the general public. This enabled us to collect the opinions and attitudes of a large sample of persons, representative of the adult population in France, and thereby to capture an estimation of BCI acceptability in the overall population that we will, in the future, compare with the one of patients and clinicians. Targeting the general public seems particularly relevant in this case for two reasons. First, due to the high prevalence of stroke that is one of the leading causes of disability in adults in France (Accident Vasculaire cérébral, 2019) and results in many of us being concerned, more or less directly, by this pathology and associated rehabilitation techniques. Second, due to the fact that the opinion and attitude of their close relatives will influence the patients' acceptability levels (Venkatesh et al., 2003).
In this paper, we first explain our methodology to achieve these objectives in Section 2: (i) the design of the model, (ii) the implementation of the associated questionnaire, (iii) the validation of these model and questionnaire, and (iv) the quantification of the influence of the different factors included in the model on BCI acceptability. Then, we present the results of this methodology in Section 3, which are based on data collected on a sample representative of the population of France (N = 753). We finish with a discussion about limitations and benefits of our research.
2. Materials and methods
2.1. Design of the acceptability model
2.1.1. Review of the literature
To build our model, we reviewed the literature and selected the models that seemed the most relevant for BCIs.
In the literature, several models dedicated to the acceptability and acceptance of technologies have been depicted, most of them can be adapted depending on the focus (i.e., acceptability or acceptance). Their objective is usually to explain, or even predict, the BI of the user. These models differ from each other in the factors they include and that influence acceptance/acceptability. The most recent and main models are the technology acceptance model (TAM) (Davis, 1989), of which there are a second (Venkatesh and Davis, 2000) and third (Venkatesh and Bala, 2008) versions, as well as the unified theory of acceptance and use of technology (UTAUT) (Venkatesh et al., 2003), of which there is a second version (Venkatesh et al., 2012). For more information about other existing acceptability models, their evolution is summarized in a recent review (Pillette et al., 2022).
2.1.2. Methodology to build our model
We have chosen to work with the most advanced versions of the evoked models: TAM3 (Venkatesh and Bala, 2008) and UTAUT2 (Venkatesh et al., 2012), in addition to a less widespread model, the components of user experience (CUE) model (Thüring and Mahlke, 2007), because we wanted up-to-date models that were adapted to our context and as exhaustive as possible. We propose to present these latter and the details of why we chose them in Table 1.
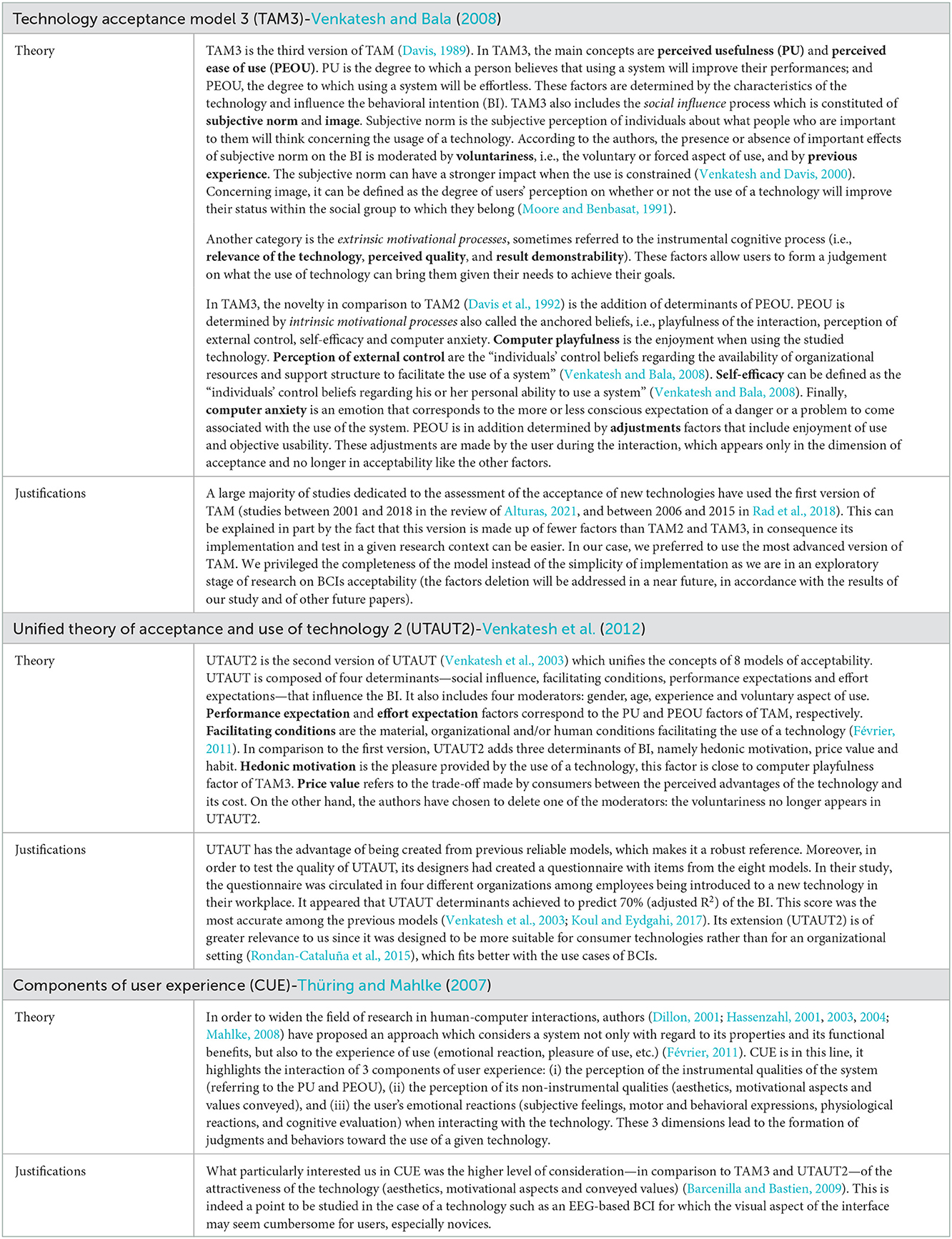
Table 1. Acceptability and acceptance models used to build our model for BCI-based post-stroke motor rehabilitation.
Using the models presented in Table 1, our work was to identify their factors that seemed relevant in the context of BCI-based post-stroke motor rehabilitation, while reflecting on missing determinants that are however useful to assess with regard to the literature on BCIs.
2.2. Creation and distribution of the questionnaire
As we explained in introduction, from our acceptability model, we developed a questionnaire to identify and weight the factors influencing the acceptability of BCI-based post-stroke motor rehabilitation within the general population. The choice to rely on the questionnaire method to test our model is explained by several aspects: (i) It is the classic method used in acceptability or acceptance assessment (Davis, 1989; Venkatesh et al., 2003). (ii) It was necessary to be able to collect a large amount of data, on a sample representative of the adult population in France, and questionnaires are particularly adapted to these expectations (Vilatte, 2007). (iii) In addition, questionnaires offer good external validity (Ghiglione and Matalon, 1998), which makes it possible to generalize the data, the information being more uniform than interviews results.
2.2.1. Creating the questions
To determine the wording of the questions, we adapted those of the questionnaires of the existing models already translated into French. The questionnaire was created on the Qualtrics tool, it was fully anonymous, and therefore not subject to the general data protection regulation (GDPR). It took approximately 15 min to be completed and consisted of four parts:
• To start, participants were provided with all the information they should know about the research project: objectives of the questionnaire, researchers involved, benefits and possible risks of filling the questionnaire, rights (e.g., anonymity preserved), methodology used and estimated completion time. Finally, the participants were asked if they consented to participate.
• Following these details, the experience of the participant with BCIs was assesed as it could have an influence on some of the predictive factors of BI.
• The third part was devoted to the evaluation of the influence of the factors of our model. Each of them was evaluated by up to three or five questions. The score of a factor was thus the average of the scores of these different questions. The scale used to measure each of the quantitative factors was a visual analog scale (VAS) from 0 to 10 (“strongly disagree” to “strongly agree”). When a question was negative (e.g., “I think learning to use a brain-computer interface would be too time consuming”), we inverted its score. To measure the categorical factors (computer self-efficacy and social support), we used checkbox questions.
• In this part, we also introduced two explanatory videos edited by ourselves: one explaining the operation of BCIs in general (video 1) and the second more specific to BCI-based stroke rehabilitation procedures (video 2). The rationale of providing these explanatory videos is presented in the next paragraph.
• We provide in Figure 1 more details on the organization of our questionnaire. The questions were organized in blocks (a block is made up of 2 or more factors). To avoid any potential order effect, i.e., that the previous questions would guide the following answers, the order of presentation of the questions was randomized within each block.
• The last part concerned the socio-demographic characteristics of the respondents (age, gender, last diploma obtained, socio-professional category, if they have had a stroke and are currently hospitalized for it, or if they have people in their close circle who have experienced it and their involvement in the rehabilitation of these relatives). Subjects were not obligated to position themselves, they had the possibility to choose the option “I do not wish to answer.”
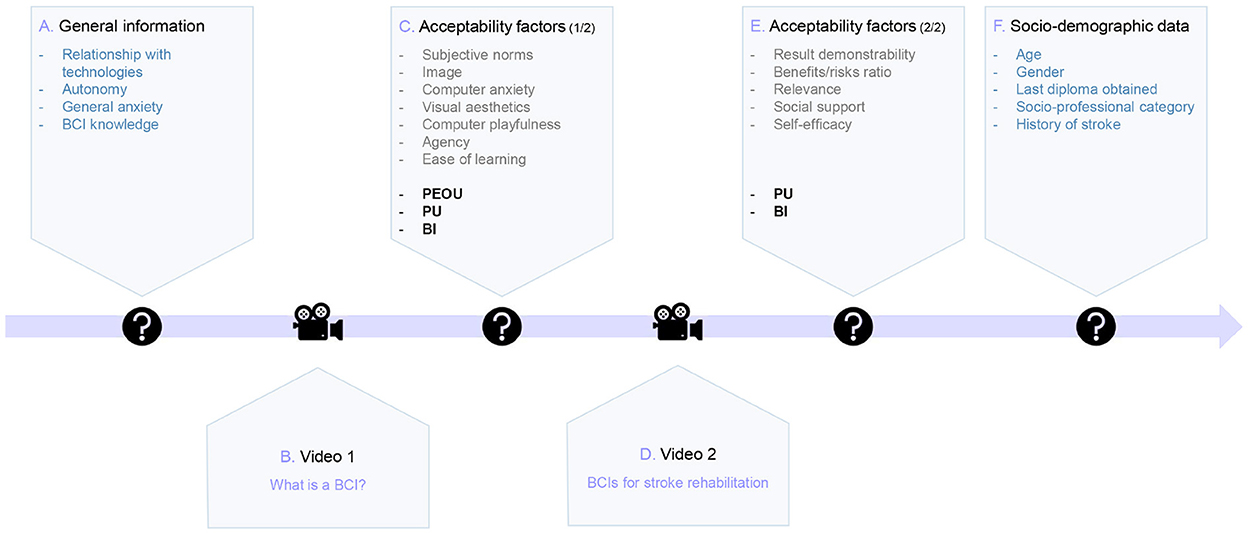
Figure 1. Schematic representation of the structure of the questionnaire: (A) Assessment of the respondents' traits and general knowledge about BCIs; (B) Presentation of the first video that aimed at providing basic information regarding BCIs (functioning, installation, etc.); (C) Items related to a subset of acceptability factors (1/2); (D) Presentation of the second video during which the application of BCIs for post-stroke motor rehabilitation was introduced. (E) Items related to a subset of acceptability factors (2/2); (F) Collection of socio-demographic data. The two subsets of acceptability factors were divided depending on the need for respondents to have knowledge about how BCIs could be used for stroke rehabilitation.
Concerning the factors, we evaluated different factors before and after the second video (Figure 1). PU and BI were measured twice (before video 2: PU1/BI1; after video 2: PU2/BI2), the questions were the same for the two moments. Our aim was to observe if the respondents' scores for the two measures were impacted by information given in the video. The factors before video 2 did not require to have plenty of information on BCIs to answer, i.e., the respondents needed to understand what BCIs are but remained novices on their use in post-stroke rehabilitation. On the other hand, for the factors following video 2, a more detailed vision of these new rehabilitation procedures was needed (factors were result demonstrability, benefits/risk ratio and relevance). Questionnaire (French and English versions) is available in Supplementary material 1.
2.2.2. Calculation of the statistical power
Our initial target was to have at least 10 respondents per item (i.e., question) on the factors influencing acceptability in order to be able to perform reliable analyzes (Kline, 2015). As we had 62 items, this gave us a sample size of N = 620 to respect this prerequisite.
2.2.3. Distribution of the questionnaire
The distribution of the questionnaire was done by the company Panelabs (https://fr.panelabs.com/) to ensure that the sample of respondents was representative of the adult population in France in terms of age, gender, place of residence and socio-professional category. We had a single exclusion criterion: minors could not participate. The experimental protocol was carried out in accordance with the Declaration of Helsinki and was approved by Institutional Review Board of Toulouse Federal University (N°2019-140). We fixed with Panelabs a sample of N = 665 minimum in order to have a few more respondents than our aim (i.e., N = 620), in case of invalid responses.
2.3. Validation of the structures of the model and questionnaire
The assessment of the validity of the structure of our model and questionnaire was performed following two steps. First, we measured the “within-factor” consistency, i.e., the internal coherence between the different items of the questionnaire that measured the same factor. Second, we assessed the “between-factor” consistency, i.e., the validity of the structure of our model.
2.3.1. Coherence of the factors: Cronbach's alpha
The Cronbach's alpha coefficient allowed us to calculate the internal consistency of each factor. Concretely, this metric estimates the extent to which the items that are meant to measure one same factor are associated with coherent scores. There is no fixed rule on the minimal value of the coefficient for the internal consistency of the factor to be considered satisfactory. Nevertheless, the value 0.7 comes up very often in the literature (Nunnally, 1994; Bland and Altman, 1997; DeVellis and Thorpe, 2021). It is also indicated that a coefficient too close to 1 is to be taken with precaution, this high value may be due to redundancy in the question statements (Tavakol and Dennick, 2011). In other words, the items would be too similar one from the other, and do not bring additional information.
2.3.2. Structure of the model and questionnaire: Confirmatory factor analysis
Confirmatory factor analysis (CFA) is a validation test, which also aims to verify the internal consistency of the questionnaire and check whether the model we propose fits correctly with the data collected. Several indicators are used to interpret the CFA (Gallagher and Brown, 2013): (i) The chi-square (X2) test which has the null hypothesis that the model fits perfectly. A good fit is shown by a p > 0.05 (i.e., not significant). This test is not always reliable on large samples because it is very sensitive to size. (ii) The comparative fit index (CFI) estimates to what extent the tested model is better than the independence model (i.e., the model where each of the factors are independent and uncorrelated). Ideally, this score should be higher than 0.95 (perfect) or at least better than 0.90 (acceptable). (iii) The Tucker-Lewis index (TLI) is very close to CFI, it evaluates the degree to which the model improves the fit with respect to the independence model. For example, if the TLI is equal to 0.95, the studied model improves the fit by 95% compared to the independence model. As CFI, this score should be higher than 0.95 or at least better than 0.90. (iv) The root mean square error of approximation (RMSEA) is the index of poor fit of the tested model. The smaller the RMSEA, the better the goodness of fit. It is thus preferable to have the smallest possible value of RMSEA (preferably less than 0.05). (v) Finally, we can also look at the standardized root mean squared residual (SRMR), this latter must be < 0.08. It measures the difference between the correlation matrix of the observed sample and the matrix predicted by the model.
2.4. Quantification of the impact of the different factors on BCI acceptability
2.4.1. Important factors in each category of our model: Mediation analysis
As one of our main aims was to determine the most influential determinants of PU, PEOU, and BI, we chose to perform mediation analyzes. This analysis is a rearranged linear regression, its objective being to decompose and quantify the total effect of a cause X on a response variable Y into a direct effect and an indirect effect through the mediator(s). This method was very relevant in our context: we had an acceptability model with independent variables, moderators (PU and PEOU) and a target variable (BI). We did one mediation analysis per category in our model (i.e., social influence, individual differences, facilitating condition, and system characteristics—these categories are depicted in Section 3.1), in order to see which factors had the most impact in each of them. This analysis was also an interesting step to enable us to propose a shorter and simplified version of our model and questionnaire in the future, one with only the most relevant variables. The mediate library from R “psych” package was used (Revelle, 2021).
2.4.2. Important factors independently of structure of the model: Random forest algorithm
After mediation analyzes, we wanted to do additional observations that do not depend on the architecture of our proposed model. We thus opted for the random forest (RF) algorithm. The principle of this algorithm is to randomly build multiple decision trees and train them on different subsets of our data. Thus, instead of trying to obtain an optimized method at once, we generate several predictors before pooling their different predictions. The final estimation is obtained, in the case of a regression as for this study, by taking the average of the predicted values. RF algorithms have the advantage of being non-parametric tests allowing the combination of quantitative and qualitative data, and making it possible to identify the factors associated with the bigger weights.
2.4.3. Intensity of the connections between factors: Correlation analysis
After using the RF algorithm, we looked at the correlations between the most salient factors which stood out. Our objective was to see if the correlations between these factors were rather positive or negative in order to understand the meaning of their relationship (RF algorithm does not provide the strength of the connection between factors and target variable). To build the correlation matrix, a non-parametric method (Spearman's coefficient) was applied (Kowalski, 1972), and p-values were adjusted using the Bonferroni method.
3. Results
In this section, we present the acceptability model we built, the results of the questionnaire, our analyzes to validate these latter and finally those for quantifying the impact of the different factors on BCI acceptability.
We performed these analyzes using data from Qualtrics, after measuring the average score of each factor for every respondent (as explained in Section 2.2, a factor is measured by several questions which we had to average). For the qualitative/categorical variables, we calculated the number of occurrences of the sub-modalities.
3.1. Design of the acceptability model
We introduce here the theoretical model of acceptability dedicated to BCI-based post-stroke rehabilitation procedures that we have created. To design this model, we selected factors from those of the existing models presented in Table 1, using studies on BCIs to estimate their suitability in our context. To these current factors, we have added new ones that seem particularly relevant to BCIs, still basing ourselves on the BCIs literature. We present in this section the factors we included in our model (Table 2 contains definition and justifications of our choices) and its structure (Figure 2).
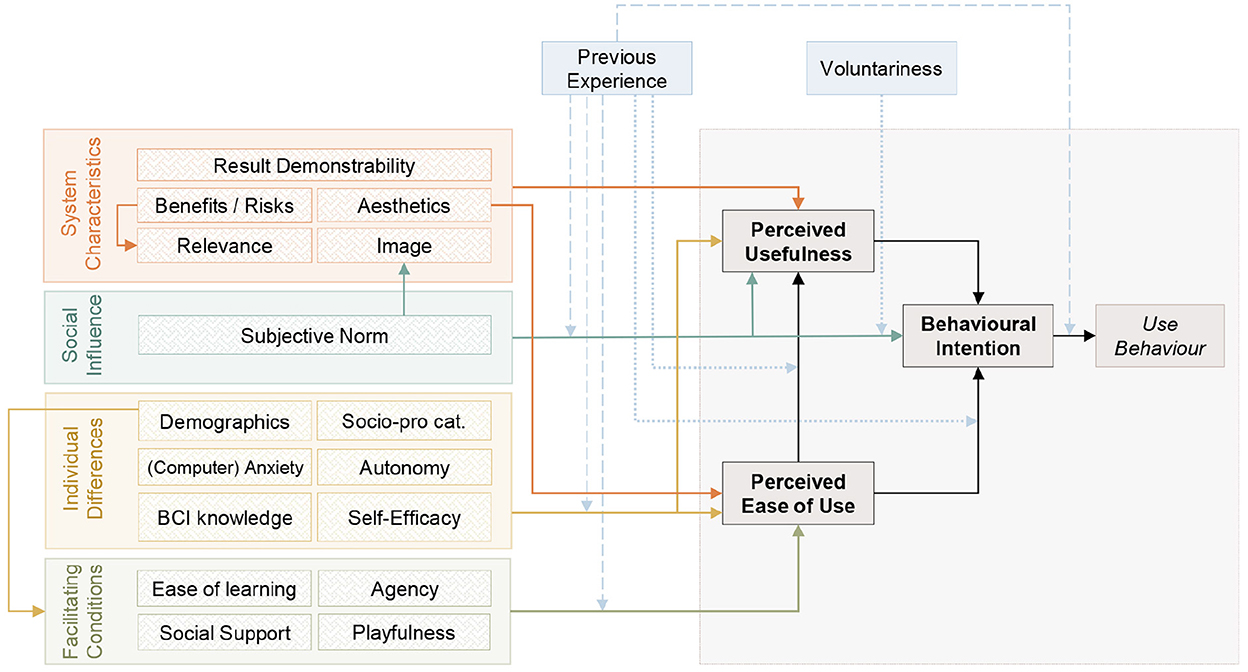
Figure 2. Representation of the tentative model of acceptability of BCIs for motor rehabilitation after stroke. On the right (in gray) are the target factors from TAM3 namely, PU, PEOU and BI. On the left are the four categories of factors that may influence the target factors: system characteristics (orange), social influence (turquoise), individual characteristics (yellow) and facilitating conditions (green). Each category includes one or more factors, themselves assessed in the questionnaire by 3–5 items. Solid arrows represent the potential influence of those categories on the target factors. Finally, on top, two moderators are represented in blue. Those factors moderate the effect of the different categories on the target factors. Dotted lines represent moderation effects presented in TAM3 while broken lines represent effects depicted in UTAUT2 (or in both).
Each factor is classified into a category: social influence, individual differences, facilitating conditions, and system characteristics. These categories are inspired by TAM3 (Venkatesh and Bala, 2008) and UTAUT2 (Venkatesh et al., 2012). Social influence, as defined in the TAM2 and 3, is the influence of an individual's relatives and social group on their choice of whether or not to adopt a system. It is a determinant of PU and BI. Its effect on BI and PU decreases with experience (according to TAM3 and UTAUT2, and only to TAM3, respectively). Individual differences is a category which groups the user personal characteristics (socio-demographic information, cognitive traits and personality). Its factors are determinants of PU and PEOU. We hypothesize that the weight of the factors of this category decrease with experience as the effect of computer anxiety on PU and PEOU decreases with experience (TAM3). Facilitating conditions brings together the factors related to the material, organizational and/or human conditions that facilitate the use of a technology (Février, 2011). This category is a determinant of PEOU (TAM3). Its impact is lessened while users acquire experience with the technology as their dependence toward external support will be reduced (Alba and Hutchinson, 1987). Finally, system characteristics is a category related to the instrumental cognitive process introduced by the TAM2. It is the mental representation developed by the user to judge what the use of a technology can bring them in relation to their objective(s) (relevance of the system, perceived quality, etc.) (Terrade et al., 2009). This category influences PU (TAM3) and in addition, among this category, visual aesthetics also influences PEOU because this factor comes from the CUE model which assumes that its effect is not limited to PU.
3.2. Results of the questionnaire
3.2.1. Participants
We managed to obtain a set of N = 753 respondents to our questionnaire based on the model. This sample was representative of the composition of the adult population in France. We provide the socio-demographic details in Table 3.
95.8% of our sample had never used BCIs—including 68.7% that didn't hear about BCIs before this questionnaire. This lack of knowledge was consistent with our objectives because it is more relevant to have novice users when measuring acceptability—as it should be before any interaction with the technology. In consequence, we didn't discuss in detail the previous experience moderator of our model in this paper, as we didn't have enough expert respondents to differentiate inexperienced/experimented users.
3.2.2. Descriptive analysis
In Table 4, we present the mean scores of each quantitative factor, and the percentages for categorical factors. None of the factors was associated with a score below 5/10, which reflects globally positive feelings and well-perceived BCIs among the respondents. Indeed, regarding the target factors, BI2 had a mean of 8.23 (SD = 1.69), for PU2 it was 8.28 (SD = 1.57) and for PEOU the mean was 7.17 (SD = 1.57).
As explained in Section 2.2, our questionnaire contained two videos. We wanted to verify if, depending on the richness of information provided to people about BCIs in rehabilitation (possibilities of use, expected results, etc.), the factors that most impact BI and PU are or not the same. In this aim, we compared the means of the two paired samples (before/after the video explaining how BCIs could be integrated in stroke rehabilitation, i.e., video 2) for BI and PU. Wilcoxon test with Bonferroni correction was used, it evaluated if there was a significant difference between the values of PU1/PU2 and BI1/BI2. We didn't measure PEOU twice, even if it is one of the main determinant of BI in literature, because the users' viewpoint about the functioning of BCI remains the same as long as they had never have the opportunity to actually test the interface before.
Wilcoxon test showed that the scores of BI1 and PU1 were significantly different from BI2 and PU2 respectively (see Figure 3). As pointed out in Table 4, the means were higher after the video, which seemed to have a positive impact on the respondents' standpoint about BCI (Before video 2: PU1 mean = 7.87, SD = 1.63/BI1 mean = 7.88, SD = 1.73. After video 2: PU2 mean = 8.28, SD = 1.57/BI2 mean = 8.23, SD = 1.69). The score of PEOU was also high (mean = 7.17, SD = 1.57).
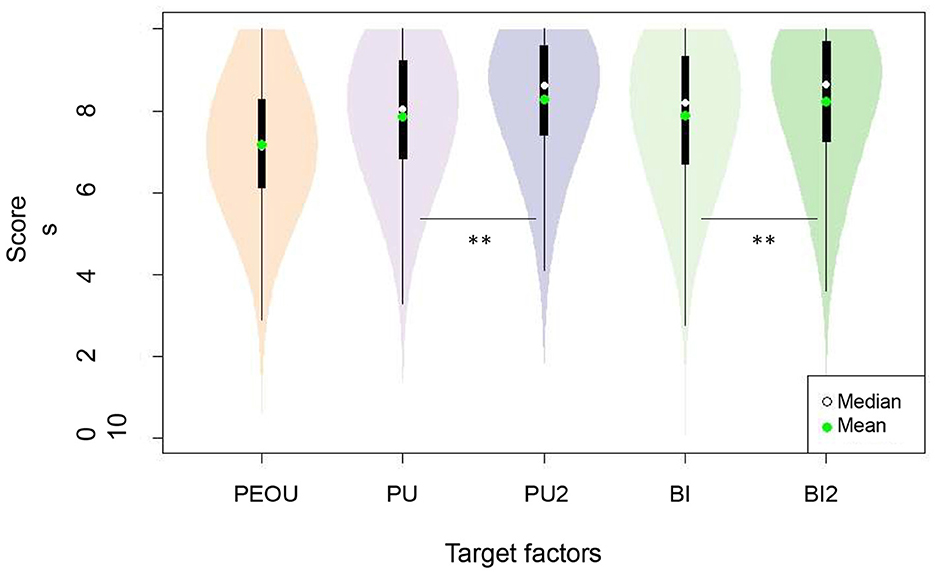
Figure 3. Distribution of the scores as a function of the different target factors. Paired Wilcoxon test: for the [PU1-PU2] and [BI1-BI2] pairs, we obtained a p < 0.001 (with Bonferroni correction), each factor was therefore significantly different. The ** symbol indicated to show that the factors were significantly different (i.e., PU/PU2 and BI/BI2).
3.3. Validation of the structures of the model and questionnaire
3.3.1. Coherence of the factors: Cronbach's alpha
Cronbach's alpha analyzes (Table 5) show that 13/17 factors had a satisfactory internal consistency, with scores comprised between 0.72 and 0.97. Among the four other factors, the scores were the following: 0.5 (agency), 0.52 (autonomy), 0.57 (ease of learning) and 0.62 (benefits/risk ratio).
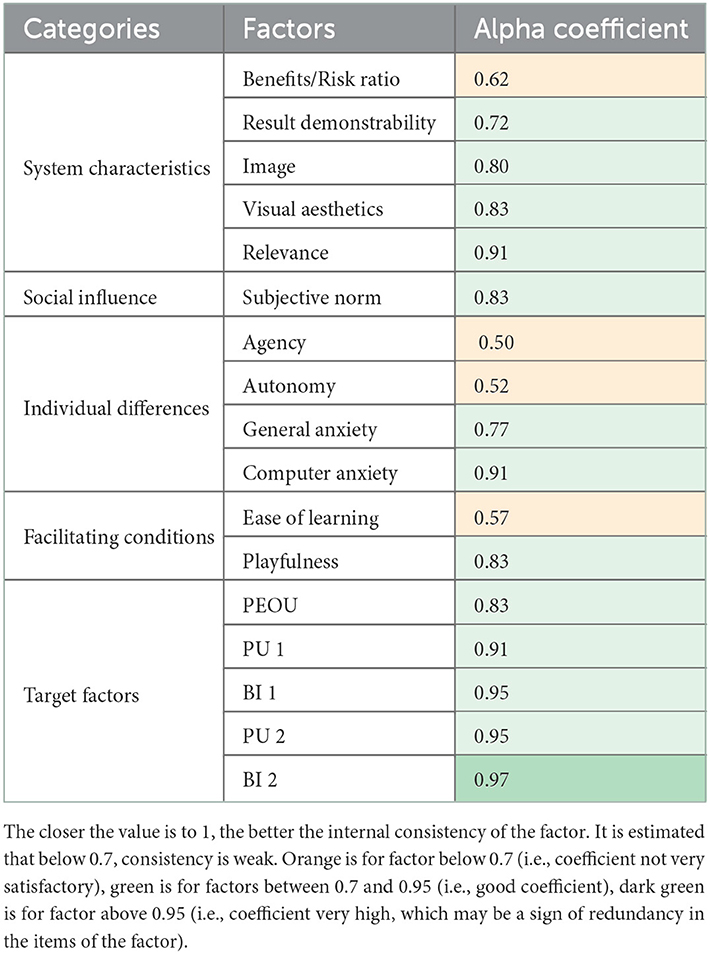
Table 5. Cronbach's alpha reliability values for the questionnaire based on our acceptability model.
3.3.2. Structure of the model: Confirmatory factor analysis
Regarding the CFA results, we obtained a p-value of 0.0 for the chi-square test. This means that the hypothesis of the perfect fit of the model to our data is rejected. Nevertheless, this can be explained by the large size of our sample. The comparative fit index (CFI) value was 0.913 and the Tucker-Lewis index (TLI) value 0.897, which indicates a good fit between the model and the data. Indeed, these scores mean that our model is better than the independence model. The RMSEA, which is the index of poor adjustment of the model, should ideally be below than 0.05. Results indicated a value of 0.059, with a confidence interval ranging from 0.056 to 0.062. It was thus close to the expected value. Finally, our SRMR was 0.076 (i.e., < 0.08, as expected as this test assesses the divergence between observed and expected correlations).
3.4. Quantification of the impact of the different factors on BCI acceptability
3.4.1. Important factors in each category of our model: Mediation analysis
Table 6 presents the different results we obtained following the mediation analyzes. It should be noted that the categorical factors are not presented here (demographics, self-efficacy, BCI knowledge and social support), we studied only the quantitative variables because our categorical variables were not binary, so it was not adapted to this method. Nevertheless, they are not left out, we present an analysis included them with RF algorithm in Section 3.4.2.
Figure 4 shows that the BI was mainly influenced by PU2 (effect: 0.94, p < 0.001), the weight of the PEOU being much lower (direct effect: 0.08, standard error SE = 0.02, p < 0.001; indirect effect: 0.65, SE = 0.03, CI = [0.59, 0.71]), i.e., PEOU had a low effect on BI2 but a significant effect on PU2.
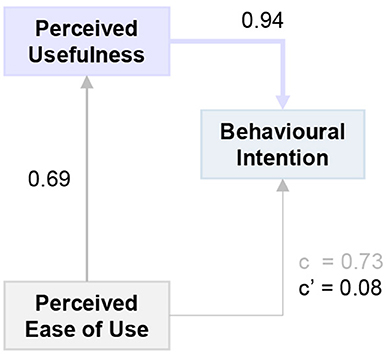
Figure 4. Mediation analysis for the target factors: Behavioral intention (BI2), Perceived usefulness (PU2) and Perceived ease of use (PEOU). R2 = 0.86 (p < 0.001). c, total effect of PEOU on BI2; c', direct effect of PEOU on BI2; c-c', indirect effect of PEOU on BI2 through PU2.
Concerning the other categories of our model, our results revealed that for the individual differences, autonomy was the most influential factor on BI, but this effect was moderate (c = 0.34, p < 0.001), it equally impacted PU2 and PEOU (respectively, 0.36 and 0.33, with p < 0.001) (quality of the model: R2 = 0.87, p = 0.0).
For social influence, subjective norm had a similar and moderate impact on both PU2 and PEOU (respectively, 0.58 and 0.57, with p < 0.001). The influence on BI2 was rather high (c = 0.63, p < 0.001) (quality of the model: R2 = 0.86, p < 0.001).
For characteristics of the system, we did two analyzes: (i) one with only PEOU as mediator, and factors present before video 2 (PU1 was not included since we chose to focus on PU2); (ii) the second only with PU2 as mediator, and factors present before and after video 2 (PEOU was among these factors since, as shown in Figure 2, it influences PU). (i) shows that visual aesthetics was the most—but weak—influential factor on PEOU (0.38, with p < 0.001). The total effect of visual aesthetics on BI2 was low: C = 0.32 (p < 0.001) (quality of the model: R2 = 0.47, p < 0.001). On the other hand (ii) revealed that relevance was the most influential factor on PU2 (0.65, with p < 0.001). Its total effect on BI2 was C = 0.56 (p < 0.001) (quality of the model: R2 = 0.87, p = 0.0).
Finally, for facilitating conditions, the variable with most impact was computer playfulness, it equally impacted PU2 and PEOU (respectively, 0.36 and 0.39, with p < 0.001). The influence of computer playfulness on BI2 was moderate (C = 0.41, p < 0.001) (quality of the model: R2 = 0.86, p < 0.001). Additional figures of mediation analysis are disponible in Supplementary material 2.
3.4.2. Important factors independently from the structure of the model: Random forest algorithm
We ran the RF algorithm in order to explain the values of our 3 target factors: BI, PU and PEOU. RF algorithms have the advantage of enabling analyzes with qualitative and quantitative variables at the same time so we used it on all our factors. Table 7 presents the important variables of BI2, PU2, and PEOU. The ordering of these variables enabled us to determine which of our factors explained the best the scores of these target factors. The three most important variables for each of them were:
• For BI2: PU2 followed by relevance and benefits/risk ratio. PU2 was in large predominance (value = 100) in comparison to the other (37.4 and 30.3, respectively). The quality of the prediction was high: 86.09% of the variance is explained.
• For PU2: Relevance, PEOU and benefits/risk ratio. Relevance was much more influential than the others (value = 100, vs. 33.5 and 33.4, respectively). The quality of the prediction was still quite high with 79.64% of the variance is explained.
• For PEOU: Ease of learning, computer playfulness and subjective norm. The values were less disparate: 100, 83.2, 80.9, respectively. But the prediction had a lower quality with 57.76% of the variance is explained.
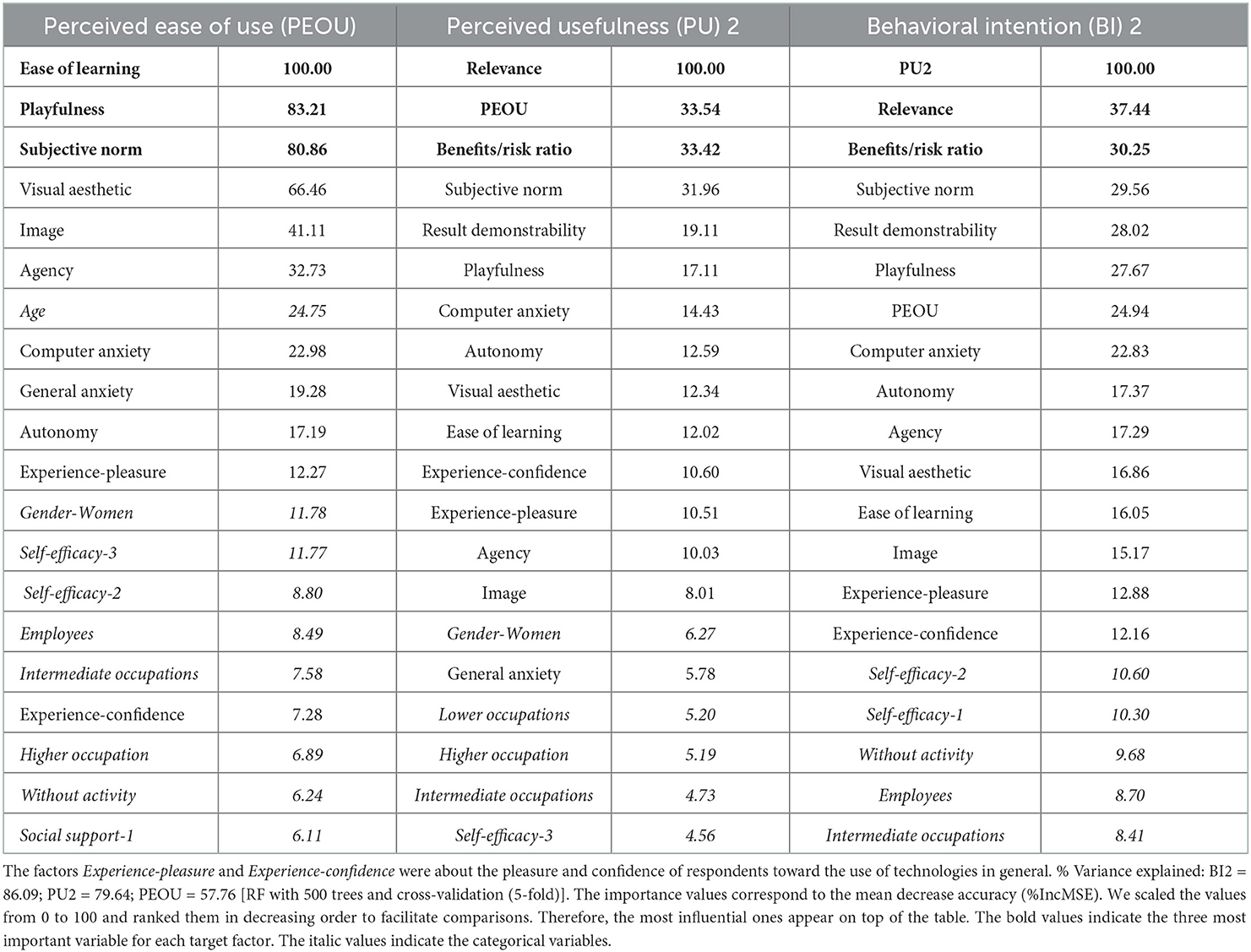
Table 7. The 20 most influential factors for each target factor (BI, PU, and PEOU) based on the RF algorithm.
Categorical factors appeared to have only moderate, if not low impact on BCI acceptability. The age was the only one in the top 10 of most influential factors, for PEOU only.
To provide a visual overview of our analyzes results, we propose a simplified version of our initial model in Figure 5, keeping only the most significant factors.
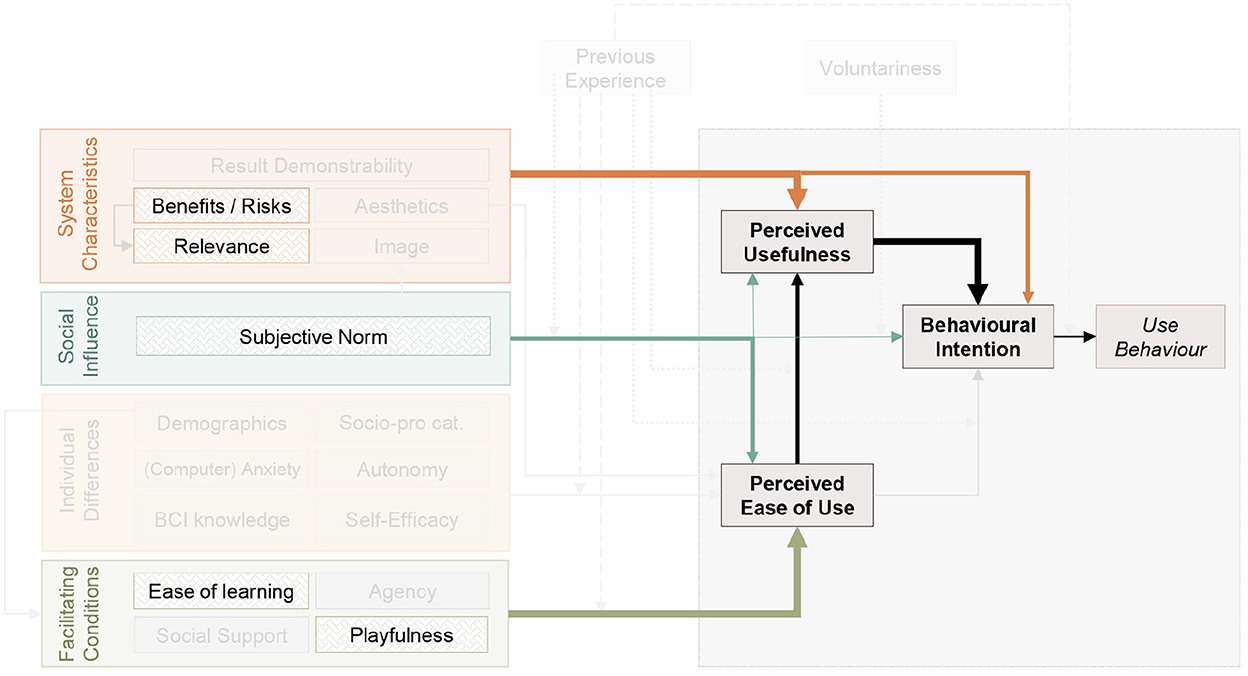
Figure 5. Representation of the factors of the tentative model of acceptability that influence the most the target factors. Boldest arrows link factors to the target factor they influence the most: ease of learning is the most influential factor for PEOU, relevance is the factor that has the highest impact on PU, itself being the most influential factor for BI. In addition, relevance also has a strong influence on BI. Benefits on risk ratio strongly influence both PU and BI. Subjective norm has a strong impact on PEOU (which was not expected based on the TAM3 and UTAUT2) and a medium impact on PU and BI. Finally, computer playfulness has a strong impact on PEOU.
3.4.3. Intensity of the connections between factors: Correlation analysis
We ran correlation analyzes between all our quantitative factors (with Bonferroni correction). For seeks of readability, we show in Table 8 only the factors that had been identified as the most influential ones based on RF analyzes. Results reveal that all the correlation coefficients were positive. The strongest correlations were between BI2 and PU2 (0.92-Bonferroni-corrected p < 0.001), PU2 and relevance (0.89-Bonferroni-corrected p < 0.001), BI2 and relevance (0.86-Bonferroni-corrected p < 0.001), but all the factors were significantly and strongly correlated with the target factors.
4. Discussion
This paper provides the following contributions. First, we designed a first-of-its-kind model of acceptability of BCIs for motor rehabilitation after stroke. This model is based on the literature, and notably on three validated models: TAM3 (Venkatesh and Bala, 2008), UTAUT2 (Venkatesh et al., 2012), and CUE (Thüring and Mahlke, 2007). Second, we created, based on this model, a questionnaire to assess acceptability. This questionnaire follows the structure of the model and includes 3 to 5 items to measure each of the factors. The quantitative items are represented as analog visual scales for which participants move a cursor from “do not agree at all” to “perfectly agree.” The position of the cursor is then translated into a score (from 0 to 10). The scores of the items measuring the same factor are averaged in order to obtain a robust estimation of this factor that is not (or at least as little as possible) dependent on the (mis)understanding of the item or on the state of the person when they answered the question. We distributed this questionnaire to a sample representative of the adult population in France (N = 753). This large and representative sample theoretically ensures the reliability of our results. Third, we performed analyzes on the data obtained to validate the structure of the model. More specifically, we assessed on the one hand the internal consistency of the factors using Cronbach's alpha analyzes. This enabled us to verify the relevance and complementarity of the items used to assess each factor.
On the other hand, we performed a confirmatory factor analysis to evaluate the internal consistency of the questionnaire, or in other words the relevance of the structure of the model. Finally, this is the fourth contribution, we quantified the impact that the different factors had on our target factors (PEOU, PU and BI) in order to identify the factors that influence the most BCI acceptability in the general public. To do so, we used two complementary methods: mediation analyzes and regressions based on random forest algorithms. The first one assessed this influence by taking into account the structure of the model while the second was independent from that structure. Our results show that BCIs are associated with high levels of acceptability for motor rehabilitation after stroke in the general public, and that the intention to use these technologies in that context is mainly driven by the perceived usefulness of the system, itself being mostly influenced by some characteristics of the system, and notably the benefits on risk ratio and scientific relevance. Facilitating conditions, and notably ease of learning and playfulness are the main determinants of the perceived ease of use. Finally, the subjective norm significantly influences the three target factors. With this methodology and results, our study is a first step toward an in-depth consideration of acceptability of BCIs for motor rehabilitation procedures after stroke.
For now, the model and questionnaire, while (we hope) insightful, are not really usable in practice due to their length and complexity. We voluntarily used an exploratory approach by including all the potential influential factors in our model, considering that the literature in the field did not enable us to have strong a priori. The extensive dataset collected enabled us to obtain first indications of the most influential, and therefore most relevant-to-assess factors. More data should now be collected to (un)validate those first results and refine the estimation of the impact that each factor has on BCI acceptability. Our objective is, ultimately, to design a shorter and more usable questionnaire that will enable the prediction of BCI acceptability based on a few factors (and so few items). This prediction could provide scientists/clinicians some indications on how to adapt the procedure, including the instructions, tasks, feedback and training environment, to favor BCI acceptability. As mentioned in the introduction, high acceptability levels could serve as levers to improve BCI efficiency. A main result of this study is that, globally, acceptability levels in terms of behavioral intention seem to be very high in the general public (with an average score of 8.23/10). This is consistent with other BCI acceptability studies (Al-Taleb et al., 2019; Voinea et al., 2019; Benaroch et al., 2021) who reported average scores of 8.0/10 (Al-Taleb et al., 2019) and 6.0/7 (Voinea et al., 2019) for perceived usefulness.
The analysis of Cronbach's alpha revealed that all the factors from the TAM3, UTAUT2 and CUE questionnaire were associated with high-quality internal consistency, i.e., scores were between 0.70 and 0.95 (Cortina, 1993; Tavakol and Dennick, 2011). It was not the case for some of the factors we added (in complement of those from TAM3, UTAUT2 and CUE) to fit with specificities of BCIs, namely, agency (0.50), autonomy (0.52), ease of learning (0.57) and benefits/risk ratio (0.62). This might be due to inadequate wording of the items. It should be noted though that the items used to assess autonomy were directly extracted, word-by-word, from the “Sociotropy-autonomy scale” (SAS, Husky et al., 2004), while those used to measure agency and ease of learning are reformulations (adapted to the context of BCIs) of items from the French adaptations of the Sense of Agency Scale (F-SoAS, Hurault et al., 2020) and of the System Usability Scale (SUS, Gronier and Baudet, 2021), respectively. In the future, it would be relevant to i) collect more data to assess the significance of this result, and ii) lead investigations regarding the comprehension of those items by potential BCI users, and maybe to reword them to increase the internal consistency to the associated factors.
Regarding the extreme score of internal consistency obtained for BI2 (0.97), we hypothesize that it might be due to the repetition of the items. Indeed, when participants saw the same items a second time, it might have happened that they automatically put some scores without really thinking about it, due to perceived redundancy. This hypothesis is supported by the fact that PU2 was also associated with very high, internal consistency scores (0.95) -while still in the “acceptable range.” This high score might also be due to a ceiling effect on those dimensions. Indeed, PU1 and BI1 were already rated with high scores (7.87+/1.63 and 7.88+/–1.73, respectively). After the second video, participants globally increased their rating and gave PU2 scores of 8.28+/1.57, and BI2 scores of 8.23+/–1.69. Thus, the range of values attributed to the items of PU2 and BI2 was narrow, resulting in low variability and thereby very high consistency within those dimensions.
To conclude on the validity of the questionnaire, while it is certainly not perfect yet -we hope that the community will help us improving it by collecting data and suggesting modifications- analyzes have globally revealed i) good internal consistency (as measured by Cronbach's alpha scores) for a large majority of the factors, and ii) a relevant structure of the model (as measured by the confirmatory factorial analysis).
If we have a closer look at the factors influencing the intention to use BCIs, thanks to the random forest-based regression analyzes, we notice that our different analyzes are consistent, notably in showing no significant impact of individual differences, including demographics (age, gender, socio-professional category) or cognitive/psychological profile (autonomy, anxiety, and self-efficacy). Yet, BCI studies have suggested an influence of those variables on BCI performance and learning (Burde and Blankertz, 2006; Nijboer et al., 2008, 2010; Witte et al., 2013; Jeunet et al., 2015). It might be possible that the weight of psychological variables such as anxiety or autonomy are stronger in persons with clinical conditions. It might also be the case that this influence is directly on efficiency as high levels of anxiety and low levels of autonomy and self-efficacy are detrimental for learning but does not alter acceptability. This reinforces the relevance of our approach consisting in optimizing acceptability in order to put the users/patients in the best conditions to favor learning despite their clinical condition, and thereby use acceptability as a lever to favor efficiency.
Behavioral intention is mainly influenced by the perceived usefulness of BCIs, itself being mainly determined by the perceived scientific relevance of the technology. This result highlights the importance of informing the population about BCIs, the way they function, and the level of scientific evidence regarding their clinical efficacy. This idea is strengthened by the significant increase of BI and PU scores following the second video in which the benefits of BCIs for motor rehabilitation after stroke are presented. In the same category of “system characteristics,” the benefits on risk ratio of the technology also seems to have a strong impact on acceptability. We hypothesize that this balance, as perceived by the user/patient, may have a moderator effect on the emphasis on scientific relevance that is objectively depicted by scientists and clinicians. Indeed, (irrational) fears or over-expectations may bias the balance making inaudible the scientific discourses.
Another main finding of this study is the influence that subjective norm has on the three target factors. This was expected for PU and BI. Nonetheless, if we refer to TAM3 and UTAUT2, this factor is not supposed to influence PEOU. In our results yet, this is on the latter that the subjective norm has the strongest impact. We hypothesize that the opinions of the patients' close ones, their technophilia and trust in science will, in the case of BCIs, not only play a role on the perceived usefulness, but will also contribute to emphasize or reduce apprehension toward the technology. This in turn may alter the perceived ease of use of the technology. In any case, the fact that social influence contributes in determining acceptability levels by acting on the three target factors reinforces the relevance of informing the general public, in which patients' relatives are included, to favor the acceptability and adoption of BCIs.
Finally, facilitating conditions, and especially ease of learning and playfulness, are the main determinants of PEOU that, while not influencing BI directly, significantly impacts PU. We believe that this result should encourage us to keep in mind that instructions should be clear and training motivating when we design BCI procedures. This will enable patients to feel confident in their ability to use a BCI. Providing an engaging environment can also be a way to make training more accessible. These results are consistent with the guidelines for successful MI-BCI training (Roc et al., 2021). The question of the transferability of this result to populations of patients could be raised. Indeed, the general population, while they do not need to use BCIs for rehabilitation, may perceive BCIs as a “toy,” which could explain this result. In fact, playfulness has also been shown to increase the compliance of patients in the rehabilitation process in other fields (Burke et al., 2009; Korn and Tietz, 2017; Lopes et al., 2018).
This question of differences between populations is definitely relevant. While we can assume some similarities and differences based on the literature, it will be necessary to lead the same approach with patients and clinicians in order to confront the results, deepen our knowledge and increase our ability to adapt BCIs accordingly. Once more, this will be a lever to improve BCI efficiency. Beyond the differences depending on the status of the respondents (patients, clinicians, and general public), there might also be differences related to their culture (Straub et al., 1997). Therefore, it also seems necessary to apply this approach on different populations around the world.
Collecting more data on diverse populations will enable us to refine our model. It is classic for acceptability models to evolve and to be adjusted to the time and context. The two versions of the UTAUT give us a perfect illustration of the necessity of adaptations. Indeed, whereas the first one was rather adapted to technologies for organizations (Venkatesh et al., 2003), the second one gravitates toward individual consumers/users (Venkatesh et al., 2012). For appropriate adaptations to be made, an open science approach will be necessary. Indeed we think that it will be possible only if people collect data, share their findings and work together on improving the soundness and reliability of the model.
4.1. Recommendations
These results offer first trails to make BCI-based stroke rehabilitation procedures more acceptable. On the one hand, we have seen that the video which explains the use of BCIs in post-stroke rehabilitation had an influence on BI and PU scores and on the predictors of these scores. Thus, informing (future) users is a key step: it is necessary to be as clear as possible on the objectives of using a BCI, on its functioning, on the expected results, but also on the constraints related to the use (learning time, cognitive cost, etc.). These recommendations are important to consider to improve the perception of the benefits/risk ratio and relevance factors. We think that one of the most interesting formats of information can be the production of educational videos that help demystify BCIs, as we did in the questionnaire. This is in line with what could be done to take social influence into account. Indeed, one of the best ways to play on subjective norm, and use the influence of this factor to improve acceptability, is to lead pedagogical actions on the general population. If people surrounding post-stroke subjects have an enlightened point of view on BCI, this could positively influence the acceptability of the therapy of this population. We want to underline that, to our viewpoint, these recommendations can be replicated in others BCIs settings, not only in post-stroke rehabilitation context.
Our most important recommendation, in any context of use of BCIs, remains to ensure that acceptability is assessed in order to adapt the protocol accordingly. It is an easy way to improve patients' well-being during rehabilitation phases and thereby, most certainly, to increase their engagement and thereby leverage the efficiency of BCI-based rehabilitation procedures.
Conclusion
This paper is dedicated to the general public acceptability of BCI-based post-stroke rehabilitation procedures. We are conscious that collecting the opinions of post-stroke subjects and caregivers is also essential. We are currently working on this, conducting questionnaires and semi-structured interviews with post-stroke subjects and caregivers. This will allow us to investigate whether the acceptability factors that stand out the most are similar to those of the general public, and if not, to try to understand what could be the cause of these differences and how to move toward more personalized acceptability models, adapted to the targeted users.
Data availability statement
The raw data supporting the conclusions of this article will be made available by the authors upon request, without undue reservation.
Ethics statement
The studies involving human participants were reviewed and approved by Institutional Review Board of Toulouse Federal University (N°2019-140). The patients/participants provided their written informed consent to participate in this study.
Author contributions
CJ-K, EG, KF, ST, FA, DG, JP, and LB conceived and designed the experiments. EG, KF, ST, and CJ-K performed the survey and analyzed the data. EG wrote the first manuscript. All authors contributed to the article and approved the submitted version.
Funding
This research was funded by the French National Research Agency (project ABCIS, grant ANR-20-CE38-0008-01).
Conflict of interest
The authors declare that the research was conducted in the absence of any commercial or financial relationships that could be construed as a potential conflict of interest.
Publisher's note
All claims expressed in this article are solely those of the authors and do not necessarily represent those of their affiliated organizations, or those of the publisher, the editors and the reviewers. Any product that may be evaluated in this article, or claim that may be made by its manufacturer, is not guaranteed or endorsed by the publisher.
Supplementary material
The Supplementary Material for this article can be found online at: https://www.frontiersin.org/articles/10.3389/fnrgo.2022.1082901/full#supplementary-material
References
Accident Vasculaire cérébral (AVC). Inserm La science pour la santé. (2019). Inserm. Available online at: https://www.inserm.fr/dossier/accident-vasculaire-cerebral-avc/ (accessed June 21, 2022).
Alba, J. W., and Hutchinson, J. W. (1987). Dimensions of consumer expertise. J. Consum. Res. 13, 411–454. doi: 10.1086/209080
Alexandre, B., Reynaud, E., Osiurak, F., and Navarro, J. (2018). Acceptance and acceptability criteria: a literature review. Cognit. Technol. Work 20, 165–177. doi: 10.1007/s10111-018-0459-1
Al-Taleb, M., Purcell, M., Fraser, M., Petric-Gray, N., and Vuckovic, A. (2019). Home used, patient self-managed, brain-computer interface for the management of central neuropathic pain post spinal cord injury: usability study. J. Neuroeng. Rehabil. 16, 1–24. doi: 10.1186/s12984-019-0588-7
Alturas, B. (2021). “Models of acceptance and use of technology research trends: literature review and exploratory bibliometric study,” in Recent Advances in Technology Acceptance Models and Theories (Cham: Springer), 13–28.
Bai, Z., Fong, K. N., Zhang, J. J., Chan, J., and Ting, K. (2020). Immediate and long-term effects of BCI-based rehabilitation of the upper extremity after stroke: a systematic review and meta-analysis. J. Neuroeng. Rehabil. 17, 1–20. doi: 10.1186/s12984-020-00686-2
Barcenilla, J., and Bastien, J. M. C. (2009). L'acceptabilité des nouvelles technologies: quelles relations avec l'ergonomie, l'utilisabilité et l'expérience utilisateur? Trav. Hum. 72, 311–331. doi: 10.3917/th.724.0311
Benaroch, C., Sadatnejad, K., Roc, A., Appriou, A., Monseigne, T., Pramij, S., et al. (2021). Long-term bci training of a tetraplegic user: adaptive riemannian classifiers and user training. Front. Hum. Neurosci. 15, 118. doi: 10.3389/fnhum.2021.635653
Blain-Moraes, S., Schaff, R., Gruis, K. L., Huggins, J. E., and Wren, P. A. (2012). Barriers to and mediators of brain-computer interface user acceptance: focus group findings. Ergonomics 55, 516–525. doi: 10.1080/00140139.2012.661082
Bland, J., and Altman, D. (1997). Statistics notes: marketing. BMJ 314, 572. doi: 10.1136/bmj.314.7080.572
Bocquelet, F., Piret, G., Aumonier, N., and Yvert, B. (2016). Ethical reflections on brain-computer interfaces. Brain Comput. Interfaces. 2, 259–288. doi: 10.1002/9781119332428.ch15
Brooke, J. (1986). System Usability Scale (sus): A Quick-And-Dirty Method of System Evaluation User Information. Reading: Digital Equipment Co., Ltd.
Burde, W., and Blankertz, B. (2006). “Is the locus of control of reinforcement a predictor of brain-computer interface performance?” in Proceedings of the 3rd International Brain-Computer Interface Workshop and Training Course. Vol. 2006. p. 108–109. Available online at: https://doc.ml.tu-berlin.de/publications/publications/BurBla06.pdf
Burke, J. W., McNeill, M., Charles, D. K., Morrow, P. J., Crosbie, J. H., and McDonough, S. M. (2009). Optimising engagement for stroke rehabilitation using serious games. Vis. Comput. 25, 1085–1099. doi: 10.1007/s00371-009-0387-4
Burton, C. A. C., Murray, J., Holmes, J., Astin, F., Greenwood, D., and Knapp, P. (2013). Frequency of anxiety after stroke: a systematic review and meta-analysis of observational studies. Int. J. Stroke 8, 545–559. doi: 10.1111/j.1747-4949.2012.00906.x
Cattell, R. B., and andCattell, H. E. P. (1995). Personality structure and the new fifth edition of the 16pf. Educ. Psychol. Meas. 55, 926–937. doi: 10.1177/0013164495055006002
Cervera, M. A., Soekadar, S. R., Ushiba, J., Millán, J.d. R, Liu, M., et al. (2018). Brain-computer interfaces for post-stroke motor rehabilitation: a meta-analysis. Ann. Clin. Transl. Neurol. 5, 651–663. doi: 10.1002/acn3.544
Compeau, D. R., and Higgins, C. A. (1995). Application of social cognitive theory to training for computer skills. Inf. Syst. Res. 6, 118–143. doi: 10.1287/isre.6.2.118
Cortina, J. M. (1993). What is coefficient alpha? an examination of theory and applications. J. Appl. Psychol. 78, 98. doi: 10.1037/0021-9010.78.1.98
Davis, F. D. (1989). Perceived usefulness, perceived ease of use, and user acceptance of information technology. MIS Q. 13, 319–340. doi: 10.2307/249008
Davis, F. D., Bagozzi, R. P., and Warshaw, P. R. (1992). Extrinsic and intrinsic motivation to use computers in the workplace 1. J. Appl. Soc. Psychol. 22, 1111–1132. doi: 10.1111/j.1559-1816.1992.tb00945.x
DeVellis, R. F., and Thorpe, C. T. (2021). Scale Development: Theory and Applications. Thousand Oaks, CA: Sage Publications.
Dillon, A. (2001). User acceptance of information technology. Encyclopedia Hum. Factors Ergon. 1, 1105–1109. doi: 10.2307/30036540
Dussard, C., Pillette, L., Jeunet, C., and George, N. (2022). “Can feedback transparency improve motor-imagery BCI performance?” in Cortico 2022 (Grenoble).
Edwards, I., Wiholm, B.-E., and Martinez, C. (1996). Concepts in risk-benefit assessment. A simple merit analysis of a medicine? Drug Safety 15, 1–7. doi: 10.2165/00002018-199615010-00001
Février, F. (2011). Vers un modèle intégrateur" expérience-acceptation": rôle des affects et de caractéristiques personnelles et contextuelles dans la détermination des intentions d'usage d'un environnement numérique de travail (Ph.D. thesis). Université Rennes 2; Université Européenne de Bretagne.
Fishbein, M., and Ajzen, I. (1977). Belief, Attitude, Intention, and Behavior: An Introduction to Theory and Research. Reading, MA: Addison-Wesley.
Gallagher, M. W., and Brown, T. A. (2013). “Introduction to confirmatory factor analysis and structural equation modeling,” in Handbook of Quantitative Methods for Educational Research (Leiden: Brill), 287–314.
Gallagher, S. (2000). Philosophical conceptions of the self: implications for cognitive science. Trends Cogn. Sci. 4, 14–21. doi: 10.1016/S1364-6613(99)01417-5
Geronimo, A., Stephens, H. E., Schiff, S. J., and Simmons, Z. (2015). Acceptance of brain-computer interfaces in amyotrophic lateral sclerosis. Amyotrophic Lateral Sclerosis Frontotemporal Degenerat. 16, 258–264. doi: 10.3109/21678421.2014.969275
Ghiglione, R., and Matalon, B. (1978). Les enquêtes sociologiques: théories et pratique. Paris: A. Colin.
Gronier, G., and Baudet, A. (2021). Psychometric evaluation of the f-sus: creation and validation of the french version of the system usability scale. Int. J. Hum. Comput. Interact. 37, 1571–1582. doi: 10.1080/10447318.2021.1898828
Gürkök, H., Hakvoort, G., and Poel, M. (2011). “Evaluating user experience in a selection based brain-computer interface game a comparative study,” in International Conference on Entertainment Computing (Berlin; Heidelberg: Springer), 77–88.
Hassenzahl, M. (2001). The effect of perceived hedonic quality on product appealingness. Int. J. Hum. Comput. Interact. 13, 481–499. doi: 10.1207/S15327590IJHC1304_07
Hassenzahl, M. (2003). “The thing and i: understanding the relationship between user and product,” in Funology (Dordrecht: Springer), 31–42.
Hassenzahl, M. (2004). The interplay of beauty, goodness, and usability in interactive products. Hum. Comput. Interact. 19, 319–349. doi: 10.1207/s15327051hci1904_2
Huggins, J. E., Moinuddin, A. A., Chiodo, A. E., and Wren, P. A. (2015). What would brain-computer interface users want: opinions and priorities of potential users with spinal cord injury. Arch. Phys. Med. Rehabil. 96, S38-S45. doi: 10.1016/j.apmr.2014.05.028
Huggins, J. E., Wren, P. A., and Gruis, K. L. (2011). What would brain-computer interface users want? Opinions and priorities of potential users with amyotrophic lateral sclerosis. Amyotrophic Lateral Sclerosis 12, 318–324. doi: 10.3109/17482968.2011.572978
Hurault, J.-C., Broc, G., Crône, L., Tedesco, A., and Brunel, L. (2020). Measuring the sense of agency: a french adaptation and validation of the sense of agency scale (f-soas). Front. Psychol. 11, 2650. doi: 10.3389/fpsyg.2020.584145
Husky, M. M., Grondin, O. S., and Compagnone, P. D. (2004). Validation de la version française du questionnaire de sociotropie-autonomie de beck et collègues. Can. J. Psychiatry 49, 851–858. doi: 10.1177/070674370404901209
Jeunet, C., Glize, B., McGonigal, A., Batail, J.-M., and Micoulaud-Franchi, J.-A. (2019). Using eeg-based brain computer interface and neurofeedback targeting sensorimotor rhythms to improve motor skills: theoretical background, applications and prospects. Neurophysiol. Clin. 49, 125–136. doi: 10.1016/j.neucli.2018.10.068
Jeunet, C., N'Kaoua, B., and Lotte, F. (2016). Advances in user-training for mental-imagery-based BCI control: psychological and cognitive factors and their neural correlates. Prog. Brain Res. 228, 3–35. doi: 10.1016/bs.pbr.2016.04.002
Jeunet, C., N'Kaoua, B., Subramanian, S., Hachet, M., and Lotte, F. (2015). Predicting mental imagery-based bci performance from personality, cognitive profile and neurophysiological patterns. PLoS ONE 10, e0143962. doi: 10.1371/journal.pone.0143962
Kaleshtari, M. H., Ciobanu, I., Seiciu, P. L., Marin, A. G., and Berteanu, M. (2016). Towards a model of rehabilitation technology acceptance and usability. Int. J. Soc. Sci. Hum. 6, 612. doi: 10.7763/IJSSH.2016.V6.720
Kelman, H. C. (1958). Compliance, identification, and internalization three processes of attitude change. J. Conflict Resolut. 2, 51–60. doi: 10.1177/002200275800200106
Kerous, B., Skola, F., and Liarokapis, F. (2018). EEG-based bci and video games: a progress report. Virtual Real. 22, 119–135. doi: 10.1007/s10055-017-0328-x
Kline, P. (2015). A Handbook of Test Construction (Psychology Revivals): Introduction to Psychometric Design. London: Routledge.
Korn, O., and Tietz, S. (2017). “Strategies for playful design when gamifying rehabilitation: a study on user experience,” in Proceedings of the 10th International Conference on Pervasive Technologies Related to Assistive Environments (New York, NY: Association for Computing Machinery), 209–214.
Koul, S., and Eydgahi, A. (2017). A systematic review of technology adoption frameworks and their applications. J. Technol. Manag. Innovat. 12, 106–113. doi: 10.4067/S0718-27242017000400011
Kowalski, C. J. (1972). On the effects of non-normality on the distribution of the sample product-moment correlation coefficient. J. R. Stat. Soc. C 21, 1–12. doi: 10.2307/2346598
Kübler, A., Holz, E. M., Riccio, A., Zickler, C., Kaufmann, T., Kleih, S. C., et al. (2014). The user-centered design as novel perspective for evaluating the usability of bci-controlled applications. PLoS ONE 9, e112392. doi: 10.1371/journal.pone.0112392
Lee, T.-S., Goh, S. J. A., Quek, S. Y., Phillips, R., Guan, C., Cheung, Y. B., et al. (2013). A brain-computer interface based cognitive training system for healthy elderly: a randomized control pilot study for usability and preliminary efficacy. PLoS ONE 8, e79419. doi: 10.1371/journal.pone.0079419
Leeb, R., Perdikis, S., Tonin, L., Biasiucci, A., Tavella, M., Creatura, M., et al. (2013). Transferring brain-computer interfaces beyond the laboratory: successful application control for motor-disabled users. Artif. Intell. Med. 59, 121–132. doi: 10.1016/j.artmed.2013.08.004
Li, Y., Pan, J., Wang, F., and Yu, Z. (2013). A hybrid bci system combining p300 and ssvep and its application to wheelchair control. IEEE Trans. Biomed. Eng. 60, 3156–3166. doi: 10.1109/TBME.2013.2270283
Lopes, S., Magalhaes, P., Pereira, A., Martins, J., Magalhaes, C., Chaleta, E., et al. (2018). Games used with serious purposes: a systematic review of interventions in patients with cerebral palsy. Front. Psychol. 9, 1712. doi: 10.3389/fpsyg.2018.01712
Lotte, F., Bougrain, L., Cichocki, A., Clerc, M., Congedo, M., Rakotomamonjy, A., et al. (2018). A review of classification algorithms for eeg-based brain-computer interfaces: a 10 year update. J. Neural Eng. 15, 031005. doi: 10.1088/1741-2552/aab2f2
Mahlke, S. (2008). User Experience of Interaction With Technical Systems (Ph.D. thesis). Technische Universität Berlin.
Martocchio, J. J., and Webster, J. (1992). Effects of feedback and cognitive playfulness on performance in microcomputer software training. Pers. Psychol. 45, 553–578. doi: 10.1111/j.1744-6570.1992.tb00860.x
Moore, G., and Benbasat, I. (1991). Development of an instrument to measure the perceptions of adopting an information technology innovation. Inf. Syst. Res. 2, 5167. doi: 10.1287/isre.2.3.192
Morone, G., Pisotta, I., Pichiorri, F., Kleih, S., Paolucci, S., Molinari, M., et al. (2015). Proof of principle of a brain-computer interface approach to support poststroke arm rehabilitation in hospitalized patients: design, acceptability, and usability. Arch. Phys. Med. Rehabil. 96, S71-S78. doi: 10.1016/j.apmr.2014.05.026
Nijboer, F. (2015). Technology transfer of brain-computer interfaces as assistive technology: barriers and opportunities. Ann. Phys. Rehabil. Med. 58, 35–38. doi: 10.1016/j.rehab.2014.11.001
Nijboer, F., Birbaumer, N., and Kübler, A. (2010). The influence of psychological state and motivation on brain-computer interface performance in patients with amyotrophic lateral sclerosis-a longitudinal study. Front. Neurosci. 171, 55. doi: 10.3389/fnins.2010.00055
Nijboer, F., Furdea, A., Gunst, I., Mellinger, J., McFarland, D., Birbaumer, N., et al. (2008). An auditory brain-computer interface (BCI). J. Neurosci. Methods. 244, 43–50. doi: 10.1016/j.jneumeth.2007.02.009
Nojima, I., Sugata, H., Takeuchi, H., and Mima, T. (2022). Brain-computer interface training based on brain activity can induce motor recovery in patients with stroke: a meta-analysis. Neurorehabil. Neural Repair. 36, 83–96. doi: 10.1177/15459683211062895
Pasqualotto, E., Simonetta, A., Gnisci, V., Federici, S., and Belardinelli, M. O. (2011). Toward a usability evaluation of bcis. Int. J. Bioelectromagn 13, 121–122. Available online at: http://hdl.handle.net/2078.1/138512
Pfurtscheller, G., Guger, C., Müller, G., Krausz, G., and Neuper, C. (2000). Brain oscillations control hand orthosis in a tetraplegic. Neurosci. Lett. 292, 211–214. doi: 10.1016/S0304-3940(00)01471-3
Pichiorri, F., Morone, G., Petti, M., Toppi, J., Pisotta, I., Molinari, M., et al. (2015). Brain-computer interface boosts motor imagery practice during stroke recovery. Ann. Neurol. 77, 851–865. doi: 10.1002/ana.24390
Pillette, L., Grevet, E., Amadieu, F., Dussard, C., Delgado-Zabalza, L., Dumas, C., et al. (2022). The acceptability of BCIs and neurofeedback: presenting a systematic review, a field-specific model and an online tool to facilitate assessment.
Pillette, L., Jeunet, C., Mansencal, B., N'kambou, R., N'Kaoua, B., and Lotte, F. (2020). A physical learning companion for mental-imagery bci user training. Int. J. Hum. Comput. Stud. 136, 102380. doi: 10.1016/j.ijhcs.2019.102380
Rad, M. S., Nilashi, M., and Dahlan, H. M. (2018). Information technology adoption: a review of the literature and classification. Universal Access Inf. Soc. 17, 361–390. doi: 10.1007/s10209-017-0534-z
Randolph, A. B. (2012). “Not all created equal: individual-technology fit of brain-computer interfaces,” in 2012 45th Hawaii International Conference on System Sciences (Maui, HI: IEEE), 572–578.
Revelle, W. (2021). How to Use the Psych Package for Mediation/Moderation/Regression Analysis. The Personality Project. Available online at: http://personality-project.org/r/psych/HowTo/mediation.pdf
Roc, A., Pillette, L., Mladenovic, J., Benaroch, C., N'Kaoua, B., Jeunet, C., et al. (2021). A review of user training methods in brain computer interfaces based on mental tasks. J. Neural Eng. 18, 011002. doi: 10.1088/1741-2552/abca17
Ron-Angevin, R., and Díaz-Estrella, A. (2009). Brain-computer interface: changes in performance using virtual reality techniques. Neurosci. Lett. 449, 123–127. doi: 10.1016/j.neulet.2008.10.099
Rondan-Cataluña, F. J., Arenas-Gaitán, J., and Ramírez-Correa, P. E. (2015). A comparison of the different versions of popular technology acceptance models: a non-linear perspective. Kybernetes 44, 788–805. doi: 10.1108/K-09-2014-0184
Schaupp, L. C., Carter, L., and McBride, M. E. (2010). E-file adoption: a study of us taxpayers' intentions. Comput. Human Behav. 26, 636–644. doi: 10.1016/j.chb.2009.12.017
Sharma, N., Pomeroy, V. M., and Baron, J.-C. (2006). Motor imagery: a backdoor to the motor system after stroke? Stroke 37, 1941–1952. doi: 10.1161/01.STR.0000226902.43357.fc
Straub, D., Keil, M., and Brenner, W. (1997). Testing the technology acceptance model across cultures: a three country study. Inf. Manag. 33, 1–11. doi: 10.1016/S0378-7206(97)00026-8
Tavakol, M., and Dennick, R. (2011). Making sense of cronbach's alpha. Int. J. Med. Educ. 2, 53. doi: 10.5116/ijme.4dfb.8dfd
Terrade, F., Pasquier, H., Juliette, R., Guingouain, G., and Somat, A. (2009). L'acceptabilité sociale: la prise en compte des déterminants sociaux dans l'analyse de l'acceptabilité des systèmes technologiques. Trav. Hum. 72, 383–395. doi: 10.3917/th.724.0383
Thüring, M., and Mahlke, S. (2007). Usability, aesthetics and emotions in human-technology interaction. Int. J. Psychol. 42, 253–264. doi: 10.1080/00207590701396674
Venkatesh, V. (2000). Determinants of perceived ease of use: Integrating control, intrinsic motivation, and emotion into the technology acceptance model. Inf. Syst. Res. 11, 1–432 doi: 10.1287/isre.11.4.342.11872
Venkatesh, V., and Bala, H. (2008). Technology acceptance model 3 and a research agenda on interventions. Decis. Sci. 39, 273–315. doi: 10.1111/j.1540-5915.2008.00192.x
Venkatesh, V., and Davis, F. D. (2000). A theoretical extension of the technology acceptance model: four longitudinal field studies. Manag. Sci. 46, 186–204. doi: 10.1287/mnsc.46.2.186.11926
Venkatesh, V., Morris, M. G., Davis, G. B., and Davis, F. D. (2003). User acceptance of information technology: toward a unified view. MIS Q. 27, 425–478.
Venkatesh, V., Thong, J. Y., and Xu, X. (2012). Consumer acceptance and use of information technology: extending the unified theory of acceptance and use of technology. MIS Q. 36, 157–178. doi: 10.2307/41410412
Vilatte, J.-C. (2007). Méthodologie de l'enquête par Questionnaire. Laboratory Culture and Communication, University of Avignon. Formation in Grisolles (France).
Voinea, G.-D., Boboc, R., Gîrbacia, F., and Postelnicu, C.-C. (2019). “Technology acceptance of a hybrid brain-computer interface for instruction manual browsing,” in Proceedings of the 14th International Conference on Virtual Learning (ICVL) (Bucharest).
Wang, Y.-M., Wei, C.-L., and Wang, M.-W. (2022). Factors influencing students' adoption intention of brain-computer interfaces in a game-learning context. Library Hi Tech. doi: 10.1108/LHT-12-2021-0506. [Epub ahead of print].
Wang, Y.-S., Wu, M.-C., and Wang, H.-Y. (2009). Investigating the determinants and age and gender differences in the acceptance of mobile learning. Br. J. Educ. Technol. 40, 92–118. doi: 10.1111/j.1467-8535.2007.00809.x
Wills, M. J., El-Gayar, O. F., and Bennett, D. (2008). Examining healthcare professionals' acceptance of electronic medical records using utaut. Issue. Infm. Syst. 9, 396–401. Available online at: https://iacis.org/iis/2008/S2008_1053.pdf
Witte, M., Kober, S., Ninaus, M., Neuper, C., and Wood, G. (2013). Control beliefs can predict the ability to up-regulate sensorimotor rhythm during neurofeedback training. Front. Hum. Neurosci. 7, 478. doi: 10.3389/fnhum.2013.00478
Wolbring, G., Diep, L., Yumakulov, S., Ball, N., and Yergens, D. (2013). Social robots, brain machine interfaces and neuro/cognitive enhancers: three emerging science and technology products through the lens of technology acceptance theories, models and frameworks. Technologies 1, 3–25. doi: 10.3390/technologies1010003
Keywords: brain-computer interface (BCI), neurofeedback (NF), acceptability, acceptance, stroke, motor rehabilitation, model, questionnaire
Citation: Grevet E, Forge K, Tadiello S, Izac M, Amadieu F, Brunel L, Pillette L, Py J, Gasq D and Jeunet-Kelway C (2023) Modeling the acceptability of BCIs for motor rehabilitation after stroke: A large scale study on the general public. Front. Neuroergon. 3:1082901. doi: 10.3389/fnrgo.2022.1082901
Received: 28 October 2022; Accepted: 09 December 2022;
Published: 01 February 2023.
Edited by:
Athanasios Vourvopoulos, Instituto Superior Técnico (ISR), PortugalReviewed by:
Mathis Fleury, Universidade de Lisboa, PortugalFloriana Pichiorri, Santa Lucia Foundation (IRCCS), Italy
Copyright © 2023 Grevet, Forge, Tadiello, Izac, Amadieu, Brunel, Pillette, Py, Gasq and Jeunet-Kelway. This is an open-access article distributed under the terms of the Creative Commons Attribution License (CC BY). The use, distribution or reproduction in other forums is permitted, provided the original author(s) and the copyright owner(s) are credited and that the original publication in this journal is cited, in accordance with accepted academic practice. No use, distribution or reproduction is permitted which does not comply with these terms.
*Correspondence: Elise Grevet, elise.grevet@u-bordeaux.fr