- 1Biological Psychology and Neuroergonomics, Technische Universität Berlin, Berlin, Germany
- 2Northrop Grumman Corporation, McLean, VA, United States
- 3Department of Psychology, Wichita State University, Wichita, KS, United States
- 4ISAE-SUPAERO, Université de Toulouse, Toulouse, France
- 5Aquitaine Institute for Cognitive and Integrative Neuroscience, CNRS and University of Bordeaux, Bordeaux, France
- 6Department of Industrial and Systems Engineering, Texas A&M University, College Station, TX, United States
- 7Institute of Neuroscience, National Research Council of Italy, Parma, Italy
Introduction
Neuroergonomics as defined by Raja Parasuraman is the study of “the brain at work and in everyday life” (Parasuraman, 2003). This rapidly growing research field aims at understanding human brain function underlying the many facets of human interaction with technical systems (Dehais et al., 2020). The term “cognition” is used to describe different processes (e.g., attention, memory, decision making) relevant to human-technology interaction. Cognitive neuroergonomics, then, can be defined as a section of neuroergonomics concerned with the investigation of the neural bases of those cognitive processes involved in the user's interaction with a technical system at work or during everyday life. One of the defining aspects of cognitive neuroergonomics is that it uses insights from analyzing neural dynamics in these settings to inform cognitive theory and models, as well as to improve our understanding of human brain function underlying cognition, in general.
To this end, new imaging methods are continuously adapted and used in a wide range of experimental scenarios that cover the entire area of ergonomics from highly controlled laboratory research protocols, to less controlled translational research, to research in the real world with little control over the factors of interest (Parada, 2018). This decreasing level of control is accompanied by an increasing level of ecological validity. Laboratory experiments provide very good control over experimental factors with high internal validity of the investigated constructs but often suffer from low levels of ecological validity. In contrast, real-world experiments might show low internal validity and lack of experimental control but provide high ecological validity that cannot be further improved. Here, the real world is the laboratory (Gramann et al., 2017). Furthermore, with increasing ecological validity, inter-acting with technical systems often involves expanding physical activity of the user. System interactions range from very low input (e.g., interaction with mobile devices; McKendrick, 2019) to larger scale interaction (e.g., Human-Robot Interaction, HRI; Tsarouchi et al., 2016) to very large scale interactions (e.g., assisted navigation; Wunderlich and Gramann, 2020). Active behavior is the basis for physically demanding workplaces as well as less physically challenging tasks that, nonetheless, require body, head and eye movements when users actively seek information or respond to external stimuli (Doshi and Trivedi, 2009). Traditionally, however, active behavior is not allowed in brain imaging protocols because established imaging modalities are usually too heavy to follow participants' movements and movement-related artifacts render the analyses of neural activity difficult (Makeig et al., 2009; Gramann et al., 2011).
With cognitive neuroergonomics maturing into a new research area with widespread research questions and methods, the focus should be put back into theory-driven studies of the human brain at work and in everyday life. Good scientific practices have to be adapted to allow for replicable science including the integration of new mobile imaging methods into the existing range of established imaging protocols. New findings have to be related to parameters known from established laboratory protocols and integrated into larger theoretical frameworks that allow for systematic replication as well as the development of robust parameters reflecting cognitive processes. From this perspective, it is our belief that the following challenges will have to be met to further develop this scientific field.
Challenge 1: Bridging Basic, Translational, and Applied Research in Cognitive Neuroergonomics
Traditionally, research in cognitive neuroergonomics takes place in different environments spanning the entire space of protocols from fundamental to applied research (Figure 1). Fundamental research questions can be addressed in controlled laboratory settings and translated toward work environments (e.g., Gateau et al., 2018; Zhu et al., 2020a). In translational research, often expensive high-fidelity simulations are used to investigate more realistic cognitive and behavioral dynamics (Baldwin et al., 2017). These environments enable sufficient control over confounding factors while permitting repetitions of simulation scenarios to gain sufficient statistical power for analyzing a specific cognitive process of interest (Hollnagel, 2010). Finally, applied research takes place during interaction with technology in real-world settings which often do not allow for controlling how users interact with the system, providing limited or no control over external factors contributing to the behavior of users (Dehais et al., 2019).
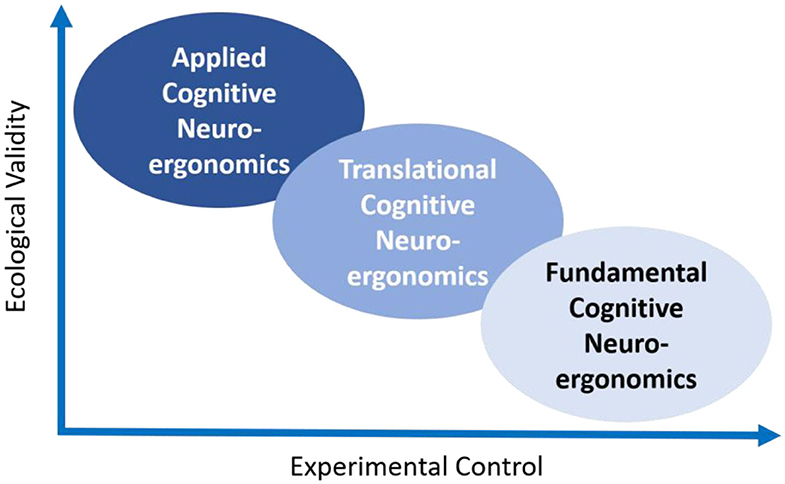
Figure 1. Experimental protocols in cognitive neuroergonomics regarding control and ecological validity.
Over the last decades, the majority of neuroergonomic studies took place in laboratories that provided the necessary infrastructure and housing for the heavy and susceptible imaging technologies. With the rise of lightweight mobile amplifiers, however, an increasing number of studies moved out of the lab using imaging methods in real-world settings (McKendrick et al., 2017; Chavarriaga et al., 2018; Protzak and Gramann, 2018).
All three areas of research in neuroergonomics are important and eventually converge to understand the cognitive and neural basis of human-technology interaction. To assure objectivity, reliability, and validity of the methods used, neuroergonomic studies have to allow for replication and falsifiability as two necessary pillars of a healthy scientific field that help to facilitate replication of key findings in the future.
Besides implementing good scientific practices, bridging fundamental, and applied studies in cognitive neuroergonomics can be achieved through the use of virtual reality (VR). VR allows for creating fully controlled and accurate virtual copies of real scenarios which are too expensive, dangerous or impossible to create in a standard experimental laboratory (Jeunet et al., 2018; e.g., Shi et al., 2020). VR can be useful to the design of technological interfaces leading to a reduction of research costs when compared to real scenarios (Chryssolouris et al., 2000). VR systems currently come with critical limitations including lower levels of fidelity when compared to real world scenarios (Hu et al., 2011), the lack of proper haptic feedback (Faure et al., 2020), and simulation sickness for a non-negligible percentage of the population (Duzmańska et al., 2018). But, VR technologies are rapidly improving and provide possible solutions to minimize many problems associated with low control, expensive access, and insufficient sample sizes in translational and applied neuroergonomics research in the next decades.
Challenge 2: Imaging Methods for Embodied Cognitive Neuroergonomics
The majority of workplaces and interfaces require active behavior for successful interaction with a system. The programming and execution of motor action influences cognition as well as accompanying brain dynamics and it is thus imperative to understand cognitive processes during inter-action with a system (Gramann et al., 2011; Jungnickel and Gramann, 2016).
Mobile brain imaging approaches from mobile EEG (Gramann and Plank, 2019) and fNRIS (Izzetoglu et al., 2005) to Mobile Brain/Body Imaging (Jungnickel et al., 2019) allow for imaging human brain dynamics in actively behaving participants interacting with a technical system. Lightweight and mobile amplifiers exist for EEG and fNIRS that can be further combined and synchronized with additional physiological measures, motion capture, and other methods. Because of the active behavior of participants in mobile recordings, the acquired data is often contaminated with activity stemming from non-brain sources, usually considered artifact. Most mobile brain imaging studies thus require careful pre-processing of the recorded data (for EEG, see Klug and Gramann, 2020; for fNRIS, see Zhu et al., 2020b) and data-driven analyses to dissociate brain from non-brain activity (Makeig et al., 2009; Vitorio et al., 2017 for EEG and fNRIS, respectively). The increasing demands in preparation, technical challenges, and data analyses come with deeper insights into human brain dynamics only if the challenges of new mobile brain imaging methods can be overcome.
Even though improved mobile brain imaging methods allow for investigating naturalistic interactions with technical systems in the workplace and everyday settings (Gehrke et al., 2019; Wascher et al., 2020), these new methods likely come with potential changes in the extracted neural parameters (Gramann et al., 2018). Such differences have to be described and embedded in a systematic fashion to allow for understanding the theoretical and methodological framework and to foster convergence with results from standard laboratory protocols, leading directly to the third grand field challenge.
Challenge 3: Generalizability of Physiological Parameters Reflecting Cognitive Processes
A variety of neurophysiological parameters have been identified in different experiments with diverging interpretations regarding their cognitive correlates. The advent of new imaging modalities and analysis approaches that allow for brain imaging in actively behaving participants, led to the discovery of new neural dynamics. It is important to embed new and diverging results in a theoretical framework rather than just accumulating new parameters that are disconnected from existing knowledge and established theory.
It is an eminent challenge in cognitive neuroergonomics to systematically compare physiological parameters across experimental tasks and neuroscientific methods to allow for identifying parameters that represent cognitive processes or user states independently of the tasks and methods used. In general, the eventual outcome of neurergonomic research, like any other research, should be a falsifiable model or theory that allows for predicting user behavior or user states based on neurophysiological data. If the model is successful in its predictions it is more likely to eventually provide generalizability (Baldwin and Penaranda, 2012). A systematic and concerted approach in the field of cognitive neuroergonomics should be able overcome some of these limitations. Advances in algorithms and data labeling have begun to show promise in these areas (McKendrick et al., 2019).
Challenge 4: Open Access to Data and Protocols in Neuroergonomics
Reproducible scientific insights are essential to a democratic discourse and provide the basis for the design of a human-centered technology. Open Access (OA) approaches have been established over the last years to counteract restrictive paywalled scientific publication models that often come with high publication costs in established journals currently dominating science. While open access is an important and central aspect of barrier-free knowledge distribution, several additional aspects of publishing should be considered for a reproducible, transparent, and self-controlling scientific system.
For scientific practice to be reproducible and transparent, the experimental procedures, collected data and analyses approaches/code have to be published in combination with the manuscripts so that other researchers can replicate and test the results of a neuroergonomic study. Data sharing is beneficial to the neuroscience community in general (Sejnowski et al., 2014), but comes with its own hurdles including standardization of data formats, incentives to share data as well as its discoverability, among others (Wiener et al., 2016). Publication of data, protocols and code in open repositories (e.g., Github, OSF) might face opposition in some research areas due to, for example, financial interest or security restrictions in applied industrial research. Even where no such restrictions exist, such as in basic research pursuits, reproducibility and transparency practices are not yet the norm. To achieve these goals, it is fundamental to apply standardized approaches that have been developed in different scientific communities and for different modalities over the last years regarding data formatting (e.g., EEG-BIDS, Pernet et al., 2019) and reporting (e.g., CRED-NF for neurofeedback, Ros et al., 2020), alongside guidelines for data analyses and sharing (e.g., COBIDAS, Pernet et al., 2020). These formats and guidelines should be considered as future standards for neuroergonomics to allow for objective checks and balances in this rapidly growing scientific field. Finally, registered reports could be a future gold standard for reproducible basic research in cognitive neuroergonomics to allow for careful planning with sufficient sample sizes providing unbiased results and hopefully more valid neurophysiological parameters that reflect cognitive processes during interaction with technical systems.
Conclusion
The rapidly growing scientific field of cognitive neuroergonomics benefits from new neuroscientific methods and technologies that allow bridging basic to applied research. Mobile imaging modalities in real world settings or near-realistic VR environments provide the opportunity to understand brain activity associated with natural interactions with technical systems. They also provide new opportunities to understand human brain functions associated with cognitive processes during active behavior in dynamically changing environments. These new technological opportunities will come with novel insights into the neural basis of cognitive states and processes. Reproducible scientific approaches in neuroergonomics based on open access and open protocols as well as open data will help to overcome some challenges of these new approaches allowing to address problems of replicability in the field (Barch and Yarkoni, 2013; Stanley et al., 2018). This way, cognitive neuroergonomics might provide new and applicable insights to improve human well-being when interacting with technical systems at work or during leisure.
Author Contributions
All authors listed have made a substantial, direct and intellectual contribution to the work, and approved it for publication.
Conflict of Interest
The authors declare that the research was conducted in the absence of any commercial or financial relationships that could be construed as a potential conflict of interest.
The handling Editor declared a shared affiliation, though no other collaboration, with one of the authors RR.
References
Baldwin, C. L., and Penaranda, B. (2012). Adaptive training using an artificial neural network and EEG metrics for within-and cross-task workload classification. NeuroImage 59, 48–56. doi: 10.1016/j.neuroimage.2011.07.047
Baldwin, C. L., Roberts, D. M., Barragan, D., Lee, J. D., Lerner, N., and Higgins, J. S. (2017). Detecting and quantifying mind wandering during simulated driving. Front. Hum. Neurosci. 11:406. doi: 10.3389/fnhum.2017.00406
Barch, D. M., and Yarkoni, T. (2013). Introduction to the special issue on reliability and replication in cognitive and affective neuroscience research. Cogn. Affect. Behav. Neurosci. 13, 687–689. doi: 10.3758/s13415-013-0201-7
Chavarriaga, R., Ušćumlić, M., Zhang, H., Khaliliardali, Z., Aydarkhanov, R., Saeedi, S., et al. (2018). Decoding neural correlates of cognitive states to enhance driving experience. IEEE Trans. Emerg. Topics Comput. Intell. 2, 288–297. doi: 10.1109/TETCI.2018.2848289
Chryssolouris, G., Mavrikios, D., Fragos, D., and Karabatsou, V. (2000). A virtual reality-based experimentation environment for the verification of human-related factors in assembly processes. Robot. Comput. Integr. Manuf. 16, 267–276. doi: 10.1016/S0736-5845(00)00013-2
Dehais, F., Duprès, A., Blum, S., Drougard, N., Scannella, S., Roy, R. N., et al. (2019). Monitoring pilot's mental workload using ERPs and spectral power with a six-dry-electrode EEG system in real flight conditions. Sensors 19:1324. doi: 10.3390/s19061324
Dehais, F., Karwowski, W., and Ayaz, H. (2020). Brain at work and in everyday life as the next frontier: grand field challenges for neuroergonomics. Front. Neuroergonomics 1:1. doi: 10.3389/fnrgo.2020.583733
Doshi, A., and Trivedi, M. M. (2009). On the roles of eye gaze and head dynamics in predicting driver's intent to change lanes. IEEE Trans. Intell. Transp. Syst. 10, 453–462. doi: 10.1109/TITS.2009.2026675
Duzmańska, N., Strojny, P., and Strojny, A. (2018). Can simulator sickness be avoided? a review on temporal aspects of simulator sickness. Front. Psychol. 9:2132. doi: 10.3389/fpsyg.2018.02132
Faure, C., Fortin-Côté, A., Robitaille, N., Cardou, P., Gosselin, C., Laurendeau, D., et al. (2020). Adding haptic feedback to virtual environments with a cable-driven robot improves upper limb spatio-temporal parameters during a manual handling task. IEEE Trans. Neural Syst. Rehabil. Eng. 28, 2246–2254. doi: 10.1109/TNSRE.2020.3021200
Gateau, T., Ayaz, H., and Dehais, F. (2018). In silico vs. over the clouds: on-the-fly mental state estimation of aircraft pilots, using a functional near infrared spectroscopy based passive-BCI. Front. Hum. Neurosci. 12:187. doi: 10.3389/fnhum.2018.00187
Gehrke, L., Akman, S., Lopes, P., Chen, A., Singh, A. K., Chen, H.-T., et al. (2019). “Detecting visuo-haptic mismatches in virtual reality using the prediction error negativity of event-related brain potentials,” in Proceedings of the 2019 CHI Conference on Human Factors in Computing Systems (Glasgow), 1–11.
Gramann, K., Fairclough, S. H., Zander, T. O., and Ayaz, H. (2017). Trends in neuroergonomics. Front. Hum. Neurosci. 11:165. doi: 10.3389/fnhum.2017.00165
Gramann, K., Gwin, J. T., Ferris, D. P., Oie, K., Jung, T.-P., Lin, C.-T., et al. (2011). Cognition in action: imaging brain/body dynamics in mobile humans. Rev. Neurosci. 22, 593–608. doi: 10.1515/RNS.2011.047
Gramann, K., Hohlefeld, F. U., Gehrke, L., and Klug, M. (2018). Heading computation in the human retrosplenial complex during full-body rotation. BioRxiv [Preprint] 417972. doi: 10.1101/417972
Gramann, K., and Plank, M. (2019). The use of electroencephalography in neuroergonomics. Neuroergonomics 2019, 11–15. doi: 10.1016/B978-0-12-811926-6.00002-6
Hollnagel, E. (2010). “Simulator Studies: the Next Best Thing?” in Simulator-Based Human Factors Studies Across 25 Years, eds A. B. Skjerve and A. Bye (Berlin: Springer), 75–90.
Hu, B., Ma, L., Zhang, W., Salvendy, G., Chablat, D., and Bennis, F. (2011). Predicting real-world ergonomic measurements by simulation in a virtual environment. Int. J. Ind. Ergon. 41, 64–71. doi: 10.1016/j.ergon.2010.10.001
Izzetoglu, M., Izzetoglu, K., Bunce, S., Ayaz, H., Devaraj, A., Onaral, B., et al. (2005). Functional near-infrared neuroimaging. IEEE Trans. Neural Syst. Rehabil. Eng. 13, 153–159. doi: 10.1109/TNSRE.2005.847377
Jeunet, C., Albert, L., Argelaguet, F., and Lécuyer, A. (2018). “Do you feel in control?”: towards novel approaches to characterise, manipulate and measure the sense of agency in virtual environments. IEEE Trans. Vis. Comput. Graph. 24, 1486–1495. doi: 10.1109/TVCG.2018.2794598
Jungnickel, E., Gehrke, L., Klug, M., and Gramann, K. (2019). MoBI—mobile brain/body imaging. Neuroergonomics 2019, 59–63. doi: 10.1016/B978-0-12-811926-6.00010-5
Jungnickel, E., and Gramann, K. (2016). Mobile brain/body imaging (MoBI) of physical interaction with dynamically moving objects. Front. Hum. Neurosci. 10:306. doi: 10.3389/fnhum.2016.00306
Klug, M., and Gramann, K. (2020). Identifying key factors for improving ICA-based decomposition of EEG data in mobile and stationary experiments. Eur. J. Neurosci. doi: 10.1111/ejn.14992. [Epub ahead of print].
Makeig, S., Gramann, K., Jung, T.-P., Sejnowski, T. J., and Poizner, H. (2009). Linking brain, mind, and behavior. Int. J. Psychophysiol. 73, 95–100. doi: 10.1016/j.ijpsycho.2008.11.008
McKendrick, R. (2019). Mobile neuroergonomics: action, interfaces, cognitive load, and selective attention. Neuroergonomics 2019, 111–116. doi: 10.1016/B978-0-12-811926-6.00018-X
McKendrick, R., Feest, B., Harwood, A., and Falcone, B. (2019). Theories and methods for labelling cognitive workload: classification and transfer learning. Front. Hum. Neurosci. 13:295. doi: 10.3389/fnhum.2019.00295
McKendrick, R., Mehta, R., Ayaz, H., Scheldrup, M., and Parasuraman, R. (2017). Prefrontal hemodynamics of physical activity and environmental complexity during cognitive work. Hum. Factors 59, 147–162. doi: 10.1177/0018720816675053
Parada, F. J. (2018). Understanding natural cognition in everyday settings: 3 pressing challenges. Front. Hum. Neurosci. 12:386. doi: 10.3389/fnhum.2018.00386
Parasuraman, R. (2003). Neuroergonomics: research and practice. Theor. Issues Ergon. Sci. 4, 5–20. doi: 10.1080/14639220210199753
Pernet, C., Garrido, M. I., Gramfort, A., Maurits, N., Michel, C. M., Pang, E., et al. (2020). Issues and recommendations from the OHBM COBIDAS MEEG committee for reproducible EEG and MEG research. Nat. Neurosci. 23, 1–11. doi: 10.1038/s41593-020-00709-0
Pernet, C. R., Appelhoff, S., Gorgolewski, K. J., Flandin, G., Phillips, C., Delorme, A., et al. (2019). EEG-BIDS, an extension to the brain imaging data structure for electroencephalography. Sci. Data 6, 1–5. doi: 10.1038/s41597-019-0104-8
Protzak, J., and Gramann, K. (2018). Investigating established EEG parameter during real-world driving. Front. Psychol. 9:2289. doi: 10.3389/fpsyg.2018.02289
Ros, T., Enriquez-Geppert, S., Zotev, V., Young, K. D., Wood, G., Whitfield-Gabrieli, S., et al. (2020). Consensus on the Reporting and Experimental Design of Clinical and Cognitive-Behavioural Neurofeedback Studies (CRED-nf Checklist). Oxford: Oxford University Press.
Sejnowski, T. J., Churchland, P. S., and Movshon, J. A. (2014). Putting big data to good use in neuroscience. Nat. Neurosci. 17, 1440–1441. doi: 10.1038/nn.3839
Shi, Y., Zhu, Y., Mehta, R. K., and Du, J. (2020). A neurophysiological approach to assess training outcome under stress: a virtual reality experiment of industrial shutdown maintenance using Functional Near-Infrared Spectroscopy (fNIRS). Adv. Eng. Inform. 46:101153. doi: 10.1016/j.aei.2020.101153
Stanley, T. D., Carter, E. C., and Doucouliagos, H. (2018). What meta-analyses reveal about the replicability of psychological research. Psychol. Bull. 144:1325. doi: 10.1037/bul0000169
Tsarouchi, P., Makris, S., and Chryssolouris, G. (2016). Human–robot interaction review and challenges on task planning and programming. Int. J. Comput. Integr. Manuf. 29, 916–931. doi: 10.1080/0951192X.2015.1130251
Vitorio, R., Stuart, S., Rochester, L., Alcock, L., and Pantall, A. (2017). FNIRS response during walking—artefact or cortical activity? a systematic review. Neurosci. Biobehav. Rev. 83, 160–172. doi: 10.1016/j.neubiorev.2017.10.002
Wascher, E., Reiser, J. E., Rinkenauer, G., Larra, M. F., Dreger, F., Schneider, D., et al (2020). Neuroergonomics on the Go. A Preview of the Potential of Mobile EEG for Work-Place Evaluation and Design. [Preprint]. Available online at: https://osf.io/vkf79
Wiener, M., Sommer, F. T., Ives, Z. G., Poldrack, R. A., and Litt, B. (2016). Enabling an open data ecosystem for the neurosciences. Neuron 92, 617–621. doi: 10.1016/j.neuron.2016.10.037
Wunderlich, A., and Gramann, K. (2020). Landmark-based navigation instructions improve incidental spatial learning in real-life environments. BioRxiv [Preprint] 789529. doi: 10.1101/789529
Zhu, Y., Johnson, C., Chang, S.-H. J., and Mehta, R. K. (2020a). “Neuroergonomics metrics to evaluate Exoskeleton based Gait Rehabilitation,” in 2020 IEEE International Conference on Systems, Man, and Cybernetics (SMC) (Toronto, ON), 3447–3453.
Keywords: cognitive neuroergonomics, mobile brain imaging, open access, virtual reality, embodied cognition, EEG, fNIRS
Citation: Gramann K, McKendrick R, Baldwin C, Roy RN, Jeunet C, Mehta RK and Vecchiato G (2021) Grand Field Challenges for Cognitive Neuroergonomics in the Coming Decade. Front. Neuroergon. 2:643969. doi: 10.3389/fnrgo.2021.643969
Received: 19 December 2020; Accepted: 18 February 2021;
Published: 08 March 2021.
Edited by:
Frederic Dehais, Institut Supérieur de l'Aéronautique et de l'Espace (ISAE-SUPAERO), FranceReviewed by:
Robert W. Proctor, Purdue University, United StatesCopyright © 2021 Gramann, McKendrick, Baldwin, Roy, Jeunet, Mehta and Vecchiato. This is an open-access article distributed under the terms of the Creative Commons Attribution License (CC BY). The use, distribution or reproduction in other forums is permitted, provided the original author(s) and the copyright owner(s) are credited and that the original publication in this journal is cited, in accordance with accepted academic practice. No use, distribution or reproduction is permitted which does not comply with these terms.
*Correspondence: Klaus Gramann, a2xhdXMuZ3JhbWFubkB0dS1iZXJsaW4uZGU=