- Division of Neurology, Faculty of Medicine, University of Manitoba, Winnipeg, MB, Canada
The internal sensation of memory, which is available only to the owner of an individual nervous system, is difficult to analyze for its basic elements of operation. We hypothesize that associative learning induces the formation of functional LINK between the postsynapses. During memory retrieval, the activation of either postsynapse re-activates the functional LINK evoking a semblance of sensory activity arriving at its opposite postsynapse, nature of which defines the basic unit of internal sensation – namely, the semblion. In neuronal networks that undergo continuous oscillatory activity at certain levels of their organization re-activation of functional LINKs is expected to induce semblions, enabling the system to continuously learn, self-organize, and demonstrate instantiation, features that can be utilized for developing artificial intelligence (AI). This paper also explains suitability of the inter-postsynaptic functional LINKs to meet the expectations of Minsky’s K-lines, basic elements of a memory theory generated to develop AI and methods to replicate semblances outside the nervous system.
Introduction
The concept of classical neural network evolved from Hebb’s postulates (Hebb, 1949) assumes that memory is stored as patterns of synaptic changes. The electro-physiological correlates of long-lasting increase and decrease in synaptic strength were demonstrated as experimental long-term potentiation (LTP) and depression (LTD) respectively. Stable high-resolution synaptic weight values were considered as feasible physical substrates for long-term memory, since synaptic weights can be optimized for any arbitrary pattern. The networks are expected to learn any arbitrary function when the training distribution is similar to the test distribution, facilitating probabilistic analysis and leading to the initial building of artificial intelligence (AI) (Achler and Amir, 1999). The same principles have been used in computational modeling (Favorov and Kursun, 2011), applied in developing synthetic neural modeling (Edelman et al., 1992), and used in developing machines with memory capabilities (Edelman, 2007).
At the level of neuronal activation, binding by neural synchrony (Legendy, 1970; Milner, 1974) was formulated as a general phenomenon (von der Malsburg, 1981) that can make coarse coding possible and was found suitable to combine elementary symbols to those with more complex structures. Based on this foundation, these structures can be identified, decomposed, and further combined to build higher structures. However, with respect to binding, there are many issues that the models based on connection (synaptic) strengths face. By assigning neurons that co-activate to represent one composite symbol at one time, neuronal activity fails to represent specific characters to be associated with a specific item (“item” means anything that relates to the real world) when more than one composite symbol is to be expressed at one time, leading to binding ambiguity (von der Malsburg, 1999). Since the amount of binding ambiguity that one network can tolerate is correspondingly small, a mechanism that can avoid this issue is yet to be discovered. Since it may take hundreds of repetitions to completely decouple non-corresponding regions to get rid of the ambiguous connections and reorganize the system, it is not seen as a physiologically feasible time-economic mechanism (von der Malsburg, 1999).
Similar to the above issue, adequate provisions for segmentation of a complex sensory modality for sensing its individual parts (von der Malsburg, 1999) have not yet been explained. Since learning algorithms require training distribution and test distribution to be the same (Achler and Amir, 1999), every pair of patterns need to be trained. Without this, simultaneous activation of sets of neurons from two previously learned symbols when presented together can lead to a superposition catastrophe (von der Malsburg, 1999; Rachkovskij and Kussul, 2001) and limit the development of AI. In addition, mechanisms should be in place for the system to generate hypotheses and to allow interaction between internally generated hypotheses and external evidence that permits sensory data to support or reject internal constructs extremely efficiently (Abbott, 2008). Another requirement of an intelligent system is pattern instantiation, where the patterns need to be evaluated reliably at physiological time-scales such that the system will be able to differentiate true correlations from background noise (von der Malsburg, 1999).
Even though correlation between synaptic weight changes and plasticity changes during learning were examined by different modeling studies, the link between abstract synaptic weights and the physical substrate for long-term memory was shown to be weakened by experimental results (Poirazi and Mel, 2001). In addition, various investigators consider that classical neural networks may not be able to explain a range of brain functions (Rosenblatt, 1961; von der Malsburg, 1986, 1999; Fodor and Pylyshyn, 1988). Even though binding by neural synchrony (Legendy, 1970; Milner, 1974; von der Malsburg, 1981) has been suggested as a mechanism that can make coarse coding possible, neither combination coding cells (Treisman and Gelade, 1980; Wiskott and von der Malsburg, 1995), nor mechanisms that can decouple non-corresponding regions to remove ambiguous connections and reorganize the system (von der Malsburg, 1999) have been discovered. A self-regulatory feedback network that can overcome the superposition catastrophe problem faces issues as well, in that its functional characteristics are difficult to derive analytically (Achler and Amir, 1999).
Even though the approach of neuroscience and AI fields support a structured mathematical-statistical framework, the actual mechanism is expected to require non-linear methods that function beyond the distribution limits (Achler and Amir, 1999). To make further progress in understanding memory, two major issues need attention. (a) Biological experiments are limited by the lack of tools that can resolve electro-physiological changes taking place at the level of individual synapses. One feasible solution is to make hypotheses from biological functions that can theoretically satisfy all the requirements and then carry out studies to replicate them in physical states. Once the operation of a simple nervous system and the basic rules of the complexity are understood, then biologically based computational models of high level cognition (O’Reilly, 2006) can be used to develop AI from natural intelligence (Potter, 2007). (b) Since it is difficult to test internal sensations of higher functions, replicating the mechanism outside the nervous system with proper read-outs for such internal sensations is required to understand their operation. With these aims, we derived a new theoretical framework based on which both computational and neuro-engineering experiments can be carried out. An abstract form of this work will be presented at the annual meeting of the Society for Neuroscience, 2011 (Vadakkan, 2011b).
Derivation of the Hypothesis
Basic units for the virtual internal sensations
Memory for each individual event can be de-constructed into a series of associative representations of the learned items (Tulving, 2002). Items in the environment can induce multiple sensory stimuli that can simultaneously stimulate different sensory systems. During these events, associative learning makes changes in the nervous system such that at a later time, the arrival of one of the stimuli alone triggers the virtual internal sensation of the remaining sensory properties of the item; namely, semblances that provide the fundamental basis of memory. The present work uses an inductive reasoning approach to define the required elements within the nervous system and examines the possible cellular mechanisms that govern them.
A large body of experimental evidence demonstrates the activities of new set of neurons by one stimulus after the animals have undergone associative learning with a second stimulus. Both experimental (Cheng and Frank, 2008; Tye et al., 2008) and computational (Kepecs et al., 2008; Lavigne and Darmon, 2008) studies have shown activities of such neurons during memory retrievals. Firing of the same neurons in the hippocampus has been shown to occur during specific memory recall in humans (Gelbard-Sagiv et al., 2008). This has led to the present consensus that memories are distributed across a population of neurons. How do these neurons represent memory? A sizable body of experimental evidence also suggests that the activation of neuronal sets evokes internal sensations of memories and hallucinations (Heit et al., 1988; Kosslyn et al., 1995; Quirk et al., 1995; Dierks et al., 1999; Kreiman et al., 2000; Kraemer et al., 2005; Gelbard-Sagiv et al., 2008). How does the firing of a neuron impart sensory meaning to the nervous system that it is a part of? Recently, arguments have been made for the requirement for substantive role of internal representation in the brain (Sullivan, 2010). We may be able to understand memory by deriving a mechanism that can explain units for virtual internal sensation concurrent with the activation of new sets of neurons that may appear to represent retrieved memories.
Potential changes during learning and memory retrieval
A feasible mechanism that can provide units to build the virtual sensation of the hidden sensory stimulus from an item has been postulated through the semblance hypothesis (Vadakkan, 2011c). During the acquisition of simultaneous multiple sensory inputs from an object in the environment, a mechanism must take place in the nervous system whereby a subset of the synapses and neurons that represent one sensation (learned item) should be able to be activated by the second sensation (cue) and vice versa during memory retrieval. This leads to the assumption that during learning, the co-activation of the postsynapses from different sensory stimuli will induce specific changes between them that will later allow one of the stimuli alone to evoke the re-activation of the postsynapses that can represent the remaining sensory stimuli from the item (Figure 1A). Since structural changes were not observed between the postsynapses during associative learning, the formation and re-activation of the interconnection between the postsynapses during learning and memory retrieval respectively are hypothesized as transient functional LINKs (capital letters are used to connote its significance).
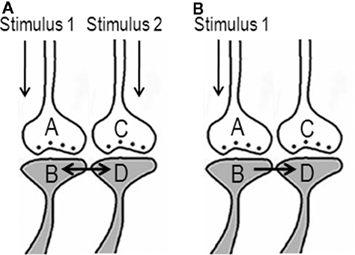
Figure 1. Anticipated changes between the postsynapses during learning that enable the activation of the postsynapse of a synapse without the activation of its corresponding presynapse. (A) The illustration shows functional LINK formation between the two postsynaptic membranes B and D during learning. The functional LINK is transient and its formation is a function of the simultaneous activation of postsynapses B and D. A and C are the corresponding presynapses. (B) During memory retrieval in the presence of one of the stimuli, the functional LINK is re-activated, resulting in the activation of postsynaptic membrane D. Activation of postsynapse D induces the semblance of activity from presynapse C (Figure was used after modification from Vadakkan, 2011c).
Activation of a postsynapse (postsynaptic terminal or dendritic spine) can be considered as a marker of the arrival of an action potential at its presynapse (presynaptic terminal). It can be assumed that the activation of the postsynapse of a synapse representing the learned item, by the cue stimulus during memory retrieval, without the activation of its corresponding presynapse induces changes at the postsynapse reminiscent of the arrival of activity at the latter’s presynapse and evokes the semblance of the arrival of activity from its presynapse. This cellular hallucination of activity arriving from the presynapse (presynapse C in Figure 1B) and is called “synaptic semblance.” In order to understand what postsynapse D is hallucinating about, we need to examine the reverse pathway from presynapse C towards the sensory receptors whose stimulation can activate presynapse C.
Activation of neurons during memory retrieval
Various experimental results (Cheng and Frank, 2008; Tye et al., 2008) have led to the idea that memories are distributed across a population of neurons. How does the mechanism of synaptic semblance (Figure 1A) lead to the concurrent firing of specific neurons during memory retrieval? This may be explained as follows. Oscillating neuronal activities take place both in the hippocampus and in the cortex. Different memory tasks are shown to be associated with frontal and hippocampal cortical theta oscillations in humans (Tesche and Karhu, 2000; Jensen and Tesche, 2002) and in rodents (Winson, 1978). During these oscillating neuronal activities, action potentials reach the axonal terminal (presynaptic terminal or presynapse) leading to synaptic transmission to the postsynaptic terminal (postsynapse) inducing an excitatory postsynaptic potential (EPSP) at the postsynapse. The spatial summation of nearly 40 EPSPs (or the temporal summation of less than 40 EPSPs) from nearly 40 postsynapses (dendritic spines) out of an average 2.4 × 104 to 8 × 104 postsynapses (Abeles, 1991) triggers action potential at some of the next order of neurons. However, many neurons in these and next orders of neurons will not get sufficient summated EPSPs to reach the threshold for inducing an action potential. Let the specific set of neurons that undergo sub-threshold activation be {Q}. Addition of more EPSPs to the sub-threshold activation in the neurons in the set {Q} by any event can induce their firing.
In the above background of baseline oscillating neuronal activity, what will happen when a new cue stimulus arrives? Since the hippocampus is an area where inputs from different sensations converge, it is anticipated that many functional LINKs will be re-activated during memory retrieval. Compared to the hippocampus, the convergence of synapses in the cortex may take place either through the convergence of higher orders of neurons from neurons in the hippocampus or through extra-hippocampal pathways. During memory retrieval, the resulting EPSPs from postsynapses (similar to postsynapse D in Figure 2) of the neurons can add to the sub-threshold activation at the axon hillocks of specific neurons in the set {Q} (described in the above paragraph) eliciting action potentials. Firing of these specific neurons during memory retrieval can lead to the interpretation that memories are distributed across a specific population of neurons.
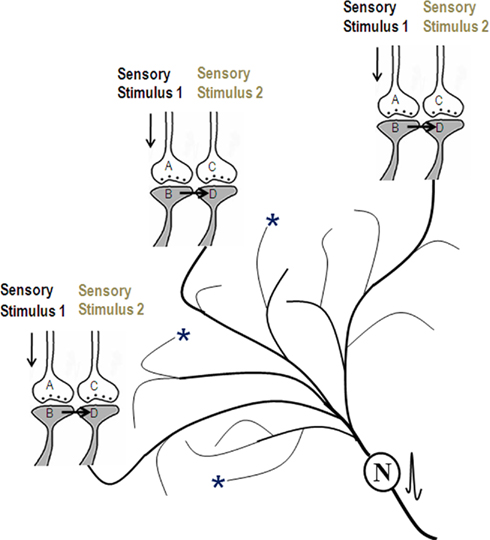
Figure 2. Schematic representation of activation of postsynapses Dn through the functional LINKs leading to the generation of action potential at the axon hillock of their neuron N (provided spatial and/or temporal summation of sufficient EPSPs is possible). Concurrent with this, synaptic semblances are induced at each postsynapses D. The dendritic spines (postsynapses) marked with asterisks denote locations where EPSPs arrive from the background oscillating neuronal activities, at their previous neuronal orders, through normal synaptic transmission. Note that the EPSPs arriving from the neuronal oscillations alone are not sufficient to elicit an action potential in neuron N. When memory retrieval induces activation of postsynapses Dn, then the summated EPSPs become sufficient to induce action potential in neuron N. (Figure was used after modification from Vadakkan, 2010).
Nature of virtual internal sensations
Here, we derive the nature of semblances occurring at the postsynapse activated through the functional LINKs (Figure 3). The derived features of virtual internal sensation are imaginary. Based on the idea of semblances (Figure 1), the activation of neuron N or postsynapse D (in Figure 3) can lead to semblance of activity coming from neuron Z in the neuronal order 8. Let us assume that neuron Z is normally depolarized by activating a set of axonal terminals of the neurons in order 7 in Figure 3 that synapse to neuron Z’s dendritic spines (postsynapses). The spatial summation of nearly 40 or the temporal summation of less than 40 EPSPs (from nearly 40 postsynapses (dendritic spines) out of the nearly 5 × 104 postsynapses of each neuron) triggers an action potential at neuron Z’s axon hillock. This may be true for a novice nervous system where we anticipate synaptic transmission to be the only method of communication between the neurons. Based on the present hypothesis, as associative learning begins to take place in a newborn, new functional LINKs are formed between the postsynapses. This then leads to the possibility that activity may also arrive at some of the postsynapses (dendritic spines) of neuron Z through the re-activation of their functional LINKs with other postsynapses either by baseline oscillatory neuronal activities or by an external sensory (cue) stimulus (Figure 3).
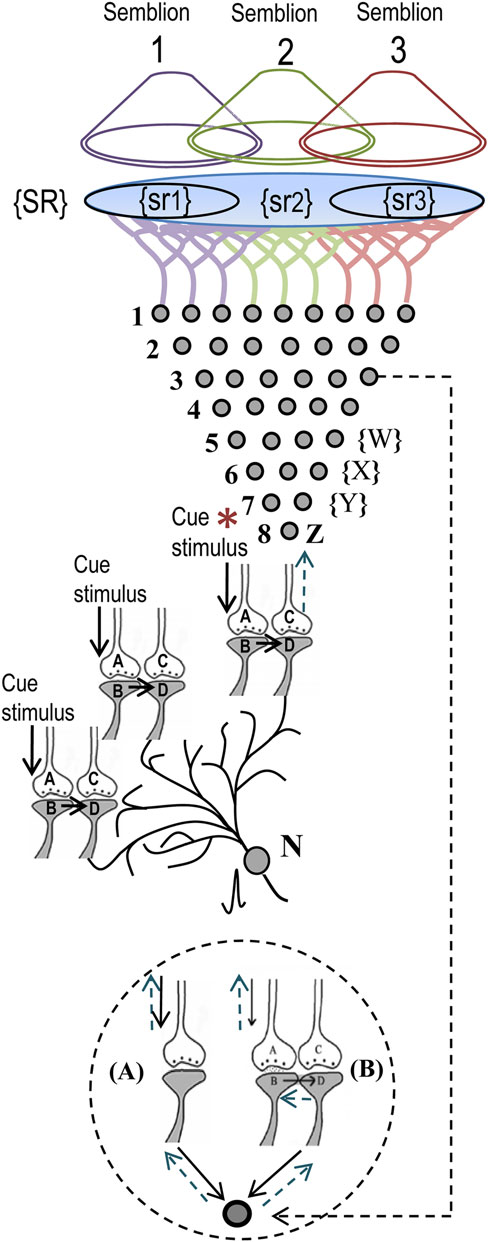
Figure 3. Schematic representation of sensory elements induced during the activation of a synapse or a neuron. The gray circles represent neurons. The numbers on the left side of the neuronal orders denote their position in relation to the sensory receptors. Neuron Z is shown in neuronal order 8. During memory retrieval, a cue-stimulus (marked by asterisk) reaching presynaptic terminal A depolarizes its postsynaptic membrane B and the resulting EPSP at postsynapse B re-activates the functional LINK that activates postsynaptic membrane D (Mechanisms other than depolarization are also discussed (Vadakkan, 2011c). When postsynaptic membrane D is depolarized, it evokes the cellular hallucination of an action potential reaching its presynaptic terminal C. This is called synaptic semblance. Note that presynapse C belongs to the neuron Z. Either synaptic semblance occurring at the postsynapse D or random activation of neuron Z produces the hallucination that it is receiving inputs from the set of neurons {Y} that synapse to it. The set of neurons {Y} are activated by the activation of the set of neurons {X}. The set of neurons {X} in turn are activated by the set of neurons {W}. (Recurrent collaterals and projection neurons can also activate a higher-order neuron. For simplicity these are not shown). Continuing this extrapolation towards the sensory level identifies a set of sensory receptors {SR}. It can be seen that stimulation of subsets of sensory receptor sets {sr1}, {sr2}, and {sr3} from the set {SR} may be capable of independently activating neuron Z. The dimensions of hypothetical packets of sensory stimuli capable of activating the sensory receptor sets {sr1}, {sr2}, and {sr3} are called semblions 1, 2, and 3 respectively. These semblions are viewed as the basic building blocks of the virtual internal sensations of memory. A cue stimulus can cause postsynapse D to hallucinate about any of the semblances 1, 2, 3, or an integral of them. Activation of the postsynapse D or the neuron N by the cue stimulus or the artificial activation of neuron Z can lead to the virtual internal sensation of semblions 1, 2, 3, or an integral of them. A method of integrating the semblions that match with the internal sensations induced by the cue stimulus may be determined by computational studies. Inset: Circles represent the soma of neurons. (A) A dendritic spine (postsynapse) of a neuron receives synaptic transmission. (B) Another of its dendritic spine receives activity through a functional LINK. Straight arrows show normal spread of activity. Dotted arrows show the direction of extrapolation of semblance.
Let the set of all combinations (for the spatial summation of EPSPs) and permutations (for the temporal summation of EPSPs) of the neurons in neuronal order 7 whose activity through normal synaptic transmission [(A) in the inset of Figure 3] can give rise to a single action potential at neuron Z’s axon hillock be {Y}. Let {Y−y} represents the set of neurons whose activity can re-activate functional LINKs [inset (B) Figure 3] that lead to the generation of potentials contributing to the generation of action potential in neuron Z. Therefore, the set of neurons whose activation can lead to generation of action potential in neuron Z is {Y} + {Y−y} = {Y}. It is likely that different neurons in set {Y} will have different weights (probabilities) for being the subject of hallucination at postsynapse D. This likely depends on previous patterns of synaptic transmission and functional LINK formation and re-activation. The exact rules for the extrapolation from neuron Z to set {Y} need further study. It is likely that this approach will lead to finding certain hotspot neurons in set {Y} on the path of extrapolation of semblances from neuron Z.
Similar to the above mechanism, the neurons in set {Y} in turn receive synaptic transmissions and spread of activity through the functional LINKs from a set of neurons {X} in neuronal order 6. By extrapolating in a retrograde fashion towards the sensory level, it will be possible to determine the set of sensory receptors {SR} whose activation could theoretically cause the activation of neuron Z. Dimensions of internal sensations resulting from the activation of neuron Z will be related to a sensory stimulus that can activate sensory receptors in the set {SR}. Similar to the hotspot neurons in the set {Y} explained in the above paragraph, hotspots of sensory receptors are expected in the set {SR} for semblances from neuron Z.
From the set {SR}, subsets of a minimum number of sensory receptors required to activate postsynapse D or neuron Z is made (example, {sr1}, {sr2}, and {sr3} in Figure 3). A hypothetical packet of minimum sensory stimuli called “semblion” capable of activating one of the above subsets of sensory receptors that can activate neuron Z or postsynapse D (Figure 3) is derived. This is considered as the basic unit of internal sensation. As the cue stimulus passes through different functional LINKs, it evokes large number of semblances as explained above. Identify all the possible semblions. The final step is to learn about the integration of semblions with the dimension of “time of synaptic delay” (to account for differences in times of induction of semblances at different neuronal orders) to obtain a multi-dimensional net semblance that matches the sensory characteristics of the item whose memory needs to be retrieved. This may reveal the nature of essential computation of the semblions, the integration process with the time of synaptic delay occurring at each neuronal order and the nature of oscillatory waveforms required to occur at specific neuronal orders for a given nervous system.
Since the number of functional LINKs continues to change (due to continued associative learning) over the life span of the nervous system, the characteristic features of the semblions are also expected to change gradually. Therefore, the nature of the internal sensations evoked as a response to a given cue stimulus also changes with time.
Advantages of functional LINKs
Continued associative learning events induce additional functional LINKs to a given postsynapse leading to the formation of islets of functional LINKs (Figure 4). Postsynapses connected though strong functional LINKs are expected to move towards the center of the islet. Based on the frequency of their previous use, the strength of these functional LINKs may vary. Islets of functional LINKs have to be viewed as highly dynamic functional domains as the strength of functional LINKs changes. It is anticipated that activation of one postsynapse can lead to the activation of other postsynapses in the islet based on the strength of functional LINKs.
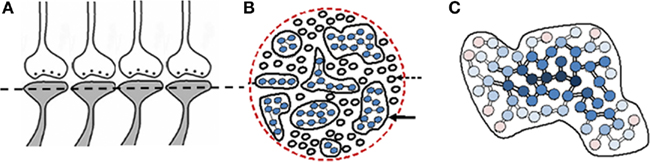
Figure 4. Islets of functionally LINKed postsynapses and their contribution to the stability of memory. (A) Four synapses are shown side by side that have the potential to form functional LINKs between their postsynapses. The dotted line shows cross-section across these and other postsynapses. (B) Cross-sectional view through a large number of postsynapses as shown in (A). When learning occurs, functional LINKs are formed between the simultaneously activated postsynapses. Continued learning using any of those already LINKed postsynapses will increase the number of inter-LINKed postsynapses forming islets of functionally LINKed postsynapses (solid arrow). The individual islets are expected to be functionally separate from each other (dotted arrow; C) Schematic representation of one large islet of functionally LINKed postsynapses. Newly LINKed postsynapses (pink) remain at the periphery of an islet. Rarely used LINKed postsynapses (pink circles) move towards the periphery and commonly used ones (dark blue) move towards the center of an islet (The periphery–central movement needs to be considered as a functional event). The rarely used and newly formed functional LINKs will lose their function unless re-used. Those postsynapses at the center (dark blue) may eventually develop more stable functional LINKs (Figure was used after modification (Vadakkan, 2011c).
The “functional” nature (semblance formation as a function of activation of the postsynapse) is essential for providing the system with flexibility (postsynapses are ready to get activated provided the cue stimulus can re-activate the functional LINKs to them) and degeneracy (activation by different cue stimuli can activate the same postsynapse that is part of an islet of functionally LINKed postsynapses), properties that will enable incremental learning along with the maintenance of specificity in responding to a specific cue stimulus at different times. In an environment with an infinite number of sensory stimuli, the above features are essential for maximizing memory storage abilities of a nervous system with finite number of basic units of memory storage.
What constitutes the functional LINK is yet to be discovered. Even though neurotransmitter spread to the neighboring synapses (Coggan et al., 2005; Fernandes et al., 2010), 2-amino-3-(5-methyl-3-oxo-1,2-oxazol-4-yl) propanoic acid (AMPA) receptor trafficking (Makino and Malinow, 2009) and ephaptic coupling (Anastassiou et al., 2011) can be hypothesized to occur between the postsynapses, these events lack some of the required characteristics of functional LINK formation and re-activation. First, it is not known whether any mechanism exists for guiding the specificity of neurotransmitter spread, AMPA receptor trafficking or ephaptic coupling between two specific postsynaptic membranes that can induce functional LINKs between them during associative learning. Secondly, the time-scales of AMPA receptor trafficking may not be sufficient to explain the time-scales of functional LINK formation and re-activation.
Suitability of Semblance Hypothesis
Observed Activation of Specific Neurons
The lateral amygdala (LA) is a site of convergence of sensory inputs from the conditioned and unconditioned stimuli in fear conditioning experiments (McDonald, 1998; Maren and Quirk, 2004). Cue-associated learning of certain reward-induced changes at the thalamo-amygdala synapses (Tye et al., 2008) has shown firing of additional LA neurons. The associative learning ability of animals is found to be directly proportional to the additional number of firing neurons, in the presence of the cue stimulus, during memory retrieval (Tye et al., 2008). According to the semblance hypothesis, these additional neurons represent the learned item (for example, neuron N in Figures 2 and 3). During learning, functional LINKs are formed between the postsynapses of the synapses belonging to the cue and the learned item at locations of their convergence. During memory retrieval, activity from the cue passes through the LINKed postsynapses at locations where synapses from two different sensory inputs converge. The EPSPs spreading through the functional LINKs then reach the dendritic tree of the neurons representing the learned item. As a result, we expect spatial and temporal summation of EPSPs at the regions of convergence enabling the cue stimulus to activate additional neurons.
Long-Term Potentiation
Long-term potentiation (LTP) is considered a long-lasting increase in synaptic efficacy following experimental high-frequency stimulation of afferent fibers and has been shown to correlate with learning (Bliss and Collingridge, 1993; Whitlock et al., 2006). During this protocol, hundreds of axons of the input pathway are stimulated, leading to the activation of the postsynapses of the next order of neurons. It can be explained that many of these postsynapses become functionally LINKed to form islets and mega-islets of functional LINKs due to the simultaneous activation of closely placed postsynapses by high-frequency stimulation (Vadakkan, 2011c). Following this, the activation of one or more postsynapses by a regular stimulus can lead to the spread of depolarization between the postsynapses within those mega-islets. Since the next order neuron from which electro-physiological changes are recorded has its postsynapses within the large mega-islets, stimulation by regular stimulus will show summated EPSPs arriving from the postsynapses of the mega-islets for long periods of time contributing to LTP. Even though many of the newly formed functional LINKs within the mega-islets of functional LINKs will lose their function over time, their cumulative effect will exhibit sufficiently increased potentiation observed as LTP for a certain period of time.
Transfer of Memories from the Hippocampus to the Cortex
Based on the present hypothesis, incorporation of hippocampal new neurons in the circuitry along with the repetition of learning or related learning or the re-activation of the same sensory receptor pairs during unrelated learning increases the number of functional LINKs in the cortex (Vadakkan, 2011a,c). This provides increase in semblances from the cortex, during memory retrieval, which when reach above a certain threshold can provide memory for the learned item without contribution of semblances from the hippocampus. At this stage, removal of the hippocampus that leads to loss of recently acquired memories while retaining the old memories evokes an apparent time-dependent transfer of memories from the hippocampus to the cortex, a process considered responsible for consolidation of memories.
Other Correlations
Provided functional LINKs are re-activated by the repetition of learning or related learning or the activation of sensory receptor pairs that took part in its formation, these functional LINKs can be maintained in a re-activable state. Depending on the cue specificity and the dilutional effect of changing features of semblions over time (due to hippocampal new neuronal incorporation and new synapse formation), the maintained functional LINKs provide possibilities for unlimited memory life-times (Vadakkan, 2011c) that is considered as an important requirement of a memory system (Rubin and Fusi, 2007). Even though both the sensory receptors and potential functional LINKs that can be formed are limited, the combinatorial re-activation of functional LINKs from a pool of shared functional LINKs during various memory retrievals provides very large memory stores (Abbott, 2008). Incorporation of new neurons without repetition of learning or related learning or the re-activation of the same sensory receptor pairs used in unrelated learning can create new connections with higher-order neurons. Since the latter neurons have already made specific functional LINKs representing different associative learning, it leads to the formation of non-specific semblances during memory retrieval, causing a reduction in previous memories.
Since semblances formed from a large pool of re-activable functional LINKs are a function of the specificity of the cue stimulus, it will not lead to overwriting of old memories by new ones – another requirement of a memory system (Rubin and Fusi, 2007). As new learning expands the islet of functional LINKs, combinatorial activation of postsynapses will help to increase the sensitivity of memory retrieval, possibly at the cost of specificity (Rubin and Fusi, 2007). A related task can be easily learned associatively since a large proportion of the required functional LINKs already exists in the nervous system from previous learning. Directional changes of the whiskers that evoke a sequence of sensory inputs (Tsytsarev et al., 2010) can be explained as sequential changes in semblances. This cue-dependent changes in semblances can be viewed accountable for dynamic representation of the somato-sensory cortex (Tommerdahl et al., 2010).
Functional LINKs Likely to Meet the Expectations of Minsky’s K-Lines
Both the functional LINKs that operate as a function of arrival of activity and the virtual internal sensation from the semblances are difficult to demonstrate in biological systems. Experiments in physical systems can use additional segments to obtain read-outs to understand their nature. In this regard, successful development of AI from the present hypothesis is one of the ways to explore biological memory storage mechanism. According to an AI proposal put forward by Marvin Minsky (Minsky, 1980, 1986), the formation of K-lines or Knowledge-lines that are mental agents representing an association of a group of mental agents is proposed to occur actively when a subject solves a certain problem or formulates a new idea (Figure 5). These K-lines are expected to facilitate thinking by analogy (Minsky, 1994). Currently, various applications of K-lines are being examined (Toptsis, 2009).
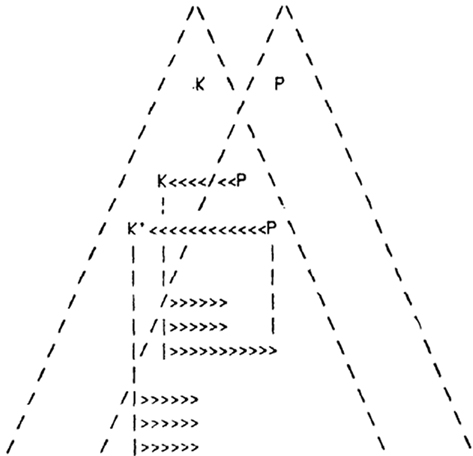
Figure 5. Minsky’s K-lines (in arrows) connecting P- and K- agents. Minsky envisioned that “K-agents lie anatomically near the P-agents of corresponding levels, making it easy for K-lines to contact either P- or K-agents. Perhaps in early stages of growth the connections are primarily to P-agents. Later, under genetic control, the preferences tend to shift over from P’s to K’s.” Figure is used with permission from (Minsky, 1980).
The ultimate purpose of the K-lines is to re-enact what was associatively learned during the retrieval of memory. While K-lines have been proposed to meet the theoretical requirements in abstract terms, functional LINKs between the postsynapses can explain the expected connections (for comparison see Table 1). The method by which these functional LINKs can produce sufficient functional attributes in the form of semblances may be translated to develop AI. For computing the semblances, we must characterize the semblions and then compute them to obtain the virtual internal sensation as an output that enable comparison of its dimensions with that of the learned item. The K-Line theory has not been yet proved, possibly due to the fact that P-pyramids and K-pyramids representing the learned item and the cue stimulus respectively are abstract features.
Machine Teaching and Retrieval of Internal Sensations
Basic Elements of the System
Neurons that connect the sensory receptors are the first order of neurons and those that form synapses with them are the second order and so on. Most of the items in the environment can stimulate more than one sensory system. The number of sensory receptors of an animal is finite. It is usually assumed that in humans there are nearly 1010 neurons, and on average, each neuron has 2.4 × 104 to 8 × 104 dendritic spines postsynapses (Abeles, 1991) and it is reasonable to assume that each neuron has the same number of axonal terminals that form presynaptic terminals at the synapses with the next order of neurons. The presynapses of one neuron synapses with the postsynapses of the neurons in the next order. The information is passed across one synapse by chemical transmission with a synaptic delay of nearly 1–2 ms. The chemical neurotransmitter molecules open the receptor channels at the postsynapses and induce an EPSP. When EPSPs from different postsynapses reach the axon hillock of the neuron (the beginning portion of the axon close to the soma), an action potential (spike) is triggered. The spatial summation of nearly 40 or the temporal summation of much less than 40 EPSPs can achieve this. Summated potential below certain threshold value will not induce action potential, making it an all-or-none phenomenon. Not all the EPSPs generated at the postsynapses can reach the axon hillock for generating the action potential for various reasons (Stuart et al., 1997).
Higher-order neurons from different sensations converge at certain locations within the nervous system. The hippocampus is an example of such an area. Some of the sensory inputs converge even before reaching the hippocampus. Therefore, we do not completely know the specific orders at which the initial convergence takes place. For computational purposes, it can be arbitrarily considered that the hippocampus contains neuronal orders 6 and 7. At this stage, we must assume that at orders of neurons where sensory inputs converge, formation, and re-activation of the functional LINKs between the postsynapses take place during associative learning and memory retrieval respectively.
After the convergence of sensory inputs, activity is transmitted through different orders of neurons. The postsynapses of higher orders of neurons in the cortex may again converge at different locations. Concurrent with the induction of semblances, the EPSPs pass through the functional LINKs to the dendritic spines (postsynapses) (for example, postsynapse D in Figure 2) at the dendritic tree of the next neuronal order (for example, neuron N in Figure 2). Provided sufficient spatial and/or temporal summation of these EPSPs occurs, an action potential is triggered at the neuron’s (for example, neuron N in Figure 2) axon hillock. Axonal collaterals that ascend back and synapse to the excitatory neuron’s own soma are seen in dentate and CA3 neurons of the hippocampus (Lisman, 2005). In the cortex, such axonal collaterals ascend back and synapse to the excitatory neurons in more superficial layers and also synapse with neurons in the horizontal plane (Douglas and Martin, 2007). The role of these collaterals in the hippocampus and cortex where oscillatory neuronal activities are observed may be understood by the present study.
Other key points that need attention include the following. Since EPSPs elicited at the synapses far away from the cell body (example, apical synapses of cortical pyramidal cells) do not reach the soma (Stuart et al., 1997), they may not lead to the propagation of activity to the higher-order neurons. It was recently shown that nearby neurons with similar orientation-tuning showed no correlated activity, suggesting that adjacent neurons share only a small percentage of their inputs (Ecker et al., 2010), which indicates that the nearly 5 × 104 synaptic inputs to a neuron come from a very large number of penultimate neurons such that no single penultimate neuron has sufficient output connections to the next order of neuron to elicit an action potential. Changes, other than the spread of EPSPs, induced through the extracellular matrix between the postsynapses may evoke changes reminiscent of an action potential arriving at the presynapse inducing semblance (Vadakkan, 2011c).
Building of an Artificial Nervous System
The media in which an artificial nervous system can be built is beyond the scope of this article. One can envision tools using transistor gates or thin layer chemistry in developing an artificial nervous system.
Step1: Assign orders of neurons for different sensations. It is reasonable to start with five sensations. To begin with, assign finite number of neurons in each order. Assign postsynapses and presynaptic axonal terminals to each neuron. The postsynapses of the first-order neurons are the sensory receptors. A schematic representation of the model is given in Figure 6. Postsynapses of neurons from different sensations in the initial neuronal orders 1 and 2 are not placed in close proximity. Start placing 5% of postsynapses that belong to neurons of layers 3, 4, and 5, 30% of postsynapses that belong to neurons of layers 6 and 7 (a region that can be considered equivalent to the hippocampus), and 5% of postsynapses that belong to neurons of layers 7 and above in close proximity to each other. Then, assign recurrent collaterals in all the neurons in layer 6 such that reverberatory activity can provide temporal summation of EPSPs in the next order of neurons. Based on previous studies (Ecker et al., 2010), assign less than 40 presynaptic connections from a neuron in one neuronal order to another neuron in the next order (orders 8 or more represent the cortex).
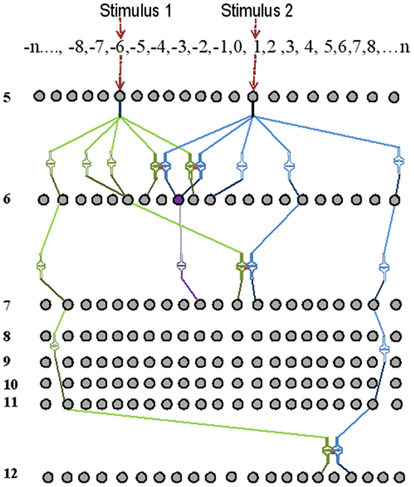
Figure 6. Schematic representation of the basic units of a memory storage device. Here, only neurons from neuronal order 5 and above are shown. Two independent stimuli are used for associative learning. The receptor numbers are shown in the top horizontal box. The intersections between lines of different intensities are synapses. During associative learning, functional LINKs are formed between the closely placed postsynapses (shown as pairs of closely placed synaptic shapes) of the neurons of the neuronal orders 6, 7, and 12. Spatial summation of nearly 40 EPSPs [from 40 synapses out of the nearly 50,000 synapses (0.0008%)] at the axonal hillock elicits an action potential. The neuron marked in violet receives sufficient EPSPs in the presence of the stimuli one and two to elicit an action potential. Note that the neuron in violet color was not activated by the stimulus 1 alone before learning.
Step 2: Trace the neuronal orders from the sensory receptors activated by the cue stimulus and the learned item to all the higher orders. Wherever their synapses are in close proximity with each other, mark them as potential inter-postsynaptic locations for functional LINK formation when they are activated together. If one postsynapse is LINKed to more than one postsynapse, provisions should be in place to form islets of functional LINKs (Figure 4). Initial computational work can be carried out by assuming that the functional LINKs are stable over time.
Step 3: Induce neuronal oscillations that are expected to occur in regions where a large number of neurons are organized similar to that in the hippocampus and cortex. Oscillations can be induced in neuronal orders 7 and 10 at the initial step of experimentation either artificially or by introducing sufficient number of structural LINKs (Vadakkan, 2011c) between the postsynapses in an attempt to induce neuronal oscillations from background sensory stimuli by adjusting the variables, as they are expected to be present in the newborns.
Step 4: Learning and retrieval of memories becomes more efficient in the adult nervous system after many years of associative learning. This indicates that functional LINKs formed in the early years of life are required for the efficient formation of memories in later years and that some of the previously formed functional LINKs within the islets of functional LINKs (Figure 4) are transferably used in new associative learning. Therefore, it is required to introduce many functional LINKs in a novice artificial machine through simple associative learning steps before achieving efficient memory capabilities.
Step 5: Induce associative learning using a cue stimulus and an item to be learned. Identify all the sensory receptors that they activate. This information is required to compare these sets of sensory receptors with the sensory receptor maps extrapolated to characterize the semblions representing retrievable memories.
Step 6: During memory retrieval, the cue stimulus will re-activate the functional LINKs that were formed during associative learning (Figure 7). This leads to the activation of the postsynaptic membranes (for examples, see D in Figure 1) inducing synaptic semblance. From the specific postsynapses where synaptic semblances occur, characterize the subsets of semblions (Figure 3). Based on the functional LINKs formed from initial associative learning events, we can inventory the semblions from each of the functionally LINKed postsynapses. By examining the integration of semblions occurring at different neuronal orders along with the time of synaptic delay at each neuronal order, the nature of the virtual sensory qualities of the memory for the learned item can be derived.
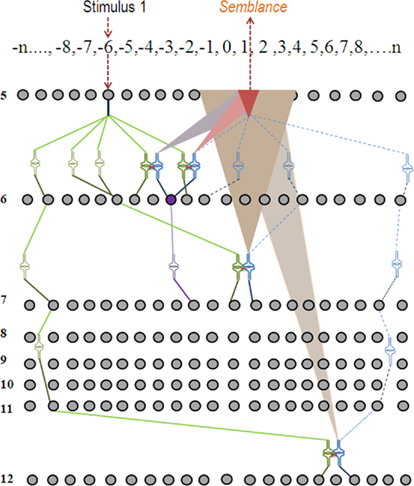
Figure 7. Schematic representation of semblance formation during memory retrieval. When stimulus one is introduced to the system after associative learning has taken place as shown in Figure 6, functional LINKs are re-activated providing semblance at the postsynaptic terminals at the opposite side of the functional LINKs. The synaptic semblances are shown as triangular shapes of different colors. The overlapped brick-colored smallest triangular shape represents the integration product of all the semblances (simplified and shown at the neuronal order 5). In summary, after learning, memory retrieval using the stimulus 1 alone can induce semblance of stimulus 2. Note that the neuron in violet color was not activated by the stimulus 1 alone before learning; however, it is activated by the stimulus 1 alone after associative learning with the stimulus 2.
Step 7: Find the matching algorithm that provides the integration rule for the subset of semblions from the list {ssn} that can match with the sensory qualities of the retrieved memories. Synaptic delay time needs to be used in the above integration process. Any time delay for the re-activation of the functional LINKs should also be considered. From the list of postsynapses that are likely to be activated during memory retrieval using a specific cue, potential semblions {ssn} are used in an integration process to obtain a net semblance that matches with the qualities of the learned item.
Step 8: Fine-tuning of the system is the last major step. Different variables in the above steps are either introduced step-by-step or simultaneously. Both computational and experimental approaches will be required to formulate a matching algorithm incorporating the number of neuronal orders, orders at which synapses converge for potential functional LINK formation during learning, number, and size of the islets of functional LINKs at different neuronal orders, functional LINKs already present from previous learning, combinations, and permutations (of the temporal activation of synapses) of the synaptic activities required for action potential generation, new neuron incorporation, addition, and deletion of new synapses, and the role of inhibitory neurotransmission and recurrent collaterals.
How many orders of neurons do the visual input needs to pass through before it induces sufficient number of functional LINKs to be used for efficient memory retrieval? From the requirement of 16 frames/s for perceiving a movie as a continuous event, it can be calculated that during the 62-ms time, the input stimuli from one frame passes through nearly 30 neuronal orders, given that upper limit of normal synaptic delay is 2 ms. Therefore, it is reasonable to begin assuming that the activity through nearly 30 synapses may induce sufficient semblances at their functionally LINKed postsynapses. In vivo studies examining the orientation plasticity of the visual cortex (Tanaka et al., 2009) may indirectly provide the extent and locations of functional LINK formation in a new nervous system. It may be anticipated that the net semblance may not have any similarity to the item whose memories are retrieved. Instead, certain constants may be involved. Studies using items with complementary features may help to resolve such issues.
Maintenance of Neuronal Oscillations
Neuronal oscillatory activities has been reported as essential for different type of memories (Winson, 1978; Dere and Zlomuzica, 2011). The oscillating neuronal activities trigger sub-threshold activities in many neurons and were discussed in the introduction section. Cue stimulus activates specific neurons on its pathway of activation. In addition, after associative learning using the same cue, specific additional neurons will be activated through the re-activation of learning-induced functional LINKs (Figure 8). The specific set of functional LINKs formed during associative learning during normal high-frequency (nearly 200 Hz) network oscillations in the hippocampus and spindle oscillations (7–14 Hz) in the cortex (Wilson and McNaughton, 1994; Buzsaki, 1996) can be specifically activated during wakeful state in the presence of similar oscillatory activity. Engineering oscillatory wave form activities at specific neuronal orders is an essential step in replicating the mechanism outside the nervous system. Even though gap junctions between the gamma amino butyric acid-releasing (GABAergic) interneurons participate in the generation of synchronization of oscillatory activities (Condorelli et al., 2000; Connors and Long, 2004), future studies may examine whether the lateral spread of activity through the functional LINKs contributes to oscillating neuronal activities.
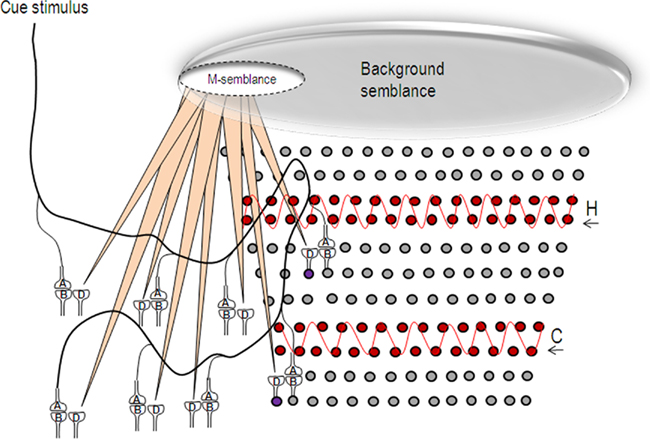
Figure 8. Schematic representation of the formation of semblance for memory (M-semblance) in the presence of background semblance. Oscillating neuronal activity (in red) both in the hippocampus (H) and cortex (C) induce a background semblance upon which memory semblance (M-semblance) is formed. On the left side, a cue stimulus induces synaptic semblances. The integral product of these semblances along with synaptic delay-time will form M-semblance for memory. On the right side, the circles represent the neurons. The neurons in red undergo oscillatory activities in the hippocampus and cortex. The semblances formed at the functional LINKs in their higher neuronal orders will get integrated to form the background semblance that can possibly contribute towards consciousness (C-semblance; Vadakkan, 2010). For simplicity, the projections of the semblances resulting from these oscillatory neuronal activities are not shown. The large elliptical shape at the top end of the figure represents the background semblance. Frequency changes of these oscillatory patterns, for example during altered conscious states, will change the dimensions of the background semblance and prevent the formation of M-semblance by the cue stimulus. The circles represent the neurons. H, hippocampus; C, cortex. The wave shapes in red show the oscillatory activities. The violet-colored neurons that normally receive sub-threshold stimulus from the oscillatory neuronal activity get activated by additional activity reaching through the functional LINKs in the presence of the cue stimulus.
The ability to access memories that makes the owner of a nervous system intelligent, is viewed as closely related to a conscious state (Minsky, 1991). Various findings that have suggested an association of some form of memories with consciousness (Tulving, 1985; Minsky, 1991; Edelman, 1992; Ramachandran and Hirstein, 1997; Crick and Koch, 1998; Rosenbaum et al., 2007) have motivated the derivation of a framework of consciousness from the basic units of semblances formed through the functional LINKs (Vadakkan, 2010). Accordingly, consciousness is viewed as net background semblance (C-semblance) formed from oscillating neuronal activity at the hippocampus and cortex that can provide the foundation on which specific semblance formation for the retrieval of specific memories can occur (Figure 8).
Read-Outs of Internal Sensations
Since it is possible to estimate the properties of the semblions of an artificial nervous system at a given time based on the presence of established functional LINKs, it may become possible to identify an optimal integration process of the specific set of semblions. More than one possible semblion formed at the postsynapses activated through the functional LINKs can be used in a matching process to discover the algorithm to obtain the best match with the learned item. Once the matching algorithm is available, additional computational processes can be employed to select and compute the semblions to provide an optimum virtual internal sensation for a given cue stimulus. Once established, it can provide appropriate read-outs of internal sensations induced by a cue stimulus.
Advantages of the Model
The nature of the semblance that mimics the learned item may be understood through computational and neuro-engineering methods by fine-tuning of various factors involved in the neuronal oscillations and the formation and re-activation of functional LINKs. The artificial nervous system is expected to operate using a finite number of sensory receptors and synapses, where specific memory retrieval will be possible through combinatorial re-activation of functional LINKs. Sharing of the functional LINKs that provide degeneracy to the memory code can also be explored. As related learning continues, the size of the islets of LINKed postsynapses increases, raising the requirement for specificity of the cue stimulus to achieve specificity of memory retrieval of previously learned items. Studies using complementary items can be used to explore the relationship between the learned item and the semblances; such studies are important, as it is possible that the net semblances may not have any similarities to the item whose memory is retrieved.
The formation of semblances during the re-activation of functional LINKs can substantiate retrieval at physiological time-scales, unlimited memory life-times (Fusi and Abbott, 2007; Abbott, 2008), fast learning (von der Malsburg, 1999), instant access to very large memory stores (Abbott, 2008), and the absence of overwriting of old memories by new ones (Fusi and Abbott, 2007; Abbott, 2008). It will be possible to test pattern instantiation, which is a fundamental capability of any intelligent system. Semblances formed at physiological time-scales will have sufficient characters to differentiate them from background noise (von der Malsburg, 1999). The formation of the same semblances from two different cues can enable the system to generate hypotheses (Abbott, 2008). Based on the suggestion that intelligence requires a degree of consciousness sufficient to enable the nervous system to access the stored memories (Minsky, 1991), it was possible to derive a framework for consciousness (Vadakkan, 2010). Constructing intelligent machines based on the principle of semblances may produce conscious machines as envisioned earlier (Minsky, 1991; Holland, 2003).
Since the variables and the final anticipated quality of the memorized item can be tested in experiments, the solution to the problem is expected to be within the reach of mathematical, computational, and engineering approaches. In developing AI, it is known that the number of possibilities that a problem-solving program has to examine (the combinatorial dimension) will be determined by the search space (Newell and Simon, 1972). In this context, the present work provides the elements present in a nervous system of a given complexity, sequences of operations to be executed by the program and the qualities with which the results need to be verified. The time-consuming part of the computational method is fine-tuning of multiple variables to match the net semblance with the qualities of the item whose memories are to be retrieved.
Disadvantages of the Model
The available experimental data are not sufficient to explain the required features of the functional LINKs as proposed by the present hypothesis. Spread of neurotransmitter to the neighboring synapses (Coggan et al., 2005; Fernandes et al., 2010), the AMPA receptor trafficking (Makino and Malinow, 2009), and ephaptic coupling (Anastassiou et al., 2011) cannot substantiate the specificity requirement for the proposed formation and re-activation of functional LINKs. This then necessitates examining their specific rules or novel mechanisms operating between the postsynapses. In addition, it is very difficult to verify the proposed changes between the postsynapses even though the cumulative effect can be explained from the results of earlier studies (Tye et al., 2008; Vadakkan, 2011c). No parallel mechanisms are known to exist in physical network systems that demonstrate internal sensations. Therefore, attempts to verify the present hypothesis need to obtain sufficient support from the present hypothesis alone. In addition, even if it leads to demonstration of the phenomenon, it may require very sophisticated experimental approaches to verify the nature of the semblions, units of internal sensations, within the nervous system.
Engineering Challenges
The engineering task involves setting up an electrical circuitry with junctions that can mimic synapses similar to previous studies (Rossier, 2009) and then provide sufficient provision for the formation of functional LINKs between the postsynapses within the circuitry as described before. Two key segments required are (1) A readout segment that provides information regarding the semblances formed when exposed to the cue stimulus after associative learning (Figure 9). (2) Capability to fine-tune the nature of the oscillations and recurrent collaterals to obtain semblances representing the learned item. In summary, we aim to build a prototype of a system with read-outs that allows testing for the formation of semblances by varying the parameters of oscillations and recurrent collaterals. Studies are required to test whether the lateral spread of activity through the functional LINKs in the system by itself can lead to the oscillatory neuronal activity.
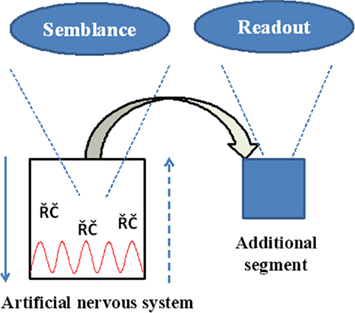
Figure 9. Schematic summary diagram of the engineering requirements. Downward arrow represents the normal neurotransmission. Upward broken arrow represents direction of semblance formation. RC: represents recurrent collaterals. Red wave form represents oscillatory neuronal activity. Replicating the semblances in physical systems comes with an opportunity as well as a challenge to configure building an additional unit for obtaining read-outs of the semblances.
Conclusion
The present work outlines a testable model to begin experiments in replicating higher cognitive functions that are internal sensations available only to the owner of the nervous system. Unlike computers, which have repetitive building units, memory storage proposed through the semblance hypothesis operates through combinatorial functional integration of its basic units. Increasing the number of associative learning events will increase the number of functional LINKs that can be used interchangeably for the formation of semblances during memory retrieval. Potential locations of the formation of functional LINKs between the postsynapses can be pre-determined from the very architecture of the nervous system, which can be modeled in an artificial system. This allows for predicting the memory capability of a given system.
At this time, it is anticipated that associative learning requires multiple exposures to different sensory stimuli. Once attempts to build these models begin, it may become possible to understand the rules for the addition of new functional LINKs to associate the expected sensory inputs from a given environment to improve memory efficiency even without associative learning. This may enable us to custom-make certain defined machines. Replicating the mechanism of operation in physical systems may make it possible to build intelligent machines with memory, the ability to hypothesize and the capability to demonstrate instantiation. When exposed to novel cues, these machines may be able to provide a set of probable net semblances that can be verified, enabling exploration of the nature. In addition, examining the internal states of the nervous system may decipher new physical properties pertaining to certain network systems. The present hypothesis should be treated as unproven until verified against experimental evidence.
Conflict of Interest Statement
The author declares that the research was conducted in the absence of any commercial or financial relationships that could be construed as a potential conflict of interest.
Acknowledgments
I acknowledge support from the Neurosearch Center in Toronto. I thank the reviewers for their constructive criticisms and comments.
References
Abeles, M. (1991). Corticonics: Neural Circuits of the Cerebral Cortex. Cambridge: Cambridge University Press.
Achler, T., and Amir, E. (1999). “Neuroscience and AI share the same elegant mathematical trap,” in Proceedings of 2009 Conference on Artificial General Intelligence, Arlington, VA, 198–199.
Anastassiou, C. A., Perin, R., Markram, H., and Koch, C. (2011). Ephaptic coupling of cortical neurons. Nat. Neurosci. 14, 217–223.
Bliss, T. V., and Collingridge, G. L. (1993). A synaptic model of memory: long-term potentiation in the hippocampus. Nature 361, 31–39.
Cheng, S., and Frank, L. M. (2008). New experiences enhance coordinated neural activity in the hippocampus. Neuron 57, 303–313.
Coggan, J. S., Bartol, T. M., Esquenazi, E., Stiles, J. R., Lamont, S., Martone, M. E., Berg, D. K., Ellisman, M. H., and Sejnowski, T. J. (2005). Evidence for ectopic neurotransmission at a neuronal synapse. Science 309, 446–451.
Condorelli, D. F., Belluardo, N., Trovato-Salinaro, A., and Mudo, G. (2000). Expression of Cx36 in mammalian neurons. Brain Res. Brain Res. Rev. 32, 72–85.
Connors, B. W., and Long, M. A. (2004). Electrical synapses in the mammalian brain. Annu. Rev. Neurosci. 27, 393–418.
Dere, E., and Zlomuzica, A. (2011). The role of gap junctions in the brain in health and disease. Neurosci. Biobehav. Rev. doi: 10.1016/j.neubiorev.2011.05.015. [Epub ahead of print].
Dierks, T., Linden, D. E., Jandl, M., Formisano, E., Goebel, R., Lanfermann, H., and Singer, W. (1999). Activation of Heschl’s gyrus during auditory hallucinations. Neuron 22, 615–621.
Douglas, R. J., and Martin, K. A. (2007). Recurrent neuronal circuits in the neocortex. Curr. Biol. 17, R496–R500.
Ecker, A. S., Berens, P., Keliris, G. A., Bethge, M., Logothetis, N. K., and Tolias, A. S. (2010). Decorrelated neuronal firing in cortical microcircuits. Science 327, 584–587.
Edelman, G. M. (1992). Bright Air, Brilliant Fire: On the Matter of the Mind. New York, NY: Basic Books.
Edelman, G. M., Reeke, G. N. Jr., Gall, W. E., Tononi, G., Williams, D., and Sporns, O. (1992). Synthetic neural modeling applied to a real-world artifact. Proc. Natl. Acad. Sci. U.S.A. 89, 7267–7271.
Favorov, O. V., and Kursun, O. (2011). Neocortical layer 4 as a pluripotent function linearizer. J. Neurophysiol. 105, 1342–1360.
Fernandes, C. C., Berg, D. K., and Gomez-Varela, D. (2010). Lateral mobility of nicotinic acetylcholine receptors on neurons is determined by receptor composition, local domain, and cell type. J. Neurosci. 30, 8841–8851.
Fodor, J. A., and Pylyshyn, Z. W. (1988). Connectionism and cognitive architecture: a critical analysis. Cognition 28, 3–71.
Fusi, S., and Abbott, L. F. (2007). Limits on the memory storage capacity of bounded synapses. Nat. Neurosci. 10, 485–493.
Gelbard-Sagiv, H., Mukamel, R., Harel, M., Malach, R., and Fried, I. (2008). Internally generated reactivation of single neurons in human hippocampus during free recall. Science 322, 96–101.
Heit, G., Smith, M. E., and Halgren, E. (1988). Neural encoding of individual words and faces by the human hippocampus and amygdala. Nature 333, 773–775.
Jensen, O., and Tesche, C. D. (2002). Frontal theta activity in humans increases with memory load in a working memory task. Eur. J. Neurosci. 15, 1395–1399.
Kepecs, A., Uchida, N., Zariwala, H. A., and Mainen, Z. F. (2008). Neural correlates, computation and behavioural impact of decision confidence. Nature 455, 227–231.
Kosslyn, S. M., Thompson, W. L., Kim, I. J., and Alpert, N. M. (1995). Topographical representations of mental images in primary visual cortex. Nature 378, 496–498.
Kraemer, D. J., Macrae, C. N., Green, A. E., and Kelley, W. M. (2005). Musical imagery: sound of silence activates auditory cortex. Nature 434, 158.
Kreiman, G., Koch, C., and Fried, I. (2000). Imagery neurons in the human brain. Nature 408, 357–361.
Lavigne, F., and Darmon, N. (2008). Dopaminergic neuromodulation of semantic priming in a cortical network model. Neurophysiologia 46, 3074–3087.
Legendy, C. (1970). “The brain and its information trapping device,” in Progress in Cybernetics, Vol. 1. ed. J. Rose (New York, NY: Gordon and Breach), 309–338.
Makino, H., and Malinow, R. (2009). AMPA receptor incorporation into synapses during LTP: the role of lateral movement and exocytosis. Neuron 64, 381–390.
Maren, S., and Quirk, G. J. (2004). Neuronal signalling of fear memory. Nat. Rev. Neurosci. 5, 844–852.
Minsky, M. (1991). “Machinery of consciousness,” in Proceedings, National Research Council of Canada, 75th Anniversary Symposium on Science in Society,Ottawa, ON.
Minsky, M. (1994). Book review of unified theories of cognition (Allen Newell) in Artificial intelligence in perspective. Cambridge, MA: The MIT press, 394.
Newell, A., and Simon, H. A. (1972). Human Problem Solving. Englewood Cliffs, NJ: Prentice Hall, 920.
O’Reilly, R. C. (2006). Biologically based computational models of high-level cognition. Science 314, 91–94.
Poirazi, P., and Mel, B. W. (2001). Impact of active dendrites and structural plasticity on the memory capacity of neural tissue. Neuron 29, 779–796.
Potter, S. M. (2007). “What can artificial intelligence get from neuroscience?” in Artificial Intelligence Festschrift: The Next 50 years, eds M. Lungarella, J. Bongard, and R. Pfeifer (Berlin: Springer-Verlag), 174–185.
Quirk, G. J., Repa, C., and LeDoux, J. E. (1995). Fear conditioning enhances short-latency auditory responses of lateral amygdala neurons: parallel recordings in the freely behaving rat. Neuron 15, 1029–1039.
Rachkovskij, D. A., and Kussul, E. M. (2001). Binding and normalization of binary sparse distributed representations by context-dependent thinning. Neural Comput. 13, 411–452.
Ramachandran, V. S., and Hirstein, W. (1997). Three laws of qualia: what neurology tells us about the biological functions of consciousness. J. Conscious. Stud. 4, 5–6, 429–457.
Rosenbaum, R. S., Stuss, D. T., Levine, B., and Tulving, E. (2007). Theory of mind is independent of episodic memory. Science 318, 1257.
Rosenblatt, F. (1961). Principles of Neurodynamics: Perceptions and the Theory of Brain Mechanisms. Washington, DC: Spartan Books.
Rossier, J. (2009). Wiring and plumbing in the brain. Front. Hum. Neurosci. 3:2. doi: 10.3389/neuro.09.002.2009
Rubin, D. D., and Fusi, S. (2007). Long memory lifetimes require complex synapses and limited sparseness. Front. Comput. Neurosci. 1:7. doi: 10.3389/neuro.10/007.2007
Stuart, G., Schiller, J., and Sakmann, B. (1997). Action potential initiation and propagation in rat neocortical pyramidal neurons. J. Physiol. (Lond.) 505, 617–632.
Sullivan, J. A. (2010). A role for representation in cognitive neurobiology. Philos. Sci. 77, 875–887.
Tanaka, S., Tani, T., Ribot, J., O’Hashi, K., and Imamura, K. (2009). A postnatal critical period for orientation plasticity in the cat visual cortex. PLoS ONE 4, e5380. doi: 10.1371/journal.pone.0005380
Tesche, C. D., and Karhu, J. (2000). Theta oscillations index human hippocampal activation during a working memory task. Proc. Natl. Acad. Sci. U.S.A. 97, 919–924.
Tommerdahl, M., Favorov, O. V., and Whitsel, B. L. (2010). Dynamic representations of the somatosensory cortex. Neurosci. Biobehav. Rev. 34, 160–170.
Toptsis, A. A. (2009). Reflective thinking, machine learning, and user authentication via artificial K-lines. Int. J. Adv. Sci. Technol. 5, 51–73.
Treisman, A. M., and Gelade, G. (1980). A feature-integration theory of attention. Cogn. Psychol. 12, 97–136.
Tsytsarev, V., Pope, D., Pumbo, E., Yablonskii, A., and Hofmann, M. (2010). Study of the cortical representation of whisker directional deflection using voltage-sensitive dye optical imaging. Neuroimage 53, 233–238.
Tye, K. M., Stuber, G. D., de Ridder, B., Bonci, A., and Janak, P. H. (2008). Rapid strengthening of thalamo-amygdala synapses mediates cue-reward learning. Nature 453, 1253–1257.
Vadakkan, K. I. (2010). Framework of consciousness from the semblance of activity at functionally LINKed postsynaptic membranes. Front. Psychol. 1:168. doi: 10.3389/fpsyg.2010.00168
Vadakkan, K. I. (2011a). A possible mechanism of transfer of memories from the hippocampus to the cortex. Med. Hypotheses 77, 234–243.
Vadakkan, K. I. (2011b). “Semblions induced through inter-postsynaptic functional LINKs, biological parallels of K-lines, as basic units of internal sensations,” in Society for Neuroscience Annual Meeting, Washington, DC.
Vadakkan, K. I. (2011c). Semblance Hypothesis of Memory, 3rd Edn. Bloomington: iUniverse Publishers.
von der Malsburg, C. (1981). The correlation theory of brain function. MPI Biophysical Chemistry, Internal Report 81–2, Göttingen. [Reprinted in Domany, E., van Hemmen, J. L., and Schulten, K. (eds). (1994). Models of Neural Networks II. Berlin: Springer].
von der Malsburg, C. (1986). “Am i thinking assemblies?” in Proceedings of the Trieste Meeting on Brain Theory, eds G. Palm, and A. Aertsen (Berlin: Springer), 1–26.
von der Malsburg, C. (1999). The what and why of binding: the modeler’s perspective. Neuron 24, 95–104.
Whitlock, J. R., Heynen, A. J., Shuler, M. G., and Bear, M. F. (2006). Learning induces long-term potentiation in the hippocampus. Science 313, 1093–1097.
Wilson, M. A., and McNaughton, B. L. (1994). Reactivation of hippocampal ensemble memories during sleep. Science 265, 676–679.
Keywords: memory, artificial intelligence, K-lines, semblance hypothesis, functional LINKs, semblions
Citation: Vadakkan KI (2011) Processing semblances induced through inter-postsynaptic functional LINKs, presumed biological parallels of K-lines proposed for building artificial intelligence. Front. Neuroeng. 4:8. doi: 10.3389/fneng.2011.00008
Received: 31 January 2011;
Paper pending published: 19 March 2011;
Accepted: 10 July 2011;
Published online: 27 July 2011.
Edited by:
Eshel Ben Jacob, Tel Aviv University, IsraelReviewed by:
Ronen Segev, Ben-Gurion University, IsraelVassiliy Tsytsarev, George Mason University, USA
Copyright: © 2011 Vadakkan. This is an open-access article subject to a non-exclusive license between the authors and Frontiers Media SA, which permits use, distribution and reproduction in other forums, provided the original authors and source are credited and other Frontiers conditions are complied with.
*Correspondence: Kunjumon I. Vadakkan, Division of Neurology, Department of Internal Medicine, University of Manitoba, GF532-820 Sherbrook Street, Winnipeg, MB, Canada R3A1R9. e-mail:dW12YWRha2tAY2MudW1hbml0b2JhLmNh