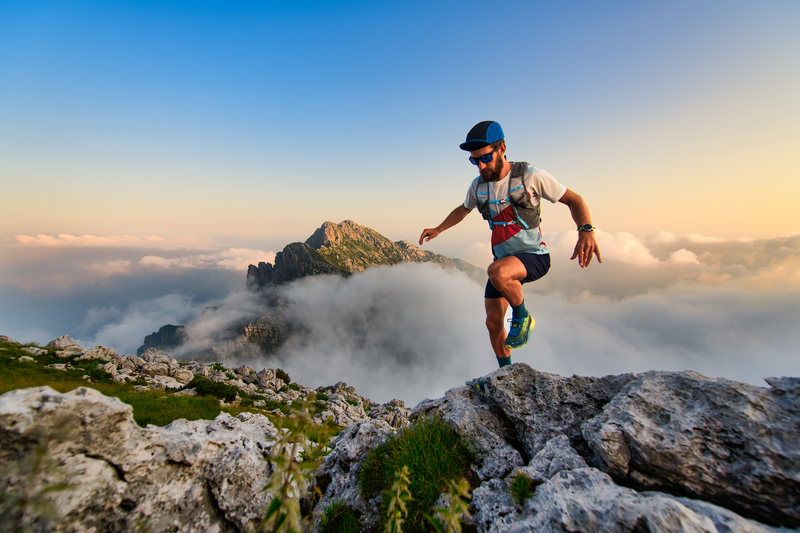
94% of researchers rate our articles as excellent or good
Learn more about the work of our research integrity team to safeguard the quality of each article we publish.
Find out more
ORIGINAL RESEARCH article
Front. Neuroanat.
Volume 19 - 2025 | doi: 10.3389/fnana.2025.1495735
This article is part of the Research Topic Imaging Brain Network and Brain Energy Metabolism Impairments in Brain Disorders View all 5 articles
The final, formatted version of the article will be published soon.
You have multiple emails registered with Frontiers:
Please enter your email address:
If you already have an account, please login
You don't have a Frontiers account ? You can register here
Resting-state networks (RSNs) have been used as biomarkers of brain diseases and cognitive performance. However, age-related changes in the RSNs of macaques, a representative animal model, are still not fully understood. In this study, we measured the RSNs in macaques aged 3-20 years and investigated the age-related changes from both functional and structural perspectives. The proportion of structural connectivity in the RSNs relative to the total fibers in the whole brain significantly decreased, whereas functional connectivity showed an increasing trend with age. Additionally, the amplitude of low-frequency fluctuations tended to increase with age, indicating that resting-state neural activity may be more active in the RSNs may increase with age. These results indicate that structural and functional alterations in typical RSNs are age-dependent and can be a marker of aging in the macaque's brain.
Keywords: macaque monkey, functional connectivity, structural connectivity, network, MRI
Received: 13 Sep 2024; Accepted: 19 Feb 2025.
Copyright: © 2025 Ouchi, Yamamoto, Obara, Sugase-Miyamoto and Tsurugizawa. This is an open-access article distributed under the terms of the Creative Commons Attribution License (CC BY). The use, distribution or reproduction in other forums is permitted, provided the original author(s) or licensor are credited and that the original publication in this journal is cited, in accordance with accepted academic practice. No use, distribution or reproduction is permitted which does not comply with these terms.
* Correspondence:
Tomokazu Tsurugizawa, National Institute of Advanced Industrial Science and Technology (AIST), Tsuluba, Japan
Disclaimer: All claims expressed in this article are solely those of the authors and do not necessarily represent those of their affiliated organizations, or those of the publisher, the editors and the reviewers. Any product that may be evaluated in this article or claim that may be made by its manufacturer is not guaranteed or endorsed by the publisher.
Research integrity at Frontiers
Learn more about the work of our research integrity team to safeguard the quality of each article we publish.