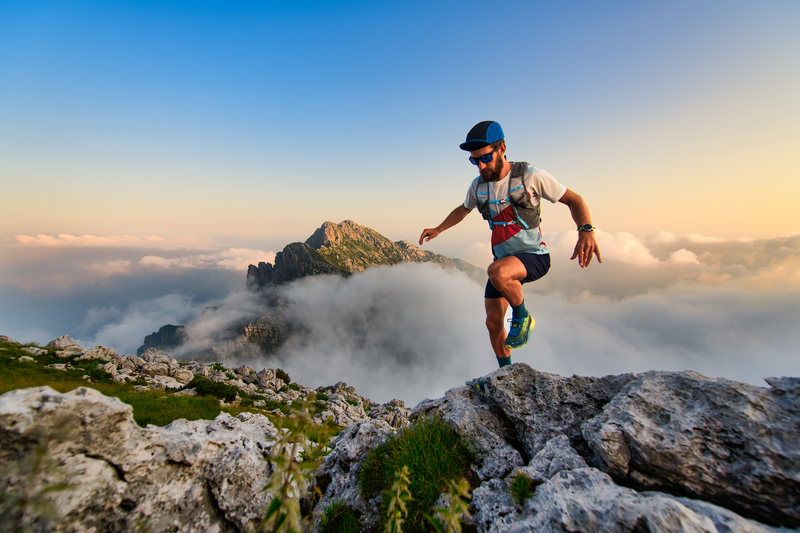
94% of researchers rate our articles as excellent or good
Learn more about the work of our research integrity team to safeguard the quality of each article we publish.
Find out more
ORIGINAL RESEARCH article
Front. Neural Circuits , 06 February 2018
Volume 12 - 2018 | https://doi.org/10.3389/fncir.2018.00003
This article is part of the Research Topic Neuromodulation of Circuits in Brain Health and Disease View all 35 articles
The basal ganglia are involved in the motivational and habitual control of motor and cognitive behaviors. Striatum, the largest basal ganglia input stage, integrates cortical and thalamic inputs in functionally segregated cortico-basal ganglia-thalamic loops, and in addition the basal ganglia output nuclei control targets in the brainstem. Striatal function depends on the balance between the direct pathway medium spiny neurons (D1-MSNs) that express D1 dopamine receptors and the indirect pathway MSNs that express D2 dopamine receptors. The striatal microstructure is also divided into striosomes and matrix compartments, based on the differential expression of several proteins. Dopaminergic afferents from the midbrain and local cholinergic interneurons play crucial roles for basal ganglia function, and striatal signaling via the striosomes in turn regulates the midbrain dopaminergic system directly and via the lateral habenula. Consequently, abnormal functions of the basal ganglia neuromodulatory system underlie many neurological and psychiatric disorders. Neuromodulation acts on multiple structural levels, ranging from the subcellular level to behavior, both in health and disease. For example, neuromodulation affects membrane excitability and controls synaptic plasticity and thus learning in the basal ganglia. However, it is not clear on what time scales these different effects are implemented. Phosphorylation of ion channels and the resulting membrane effects are typically studied over minutes while it has been shown that neuromodulation can affect behavior within a few hundred milliseconds. So how do these seemingly contradictory effects fit together? Here we first briefly review neuromodulation of the basal ganglia, with a focus on dopamine. We furthermore use biophysically detailed multi-compartmental models to integrate experimental data regarding dopaminergic effects on individual membrane conductances with the aim to explain the resulting cellular level dopaminergic effects. In particular we predict dopaminergic effects on Kv4.2 in D1-MSNs. Finally, we also explore dynamical aspects of the onset of neuromodulation effects in multi-scale computational models combining biochemical signaling cascades and multi-compartmental neuron models.
To survive and thrive it is crucial for all species to recognize and evaluate environmental cues, select actions and learn from previous behavioral outcomes (Redgrave et al., 1999). A brain area of specific importance in this task is the basal ganglia (BG), a set of interconnected nuclei, specialized in generating goal-directed and habitual motor- and cognitive behaviors.
The BG are centrally located in the forebrain and receive inputs from structures throughout the neuraxis, directly from different parts of the cortex and thalamus, or indirectly (via the thalamus) from many regions in the brainstem (Gerfen, 2004). The same brain structures (e.g., frontal cortex) that provide input to the BG also receive direct (via thalamus and brainstem structures) or indirect feedback from the BG output nuclei (Chevalier and Deniau, 1990). This architecture is often viewed as a series of partially segregated parallel projecting re-entrant loops involving both cortical and sub-cortical structures, controlling associated behaviors (Alexander et al., 1986; McHaffie et al., 2005). The moment to moment signaling through these BG loops are controlled by neuromodulation (see Hong, 2013), of which one of the most influential and studied neuromulatory system is dopamine (DA). Importantly, also learning/plasticity requires intact DA and acetylcholine (ACh) signaling (Knowlton et al., 1996; Matsumoto et al., 1999; Kitabatake et al., 2003).
As the largest input nucleus of the BG, the striatum plays a key role in regulating the information flow through these loops. The principal neuron of striatum is the medium spiny neuron (MSN) that can be further divided into two subpopulations based on DA type 1 (D1R) or DA type 2 (D2R) receptor expression. The two MSN subpopulations are classically thought to have opposing roles in the circuit. The D1R expressing MSN (D1-MSN) is in this model thought to facilitate the initiation of action/behavior via activation of the so called “direct pathway” of the BG, and this is enhanced when DA is increased (Gerfen and Surmeier, 2011). MSNs of this type directly inhibits the BG output stages (see Figure 1) which in turn inhibits downstream targets. Activation of these MSNs therefore leads to disinhibition of downstream targets. In addition to the direct pathway, there is the “indirect pathway,” starting with the D2R expressing MSN (D2-MSN), which inhibits actions/behaviors (Gerfen and Surmeier, 2011). This “classical” model of the role of the direct- and indirect pathways have in recent years been challenged (Cui et al., 2013; Tecuapetla et al., 2016) but there are also data in support of such functional generalization (Kreitzer and Berke, 2011; Kravitz et al., 2012).
Figure 1. A multi-scale view of basal ganglia. (Left) an illustration of the basal ganglia macro-circuitry, showing the glutamatergic and dopaminergic afferent inputs to striatum, as well as the projections via the direct and indirect pathways. Ca 50% of MSNs belong to the direct pathways, they carry dopamine type 1 receptors and project to GPi/SNr (D1-MSN). The other MSNs express dopamine type 2 receptors and project to GPe (globus pallidus externa) before reaching basal ganglia output structures (D2-MSN). At rest these output structure neurons keep motor programs in the brain stem and thalamus under tonic inhibition. When e.g., direct pathway GABAergic MSNs in the striatum become active they inhibit these tonically active neurons, and thereby the inhibition from basal ganglia onto the action/motor centers is relieved. This is the basis for the classical model of basal ganglia function in selection of (motor) actions. (Middle) a schematic representation of the striatal micro-circuitry. The main neuron type in the striatum is the medium spiny neuron (MSN), constituting ~95% of striatal neurons. MSNs are the projection neurons from the striatum and receive convergent excitatory glutamatergic input from cortex and thalamus, inhibitory GABAergic input from neighboring MSNs and striatal fast-spiking (FS) and low-threshold spiking (LTS) neurons, cholinergic (ACh) input from cholinergic interneurons (ChINs) and pedunculopontine nucleus (PPN), and dopaminergic input from substantia nigra compacta (SNc). (Right) Examples of receptor induces cascades involved in controlling LTP in the cortico-striatal synapse.
The effects of DA are widespread and affect the system via different receptor dependent mechanisms. Such “small scale effects” can only be understood as a part of the larger system. Because of that, and considering that this study is part of a special edition on neuromodulation, we will briefly highlight the interdependencies between the BG structures and the neuromodulatory systems to provide a wider perspective before we focus on specific aspects of the neuromodulation.
Modulatory effects of DA are found throughout the entire BG system, influencing neuronal excitability and synaptic efficacy across all of its nuclei (see Table 1). It is, however, not well understood to what extent the resulting system level effects are relying mainly on the alteration of membrane excitability and/or the modification of transmitter release and synaptic short-term-plasticity. Also in addition to the acute effects, secondary changes occur, such as synaptic plasticity and/or homeostasis. Such secondary changes play crucial roles in the development, progression and side effects of treatments to diseases, such as Parkinson's disease (PD) and drug addiction.
Table 1. Effect of dopamine on neuron excitability and synaptic connectivity in basal ganglia nuclei.
The dopaminergic modulation is relatively more well-studied in the striatum and its principal neurons than in other BG structures. The current view that dopaminergic innervation to MSNs is “excitatory” and “inhibitory” to the direct and indirect pathway, respectively (Gerfen and Surmeier, 2011), would predict that loss of dopamine results in an imbalance between the activities of these two pathways (Albin et al., 1989; Mallet et al., 2006; López-Huerta et al., 2013; Fieblinger et al., 2014).
Recent research highlights that not only an appropriate background DA level is needed for an appropriate functioning of the BG, but transient (phasic; over the order of 100 ms) regulation of the striatal DA levels is needed for ongoing fast-timescale motor control (Howe and Dombeck, 2016). In this case a fast regulation of the membrane excitability might take place via the activation of subcellular signaling cascades affecting various membrane conductances in selected neuronal populations in striatum.
A dynamic DA signal is also thought to drive reward learning by signaling unexpected reward (Schultz et al., 1997; Schultz, 2002). During reward learning long-term synaptic changes are assumed to take place in, for instance, the excitatory cortico-striatal synapses (Centonze et al., 2003; Calabresi et al., 2006; Wang et al., 2006; Wickens, 2009; Surmeier et al., 2011). The intracellular cAMP/PKA signaling (see Figure 1) is important for cortico-striatal LTP (Shen et al., 2008; Kheirbek et al., 2009; Castro et al., 2013). Additionally, dopamine dependent PKA signaling in D1 MSNs also creates an “eligibility trace-like” integration time window for associative learning (Yagishita et al., 2014), possibly via striatally enriched phosphoproteins (Nair et al., 2016).
At the systems level, the extensive research using animal models of PD have been useful to illustrate how the presence or absence of dopamine, affects network activity in behaving animals. In these PD models, death of DAergic neurons in substantia nigra pars compacta (SNc) is induced through application of toxins such as 6-OHDA (in rodents) and MPTP (in non-human primates). This results in major changes in the activity of the BG sub-networks. For instance, both in rodents and non-human primates, DA depletion leads to pathological beta band oscillations (15–30 Hz) of Subthalamic nucleus (STN)-Globus pallidus externa (GPe) populations (Hammond et al., 2007). These oscillations are accompanied by an increase in the firing rate of STN neurons, a corresponding decrease in the firing rate of GPe neurons, and increased synchrony between STN and GPe neurons (Raz et al., 2000; Mallet et al., 2008). Also, the ongoing firing rate of D2-MSNs is significantly increased (Mallet et al., 2006). Furthermore, dopamine depletion increases spike bursting in both STN and GPe (Tachibana et al., 2011; Nambu and Tachibana, 2014), affects the phase locking of GPe neuron activity to cortical slow-wave oscillations (Mallet et al., 2008) and increases correlations between the two principal types of neurons within GPe (Mallet et al., 2008, 2012).
Secondary and compensatory mechanisms come into play if the change in DA drive persists (Cadet et al., 1991; Przedborski et al., 1995; Courtiere et al., 2005; Capper-Loup and Kaelin-Lang, 2013; Golden et al., 2013). Thus, in animal models of DA depletion the acute effects are accompanied or followed by other alterations in the circuitry. In PD patients, in addition to the well-studied motor impairments, these patients also suffer from sensory impairments such as alterations of olfactory, tactile, nociceptive, thermal, and proprioceptive perception (Artieda et al., 1992; Sathian et al., 1997; Boecker et al., 1999; Conte et al., 2013), including impairments in bilateral tactile discrimination (Sathian et al., 1997; Zia et al., 2003). It was recently shown that DA depletion affects both the intrinsic properties of MSNs and sensory processing in the mouse striatum (Ketzef et al., 2017). MSNs in intact mice differ in their input resistance and excitability both in vivo and in vitro, with D2-MSN having larger values in both parameters (see also Maurice et al., 2015, in vitro findings, and Mallet et al., 2006 extracellular in vivo data). D1-MSNs normally separate responses to ipsi- and contralateral whisker stimulation better than D2-MSNs (Reig and Silberberg, 2014). When DA is depleted from the striatum, especially D1-MSNs lose this lateral encoding (Ketzef et al., 2017). Also, input resistance and excitability of the D1-MSN is increased following DA depletion, reducing the difference between the two types of MSN observed under control conditions (Maurice et al., 2015; Ketzef et al., 2017; but see also studies in rat Tseng et al., 2001; Mallet et al., 2006). These types of findings point toward compensatory mechanisms playing a role, in such a way that acute blockades of DA receptors can have opposite effects compared to chronic alterations.
In addition to DA, ACh is likewise crucial for BG function. It has been assumed since the early 1960s that ACh and DA counterbalance one another, such that an increase in ACh is associated with a decrease in DAergic effects in the striatum (Barbeau, 1962; McGeer et al., 1974). Thus, anti-cholinergic drugs were often prescribed to treat Parkinson's Disease (Duvoisin, 1967). Since then, treatment has focused on increasing dopamine levels through administration of L-DOPA. However, newer work suggests that specific muscarinic receptors (M1, M4) could be a potential target for more specific treatments, reducing negative side-effects (Xiang et al., 2012; Ztaou et al., 2016). Yet another example of the importance of the balance between DA and ACh relates to the use of recreational drugs, such as cocaine and morphine, that lead to elevated DA levels in the ventral striatum (Hikida et al., 2003). As ACh and DA are believed to serve antagonistic roles, increasing cholinergic tone through acetylcholinesterase inhibitors can suppress addictive symptoms (Staley et al., 2006). Maintaining a proper cholinergic tone in the BG then appears crucial in preventing addiction.
The cellular bases of the observed modulatory effects of ACh on the striatal network is starting to be understood (reviewed by Oldenburg and Ding, 2011, etc.). Subcellular effects of the interaction between ACh and DA are also relatively well understood. Interestingly, cholinergic interneurons (ChINs), which normally are tonically active, may show a pause in their activity when DA is transiently elevated during reward learning (Aosaki et al., 1994, 1995, 2010; Morris et al., 2004). The mechanism responsible for the pause is at least partly mediated by activation of D2 receptors on the ChINs, as described by Gerfen and Surmeier (2011). Computational modeling has further shown that such coordinated DA and ACh interaction could set up a time window for the required activation of LTP-dependent subcellular signaling, e.g., PKA, in striatal D1-MSNs (see Figure 1; Nair et al., 2015). On the other hand, in the indirect pathways neurons, a DA dip (as seen during omitted but expected rewards or following aversive stimuli) is hypothesized to gate adenosine dependent PKA signaling, which controls LTP in these neurons (Shen et al., 2008; Yu et al., 2009; Nair et al., 2015; Yapo et al., 2017). These effects of co-occurring ACh/DA (in D1-MSNs) and DA/adenosine-dependent plasticity (in D2-MSNs) could be further modulated by other neuromodulators such as the serotonergic system representing additional aspects of the emotional state of the organism (Tanaka et al., 2007; Seo et al., 2008). As for DA, sub-second ACh burst-pause dynamics might also be important for controlling the membrane excitability during ongoing behavior.
Thus, there is ample experimental evidence that ACh and DA can regulate synaptic plasticity and cellular excitability. This can mechanistically take place through joint regulations of the same receptor activated pathways. Given the importance of an adequate balance and timing between the activation of the different neuromodulators it might not come as a surprise that these same neuro-modulatory systems are also directly influencing and controlling each other. Threlfell et al. (2012) further highlighted these dynamic interdependencies when they reported that synchronous activity of ChINs was capable of driving DA release through local axo-axonal collaterals. Striatal ChINs could thus dictate the local dopaminergic tone, regardless of the activity of the midbrain neurons responsible for these collaterals. Moreover, it was recently shown that putative DA axons co-release GABA and glutamate when activated (Tritsch et al., 2012), suggesting that activation of DA axons mediates various effects by activation of different receptors with different time courses (Straub et al., 2014). Work by Nelson et al. (2014) showed that such GABA co-release can also be initiated by synchronized activity of ChINs, thus forming a negative feedback loop.
More subtle effects have also been observed: ChINs exhibit strong feed-forward inhibitory connections onto neighboring ChINs as shown by Sullivan et al. (2008), presumably mediated by GABAergic neurons. This recurrent lateral inhibition is well suited to synchronize ChINs to a degree necessary to elicit DA release. While the nature of the intermediate neuron is not yet known, it too appears to be modulated by DA, possibly through a D2 mediated inhibition of presynaptic terminals via N-type Calcium Channels, as demonstrated by Momiyama and Nishijo (2017). Taken together, these interactions point toward a spatio-temporal coordination of recurrent control between the cholinergic and dopaminergic systems.
Not only do the DA and ACh systems control the BG circuitry and more specifically each other in a dynamic manner, but in similar ways as the BG output nuclei control (motor-)targets in the brainstem (see Figure 1) signaling through the BG directly regulates the activity of midbrain dopaminergic- as well as brain stem cholinergic nuclei. The striatum is divided into a matrisome and striosome compartment (Graybiel, 1984; Crittenden et al., 2016). Whereas the matrisome compartment is involved in the control of motion via the direct and indirect pathways, the striosome compartment is engaged in controlling the level of activity in the DA neurons. The MSNs of the striosomes project directly to the DA neurons and thus provide inhibition, but they also project to a subpopulation of glutamatergic neurons in globus pallidus (GP) that in turn projects to the lateral habenula (LH). The LH projects preferentially to a GABAergic nucleus that in turn inhibits the DA neurons (Stephenson-Jones et al., 2012, 2013, 2016). Aversive stimuli lead to an enhanced activity in habenula-projecting globus pallidus (GPh), and the LH (Matsumoto and Hikosaka, 2007; Hong and Hikosaka, 2008; Stephenson-Jones et al., 2016) and to a net inhibition of the DA activity, whereas reward type stimuli causes a decrease of the GPh/LH and a disinhibition of the DA neurons (i.e., an increase). It appears that the striosomes thus are engaged in regulating the activity level of DA neurons directly and via the GPh/LH link. It hence appears as if the striosomes and related circuitry are engaged in the evaluation of the result of different motor tasks, which clearly is a very important aspect in terms of motor learning, not least in the reinforcement perspective (Stephenson-Jones et al., 2013, 2016; Grillner and Robertson, 2016).
Experimental data from the systems-, cellular-, and subcellular levels are all possible to align with e.g., the general views that the dopaminergic innervation can control learning in the BG and the moment to moment balance between the direct- and indirect pathways, but the quantitative dependencies between neuromodulation and changes in the BG function is not well understood, but rather fragments of experimental findings are distributed over many different published studies with little attempts to synthesize the knowledge in a quantitative manner. Hence, to support our understanding and allow formation of testable and more quantitative predictions, models spanning the subcellular—microcircuit—systems level are useful tools.
To create hypotheses how effects of neuromodulation on membrane excitability and synaptic properties are linked to BG systems level function (for instance “action selection”) and/or BG network dynamics, theory- and simulation based research is needed to drive understanding. BG modeling faces the challenge that quantitative constraints on effective connectivity between different BG sub-nuclei is not available and often has to be indirectly estimated (e.g., Bahuguna et al., 2017). Despite this challenge there has been attempts to integrate most of the DA dependent modifications from Table 1 in large-scale computational models of the BG system, see for example (Lindahl and Hellgren Kotaleski, 2016). Besides full models of the Cortico-BG loop, models of striatum (e.g., Humphries et al., 2009; Ponzi and Wickens, 2010; Yim et al., 2011; Tomkins et al., 2013; Bahuguna et al., 2015; Berthet et al., 2016; Belić et al., 2017; Spreizer et al., 2017) and STN-GPe network (e.g., Humphries et al., 2006; Leblois et al., 2006; Kumar et al., 2011; Pavlides et al., 2015; Corbit et al., 2016; Schwab et al., 2017), have been widely used in predicting underlying mechanisms for how various functional or dynamical phenomena described above may arise.
Here we address the question: how the wide spread effects of DA on single channels or receptors can drive the changes in excitability seen in in vitro experimentally (typically mapping to “static” effects) and during ongoing behavior in vivo (also considering “dynamic” effects). Specifically, we address the question whether the experimentally reported intracellular mechanisms are fast enough to give rise to the observed behavior, which seem to take place in the sub-second time scale (Howe and Dombeck, 2016). Furthermore, we highlight the importance of quantitative measurements of the dynamics in the subcellular signaling. The method we use is multiscale modeling, spanning the cellular-subcellular levels, which gives us access to (and full control over) the state of the cell. As a modeling system we use the D1-MSN of the direct pathway, including an intracellular receptor induced cascade connecting transient DA release to single channel conductances.
In this study a biophysically detailed compartmentalized model is used, which gives us complete control over the state of the cell. In such models, a cell is divided into a finite number of “compartments.” Each compartment is an electrical circuit that is set to represent the electrical properties of a small part of the neuron. A few compartments are for example used to model the behavior of the axon initial segment and the soma while multiple compartments are used for the dendritic tree. The compartments of the model are sequentially connected, providing a representation of the electrical circuit of the whole cell. Current flow over the membrane is modeled using passive, ion concentration dependent, and voltage dependent ion channels, inserted non-uniformly into the compartments. Current flow between compartments are proportional to the voltage difference between neighboring compartments. External currents are given in the form of current injections and synaptic currents. The current injections are modeled as constant currents given to one of the somatic compartments. Synaptic currents are placed in the dendritic compartments and modeled with amplitudes given by time dependent exponential functions. DA modulation of ion channels and synaptic currents (“mechanisms”) are modeled using a fixed or time varying change of mechanism conductance. The time varying modulation is implemented by connecting the mechanism conductance to the concentration of intracellular substrates, dynamically changed when dopamine binds to receptors in the cell membrane. A more detailed description of various aspects of the model is given below.
The D1-MSN model used in this project is derived from a generic MSN model previously used in our group (Du et al., 2017). The morphology of the model is based on a digitally reconstructed MSN (Figure 2A) and the behavior of the model was tuned to fit experimental data from D1-MSN in the form of rheobase current, current-frequency curve (Planert et al., 2013) and calcium response to backpropagating action potential (Day et al., 2008) (Figures 2B–D). Kinetics of ion channels were increased using Q-factors whenever possible to map experimental behavior in room temperature to physiological temperatures (about 35°C). The setup of the model has been described in detail before (Evans et al., 2012; Paille et al., 2013; Du et al., 2017). Here we will therefore give a general overview with focus on updates introduced in the current version.
Figure 2. Validation. The model is validated against experimental data from striatonigral medium spiny neurons of the direct basal ganglia pathway. Both somatic (B and C) as well as dendritic excitability (D) is validated. In (A), the dendritic arborisation of the morphology is shown. (B) is showing the voltage response of the cell, following current injections ranging from −100 to 340 pA in steps of 40 pA. In (C) the current-frequency curve of the model is plotted together with experimental curves from Planert et al. (2013). In (D) the dendritic excitability is validated as the local change in calcium concentration as a function of somatic distance following a backpropagating action potential (Day et al., 2008).
The model was built in NEURON+Python (v7.4, Hines and Carnevale, 1997). Simulations were performed on standard laptops with the Linux operating system Ubuntu 16.04 and on resources provided by the Swedish National Infrastructure for Computing (SNIC) at PDC KTH. The model is uploaded to modelDB.
Morphological reconstruction of the principal cell from dorsolateral striatum of the mouse was used for the MSN model (Cazorla et al., 2012; neuromorpho.org, archive Kellendonk, ID NMO_08390). Spatial and structural data was supplemented with distribution of dendritic diameters from another set of reconstructions (Martone et al., 2003; neuromorpho.org, archive Martone, ID NMO_04518 through NMO_04523) where it was specifically addressed. At any given node, the dendritic diameter was assigned a value, in micrometers,
where L is the total dendritic length of the branch rooted at the given node, dp is the diameter of the parent node (in the dendrite or soma for the first node of the trunk), and l is the distance to the parent node. Four additional nodes were added to the morphology, two somatic and two axonal. The length and diameter of the soma were 12.2 and 11.2 μm, respectively, while the axonal initial segment had a total length of 60 μm and a diameter of 1 μm. The morphology is shown in Figure 2A.
The passive properties of the model were uniformly set to values within accepted range. The specific axial resistance, Ra, was decreased with this in mind from 400 Ohm/cm (Du et al., 2017) to 150 Ohm/cm. A change that brought it into the range of most commonly reported values (70–220) over multiple brain areas (Bekkers and Stevens, 1996; Stuart and Spruston, 1998; Roth and Hausser, 2001; Golding et al., 2005). The specific capacitance Cm, was set to 1 μF/cm2. Leak conductance was set to 1.25e-5 S/cm2 and reversal potential of leak to −70 mV. Reversal potential of Potassium and Sodium were set to −85 (Podda et al., 2010) and 50 mV (Wolf et al., 2005), respectively.
The Sodium and Potassium channels of the model came from the last published version of the model (Du et al., 2017) with some minor updates. The inward rectifying Potassium channel (KIR) was updated with smooth kinetics instead of tabulated values. The inactivation time constant of the fast A-type Potassium channel (Kaf) was decreased to consistently simulate physiological temperatures (the Q-factor was applied to both gates instead of just one of them). The kinetics of the transient fast inactivating sodium channel (Naf) was slowed down at depolarized potentials. This smoothed and decreased the amplitude of the afterhyperpolarization (AHP) and decreased the slope of the FI curve. The Q-factor of the Naf channel was also reduced from 2 to 1.8 to further slowdown the spiking frequency while staying within the experimentally reported range (Schwarz, 1986).
The Ca channels and dynamics were updated from the original version of the model (Wolf et al., 2005). The blocker resistant Ca (CaR) channel was updated with the activation curve fitted to m3 kinetics (Evans et al., 2013) and upscaled activation time constant, accordingly. The inactivation time constant was implemented using smooth fit to experimental data (Brevi et al., 2001). The long-lasting Ca (CaL) channel was implemented in two versions, CaL1.2 and CaL1.3. The kinetics of these channels followed mh and m2h, respectively. The activation time constant of both channels were also refitted to avoid singularities. The neuronal, N-type Ca (CaN) channel followed m2h kinetics (Kasai and Neher, 1992), where activation of m2 was fitted to experimental data (Bargas et al., 1994; Evans et al., 2012). The activation time constant was scaled up as well (Kasai and Neher, 1992). The transient opening T-type Ca (CaT) channel was also implemented in two versions, CaT3.2 and CaT3.3. Both versions followed m3h kinetics (Crunelli et al., 2005; Evans et al., 2013) with upscaled activation kinetics accordingly. The Ca dependent small conductance Potassium (SK) channel was implemented with smooth activation of Ca dependence (Evans et al., 2013).
Uniform channel distribution was used unless experimental data suggested different and/or other distributions gave a better fit to experimental data. The distribution of the Kaf channel in the dendrites was set to increase with increased somatic distance while the slowly inactivating Potassium (Kas) channel and the Naf channels were set using the reversed distribution. These non-uniform distributions were used in agreement with experimental data suggesting that Kaf regulates dendritic excitability while Kas and Naf regulates somatic (Day et al., 2008). A similar increase in dendritc Kaf distribution has also been directly demonstrated in the larger pyramidal cells of hippocampus where direct patching of dendrites is possible (Hoffman et al., 1997). The Kas and the Naf channels were also distributed to the spike initiation zone in the axonal initial segment. The rest of the channels followed the same distribution as the earlier versions of the model. Maximal conductance/permeability and channel distribution of all channels are given in Table 2.
Synaptic noise was modeled by placing one randomly activated glutamatergic and GABAergic synapse, respectively, in each section of the cell. Each synapse in the model hence represents many synapses in the real cell. Mean activation frequency and maximal conductance of the noise were tuned to make the cell fluctuate around −70 mV as has been reported in vivo (Reig and Silberberg, 2014). The mean activation frequency of glutamatergic synapses was 17 Hz while GABAergic synapses were activated using 4 Hz, giving a ratio of glutamate to GABA of about 4 to 1 (Wilson, 2007). Maximal conductance of the synapses was set to 150 and 450 pS for glutamate and GABA, respectively. NMDA to AMPA ratio of the glutamatergic synapse was set to 1. The synaptic receptors themselves were modeled using double exponential mechanisms with short term depression (STD, Tsodyks et al., 2000). STD were modeled using a time constant of 100 ms while the other time constants of the receptors followed Wolf et al. (2005). The NMDA part of the excitatory synaptic current was also filtered by a voltage dependent magnesium block identical to the one used in Du et al. (2017).
Dopamine triggers intracellular signaling events in striatal MSNs, via neuron-type specific DA receptors. This could result in the phosphorylation of various ion channels, thereby affecting the electrical properties of the neuron. Some aspects regarding this have been for the D1-MSNs implemented in NEURON by coupling intracellular signaling with the state variables controlling the electrical properties. The basic building blocks for the intracellular signaling are biochemical reactions, such as binding and enzymatic reactions. Individual reactions were mathematically modeled as differential equations dictated by mass action kinetics. The reactions for DA-dependent intracellular signaling were taken from an existing experimentally-constrained model (Nair et al., 2016). Briefly, the signaling network contains reactions for the activation of D1R by DA which in turn activates the G-protein associated with the receptor. The active G-protein binds to adenylyl cyclase (AC) thereby increasing the enzymatic activity of AC. This increases the cAMP production rate. The network also includes phosphodiesterases responsible for the degradation of cAMP. cAMP activates the main kinase downstream, PKA. PKA could phosphorylate various ion channels. To capture this effect of PKA on its substrate, we included a test substrate which could be efficiently phosphorylated by PKA and dephosphorylated by PP1. The phosphorylation level of this sample substrate is used as a proxy for the extent of PKA-dependent phosphorylation of a given ion channel in the following sections. Even though this approach is simplistic and does not consider channel-specific reaction parameters, it could illustrate the intracellular signaling-triggered transition between various operational states of the neuron.
The dopamine-dependent intracellular signaling was implemented using the standardized markup language, Systems Biology Markup Language (SBML) from where it was converted into a NEURON readable mod file. This conversion was done in two steps using NeuroML (Cannon et al., 2014). First the SBML file was exported to the standard NeuroML input format, LEMS, and from that format it was then exported to the mod format. To increase the readability of the mod file, the substrate ID's were mapped and exchanged for their respective names in the original SBML file. This was done using a custom-made python script. The script read the mod file, line by line, extracting ID's of parameters etc, then searched the SBML file for the corresponding tag and finally exchanged ID for name. To avoid errors due to long variable names the longest names were also reduced to shorter versions using the same script (e.g., rate__revreaction_12 was reduced to r_r_12). The commands used for the conversion were:
1. ./jnml -sbml-import SBML.xlm 1 1
2. ./jnml SBML.xlm_LEMS.xml -neuron
3. python sbml_neuroML_mod_parameterCleaner2.py <mod file from 2> <SBML from 1> <py file from 2>
An application for converting SBML to NEURON, embedding it in a neuron model, and running the code in NEURON from the HBP collaboratory, is currently under construction (https://collab.humanbrainproject.eu). This application uses the NeuroML conversion tool (https://www.neuroml.org/neuron_tools) to create a mechanism.mod file. This mod file can then be inserted within a neuron model. The interface is written as an iPython Jupyter notebook and stimulation and plotting of simulation variables is performed from within the notebook.
DA modulation was implemented in the model in two ways. First a static modulation was used to investigate how the contribution of individual modulated channels can lead to increased excitability of the D1-MSN (Flores-Barrera et al., 2011; Planert et al., 2013). Here the maximal conductance of individual channels was multiplied by a modulation factor (MF) that stayed constant throughout the simulation. The range of individual MFs were obtained using a thorough literature study, revising and extending the work done by Moyer et al. (2007). Individual simulations could fall in one of three categories based on excitability compared to control; Less, Equal, or More excitable. Rheobase current was here used as the readout of excitability. To increase the number of simulations in the Equal group, changes smaller than 10 pA compared to control was categorized as equal excitable. A current step of 10 pA corresponded to about 2 mV change in membrane potential close to the spike threshold. This is also within the interval of glutamate uncaging triggered post synaptic potentials used experimentally (Plotkin et al., 2011). This part of the study was designed to simulate an in vitro like situation with no ongoing synaptic drive.
Secondly a dynamic modulation was implemented in which the conductance of individual channels was dynamically coupled to substrates of the cascade. In this setup we used random synaptic activation to drive the cell. It was hence designed to simulate an in vivo like situation. This second technique was primarily used to investigate timing aspects of the DA modulation.
Since the uncertainty underlying channel modulation is large we assessed excitability changes using large sets of randomly combined MFs, extending outside of the reported range. In these simulations one MF per channel were uniformly drawn from the interval 0-2 (with 1 representing zero modulation) and multiplied onto the conductance of the channel. The range of modulation thus corresponds to ±100%. About 170,000 simulations were run in this way. Additional simulations were run in which individual factors were restricted to the range reported in the literature. Around 8,000 simulations were run in each restricted set.
Spikes were extracted from the voltage traces by checking where the latter crossed zero mV. This was done by extracting the index of all positive data point from the potential trace, creating small islands in the membrane potential land scape. Since each island represents one spike, one index per island (the first) were chosen and the time points were extracted from the time trace.
The standard, Pearson correlation coefficient was used to calculate correlations. Specifically, this was done using the corr function of the Pandas library in the Python programming language. Plotting of correlation was done using Hinton diagram, with the magnitude of the correlation visualized as the size of a square. The color (orange or black) of the square reported positive or negative correlation, respectively.
Experimental data were extracted from published articles using an online extraction tool (Rohatgi, 2016).
DA modulation affects multiple levels and time scales as summarized in the Introduction. Multiple conductances are also affected at the single D1-MSN level. We therefore started by investigating these effects using a literature review. The review of literature revealed that the Naf, Kas, and CaN/P channels are down-regulated by DA (about 20–40, 15–35, and 20–50, respectively); the KIR and the GABA channels have been reported to be both up- and down-regulated (ranging about −20% to +25% and −20% to +40%, respectively); the Kaf channel is reported not to be modulated in striatum, although there is some indirect evidence in support of modulation, see Discussion; and finally the CaL, AMPA, and NMDA channels are all up regulated (at least 20, 0–40, and 20–60%, respectively). The intrinsic and synaptic single channel effects of DA are summarized in Tables 3 and 4, respectively.
Table 4. Summary of the literature study on synaptic effect of D1R activation given as percentage of control.
In this study we specifically investigated how single channel effects could be combined to increase the overall excitability of the D1-MSNs (Flores-Barrera et al., 2011; Planert et al., 2013). To this end we created a large set of simulations where the MF of each ionic conductance was randomly drawn from a sample distribution (See method). This Monte Carlo based approach was used since a systematic variation was not feasible given the possible number of MF combinations. As described in the Methods section, the simulations were then grouped into categories based on their respective rheobase current. All simulations that needed at least 10 pA less or more current to make the cell spike, were grouped into the More or Less excitable categories, respectively, the rest were categorized as Equal excitable (Figure 3A). The proportions of random simulations that fell into each category were then assessed from the full sample set of random simulations. Of all the simulations we considered 36% more excitable, 62% less excitable the rest were considered equal excitable (Figure 3B).
Figure 3. Distribution of modulation factors and overall model excitability. The overall excitability of the uniformly distributed sample set is compared to the unmodulated control case and the contribution of different ion channels to the change in excitability is assessed. In (A), the general setup of the simulation is illustrated. First a random modulation factor (MF) is drawn for each modulated channel and the conductances are scaled accordingly; then the excitability (in the form or rheobase current) of the new setup is compared to the unmodulated control case and is sorted into groups (More, Equal, and Less). A trial that needs at least 10 pA more or less current to spike is sorted into the Less (blue) and More (red) groups, respectively, the rest are categorized as Equal excitable (gray). In (B), the proportions of simulations in each group is shown. In (C), the MF distribution over groups is shown. In (D), the correlation of each channel with excitability is shown as a Hilton diagram. The size of the square is here indicating the magnitude of the correlation while the color of the square shows if the correlation is positive (orange) or negative (black). In (E), the channel-channel correlations as well as pair vice scatter plots are shown for the potassium and sodium channels. In (F), information of all modulated potassium and sodium channels are shown as a function of excitability group. The x and y axes, the color and the size of the dots here shows the modulation factors of the Kaf, Naf, Kir, and Kas channels, respectively.
To investigate which channels that had the strongest influence on cell excitability, we next plotted MF distribution as well as pairwise channel-excitability and channel-channel correlations over the three groups (More, Equal, and Less excitable). The distribution of the MFs and the channel-excitability correlations showed that the potassium and sodium channels had the biggest impact on cell excitability, while the Ca channels had little influence (Figures 3C and D). For this reason, the Ca channels were excluded from the rest of the analysis. The channel-channel correlation further showed that the Kaf-Naf channel pair was positively correlated over all three excitability groups, while the Kaf-Kir channel pair was strongly negatively correlated (Equal and More excitable groups, Figure 3E, upper row). The scatter plot of these channel pairs, further showed that while the Less excitable group were generally more uniformly distributed, the Equal and More excitable groups were clearly confined to specific regions. As expected, most simulations with either increase in both the Kir and Kaf channels, or increase in the Kaf and decrease in Naf channel, gave very few More excitable simulations (Figure 3E, lower rows). By adding information from the Kir and Kas channel to the Kaf-Naf scatter plot we could see that More and Equal excitable simulations with relatively large Kaf MFs in general had low MFs of the Kir and Kas channels (Figure 3F). A strong Kaf current can hence be compensated for, to some extent, by a decrease in the other potassium carrying channels (primarily Kir).
When restricting the sample set to only include MFs within the reported range (see Table 3), with no modulation of the Kaf channel, all simulations fell within the Less excitable category (Figure 4A). When also allowing modulation of the Kaf channel (80 ± 5%) it decreased the proportion of Less excitable simulations to 64%. But the proportion of More excitable simulations were still low (23%, Figure 4B). Since the full sample set showed that the Naf and the Kir channels were also influential in increasing the excitability of the cell, we next extended the range of modulation allowed for these channels, one at a time. The upper range of the Naf channel MF extended from 80 to 100% of control (no modulation) while the lower range of the Kir channel MF was decreased from 85 to 0% (zero conductance). Both changes resulted in a reduction in the proportion of Less excitable simulations, but only with a few percent. The proportion of Less excitable simulations was still 90% under both conditions, with only a few percent in the other two groups (Figures 4D and E). Allowing a larger down-regulation of the Kas channel (lower limit from 65 to 0%) also showed only minor effect (Figure 4F). To conclude this section, a down-regulation of the sodium channel by DA in D1-MSN, leads to decreased excitability that to some extent can be compensated for by decreased potassium currents. Primarily the Kaf and the Kir channels are influential in this compensation.
Figure 4. Static modulation. In (A), the modulation factors (MF) are restricted to the experimentally reported range (see Table 3) except for the Ca channels that are kept as free parameters since they did not contribute much to the excitability (see Figures 3C and D). In the top panel the distribution of each channel is shown split over subgroup (Less, Equal, or More excitable). The middle panel shows spike rasters. All black dots here represent spikes from modulated traces while the large gray stripes show the timing of spikes from the unmodulated control case. The bottom panel shows the proportion of the samples of each category. The same type of plots is shown in (B and C) but here the underlying sample sets have been restricted in different ways. In both plots the Kaf channel is also modulated 80 ± 5% and in (C) the axon initial segment is additionally not modulated. In (D), the Kir channel is allowed to reduce more than reported in the literature (down to zero conductance). In (E), the reduction of the fast sodium channel is allowed to reduce less than experimentally reported (up to no modulation). In (F), the slow potassium channel (Kas) is allowed to reduce down to zero conductance. In (G), the same modulation is used as in (A), with the difference that the axon initial segment is not modulated.
The Naf current in our model is a compound current which is carried by several different members of the sodium channel family. These channels are preferentially distributed to different compartments of the cell. The members that are located in the soma and proximal dendrites are more easily phosphorylated by PKA than the ones in the axon initial segment (Maurice et al., 2001; Hu et al., 2009). Hence, it is possible that different compartments are differently modulated. To test this hypothesis, we performed additional simulations in which the axon initial segment was not modulated by DA, i.e., no channels in the axonal compartments (Naf and Kas) were modulated by DA. To our surprise this did not increase the proportion of simulations that were more excitable following DA modulation (100% were still Less excitable, Figure 4G). If this effect was combined with a decreased Kaf channel, on the other hand, we saw a large increase in excitability (65% were More excitable and only about 15% were Less excitable, Figure 4C).
To conclude this section, if the spike initiation zone in the axon initial segment is not modulated and the Kaf channel is modulated, a large number of MF combinations, within reported range, will make the cell more excitable.
Since the Kaf channel primarily regulates the dendritic excitability (Day et al., 2008), it is perhaps not that surprising that experimental studies using dissociated cells, fail to find DAergic effects on this channel (Kitai and Surmeier, 1993; Dong and White, 2003). More surprisingly, direct blocking of the Kaf channel, but not local dendritic application of DA, increased the fluorescent signal following a bAP (Day et al., 2008). It was however proposed by the authors that the most likely explanation to their negative finding was that this modulation is not effectively assayed by a single bAP. We therefore asked if DA modulation of the Kaf channel can induce changed excitability while not affecting the bAP? To test this, we repeated the simulation of the bAP, while blocking the Kaf channel partially (to mimic the DA effect) and fully (to mimic the blockage by 4-AP), and compared with the results in Day et al. (2008). The result of this simulation showed that a full block of the channel gave a substantially increased signal while a partial block, in the range of the assumed DA induced modulation (20%), hardly gave any increase at all (Figure 5). Our simulations thus confirms the hypothesis in Day et al. (2008), and strengthen our prediction that increased excitability of D1-MSNs, following dopamine administration, is Kaf channel dependent.
Figure 5. Kaf channel control of calcium influx following a backpropagating action potential. In (A), an illustration of the simulation setup is shown. An action potential is triggered by giving a strong depolarizing current pulse (2 nA, 2 ms) to the soma, and the calcium (Ca) concentration is monitored as a function of somatic distance. Similar to florescent imaging. This setup is repeated for different proportions of Kaf channel blocking; 100, 50, 20, and 0% (control). In (B), example traces of concentration are shown for either proximal (30–50 μm), or distal (170–200 μm) dendritic compartments. The upper panel shows all groups of blocking proportions and the lower panel only holds control and the assumed proportion blocked by dopamine (20%). In (C), the mean change in amplitude of the Ca concentration, is plotted, as a function of somatic distance. The curves are normalized to the proximal location, mimicking experimental data (brown trace, Day et al., 2008).
In the previous sections we used an in vitro like setup where there was no ongoing synaptic activity. Next we asked how modulation of channels in the reported range would affect an in vivo like situation where synaptic input would drive the cell. Using the simulation design described in the Methods section, with channels and receptors dynamically modulated by PKA from an intracellular cascade, we created a large set of simulations using random MFs. The range for the factors of different channels were restricted to the ones tested statically in the in vitro like situation. The ranges for the AMPA, NMDA and GABA receptors were set to the reported ranges, summarized in Table 4. Since the model was tuned to fluctuate around −70 mV in control condition, it did not spike. For this reason we had only two groups in the analysis, spiking and non-spiking. If the cell spiked within 1 s from the onset of the DA cascade it was categorized as spiking and if it did not, it was categorized as non-spiking. The simulations were further divided into different modulation paradigms. In the first paradigm only AMPA and NMDA channels were modulated (Excitatory), the second also included GABA modulation (Synaptic), the third held only non-receptor gated ion channels (Intrinsic), the fourth held intrinsic and excitatory (No GABA) and in the final all channels were modulated (PKA).
The results showed that the excitatory or the intrinsic groups alone triggered spiking in 5 and 0% of the cases, respectively. Combining the two on the other hand, increased the spiking proportion to 33% (No GABA, Figure 6D). If the sodium channels are not modulated in the subsecond time scale the proportion spiking is further increased to 55% (no Naf, Figure 6D). Modulating GABA as well had little or no effect since the spiking proportion stayed constant (compare excitatory with synaptic and PKA with the no GABA group in Figure 6D). We further saw that the synergy of combining synaptic and intrinsic modulation was gone if the Kaf channel was not modulated (no Kaf, Figure 6D). The proportion of spiking simulations was increased when longer duration of the modulation was allowed (up to 1,500 ms, see PKA 1.5 s, Figure 6D).
Figure 6. Dynamic modulation. The timing aspects of the DA modulation is assessed by connecting the channel conductances to a DA transient. The transient follows an alpha function with 0.5 μM amplitude and a time constant of 500 ms. In (A), the simulation setup is outlined, showing a simplified illustration of the intracellular cascade. The model is here driven using synaptic activation as in an in vivo like situation. In (B), the time to first spike is quantified as a function of modulating substrate (bottom panel). The median value for each substrate is given above respective box. In the top panel the underlying kinetics of the modulating substrates are shown. In the middle panel a few examples of voltage traces are shown. Both modulated (black) and unmodulated control traces (gray) are shown. In (C), different modulation paradigms are used; Intrinsic means that only intrinsic ion channels are modulated, Excitatory means that only AMPA and NMDA channels are modulated (for range see Table 4), Synaptic means that the GABA channels as well as the AMPA and NMDA channels are modulated, No GABA means that all channels but the GABA channels are modulated, and in the final group (PKA) all channels are modulated. In (D) the proportion of traces that spikes are shown as a function of modulating substrate and modulation paradigm (all connected to PKA). Additionally to the ones shown in (C), there are also the groups No Naf, No Kaf, “No Kaf, Naf,” and PKA 1.5 s. In the first three groups the simulation is run without modulation of the respective channel(s) mentioned in the title. In the last group the simulation time is extended from 1 to 1.5 s.
Hence, our simulations show that the combined effects of DA modulation on excitatory synaptic channels and intrinsic ion channels are needed to cause spiking in D1-MSN. We further predict that the mechanism causing the synergy depends on down-regulation of the Kaf channel.
Next, we tested how activation of DAergic terminals within striatum can trigger movement within a few hundred milliseconds (Howe and Dombeck, 2016) when channel effects are usually measured over minutes (Planert et al., 2013). For this reason, we coupled various substrates from the intracellular cascade previously mentioned (see Methods), to the channels (see Figure 6A for an illustration), and quantified the time to first spike for each of them (Figure 6B, lower panel, and Figure 6C). For a detailed description on how the coupling was done see Methods. In short, the substrate concentration was set to influence the maximal conductance of the modulated channels. Three of the substrates tested; PKA, cAMP, and Gβγ, have all been proposed as mechanisms of DA modulation for at least some channel (see Table 5). The last one, direct D1R-channel interaction was further tested, despite low credibility, since it would speed up modulation. The reason why we at all considered this is that D1Rs have been shown to co-localize with CaN channels in the membrane of cortical pyramidal neurons (Kisilevsky et al., 2008). However, it is important to note that DA modulation in this study was still G-protein dependent and that the authors failed to find such an organization in striatum.
In this simulation we used modulation of all channels since we showed earlier that this gave the highest proportion of spiking and our main focus in this study was to investigate kinetics of the modulation. We still quantified the proportions of spiking simulations since the different non-linear kinetics of ion channels and substrates might cause unforeseen dynamical effects.
The median first spike resulting from the assumed PKA stimulation was triggered close to the upper limit of 1,000 ms used in the simulation. This delay makes it much too slow to cause the observed behavior. cAMP was slightly faster, about 890 ms. Direct Gβγ interaction decreased the time to first spike to a median of 650 ms while channel-D1R complexes triggered spiking in about 270 ms.
To conclude this section, PKA can in our model trigger spiking with a latency of about 1 s from the onset of DA activation. Other signaling substrates in the intracellular network, closer to the upstream receptor, can trigger spiking faster, but only direct D1R-channel complexes are, in our model, fast enough to explain action initiation within a few hundred milliseconds. Since such connections have not been found within striatum, either the model does not faithfully reproduce the underlying physiological processes (see section Discussion), or modulation of channels and receptors is not the primary mechanism of fast DAergic action initiation. Either way these results highlight that we need to learn more about the kinetics and spatial organization of substrates within neurons under neuromodulation to explain subsecond neuromodulatory effects (see Chuhma et al., 2017).
In this study we briefly reviewed the role of DA in the BG. In an attempt to investigate more specifically how DA brings about major changes in the sub-nuclei of the BG, we here focused on the DAergic effect on D1-MSNs. The choice of D1-MSNs was motivated by the facts that (1) the D1-MSNs are the starting point of the so called direct-pathways (Gerfen, 2004), which is important in action selection (Bahuguna et al., 2015; Lindahl and Hellgren Kotaleski, 2016) (2) D1-MSNs have been extensively studied so much data have been reported that can be incorporated into the model.
We have further shown how computational techniques can be used to integrate the result from different studies to make predictions where experiments are indecisive. Here we exemplified this by investigating specific aspects of DA modulation on the striatal D1-MSN, but similar studies could and perhaps should be conducted for all cell types in the BG. As a starting point for such studies we provide an overview table showing the widespread effect of dopamine modulation in the BG (Table 1). New techniques in the transcriptomics and imaging fields should further be used to provide additional information on the subcellular substrates involved in the modulation over cell types and nuclei, e.g., fluorescent in situ RNA sequencing (Lee et al., 2014).
In this study we found that down-regulation of the Kaf channel was necessary to increase the excitability of the D1-MSN. At first, this result seems to contradict experimental studies, unable to find effects of DA on the fast potassium current (Kitai and Surmeier, 1993; Dong and White, 2003; Day et al., 2008). The first two studies however, are conducted in dissociated cells where most of the dendrites are lost, and the Kaf channel, on the other hand, is most influential in the dendrites (Day et al., 2008). In the third study, Day et al. (2008), further detected no significant change in bAP amplitude following puffing of DA in the dendrites, while blocking of the Kaf channel had an effect. They however hypothesized that the experimental setup was not suited to catch such a change. This is also in accordance with our simulations showing that a small decrease (20%) of Kaf conductance can increase excitability while the bAP amplitude is not much affected (see Figure 5C).
The Kaf channel in the model is a composite channel primarily carried by the Kv4.2 channel (Tkatch et al., 2000) and there is indirect evidence in support of modulation of the Kv4.2 channel. If the channel has formed a complex with an ancillary subunit, the potassium Channel Interacting Protein 3 (KChiP3), it can be down regulated by PKA (Schrader et al., 2002) and KChiP3 is expressed in striatum (Xiong et al., 2004).
The Kv4.2 channel can further bind to A-kinase anchoring proteins (AKAPs) known to enable fast reliable PKA signaling (Lin et al., 2010). Disruption of this anchoring mechanism decreases excitability of pyramidal cells in hippocampus (Lin et al., 2011), providing a link between PKA phosphorylation of the Kv4.2 channel and excitability. Another possibility is that the lack of direct evidence in support of DA modulation of the channel indicates a high degree of phosphorylation under control conditions. This would be consistent with the decreased excitability following disruption of the anchoring mechanism. In such a scenario we would expect to find that other neuromodulators, acting to decrease PKA signaling, would increase the Kaf current and thereby decrease the excitability of the cell.
Phosphorylation, e.g., by PKA has also been shown to decrease the Kaf current in hippocampal pyramidal neurons, by shifting the voltage dependence of the activation gate into more depolarized potentials (Hoffman and Johnston, 1998).
Regarding the Naf channel, DA modulation causes an enhancement of the slow inactivation (SI) mechanism and thereby a decreased current (Carr et al., 2003; Chen et al., 2006). SI is driven by the collapse of the channel pore (Chen et al., 2006; Payandeh et al., 2012), and develops over seconds (Rudy, 1978). The process is hence too slow to be involved in the observed behavior (Howe and Dombeck, 2016) but will likely affect excitability in slice experiments. The experimental setup will further affect the degree of modulation. The SI is not only voltage dependent (Cantrell et al., 1999) but is also affected by other post-translational modifications, such as PKC phosphorylation and methylation level, both of which also modulates sodium currents (Chen et al., 2006; Baek et al., 2014). Given the complexity of small scale effects, it is therefore clear that DA modulation cannot be fully understood alone, but only as a part of the bigger puzzle.
DA modulation of Ca channels had a low influence on excitability in our model. This indicates that either is the base expression level higher than in the model or these channels have primarily other functions. Many intracellular cascades are regulated by Ca, so it is possible that these channels are mostly involved in plasticity and learning. For example, L-type channels are not necessary for LTP induction in striatal slice preparations (Calabresi et al., 2000), or dopamine induced enhancement of NMDA currents in dissociated MSNs (Flores-Hernandez et al., 2002), but is needed for LTD (Paille et al., 2013). The NMDA channel is a prominent source of Ca itself, so increasing this current, due to DA modulation, might facilitate LTP. Some of the Ca channels are also directly coupled to the Ca-dependent potassium channels, and the blocking of these channels have been shown to affect excitability (Hopf et al., 2010).
We here found that if the axon initial segment was not modulated it gave a largely increased proportion of factor combinations that made the cell more excitable. Experimentally there are at least two pieces of evidence in support of such an organization. Firstly, as mentioned in the Result section, some channels that are preferentially distributed to the axon initial segment are less easily phosphorylated by DA (Maurice et al., 2001; Chen et al., 2008; Hu et al., 2009). And secondly the DAergic connection to MSNs is primarily dendritic (Pickel et al., 1981; Freund et al., 1984).
There are also questions regarding the kinetics of the modulation. In our simulations, the only substrate that could trigger a subsecond behavior was direct receptor-channel coupling. Such interaction has not been reported in striatum. To the contrary, the study reporting the connection in prefrontal cortex, failed to find such connection in striatum (Kisilevsky et al., 2008). This leaves a few possible explanations, (1) that the cascade we used is too slow, despite being speeded up to explain the timing dependency in reward learning within striatum (Nair et al., 2016), (2) the channels are more sensitive to substrate change than modeled, (3) the “resting state” of the membrane potential in vivo is closer to the spike threshold than what is used in the model, the cell will thereby respond faster to increased excitability, and/or (4) the activation of DAergic terminals activates fast, excitatory ionotropic receptors to a higher degree than previously reported in dorsal striatum (see below and review by Chuhma et al., 2017).
Specific anchoring proteins, e.g., AKAPs, form large signaling complexes by direct (Gao et al., 1997; Westphal et al., 1999), as well as indirect (Colledge et al., 2000) binding to channels. Disruption of these links cause abolished modulation by DA (Rosenmund et al., 1994; Few et al., 2007) as well as impaired learning (Carlisle et al., 2008; Nithianantharajah et al., 2012). In addition to such functional compartmentalization, neuronal geometry could also play a role into how signals are integrated by intracellular molecular mechanisms (Neves et al., 2008). The signaling model that is currently used, does not take the functional compartmentalization and geometrical aspects explicitly. Rather, the reaction parameters have been selected to capture the overall characteristics of the downstream responses as observed in measurements (Nair et al., 2016).
Co-release of several neurotransmitters from the same presynaptic neurons has been shown in various synaptic pathways in the brain, often involving combinations of “fast” neurotransmitters with slower “neuromodulators” (Yu et al., 2015; also see review by Tritsch et al., 2016). In the striatum, dopaminergic terminals originating from the midbrain (VTA and SNc) were shown to co-release glutamate onto MSNs in the nucleus accumbens (Chuhma et al., 2004; Tecuapetla et al., 2010). Recently, it was shown that dopaminergic terminals in striatum also co-release GABA (Tritsch et al., 2012). While there are still many open questions regarding co-release, e.g., the topographical arrangement, the target selectivity, dynamics, and reuptake mechanisms, it is clear that both types of MSNs and ChINs receive GABA input from dopaminergic neurons as studied in DAT-Cre mice. In a recent study, marked differences in the co-release of dopamine with GABA or glutamate were shown between the nucleus accumbens core and shell regions and dorsal striatum (Chuhma et al., 2014). While inhibitory responses were seen in the dorsal striatum, neurons in the medial shell region of nucleus accumbens responded with ePSPs mediated by glutamate (Chuhma et al., 2014). The strength of ePSPs depended on the postsynaptic striatal cell types, with ChINs responding stronger than MSNs and FS interneurons. These finding demonstrate diverse effects of the midbrain dopamine system onto different striatal regions and neuron types, and further suggest complex interactions between the dopaminergic and cholinergic systems in the striatum.
Based on the predictions of the model that the fast potassium channel must be modulated by DA to explain the increased excitability of D1-MSNs, we propose that staining is used to elucidate if Kv4.2-KChIP3 complexes are indeed formed within striatum. Another member of the KChIP family (KChIP1) shows a high specificity and can be used as a marker for the striosomal compartment in striatum (Mikula et al., 2009). This indicates that potassium channels of the striosome and matrix compartments are differently modulated by neuromodulators, such as DA. It would therefore be interesting to see if other Kv4-KChIP complexes are formed, and if so how this affects channel currents and modulation. In addition, our results when it comes to subcellular signaling suggest that co-transmission of glutamate, or some other fast, non PKA-dependent mechanism is triggered by activation of DAergic terminals in dorsal striatum. Further research on the intracellular cascades triggered by dopamine, as well as network effects following activation of DAergic terminals is needed in order to elucidate this mechanism.
All authors contributed actively in collecting information regarding dopamine effects. AN contributed the subcellular cascade model. DK and RL exported the cascade into Neuron and prepared the setup at the HBP Collaboratory. RL, AKK, KD constructed the MSN model. RL performed the simulations and analyzed the data. RL and JH planned the study. All authors contributed to the writing.
The research leading to these results has received funding from the European Union Seventh Framework Programme (FP7/2007-2013) under grant agreement n°604102 (Human Brain Project); The European Horizon2020 Framework Programme under grant agreement n°720270 (Human Brain Project SGA1); The Swedish Research Council; NIAAA (grant 2R01AA016022); Swedish e-Science Research Center; EuroSPIN – an Erasmus Mundus Joint Doctoral program.
The handling Editor declared a shared affiliation, though no other collaboration, with one of the authors DK.
The authors declare that the research was conducted in the absence of any commercial or financial relationships that could be construed as a potential conflict of interest.
The simulations were performed on resources provided by the Swedish National Infrastructure for Computing (SNIC) at PDC KTH.
Albin, R. L., Young, A. B., and Penney, J. B. (1989). The functional anatomy of basal ganglia disorders. Trends Neurosci. 12, 366–375. doi: 10.1016/0166-2236(89)90074-X
Alexander, G. E., DeLong, M. R., and Strick, P. L. (1986). Parallel organization of functionally segregated circuits linking basal ganglia and cortex. Ann. Rev. Neurosci. 9, 357–381. doi: 10.1146/annurev.ne.09.030186.002041
Aosaki, T., Kimura, M., and Graybiel, A. M. (1995). Temporal and spatial characteristics of tonically active neurons of the primate's striatum. J. Neurophysiol. 73, 1234–1252. doi: 10.1152/jn.1995.73.3.1234
Aosaki, T., Kiuchi, K., and Kawaguchi, Y. (1998). Dopamine D1-like receptor activation excites rat striatal large aspiny neurons in vitro. J. Neurosci. 18, 5180–5190.
Aosaki, T., Miura, M., Suzuki, T., Nishimura, K., and Masuda, M. (2010). Acetylcholine-dopamine balance hypothesis in the striatum: an update. Geriatr. Gerontol. Int. 10, S148–S157. doi: 10.1111/j.1447-0594.2010.00588.x
Aosaki, T., Tsubokawa, H., Ishida, A., Watanabe, K., Graybiel, A. M., and Kimura, M. (1994). Responses of tonically active neurons in the primate's striatum undergo systematic changes during behavioral sensorimotor conditioning. J. Neurosci. 14, 3969–3984.
Artieda, J., Pastor, M. A., Lacruz, F., and Obeso, J. A. (1992). Temporal discrimination is abnormal in Parkinson's disease. Brain 115 (Pt 1), 199–210. doi: 10.1093/brain/115.1.199
Baek, J. H., Rubinstein, M., Scheuer, T., and Trimmer, J. S. (2014). Reciprocal changes in phosphorylation and methylation of mammalian brain sodium channels in response to seizures. J. Biol. Chem. 289, 15363–15373. doi: 10.1074/jbc.M114.562785
Bahuguna, J., Aertsen, A., and Kumar, A. (2015). Existence and Control of Go/No-Go Decision Transition Threshold in the Striatum. PLOS Comput. Biol. 11:p.e1004233. doi: 10.1371/journal.pcbi.1004233
Bahuguna, J., Tetzlaff, T., Kumar, A., Hellgren Kotaleski, J., and Morrison, A. (2017). Homologous basal ganglia network models in physiological and parkinsonian conditions. Front. Comput. Neurosci. 11:79. doi: 10.3389/fncom.2017.00079
Banke, T. G., Bowie, D., Lee, H., Huganir, R. L., Schousboe, A., and Traynelis, S. F. (2000). Control of GluR1 AMPA receptor function by cAMP-dependent protein kinase. J Neurosci. 20, 89–102.
Barbeau, A. (1962). The pathogenesis of Parkinson's disease: a new hypothesis. Can. Med. Assoc. J. 87, 802–807.
Bargas, J., Howe, A., Eberwine, J., Cao, Y., and Surmeier, D. J. (1994). Cellular and molecular characterization of Ca2+ currents in acutely isolated, adult rat neostriatal neurons. J. Neurosci. 14(11 Pt 1), 6667–6686.
Baufreton, J., and Bevan, M. D. (2008). D2-like dopamine receptor-mediated modulation of activity-dependent plasticity at GABAergic synapses in the subthalamic nucleus. J. Physiol. 586, 2121–2142. doi: 10.1113/jphysiol.2008.151118
Beckstead, M. J., Grandy, D. K., Wickman, K., and Williams, J. T. (2004). Vesicular dopamine release elicits an inhibitory postsynaptic current in midbrain dopamine neurons. Neuron 42, 939–946. doi: 10.1016/j.neuron.2004.05.019
Bekkers, J. M., and Stevens, C. F. (1996). Cable properties of cultured hippocampal neurons determined from sucrose-evoked miniature EPSCs. J. Neurophysiol. 75, 1250–1255. doi: 10.1152/jn.1996.75.3.1250
Belić, J. J., Kumar, A., and Hellgren Kotaleski, J. (2017). Interplay between periodic stimulation and GABAergic inhibition in striatal network oscillations. PLOS ONE 12:e0175135. doi: 10.1371/journal.pone.0175135
Berthet, P., Lindahl, M., Tully, P. J., Hellgren-Kotaleski, J., and Lansner, A. (2016). Functional relevance of different basal ganglia pathways investigated in a spiking model with reward dependent plasticity. Front. Neural Circuits 10:53. doi: 10.3389/fncir.2016.00053
Boecker, H., Ceballos-Baumann, A., Bartenstein, P., Weindl, A., Siebner, H. R., Fassbender, T., et al. (1999). Sensory processing in Parkinson's and Huntington's disease: investigations with 3D H(2)(15)O-PET. Brain 122 (Pt 9), 1651–1665. doi: 10.1093/brain/122.9.1651
Bracci, E., Centonze, D., Bernardi, G., and Calabresi, P. (2002). Dopamine excites fast-spiking interneurons in the striatum. J. Neurophysiol. 87, 2190–2194. doi: 10.1152/jn.00754.2001
Brevi, S., de Curtis, M., and Magistretti, J. (2001). Pharmacological and biophysical characterization of voltage-gated calcium currents in the endopiriform nucleus of the guinea pig. J. Neurophysiol. 85, 2076–2087. doi: 10.1152/jn.2001.85.5.2076
Cadet, J. L., Last, R., Kostic, V., Przedborski, S., and Jackson-Lewis, V. (1991). Long-term behavioral and biochemical effects of 6-hydroxydopamine injections in rat caudate-putamen. Brain Res. Bull. 26, 707–713. doi: 10.1016/0361-9230(91)90164-F
Calabresi, P., Gubellini, P., Centonze, D., Picconi, B., Bernardi, G., Chergui, K., et al. (2000). Dopamine and cAMP-regulated phosprotein 32 kDa controls both striatal long-term depression and long-term potentiation, opposing forms of synaptic plasticity. J. Neurosci. 20, 8443–8451.
Calabresi, P., Picconi, B., Parnetti, L., and Di Filippo, M. (2006). A convergent model for cognitive dysfunctions in Parkinson's disease: the critical dopamine-acetylcholine synaptic balance. Lancet Neurol. 5, 974–983. doi: 10.1016/S1474-4422(06)70600-7
Cannon, R. C., Gleeson, P., Crook, S., Ganapathy, G., Marin, B., Piasini, E., et al. (2014). LEMS: a language for expressing complex biological models in concise and hierarchical form and its use in underpinning NeuroML 2. Front. Neuroinformatics 8:79. doi: 10.3389/fninf.2014.00079
Cantrell, A. R., Scheuer, T., and Catterall, W. A. (1999). Voltage-dependent neuromodulation of Na+ channels by D1-like dopamine receptors in rat hippocampal Neurons 19, 5301–5310.
Capper-Loup, C., and Kaelin-Lang, A. (2013). Locomotor velocity and striatal adaptive gene expression changes of the direct and indirect pathways in Parkinsonian rats. J. Parkinsons Dis. 3, 341–349. doi: 10.3233/JPD-130202
Carlisle, H. J., Fink, A. E., Grant, S. G., and O'Dell, T. J. (2008). Opposing effects of PSD-93 and PSD-95 on long-term potentiation and spike timing-dependent plasticity. J. Physiol. 586, 5885–5900. doi: 10.1113/jphysiol.2008.163469
Carr, D. B., Day, M., Cantrell, A. R, Held, J., Scheuer, T., Catterall, et al. (2003). Transmitter modulation of slow, activity-dependent alterations in sodium channel availability endows neurons with a novel form of cellular plasticity. Neuron, 39, 793–806. doi: 10.1016/S0896-6273(03)00531-2
Castro, L. R., Brito, M., Guiot, E., Polito, M., Korn, C. W., Hervé, D., et al. (2013). Striatal neurones have a specific ability to respond to phasic dopamine release. J. Physiol. 591, 3197–3214. doi: 10.1113/jphysiol.2013.252197
Castro, N. G., de Mello, M. C., de Mello, F. G., and Aracava, Y. (1999). Direct inhibition of the N-methyl-D-aspartate receptor channel by dopamine and (+)-SKF38393. Br. J. Pharmacol. 126, 1847–1855. doi: 10.1038/sj.bjp.0702479
Cazorla, M., Shegda, M., Ramesh, B., Harrison, N. L., and Kellendonk, C. (2012). Striatal D2 receptors regulate dendritic morphology of medium spiny neurons via Kir2 channels. J. Neurosci. 32, 2398–2409. doi: 10.1523/JNEUROSCI.6056-11.2012
Centonze, D., Gubellini, P., Pisani, A., Bernardi, G., and Calabresi, P. (2003). Dopamine, acetylcholine and nitric oxide systems interact to induce corticostriatal synaptic plasticity. Rev Neurosci. 14, 207–216. doi: 10.1515/REVNEURO.2003.14.3.207
Cepeda, C., Buchwald, N. A., and Levine, M. S. (1993). Neuromodulatory actions of dopamine in the neostriatum are dependent upon the excitatory amino acid receptor subtypes activated. Proc. Natl. Acad. Sci. U.S.A. 90, 9576–9580. doi: 10.1073/pnas.90.20.9576
Cepeda, C., Colwell, C. S., Itri, J. N., Chandler, S. H., and Levine, M. S. (1998). Dopaminergic modulation of NMDA-induced whole cell currents in neostriatal neurons in slices: contribution of calcium conductances. J. Neurophysiol. 79, 82–94. doi: 10.1152/jn.1998.79.1.82
Chao, S. Z., Lu, W., Lee, H. K., Huganir, R. L., and Wolf, M. E. (2002). D(1) dopamine receptor stimulation increases GluR1 phosphorylation in postnatal nucleus accumbens cultures. J. Neurochem. 81, 984–992. doi: 10.1046/j.1471-4159.2002.00877.x
Chen, Y., Yu, F., Sharp, E., Beacham, D., Scheuer, T., and Catterall, W. (2008). Functional properties and differential neuromodulation of Nav1.6 channels. Mol. Cell. Neurosci. 38, 607–615. doi: 10.1016/j.mcn.2008.05.009
Chen, Y., Yu, F. H., Surmeier, D. J., Scheuer, T., and Catterall, W. A. (2006). Neuromodulation of Na+ Channel Slow Inactivation via cAMP-Dependent Protein Kinase and Protein Kinase, C. Neuron 49, 409–420. doi: 10.1016/j.neuron.2006.01.009
Chevalier, G., and Deniau, J. M. (1990). Disinhibition as a basic process in the expression of striatal functions. Trends Neurosci. 13, 277–280. doi: 10.1016/0166-2236(90)90109-N
Chuhma, N., Mingote, S., Kalmbach, A., Yetnikoff, L., and Rayport, S. (2017). Heterogeneity in dopamine neuron synaptic actions across the striatum and its relevance for Schizophrenia. Biol. Psychiatry 81, 43–51. doi: 10.1016/j.biopsych.2016.07.002
Chuhma, N., Mingote, S., Moore, H., and Rayport, S. (2014). Dopamine neurons control striatal cholinergic neurons via regionally heterogeneous dopamine and glutamate signaling. Neuron 81, 901–912. doi: 10.1016/j.neuron.2013.12.027
Chuhma, N., Tanaka, K. F., Hen, R., and Rayport, S. (2011). Functional connectome of the striatal medium spiny neuron. J. Neurosci. 31, 1183–1192. doi: 10.1523/JNEUROSCI.3833-10.2011
Chuhma, N., Zhang, H., Masson, J., Zhuang, X., Sulzer, D., Hen, R., et al. (2004). Dopamine neurons mediate a fast excitatory signal via their glutamatergic synapses. J. Neurosci. 24, 972–981. doi: 10.1523/JNEUROSCI.4317-03.2004
Colledge, M., Dean, R. A., Scott, G. K., Langeberg, L. K., Huganir, R. L., and Scott, J. D. (2000). Targeting of PKA to glutamate receptors through a MAGUK-AKAP Complex. Neuron 27, 107–119. doi: 10.1016/S0896-6273(00)00013-1
Conte, A., Khan, N., Defazio, G., Rothwell, J. C., and Berardelli, A. (2013). Pathophysiology of somatosensory abnormalities in Parkinson disease. Nat. Rev. Neurol. 9, 687–697. doi: 10.1038/nrneurol.2013.224
Cooper, A. J., and Stanford, I. M. (2001). Dopamine D2 receptor mediated presynaptic inhibition of striatopallidal GABAA IPSCs in vitro. Neuropharmacology. 41, 62–71. doi: 10.1016/S0028-3908(01)00038-7
Corbit, V. L., Whalen, T. V., Zitelli, K. T., Crilly, S. Y., Rubin, J. E., and Gittis, A. H. (2016). Pallidostriatal projections promote oscillations in a dopamine-depleted biophysical network model. J. Neurosci. 36, 5556–5571. doi: 10.1523/JNEUROSCI.0339-16.2016
Courtière, A., Hardouin, J., Locatelli, V., Turle-Lorenzo, N., Amalric, M., Vidal, F., et al. (2005). Selective effects of partial striatal 6-OHDA lesions on information processing in the rat. Eur. J. Neurosci. 21, 1973–1983. doi: 10.1111/j.1460-9568.2005.04015.x
Cragg, S. J., Baufreton, J., Xue, Y., Bolam, J. P., and Bevan, M. D. (2004). Synaptic release of dopamine in the subthalamic nucleus. Eur. J. Neurosci. 20, 1788–1802. doi: 10.1111/j.1460-9568.2004.03629.x
Crittenden, J. R., Tillberg, P. W., Riad, M. H., Shima, Y., Gerfen, C. R., Curry, J., et al. (2016). Striosome–dendron bouquets highlight a unique striatonigral circuit targeting dopamine-containing neurons. Proc. Natl. Acad. Sci. U.S.A. 113, 11318–11323. doi: 10.1073/pnas.1613337113
Crunelli, V., Tóth, T. I., Cope, D. W., Blethyn, K., and Hughes, S. W. (2005). The 'window' T-type calcium current in brain dynamics of different behavioural states. J. Physiol. 562(Pt 1), 121–129. doi: 10.1113/jphysiol.2004.076273
Cui, C., Xu, M., and Atzori, M. (2006). Voltage-dependent block of N-methyl-D-aspartate receptors by dopamine D1 receptor ligands. Mol. Pharmacol. 70, 1761–1770. doi: 10.1124/mol.106.028332
Cui, G., Jun, S. B., Jin, X., Pham, M. D., Vogel, S. S., Lovinger, D. M., et al. (2013). Concurrent activation of striatal direct and indirect pathways during action initiation. Nature 494, 238–242. doi: 10.1038/nature11846
Day, M., Wokosin, D., Plotkin, J. L., Tian, X., and Surmeier, D. J. (2008). Differential excitability and modulation of striatal medium spiny neuron dendrites. J. Neurosci. 28, 11603–11614. doi: 10.1523/JNEUROSCI.1840-08.2008
Dong, Y., and White, F. J. (2003). Dopamine D1-class receptors selectively modulate a slowly inactivating potassium current in rat medial prefrontal cortex pyramidal neurons. J. Neurosci. 23, 2686–2695.
Dong, Y., Cooper, D., Nasif, F., Hu, X. T., and White, F. J. (2004). Dopamine modulates inwardly rectifying potassium currents in medial prefrontal cortex pyramidal neurons. J. Neurosci. 24, 3077–3085. doi: 10.1523/JNEUROSCI.4715-03.2004
Du, K., Wu, Y., Lindroos, R., Liu, Y., Rózsa, B., Katona, G., et al. (2017). Cell-type–specific inhibition of the dendritic plateau potential in striatal spiny projection neurons. Proc. Natl. Acad. Sci. U.S.A. 114, E7612–E7621. doi: 10.1073/pnas.1704893114
Dunah, A. W., Sirianni, A. C., Fienberg, A. A., Bastia, E., Schwarzschild, M. A., and Standaert, D. G. (2004). Dopamine D1-dependent trafficking of striatal N-methyl-D-aspartate glutamate receptors requires Fyn protein tyrosine kinase but not DARPP-32. Mol. Pharmacol. 65, 121–129. doi: 10.1124/mol.65.1.121
Duvoisin, R. C. (1967). Cholinergic-anticholinergic antagonism in parkinsonism. Arch. Neurol. 17, 124–136. doi: 10.1001/archneur.1967.00470260014002
Evans, R. C., Maniar, Y. M., and Blackwell, K. T. (2013). Dynamic modulation of spike timing-dependent calcium influx during corticostriatal upstates. J. Neurophysiol. 110, 1631–1645. doi: 10.1152/jn.00232.2013
Evans, R. C., Morera-Herreras, T., Cui, Y., Du, K., Sheehan, T., Kotaleski, J. H., et al. (2012). The effects of NMDA subunit composition on calcium influx and spike timing-dependent plasticity in striatal medium spiny neurons. PLoS Comput. Biol. 8:e1002493. doi: 10.1371/journal.pcbi.1002493
Evans, R. C., Zhu, M., and Khaliq, Z. M. (2017). Dopamine inhibition differentially controls excitability of substantia nigra dopamine neuron subpopulations through T-Type calcium channels. J. Neurosci. 37, 3704–3720. doi: 10.1523/JNEUROSCI.0117-17.2017
Few, W. P., Scheuer, T., and Catterall, W. A. (2007). Dopamine modulation of neuronal Na+ channels requires binding of A kinase-anchoring protein 15 and PKA by a modified leucine zipper motif. Proc. Natl. Acad. Sci. U.S.A. 104, 5187–5192. doi: 10.1073/pnas.0611619104
Fieblinger, T., Graves, S. M., Sebel, L. E., Alcacer, C., Plotkin, J. L., Gertler, T. S., et al. (2014). Cell type-specific plasticity of striatal projection neurons in parkinsonism and L-DOPA-induced dyskinesia. Nat. Commun. 5:5316. doi: 10.1038/ncomms6316
Floran, B., Floran, L., Sierra, A., and Aceves, J. (1997). D2 receptor-mediated inhibition of GABA release by endogenous dopamine in the rat globus pallidus. Neurosci. Lett. 237, 1–4. doi: 10.1016/S0304-3940(97)00784-2
Flores-Barrera, E., Vizcarra-Chacón, B. J., Bargas, J., Tapia, D., and Galarraga, E. (2011). Dopaminergic modulation of corticostriatal responses in medium spiny projection neurons from direct and indirect pathways. Front. Sys. Neurosci. 5:15. doi: 10.3389/fnsys.2011.00015
Flores-Hernández, J., Cepeda, C., Hernández-Echeagaray, E., Calvert, C. R., Jokel, E. S., Fienberg, A. A., et al. (2002). Dopamine enhancement of NMDA currents in dissociated medium-sized striatal neurons: role of D1 receptors and DARPP-32. J. Neurophysiol. 88, 3010–3020. doi: 10.1152/jn.00361.2002
Flores-Hernandez, J., Hernandez, S., Snyder, G. L., Yan, Z., Fienberg, A. A., Moss, S. J., et al. (2000). D(1) dopamine receptor activation reduces GABA(A) receptor currents in neostriatal neurons through a PKA/DARPP-32/PP1 signaling cascade. J. Neurophysiol. 83, 2996–3004. doi: 10.1152/jn.2000.83.5.2996
Freund, T. F., Powell, J. F., and Smith, A. D. (1984). Tyrosine hydroxylase-immunoreactive boutons in synaptic contact with identified striatonigral neurons, with particular reference to dendritic spines. Neuroscience 13, 1189–1215. doi: 10.1016/0306-4522(84)90294-X
Galarraga, E., Hernandez-Lopez, S., Reyes, A., Barral, J., and Bargas, J. (1997). Dopamine facilitates striatal EPSPs through an L-type Ca2+ conductance. Neuroreport 8, 2183–2186. doi: 10.1097/00001756-199707070-00019
Gao, T., Yatani, A., Dell'Acqua, M., Sako, H., Green, S., Dascal, N., et al. (1997). cAMP-Dependent regulation of Cardiac L-Type Ca2+ channels requires membrane targeting of PKA and phosphorylation of channel subunits. Neuron 19, 185–196. doi: 10.1016/S0896-6273(00)80358-X
Gerfen, C. R. (2004). “Chapter 18: Basal ganglia,” in The Rat Nervous System 3rd Edn, ed G. Paxinos (Burlington, NJ: Academic Press), 455–508. doi: 10.1016/B978-012547638-6/50019-5
Gerfen, C. R., and Surmeier, D. J. (2011). Modulation of striatal projection systems by dopamine. Annu. Rev. Neurosci. 34, 441–466. doi: 10.1146/annurev-neuro-061010-113641
Glajch, K. E., Kelver, D. A., Hegeman, D. J., Cui, Q., Xenias, H. S., Augustine, E. C., et al. (2016). Npas1+ pallidal neurons target striatal projection neurons. J. Neurosci. 36 5472–5488. doi: 10.1523/JNEUROSCI.1720-15.2016
Glovaci, I., Caruana, D., and Chapman, C. (2014). Dopaminergic enhancement of excitatory synaptic transmission in layer II entorhinal neurons is dependent on D1-like receptor-mediated signaling. Neuroscience 258, 74–83. doi: 10.1016/j.neuroscience.2013.10.076
Golden, J. P., Demaro, J. A. III., Knoten, A., Hoshi, M., Pehek, E., Johnson, E. M. Jr., et al. (2013). Dopamine-dependent compensation maintains motor behavior in mice with developmental ablation of dopaminergic neurons. J. Neurosci. 33, 17095–17107. doi: 10.1523/JNEUROSCI.0890-13.2013
Golding, N. L., Mickus, T. J., Katz, Y., Kath, W. L., and Spruston, N. (2005). Factors mediating powerful voltage attenuation along CA1 pyramidal neuron dendrites. J. Physiol 568, 69–82. doi: 10.1113/jphysiol.2005.086793
Graybiel, A. (1984). Correspondence between the Dopamine islands and striosomes of the mammalian striatum. Neuroscience 13, 1157–1187. doi: 10.1016/0306-4522(84)90293-8
Grillner, S., and Robertson, B. (2016). The basal Ganglia over 500 million years. Curr. Biol. 26, R1088–R1100. doi: 10.1016/j.cub.2016.06.041
Guzmán, J. N., Hernández, A., Galarraga, E., Tapia, D., Laville, A., Vergara, R., et al. (2003). Dopaminergic modulation of axon collaterals interconnecting spiny neurons of the rat striatum. J. Neurosci. 23, 8931–8940.
Hallett, P. J., Spoelgen, R., Hyman, B. T., Standaert, D. G., and Dunah, A. W. (2006). Dopamine D1 activation potentiates striatal NMDA receptors by tyrosine phosphorylation-dependent subunit trafficking. J. Neurosci. 26, 4690–4700. doi: 10.1523/JNEUROSCI.0792-06.2006
Hammond, C., Bergman, H., and Brown, P. (2007). Pathological synchronization in Parkinson's disease: networks, models and treatments. Trends Neurosci. 30, 357–364. doi: 10.1016/j.tins.2007.05.004
Harsing, L. G., and Zigmond, M. J. (1997). Influence of dopamine on GABA release in striatum: evidence for D1–D2 interactions and non-synaptic influences. Neuroscience 77, 419–429. doi: 10.1016/S0306-4522(96)00475-7
Hegeman, D. J., Hong, E. S., Hernández, V. M., and Chan, C. S. (2016). The external globus pallidus: progress and perspectives. Eur. J. Neurosci. 43, 1239–1265. doi: 10.1111/ejn.13196
Herlitze, S., Garcia, D., Mackie, K., Hille, B., Scheuer, T., and Catterall, W. (1996). Modulation of Ca2+ channels by G-protein βγ subunits. Nature 380, 258–262. doi: 10.1038/380258a0
Hernández, A., Ibáñez-Sandoval, O., Sierra, A., Valdiosera, R., Tapia, D., Anaya, V., et al. (2006). Control of the subthalamic innervation of the rat globus pallidus by D2/3 and D4 dopamine receptors. J. Neurophysiol. 96, 2877–2888. doi: 10.1152/jn.00664.2006
Hernández, A., Sierra, A., Valdiosera, R., Florán, B., Erlij, D., and Aceves, J. (2007). Presynaptic D1 dopamine receptors facilitate glutamatergic neurotransmission in the rat globus pallidus. Neurosci. Lett. 425, 188–191. doi: 10.1016/j.neulet.2007.08.026
Hernández-Echeagaray, E., Cepeda, C., Ariano, M. A., Lobo, M. K., Sibley, D. R., and Levine, M. S. (2007). Dopamine reduction of GABA currents in striatal medium-sized spiny neurons is mediated principally by the D(1) receptor subtype. Neurochem. Res. 32, 229–240. doi: 10.1007/s11064-006-9141-8
Hernández-Echeagaray, E., Starling, A. J., Cepeda, C., and Levine, M. S. (2004). Modulation of AMPA currents by D2 dopamine receptors in striatal medium-sized spiny neurons: are dendrites necessary? Eur. J. Neurosci. 19, 2455–2463. doi: 10.1111/j.0953-816X.2004.03344.x
Hernandez-Lopez, S., Bargas, J., Surmeier, D. J., Reyes, A., and Galarraga, E. (1997). D1 receptor activation enhances evoked discharge in neostriatal medium spiny neurons by modulating an L-type Ca2+ conductance. J. Neurosci. 17, 3334–3342.
Hernández-López, S., Tkatch, T., Perez-Garci, E., Galarraga, E., Bargas, J., Hamm, H., et al. (2000). D2 dopamine receptors in striatal medium spiny neurons reduce L-Type Ca2+ currents and excitability via a novel PLCβ1–IP3–calcineurin-signaling cascade. J. Neurosci. 20, 8987–8995.
Hikida, T., Kitabatake, Y., Pastan, I., and Nakanishi, S. (2003). Acetylcholine enhancement in the nucleus accumbens prevents addictive behaviors of cocaine and morphine. Proc. Natl. Acad. Sci. U.S.A. 100, 6169–6173. doi: 10.1073/pnas.0631749100
Hines, M. L., and Carnevale, N. T. (1997). The NEURON simulation environment. Neural Comput. 9, 1179–1209. doi: 10.1162/neco.1997.9.6.1179
Hoffman, D. A., and Johnston, D. (1998). Downregulation of transient K+ channels in dendrites of hippocampal CA1 pyramidal neurons by activation of PKA and PKC. J. Neurosci. 18, 3521–3528.
Hoffman, D. A., Magee, J. C., Colbert, C. M., and Johnston, D. (1997). K+ channel regulation of signal propagation in dendrites of hippocampal pyramidal neurons. Nature 387, 869–875. doi: 10.1038/43119
Hong, S. (2013). Dopamine system: manager of neural pathways. Front. Hum. Neurosci. 7:854. doi: 10.3389/fnhum.2013.00854
Hong, S., and Hikosaka, O. (2008). The globus pallidus sends reward-related signals to the lateral habenula. Neuron 60, 720–729. doi: 10.1016/j.neuron.2008.09.035
Hopf, F. W., Seif, T., Mohamedi, M. L., Chen, B. T., and Bonci, A. (2010). The small-conductance calcium-activated potassium channel is a key modulator of firing and long-term depression in the dorsal striatum. Eur. J. Neurosci. 31, 1946–1959. doi: 10.1111/j.1460-9568.2010.07231.x
Howe, M. W., and Dombeck, D. A. (2016). Rapid signalling in distinct dopaminergic axons during locomotion and reward. Nature 535, 505–510. doi: 10.1038/nature18942
Hu, W., Tian, C., Li, T., Yang, M., Hou, H., and Shu, Y. (2009). Distinct contributions of Nav1.6 and Nav1.2 in action potential initiation and backpropagation. Nat. Neurosci. 12, 996–1002. doi: 10.1038/nn.2359
Humphries, M. D., Stewart, R. D., and Gurney, K. N. (2006). A physiologically plausible model of action selection and oscillatory activity in the basal ganglia. J. Neurosci. 26, 12921–12942. doi: 10.1523/JNEUROSCI.3486-06.2006
Humphries, M. D., Wood, R., and Gurney, K. (2009). Dopamine-modulated dynamic cell assemblies generated by the GABAergic striatal microcircuit. Neural Netw, 22, 1174–1188. doi: 10.1016/j.neunet.2009.07.018
Janssen, M. J., Ade, K. K., Fu, Z., and Vicini, S. (2009). Dopamine modulation of GABA tonic conductance in striatal output neurons. J. Neurosci. 29, 5116–5126. doi: 10.1523/JNEUROSCI.4737-08.2009
Kasai, H., and Neher, E. (1992). Dihydropyridine-sensitive and omega-conotoxin-sensitive calcium channels in a mammalian neuroblastoma-glioma cell line. J. Physiol. 448, 161–188. doi: 10.1113/jphysiol.1992.sp019035
Ketzef, M., Spigolon, G., Johansson, Y., Bonito-Oliva, A., Fisone, G., and Silberberg, G. (2017). Dopamine depletion impairs bilateral sensory processing in the striatum in a pathway-dependent manner. Neuron 94, 855–865.e5. doi: 10.1016/j.neuron.2017.05.004
Kheirbek, M. A., Britt, J. P., Beeler, J. A., Ishikawa, Y., McGehee, D. S., and Zhuang, X. (2009). Adenylyl cyclase type 5 contributes to corticostriatal plasticity and striatum-dependent learning. J. Neurosci. 29, 12115–12124. doi: 10.1523/JNEUROSCI.3343-09.2009
Kisilevsky, A. E., Mulligan, S. J., Altier, C., Iftinca, M. C., Varela, D., Tai, C., et al. (2008). D1 receptors physically interact with N-type calcium channels to regulate channel distribution and dendritic calcium entry. Neuron 58, 557–570. doi: 10.1016/j.neuron.2008.03.002
Kitabatake, Y., Hikida, T., Watanabe, D., Pastan, I., and Nakanishi, S. (2003). Impairment of reward-related learning by cholinergic cell ablation in the striatum. Proc. Natl. Acad. Sci. U.S.A. 100, 7965–7970. doi: 10.1073/pnas.1032899100
Kitai, S. T., and Surmeier, D. J. (1993). Cholinergic and dopaminergic modulation of potassium conductances in neostriatal neurons. Adv. Neurol. 60, 40–52.
Kliem, M. A., Maidment, N. T., Ackerson, L. C., Chen, S., Smith, Y., and Wichmann, T. (2007). Activation of nigral and pallidal dopamine D1-like receptors modulates basal ganglia outflow in monkeys. J. Neurophysiol. 98, 1489–1500. doi: 10.1152/jn.00171.2007
Knowlton, B. J., Mangels, J. A., and Squire, L. R. (1996). A neostriatal habit learning system in humans. Science 273, 1399–1402. doi: 10.1126/science.273.5280.1399
Kohnomi, S., Koshikawa, N., and Kobayashi, M. (2012). D2-like dopamine receptors differentially regulate unitary IPSCs depending on presynaptic GABAergic neuron subtypes in rat nucleus accumbens shell. J. Neurophysiol. 107, 692–703. doi: 10.1152/jn.00281.2011
Kravitz, A. V., Tye, L. D., and Kreitzer, A. C. (2012). Distinct roles for direct and indirect pathway striatal neurons in reinforcement. Nat. Neurosci. 15, 816–818. doi: 10.1038/nn.3100
Kreitzer, A. C., and Berke, J. D. (2011). Investigating striatal function through cell-type-specific manipulations. Neuroscience 198, 19–26. doi: 10.1016/j.neuroscience.2011.08.018
Kumar, A., Cardanobile, S., Rotter, S., and Aertsen, A. (2011). The role of inhibition in generating and controlling Parkinson?s disease oscillations in the Basal Ganglia. Front. Sys. Neurosci. 5:86. doi: 10.3389/fnsys.2011.00086
Lacey, M. G., Mercuri, N. B., and North, R. A. (1990). Actions of cocaine on rat dopaminergic neurones in vitro. Br. J. Pharmacol. 99, 731–735. doi: 10.1111/j.1476-5381.1990.tb12998.x
Lavian, H., Almog, M., Madar, R., Loewenstern, Y., Bar-Gad, I., Okun, E., et al. (2017). Dopaminergic modulation of synaptic integration and firing patterns in the rat entopeduncular nucleus. J. Neurosci. 37, 7177–7187. doi: 10.1523/JNEUROSCI.0639-17.2017
Leblois, A., Boraud, T., Meissner, W., Bergman, H., and Hansel, D. (2006). Competition between feedback loops underlies normal and pathological dynamics in the basal Ganglia. J. Neurosci. 26, 3567–3583. doi: 10.1523/JNEUROSCI.5050-05.2006
Lee, J. H., Daugharthy, E. R., Scheiman, J., Kalhor, R., Yang, J. L., Ferrante, T. C., et al. (2014). Highly multiplexed subcellular RNA sequencing in situ. Science 343, 1360–1363. doi: 10.1126/science.1250212
Levine, M. S., Altemus, K. L., Cepeda, C., Cromwell, H. C., Crawford, C., Ariano, M. A., et al. (1996). Modulatory actions of dopamine on NMDA receptor-mediated responses are reduced in D1A-deficient mutant mice. J. Neurosci. 16, 5870–5882.
Levine, M. S., Li, Z., Cepeda, C., Cromwell, H. C., and Altemus, K. L. (1996b). Neuromodulatory actions of dopamine on synaptically-evoked neostriatal responses in slices. Synapse 24, 65–78. doi: 10.1002/syn.890240102
Lin, L., Sun, W., Kung, F., Dell'Acqua, M. L., and Hoffman, D. A. (2011). AKAP79/150 impacts intrinsic excitability of hippocampal neurons through phospho-regulation of A-type K+ channel trafficking. J. Neurosci. 31, 1323–1332. doi: 10.1523/JNEUROSCI.5383-10.2011
Lin, L., Sun, W., Wikenheiser, A. M., Kung, F., and Hoffman, D. A. (2010). KChIP4a regulates Kv4.2 channel trafficking through PKA phosphorylation. Mol. Cell Neurosci. 43, 315–325. doi: 10.1016/j.mcn.2009.12.005
Lindahl, M., and Hellgren Kotaleski, J. (2016). Untangling Basal Ganglia network dynamics and function: role of dopamine depletion and inhibition investigated in a spiking network model. Eneuro 3, 1–29. doi: 10.1523/ENEURO.0156-16.2016
López-Huerta, V. G., Carrillo-Reid, L., Galarraga, E., Tapia, D., Fiordelisio, T., Drucker-Colin, R., et al. (2013). The balance of striatal feedback transmission is disrupted in a model of parkinsonism. J. Neuroscience 33, 4964–4975. doi: 10.1523/JNEUROSCI.4721-12.2013
Mallet, N., Ballion, B., Le Moine, C., and Gonon, F. (2006). Cortical inputs and GABA interneurons imbalance projection neurons in the striatum of parkinsonian rats. J. Neurosci. 26, 3875–3884. doi: 10.1523/JNEUROSCI.4439-05.2006
Mallet, N., Micklem, B. R., Henny, P., Brown, M. T., Williams, C., Bolam, J. P., et al. (2012). Dichotomous organization of the external globus pallidus. Neuron 74, 1075–1086. doi: 10.1016/j.neuron.2012.04.027
Mallet, N., Pogosyan, A., Márton, L. F., Bolam, J. P., Brown, P., and Magill, P. J. (2008). Parkinsonian beta oscillations in the external globus pallidus and their relationship with subthalamic nucleus activity. J. Neurosci. 28, 14245–14258. doi: 10.1523/JNEUROSCI.4199-08.2008
Mamad, O., Delaville, C., Benjelloun, W., and Benazzouz, A. (2015). Dopaminergic control of the globus pallidus through activation of D2 receptors and its impact on the electrical activity of subthalamic nucleus and substantia nigra reticulata neurons. PLoS ONE 10:e0119152. doi: 10.1371/journal.pone.0119152
Martone, M. E., Zhang, S., Gupta, A., Qian, X., He, H., Price, D. L., et al. (2003). Neuroinform 1:379. doi: 10.1385/NI:1:4:379
Matsumoto, M., and Hikosaka, O. (2007). Lateral habenula as a source of negative reward signals in dopamine neurons. Nature 447, 1111–1115. doi: 10.1038/nature05860
Matsumoto, N., Hanakawa, T., Maki, S., Graybiel, A. M., and Kimura, M. (1999). Nigrostriatal dopamine system in learning to perform sequential motor tasks in a predictive manner. J. Neurophysiol. 82, 978–998. doi: 10.1152/jn.1999.82.2.978
Maurice, N., Liberge, M., Jaouen, F., Ztaou, S., Hanini, M., Camon, J., et al. (2015). Striatal cholinergic interneurons control motor behavior and Basal Ganglia function in experimental Parkinsonism. Cell Reports 13, 657–666. doi: 10.1016/j.celrep.2015.09.034
Maurice, N., Tkatch, T., Meisler, M., Sprunger, L. K., and Surmeier, D. J. (2001). D1/D5 dopamine receptor activation differentially modulates rapidly inactivating and persistent sodium currents in prefrontal cortex pyramidal neurons. J. Neurosci. 21, 2268–2277.
McGeer, P. L., Grewaal, D. S., and McGeer, E. G. (1974). Influence of noncholinergic drugs on rat striatal acetylcholine levels. Brain Res. 80, 211–217. doi: 10.1016/0006-8993(74)90685-4
McHaffie, J. G., Stanford, T. R., Stein, B. E., Coizet, V., and Redgrave, P. (2005). Subcortical loops through the basal ganglia. Trends Neurosci. 28, 401–407. doi: 10.1016/j.tins.2005.06.006
Mikula, S., Parrish, S. K., Trimmer, J. S., and Jones, E. G. (2009). Complete 3D visualization of primate striosomes by KChIP1 immunostaining. J. Comp. Neurol. 514, 507–517. doi: 10.1002/cne.22051
Mintz, I., Hammond, C., and Féger, J. (1986). Excitatory effect of iontophoretically applied dopamine on identified neurons of the rat subthalamic nucleus. Brain Res. 375, 172–175. doi: 10.1016/0006-8993(86)90971-6
Momiyama, T., and Nishijo, T. (2017). Dopamine and serotonin-induced modulation of GABAergic and glutamatergic transmission in the striatum and basal forebrain. Front. Neuroanatomy 11:42. doi: 10.3389/fnana.2017.00042
Morris, G., Arkadir, D., Nevet, A., Vaadia, E., and Bergman, H. (2004). Coincident but distinct messages of midbrain dopamine and striatal tonically active neurons. Neuron 43, 133–143. doi: 10.1016/j.neuron.2004.06.012
Moyer, J. T., Wolf, J. A., and Finkel, L. H. (2007). Effects of dopaminergic modulation on the integrative properties of the ventral striatal medium spiny neuron. J. Neurophysiol. 98, 3731–3748. doi: 10.1152/jn.00335.2007
Nair, A. G., Gutierrez-Arenas, O., Eriksson, O., Vincent, P., and Hellgren Kotaleski, J. (2015). Sensing positive versus negative reward signals through adenylyl cyclase coupled GPCRs in direct and indirect pathway striatal medium spiny neurons. J. Neurosci. 35, 14017–14030. doi: 10.1523/JNEUROSCI.0730-15.2015
Nair, A. G., Bhalla, U. S., and Hellgren Kotaleski, J. (2016). Role of DARPP-32 and ARPP-21 in the emergence of temporal constraints on striatal calcium and dopamine integration. PLOS Comput. Biol. 12:e1005080. doi: 10.1371/journal.pcbi.1005080
Nambu, A., and Tachibana, Y. (2014). Mechanism of parkinsonian neuronal oscillations in the primate basal ganglia: some considerations based on our recent work. Front. Sys. Neurosci. 8:74. doi: 10.3389/fnsys.2014.00074
Napier, T. C., Simson, P. E., and Givens, B. S. (1991). Dopamine electrophysiology of ventral pallidal/substantia innominata neurons: comparison with the dorsal globus pallidus. J. Pharmacol. Exp. Therapeut. 258, 249–262.
Nelson, A. B., Hammack, N., Yang, C. F., Shah, N. M., Seal, R. P., and Kreitzer, A. C. (2014). Striatal cholinergic interneurons drive gaba release from dopamine terminals. Neuron 82, 63–70. doi: 10.1016/j.neuron.2014.01.023
Neves, S. R., Tsokas, P., Sarkar, A., Grace, E. A., Rangamani, P., Taubenfeld, S. M., Iyengar, R., et al. (2008). Cell shape and negative links in regulatory motifs together control spatial information flow in signaling networks. sCell 133, 666–680. doi: 10.1016/j.cell.2008.04.025
Ni, Z., Gao, D., Bouali-Benazzouz, R., Benabid, A.-L., and Benazzouz, A. (2001). Effect of microiontophoretic application of dopamine on subthalamic nucleus neuronal activity in normal rats and in rats with unilateral lesion of the nigrostriatal pathway. Eur. J. Neurosci. 14, 373–381. doi: 10.1046/j.0953-816x.2001.01644.x
Nieto Mendoza, E., and Hernández Echeagaray, E. (2015). Dopaminergic modulation of striatal inhibitory transmission and long-term plasticity. Neural Plasticity 2015:789502. doi: 10.1155/2015/789502
Nithianantharajah, J., Komiyama, N. H., McKechanie, A., Johnstone, M., Blackwood, D. H., Clair, D., et al. (2012). Synaptic scaffold evolution generated components of vertebrate cognitive complexity. Nat. Neurosci. 16, 16–24. doi: 10.1038/nn.3276
Oldenburg, I. A., and Ding, J. B. (2011). Cholinergic modulation of synaptic integration and dendritic excitability in the striatum. Curr. Opin. Neurobiol. 21, 425–432. doi: 10.1016/j.conb.2011.04.004
Pacheco-Cano, M. T., Bargas, J., Hernández-López, S., Tapia, D., and Galarraga, E. (1996). Inhibitory action of dopamine involves a subthreshold Cs(+)-sensitive conductance in neostriatal neurons. Exp Brain Res. 110, 205–211. doi: 10.1007/BF00228552
Paille, V., Fino, E., Du, K., Morera-Herreras, T., Perez, S., Kotaleski, J. H., et al. (2013). GABAergic circuits control spike-timing-dependent plasticity. J. Neurosci. 33, 9353–9363. doi: 10.1523/JNEUROSCI.5796-12.2013
Pavlides, A., Hogan, S. J., and Bogacz, R. (2015). Computational models describing possible mechanisms for generation of excessive beta oscillations in Parkinson's disease. PLoS Comput. Biol. 11:e1004609. doi: 10.1371/journal.pcbi.1004609
Payandeh, J., Gamal El-Din, T. M., Scheuer, T., Zheng, N., and Catterall, W. A. (2012). Crystal structure of a voltage-gated sodium channel in two potentially inactivated states. Nature 486, 135–139. doi: 10.1038/nature11077
Pickel, V. M., Beckley, S. C., Joh, T. H., and Reis, D. J. (1981). Ultrastructural immunocytochemical localization of tyrosine hydroxylase in the neostriatum. Brain Res. 225, 373–385. doi: 10.1016/0006-8993(81)90843-X
Pisani, A., Bonsi, P., Centonze, D., Calabresi, P., and Bernardi, G. (2000). Activation of D2-Like dopamine receptors reduces synaptic inputs to striatal cholinergic interneurons. J. Neuroscience 20:RC69.
Planert, H., Berger, T. K., and Silberberg, G. (2013). Membrane properties of striatal direct and indirect pathway neurons in mouse and rat slices and their modulation by dopamine. PLoS ONE 8:e57054. doi: 10.1371/journal.pone.0057054
Plotkin, J., Day, M., and Surmeier, D. (2011). Synaptically driven state transitions in distal dendrites of striatal spiny neurons. Nat. Neurosci. 14, 881–888. doi: 10.1038/nn.2848
Podda, M. V., Riccardi, E., D'Ascenzo, M., Azzena, G. B., and Grassi, C. (2010). Dopamine D1-like receptor activation depolarizes medium spiny neurons of the mouse nucleus accumbens by inhibiting inwardly rectifying K+ currents through a cAMP-dependent protein kinase A-independent mechanism. Neuroscience 167, 678–690. doi: 10.1016/j.neuroscience.2010.02.075
Ponzi, A., and Wickens, J. (2010). Sequentially switching cell assemblies in random inhibitory networks of spiking neurons in the striatum. J. Neurosci. 30, 5894–5911. doi: 10.1523/JNEUROSCI.5540-09.2010
Price, C. J., Kim, P., and Raymond, L. A. (1999). D1 dopamine receptor-induced cyclic AMP-dependent protein kinase phosphorylation and potentiation of striatal glutamate receptors. J. Neurochem. 73, 2441–2446. doi: 10.1046/j.1471-4159.1999.0732441.x
Przedborski, S., Levivier, M., Jiang, H., Ferreira, M., Jackson-Lewis, V., Donaldson, D., et al. (1995). Dose-dependent lesions of the dopaminergic nigrostriatal pathway induced by intrastriatal injection of 6-hydroxydopamine. Neuroscience 67, 631–647. doi: 10.1016/0306-4522(95)00066-R
Raz, A., Vaadia, E., and Bergman, H. (2000). Firing patterns and correlations of spontaneous discharge of pallidal neurons in the normal and the tremulous 1-methyl-4-phenyl- 1,2,3,6-tetrahydropyridine vervet model of parkinsonism. J. Neurosci. 20, 8559–8571.
Redgrave, P., Prescott, T. J., and Gurney, K. (1999). The basal ganglia: a vertebrate solution to the selection problem? Neuroscience 89, 1009–1023. doi: 10.1016/S0306-4522(98)00319-4
Reig, R., and Silberberg, G. (2014). Multisensory integration in the mouse striatum. Neuron 83, 1200–1212. doi: 10.1016/j.neuron.2014.07.033
Rohatgi, A. (2016). WebPlotDigitizer v. 3.10 [webpage]. Available online at: http://arohatgi.info/WebPlotDigitizer
Rosenmund, C., Carr, D., Bergeson, S., Nilaver, G., Scott, J., and Westbrook, G. (1994). Anchoring of protein kinase A is required for modulation of AMPA/kainate receptors on hippocampal neurons. Nature 368, 853–856. doi: 10.1038/368853a0
Roth, A., and Häusser, M. (2001). Compartmental models of rat cerebellar Purkinje cells based on simultaneous somatic and dendritic patch-clamp recordings. J. Physiol. 535(Pt 2), 445–472. doi: 10.1111/j.1469-7793.2001.00445.x
Rudy, B. (1978). Slow inactivation of the sodium conductance in squid giant axons. Pronase resistance. J. Physiol. 283, 1–21. doi: 10.1113/jphysiol.1978.sp012485
Ruskin, D. N., Bergstrom, D. A., and Walters, J. R. (2002) Nigrostriatal lesion dopamine agonists affect firing patterns of rodent entopeduncular nucleus neurons. J. Neurophysiol. 88, 487–496. doi: 10.1152/jn.00844.2001
Sathian, K., Zangaladze, A., Green, J., Vitek, J. L., and DeLong, M. R. (1997). Tactile spatial acuity and roughness discrimination: impairments due to aging and Parkinson's disease. Neurology 49, 168–177. doi: 10.1212/WNL.49.1.168
Schiffmann, S. N., Desdouits, F., Menu, R., Greengard, P., Vincent, J. D., Vanderhaeghen, J. J., et al. (1998). Modulation of the voltage-gated sodium current in rat striatal neurons by DARPP-32, an inhibitor of protein phosphatase. Eur. J. Neurosci. 10, 1312–1320. doi: 10.1046/j.1460-9568.1998.00142.x
Schiffmann, S. N., Lledo, P. M., and Vincent, J. D. (1995). Dopamine D1 receptor modulates the voltage-gated sodium current in rat striatal neurones through a protein kinase A. J. Physiol. (Lond). 483 (Pt 1), 95–107. doi: 10.1113/jphysiol.1995.sp020570
Schrader, L. A., Anderson, A. E., Mayne, A., Pfaffinger, P. J., and Sweatt, J. D. (2002). PKA modulation of Kv4.2-encoded A-type potassium channels requires formation of a supramolecular complex. J. Neurosci. 22, 10123–10133.
Schultz, W. (2002). Getting formal with dopamine and reward. Neuron 36, 241–263. doi: 10.1016/S0896-6273(02)00967-4
Schultz, W., Dayan, P., and Montague, P. R. (1997). A neural substrate of prediction and reward. Science 275, 1593–1599d. doi: 10.1126/science.275.5306.1593
Schwab, B., van Wezel, R., and van Gils, S. (2017). Sparse pallidal connections shape synchrony in a network model of the basal ganglia. Eur. J. Neurosci. 45, 1000–1012. doi: 10.1111/ejn.13324
Schwarz, J. (1986). The effect of temperature on Na currents in rat myelinated nerve fibres. Pflüg. Archiv. Eur. J. Physiol. 406, 397–404. doi: 10.1007/BF00590943
Seo, D., Patrick, C. J., and Kennealy, P. J. (2008). Role of serotonin and dopamine system interactions in the neurobioloy of impulsive aggression and its comorbidity with other clinical disorders. Aggress. Violent Behav. 13, 383–395. doi: 10.1016/j.avb.2008.06.003
Shen, K. Z., and Johnson, S. W. (2000). Presynaptic dopamine D2 and muscarine M3 receptors inhibit excitatory and inhibitory transmission to rat subthalamic neurones in vitro. J. Physiol. 525(Pt 2), 331–341. doi: 10.1111/j.1469-7793.2000.00331.x
Shen, W., Flajolet, M., Greengard, P., and Surmeier, D. J. (2008). Dichotomous dopaminergic control of striatal synaptic plasticity. Science 321, 848–851. doi: 10.1126/science.1160575
Snyder, G. L., Allen, P. B., Fienberg, A. A., Valle, C. G., Huganir, R. L., Nairn, A. C., et al. (2000). Regulation of phosphorylation of the GluR1 AMPA receptor in the neostriatum by dopamine and psychostimulants in vivo. J. Neurosci. 20, 4480–4488.
Spreizer, S., Angelhuber, M., Bahuguna, J., Aertsen, A., and Kumar, A. (2017). Activity dynamics and signal representation in a striatal network model with distance-dependent connectivity. Eneuro 4, 1–15. doi: 10.1523/ENEURO.0348-16.2017
Staley, J. K., Krishnan-Sarin, S., Cosgrove, K. P., Krantzler, E., Frohlich, E., Perry, E., et al. (2006). Human tobacco smokers in early abstinence have higher levels of β2* nicotinic acetylcholine receptors than nonsmokers. J. Neurosci. 26, 8707–8714. doi: 10.1523/JNEUROSCI.0546-06.2006
Stephenson-Jones, M., Floros, O., Robertson, B., and Grillner, S. (2012). Evolutionary conservation of the habenular nuclei and their circuitry controlling the dopamine and 5-hydroxytryptophan (5-HT) systems. Proc. Natl. Acad. Sci. U.S.A. 109, E164–E173. doi: 10.1073/pnas.1119348109
Stephenson-Jones, M., Kardamakis, A. A., Robertson, B., and Grillner, S. (2013). Independent circuits in the basal ganglia for the evaluation and selection of actions. Proc. Natl. Acad. Sci. U.S.A. 110, E3670–E3679. doi: 10.1073/pnas.1314815110
Stephenson-Jones, M., Yu, K., Ahrens, S., Tucciarone, J. M., van Huijstee, A. N., Mejia, L. A., et al. (2016). A basal ganglia circuit for evaluating action outcomes. Nature 539, 289–293. doi: 10.1038/nature19845
Straub, C., Tritsch, N. X., Hagan, N. A., Gu, C., and Sabatini, B. L. (2014). Multiphasic modulation of cholinergic interneurons by nigrostriatal afferents. J. Neurosci. 34, 8557–8569. doi: 10.1523/JNEUROSCI.0589-14.2014
Stuart, G., and Spruston, N. (1998). Determinants of voltage attenuation in neocortical pyramidal neuron dendrites. J. Neurosci. 18, 3501–3510.
Sullivan, M. A., Chen, H., and Morikawa, H. (2008). Recurrent inhibitory network among striatal cholinergic interneurons. J. Neurosci. 28, 8682–8690. doi: 10.1523/JNEUROSCI.2411-08.2008
Surmeier, D. J., Bargas, J., Hemmings, H. C., Nairn, A. C., and Greengard, P. (1995). Modulation of calcium currents by a D1 dopaminergic protein kinase/phosphatase cascade in rat neostriatal neurons. Neuron 14, 385–397. doi: 10.1016/0896-6273(95)90294-5
Surmeier, D. J., Carrillo-Reid, L., and Bargas, J. (2011). Dopaminergic modulation of striatal neurons, circuits and assemblies. Neuroscience 198, 3–18. doi: 10.1016/j.neuroscience.2011.08.051
Surmeier, D. J., Ding, J., Day, M., Wang, Z., and Shen, W. (2007). D1 and D2 dopamine-receptor modulation of striatal glutamatergic signaling in striatal medium spiny neurons. Trends Neurosci. 30, 228–235. doi: 10.1016/j.tins.2007.03.008
Surmeier, D. J., Eberwine, J., Wilson, C. J., Cao, Y., Stefani, A., and Kitai, S. T. (1992). Dopamine receptor subtypes colocalize in rat striatonigral neurons. Proc. Natl. Acad. Sci. U.S.A. 89, 10178–10182. doi: 10.1073/pnas.89.21.10178
Tachibana, Y., Iwamuro, H., Kita, H., Takada, M., and Nambu, A. (2011). Subthalamo-pallidal interactions underlying parkinsonian neuronal oscillations in the primate basal ganglia. Eur. J. Neurosci. 34, 1470–1484. doi: 10.1111/j.1460-9568.2011.07865.x
Tanaka, S. C., Schweighofer, N., Asahi, S., Shishida, K., Okamoto, Y., Yamawaki, S., et al. (2007). Serotonin differentially regulates short-and long-term prediction of rewards in the ventral and dorsal striatum. PLoS ONE 2:e1333. doi: 10.1371/journal.pone.0001333
Tecuapetla, F., Jin, X., Lima, S. Q., and Costa, R. M. (2016). Complementary contributions of striatal projection pathways to action initiation and execution. Cell 166, 703–715. doi: 10.1016/j.cell.2016.06.032
Tecuapetla, F., Patel, J. C., Xenias, H., English, D., Tadros, I., Shah, F., et al. (2010). Glutamatergic signaling by mesolimbic dopamine neurons in the nucleus accumbens. J. Neurosci. 30, 7105–7110. doi: 10.1523/JNEUROSCI.0265-10.2010
Threlfell, S., Lalic, T., Platt, N. J., Jennings, K. A., Deisseroth, K., and Cragg, S. J. (2012). Striatal dopamine release is triggered by synchronized activity in cholinergic interneurons. Neuron 75, 58–64. doi: 10.1016/j.neuron.2012.04.038
Tkatch, T., Baranauskas, G., and Surmeier, D. J. (2000). Kv4.2 mRNA abundance and A- type K(+) current amplitude are linearly related in basal ganglia and basal forebrain neurons. J. Neurosci. 20, 579–588.
Tomkins, A., Vasilaki, E., Beste, C., Gurney, K., and Humphries, M. D. (2013). Transient and steady-state selection in the striatal microcircuit. Front. Comput. Neurosci. 7:192. doi: 10.3389/fncom.2013.00192
Tong, H., and Gibb, A. J. (2008). Dopamine D1 receptor inhibition of NMDA receptor currents mediated by tyrosine kinase-dependent receptor trafficking in neonatal rat striatum. J. Physiol. (Lond). 586, 4693–4707. doi: 10.1113/jphysiol.2008.158931
Tritsch, N. X., and Sabatini, B. L. (2012). Dopaminergic modulation of synaptic transmission in cortex and striatum. Neuron 76, 33–50. doi: 10.1016/j.neuron.2012.09.023
Tritsch, N. X., Ding, J. B., and Sabatini, B. L. (2012). Dopaminergic neurons inhibit striatal output through non-canonical release of gaba. Nature 490, 262–266. doi: 10.1038/nature11466
Tritsch, N. X., Granger, A. J., and Sabatini, B. L. (2016). Mechanisms and functions of GABA co-release. Nat. Rev. Neurosci. 17, 139–145. doi: 10.1038/nrn.2015.21
Tseng, K. Y., Kasanetz, F., Kargieman, L., Riquelme, L. A., and Murer, M. G. (2001). Cortical slow oscillatory activity is reflected in the membrane potential and spike trains of striatal neurons in rats with chronic nigrostriatal lesions. J. Neurosci. 21, 6430–6439.
Tsodyks, M., Uziel, A., and Markram, H. (2000). Synchrony generation in recurrent networks with frequency-dependent synapses. J. Neurosci. 20:RC50.
Umemiya, M., and Raymond, L. A. (1997). Dopaminergic modulation of excitatory postsynaptic currents in rat neostriatal neurons. J. Neurophysiol. 78, 1248–1255. doi: 10.1152/jn.1997.78.3.1248
Wang, Z., Kai, L., Day, M., Ronesi, J., Yin, H. H., Ding, J., et al. (2006). Dopaminergic control of corticostriatal long-term synaptic depression in medium spiny neurons is mediated by cholinergic interneurons. Neuron 50, 443–452. doi: 10.1016/j.neuron.2006.04.010
Westphal, R. S., Tavalin, S. J., Lin, J. W., Alto, N. M., Fraser, I. D., Langeberg, L., Sheng, M., and Scott, J. (1999). Regulation of NMDA receptors by an associated phosphatase-kinase signaling complex. Science 285, 93–96. doi: 10.1126/science.285.5424.93
Wickens, J. R. (2009). Synaptic plasticity in the basal ganglia. Behav. Brain Res. 199, 119–128. doi: 10.1016/j.bbr.2008.10.030
Wilson, C. J. (2007). GABAergic inhibition in the neostriatum. Prog. Brain Res. 160, 91–110. doi: 10.1016/S0079-6123(06)60006-X
Wolf, J. A., Moyer, J. T., Lazarewicz, M. T., Contreras, D., Benoit-Marand, M., et al. (2005). NMDA/AMPA ratio impacts state transitions and entrainment to oscillations in a computational model of the nucleus accumbens medium spiny projection neuron. J. Neurosci. 25, 9080–9095. doi: 10.1523/JNEUROSCI.2220-05.2005
Xiang, Z., Thompson, A. D., Jones, C. K., Lindsley, C. W., and Conn, P. J. (2012). Roles of the m1 muscarinic acetylcholine receptor subtype in the regulation of basal ganglia function and implications for the treatment of parkinson's disease. J. Pharmacol. Exp. Therapeut. 340, 595–603. doi: 10.1124/jpet.111.187856
Xiong, H., Kovacs, I., and Zhang, Z. (2004). Differential distribution of KChIPs mRNAs in adult mouse brain. Brain Res. Mol. Brain Res. 128, 103–111. doi: 10.1016/j.molbrainres.2004.06.024
Xue, B., Chen, E. C., He, N., Jin, D. Z., Mao, L. M., et al. (2016). Integrated regulation of AMPA glutamate receptor phosphorylation in the striatum by dopamine and acetylcholine. Neuropharmacology. Apr. 112, 57–65. doi: 10.1016/j.neuropharm.2016.04.005
Yagishita, S., Hayashi-Takagi, A., Ellis-Davies, G. C., Urakubo, H., Ishii, S., and Kasai, H. (2014). A critical time window for dopamine actions on the structural plasticity of dendritic spines. Science 345, 1616–1620. doi: 10.1126/science.1255514
Yan, Z., Hsieh-Wilson, L., Feng, J., Tomizawa, K., Allen, P. B., Fienberg, A. A., et al. (1999). Protein phosphatase 1 modulation of neostriatal AMPA channels: regulation by DARPP-32 and spinophilin. Nat. Neurosci. 2, 13–17. doi: 10.1038/4516
Yang, J., Ye, M., Tian, C., Yang, M., Wang, Y., and Shu, Y. (2013). Dopaminergic modulation of axonal potassium channels and action potential waveform in pyramidal neurons of prefrontal cortex. J. Physiol. (Lond). 591, 3233–3251. doi: 10.1113/jphysiol.2013.251058
Yanovsky, Y., Mades, S., and Misgeld, U. (2003). Retrograde signaling changes the venue of postsynaptic inhibition in rat substantia nigra. Neuroscience 122, 317–328. doi: 10.1016/S0306-4522(03)00607-9
Yapo, C., Nair, A. G., Clement, L., Castro, L. R., Hellgren Kotaleski, J., and Vincent, P. (2017). Detection of phasic dopamine by D1 and D2 striatal medium spiny neurons. J. Physiol. 595, 7451–7475. doi: 10.1113/JP274475
Yim, M. Y., Aertsen, A., and Kumar, A. (2011). Significance of input correlations in striatal function. PLoS Comput. Biol. 7:e1002254. doi: 10.1371/journal.pcbi.1002254
Yu, C., Gupta, J., Chen, J. F., and Yin, H. H. (2009). Genetic deletion of A2A adenosine receptors in the striatum selectively impairs habit formation. J. Neurosci. 29, 15100–15103. doi: 10.1523/JNEUROSCI.4215-09.2009
Yu, X., Ye, Z., Houston, C. M., Zecharia, A. Y., Ma, Y., Zhang, Z., et al. (2015). Wakefulness is governed by GABA and histamine cotransmission. Neuron 87, 164–178. doi: 10.1016/j.neuron.2015.06.003
Zhang, X. F., Cooper, D. C., and White, F. J. (2002). Repeated cocaine treatment decreases whole-cell calcium current in rat nucleus accumbens neurons. J. Pharmacol. Exp. Ther. 301, 1119–1125. doi: 10.1124/jpet.301.3.1119
Zhang, X. F., Hu, X. T., and White, F. J. (1998). Whole-cell plasticity in cocaine withdrawal: reduced sodium currents in nucleus accumbens neurons. J. Neurosci. 18, 488–498.
Zhao, B., Zhu, J., Dai, D., Xing, J., He, J., Fu, Z., et al. (2016). Differential dopaminergic regulation of inwardly rectifying potassium channel mediated subthreshold dynamics in striatal medium spiny neurons. Neuropharmacology 107, 396–410. doi: 10.1016/j.neuropharm.2016.03.037
Zhou, F. W., Jin, Y., Matta, S. G., Xu, M., and Zhou, F. M. (2009). An ultra-short dopamine pathway regulates Basal Ganglia output. J. Neurosci. 29, 10424–10435. doi: 10.1523/JNEUROSCI.4402-08.2009
Zhu, Z. T., Shen, K. Z., and Johnson, S. W. (2002). Pharmacological identification of inward current evoked by dopamine in rat subthalamic neurons in vitro. Neuropharmacology 42, 772–781. doi: 10.1016/S0028-3908(02)00035-7
Zia, S., Cody, F. W., and O'Boyle, D. J. (2003). Discrimination of bilateral differences in the loci of tactile stimulation is impaired in subjects with Parkinson's disease. Clin. Anat. 16, 241–247. doi: 10.1002/ca.10100
Keywords: striatum, medium spiny projection neurons, dopamine, simulations, Kv4.2, subcellular signaling, kinetic modeling
Citation: Lindroos R, Dorst MC, Du K, Filipović M, Keller D, Ketzef M, Kozlov AK, Kumar A, Lindahl M, Nair AG, Pérez-Fernández J, Grillner S, Silberberg G and Hellgren Kotaleski J (2018) Basal Ganglia Neuromodulation Over Multiple Temporal and Structural Scales—Simulations of Direct Pathway MSNs Investigate the Fast Onset of Dopaminergic Effects and Predict the Role of Kv4.2. Front. Neural Circuits 12:3. doi: 10.3389/fncir.2018.00003
Received: 10 October 2017; Accepted: 09 January 2018;
Published: 06 February 2018.
Edited by:
Srikanth Ramaswamy, École Polytechnique Fédérale de Lausanne, SwitzerlandReviewed by:
Kelly R. Tan, University of Basel, SwitzerlandCopyright © 2018 Lindroos, Dorst, Du, Filipović, Keller, Ketzef, Kozlov, Kumar, Lindahl, Nair, Pérez-Fernández, Grillner, Silberberg and Hellgren Kotaleski. This is an open-access article distributed under the terms of the Creative Commons Attribution License (CC BY). The use, distribution or reproduction in other forums is permitted, provided the original author(s) and the copyright owner are credited and that the original publication in this journal is cited, in accordance with accepted academic practice. No use, distribution or reproduction is permitted which does not comply with these terms.
*Correspondence: Jeanette Hellgren Kotaleski, amVhbmV0dGVAa3RoLnNl
Disclaimer: All claims expressed in this article are solely those of the authors and do not necessarily represent those of their affiliated organizations, or those of the publisher, the editors and the reviewers. Any product that may be evaluated in this article or claim that may be made by its manufacturer is not guaranteed or endorsed by the publisher.
Research integrity at Frontiers
Learn more about the work of our research integrity team to safeguard the quality of each article we publish.