- 1Lendület Laboratory of Systems Neuroscience, Department of Cellular and Network Neurobiology, Institute of Experimental Medicine – Hungarian Academy of Sciences, Budapest, Hungary
- 2János Szentágothai Doctoral School of Neurosciences, Semmelweis University, Budapest, Hungary
Some neurons are more equal than others: neuroscience relies heavily on the notion that there is a division of labor among different subtypes of brain cells. Therefore, it is important to recognize groups of neurons that participate in the same computation or share similar tasks. However, what the best ways are to identify such collections is not yet clear. Here, we argue that monitoring the activity of genetically defined cell types will lead to new insights about neural mechanisms and improve our understanding of disease vulnerability. Through highlighting how central cholinergic neurons encode reward and punishment that can be captured by a unified framework of reinforcement surprise, we hope to provide an instructive example of how studying a genetically defined cell type may further our understanding of neural function.
Introduction
In 2014 Mahlon DeLong and Alim-Louis Benabid got the Lasker prize for deep brain stimulation, serving as a vivid reminder that one of neuroscience’s greatest clinical success stories was based on diligent – and often tedious – recording of hundreds of basal ganglia neurons from monkeys (DeLong, 1969, 1971; Wichmann and Delong, 2002, 2006). Although those recordings were done in the seventies and eighties, similar electrophysiology experiments are still vital today. For instance, amidst the gloomy mood ensuing the disappointing outcome of the phase 3 clinical trials of anti-amyloid antibodies for treating Alzheimer’s disease, a commentary in Nature asserted that “the biggest clues (for therapy) will come from monitoring collections of neurons" (Kosik, 2013).
As often as not, the devil is in the details: what defines a ‘collection’ of neurons? The way we choose which neuronal pool to study will determine the interpretation and impact of our experiments – and it is usually far from trivial to pick the ‘right’ collection. Indeed, sampling random sets of brain cells results in what was coined the neuronal ‘response zoo’: the perplexing complexity of individual activities lacking clear boundaries and not yielding clear answers.
Multiple ways of Defining ‘Collections’ of Neurons
There are multiple ways of defining more specific – and thus often more useful – collections (Figure 1). These collections may encode ‘low-level’ behavioral information in the form of computationally tractable behavioral variables, like reward expectation, subjective value, expected and unexpected uncertainty, temporal anticipation, etc. (Schultz et al., 1997; Yu and Dayan, 2005; Dayan, 2012). For instance, we can identify groups of neurons that may mediate specific brain functions by selecting them according to their connectivity (Figure 1A). One nice example of such dissociation between hodology-based collections was demonstrated between midbrain dopaminergic neurons with different projection targets (Parker et al., 2016). It was found that while both collections coded reward prediction errors as typical for dopaminergic neurons (Schultz et al., 1997), those projecting to the dorsomedial striatum responded more to contralateral choice, whereas dopamine neurons with projections to the nucleus accumbens responded prominently to reward consumption and reward-predicting cues (Parker et al., 2016). Another study defined collections of midbrain dopaminergic neurons based on their afferent rather than their efferent connectivity (Dautan et al., 2016). Dautan et al. (2016) demonstrated that dopaminergic neurons receiving cholinergic input from the laterodorsal tegmentum were excited, whereas those innervated by the pedunculopontine cholinergic neurons were inhibited by aversive stimuli. The prefrontal cortex (PFC) also represents an important hub of the reward processing circuitry. Projection-specific coding has recently been demonstrated in the PFC: corticostriatal neurons show excitatory, whereas corticothalamic projection neurons develop inhibitory responses to reward-predicting cues during learning (Otis et al., 2017). Bidirectional projection neurons between the PFC and the amygdala also carry out target-specific functions both from PFC to amygdala (Courtin et al., 2013) and vice versa (Senn et al., 2014). In addition, functional differences between corticopontine and commissural PFC neurons were also demonstrated (Dembrow et al., 2010).
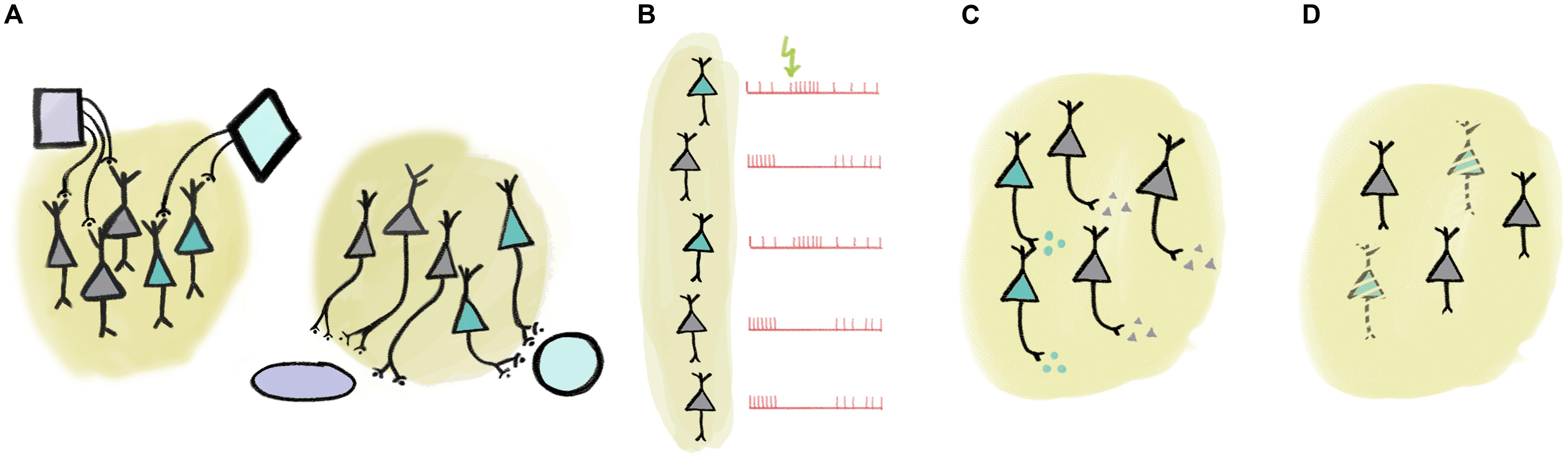
FIGURE 1. Multiple ways of defining ‘collections’ of neurons. (A) Collections of neurons may be defined by input or output connectivity. (B) Response to external or internal events may identify a set of functionally similar neurons. (C) Neurochemical identity (e.g., neurotransmitter profile) defines cell types. (D) Certain cell types may be specifically linked to disease.
Responses to sensory stimulation as well as other characteristic response properties may also be employed to define functional collections (Figure 1B). For instance, there is an association between tone-responsiveness and disinhibition by VIP interneurons in the auditory cortex principal neuron population (Pi et al., 2013). As another example, putative non-cholinergic neurons of the basal forebrain that show stereotypical burst responses to reward-predictive cues share a number of functional properties (Lin and Nicolelis, 2008; Avila and Lin, 2014). Naturally, these defining features may be strongly correlated, in that cells with shared inputs likely have similar response properties and may have overlapping efferent connectivity (Varga et al., 2010; Gielow and Zaborszky, 2017).
One particularly useful trait for cell type identification is by neurochemical profile (Figure 1C). For instance, neurons of a given area that use different neurotransmitters are often distinguished by local and long-range connectivity as well as activity patterns in response to external stimuli or internal variables, lending credibility to the notion of treating them as a functional ‘collection’ (Varga et al., 2010; Do et al., 2016; Gielow and Zaborszky, 2017). Nevertheless, neurotransmitters alone may fall short in delineating practical functional groups. In case of glutamatergic neurons, combination with efferent connectivity (Dembrow et al., 2010; Otis et al., 2017) or more detailed neurochemical identification (Ye et al., 2016) may prove sufficient. In GABAergic interneurons, expression of other genetically defined markers like calcium-binding proteins or specific receptors provide effective genetic handles on functional collections. For instance, interneurons that express the calcium-binding protein parvalbumin show functional homogeneity in PFC (Hartwich et al., 2009; Massi et al., 2012; Kvitsiani et al., 2013; but see Lagler et al., 2016), motor cortex (Isomura et al., 2009), somatosensory cortex (Sachidhanandam et al., 2016), visual cortex (Atallah et al., 2012; Lee et al., 2012; Wilson et al., 2012), auditory cortex (Moore and Wehr, 2013), or hippocampus (Lapray et al., 2012; Viney et al., 2013). An association between projection targets, genetic labels (NPAS4-expression) and coding properties during behavior was elegantly demonstrated in the PFC recently (Ye et al., 2016).
The notion of genetically defined functional collections is underscored by observations of specific loss of neurotransmitter-defined cell types in many neurological diseases (Figure 1D). For instance, Alzheimer’s disease is characterized by the gradual loss of basal forebrain cholinergic neurons (Whitehouse et al., 1982; Arendt and Bigl, 1986). While the atrophy of the same cells is associated with the cognitive symptoms developed in most Parkinson’s patients, the motor symptoms that define the disease are a result of the loss of dopaminergic innervation (Gratwicke et al., 2015). Other examples include the association between the dysfunction of parvalbumin-expressing cortical interneurons and schizophrenia (Sohal et al., 2009; McNally and McCarley, 2016), or narcolepsy caused by the loss of orexin-signaling (Lin et al., 1999; Peyron et al., 2000; Thannickal et al., 2000; Kohlmeier et al., 2013).
Central Cholinergic Neurons as an Example Collection to Study
As mentioned above, Alzheimer’s disease is characterized by the gradual loss of basal forebrain cholinergic neurons. These neurons are situated at the bottom of the forebrain and send extensive projections to all cortical areas (Saper, 1984; Zaborszky et al., 2012, 2013; Do et al., 2016), releasing the neurotransmitter acetylcholine from their terminals. Experiments with selective ablation of cholinergic neurons or pharmacological blockade of acetylcholine suggest that these neurons mediate important cognitive functions, including learning and attention (Everitt and Robbins, 1997; Wrenn and Wiley, 1998; Parikh et al., 2007). Indeed, cognitive deficits in Alzheimer’s patients are strongly correlated with the extent of cholinergic cell loss (Whitehouse et al., 1982; Arendt and Bigl, 1986).
Cholinergic neurons would thus appear like the ideal ‘collection’ of neurons to study; forming an anatomically and neurochemically distinct group, having important functions and a strong relevance to human disease (Figure 2). Given this, it may come as a surprise that the activity of forebrain cholinergic neurons during any behavior is largely unknown. The reason for this has been the lack of tools to specifically probe cholinergic neurons, which are intermingled with more numerous cell types, in awake behaving animals. The game changer is the recent advent of optogenetic methods that allow the expression of light-sensitive ion channels in genetically defined cell types – like those that express synthesizing enzymes for acetylcholine and are therefore cholinergic (Boyden et al., 2005; Rossi et al., 2011). Using optogenetics, one can activate cholinergic neurons by light and in this way identify them while the animal is awake and able to perform learned behaviors.
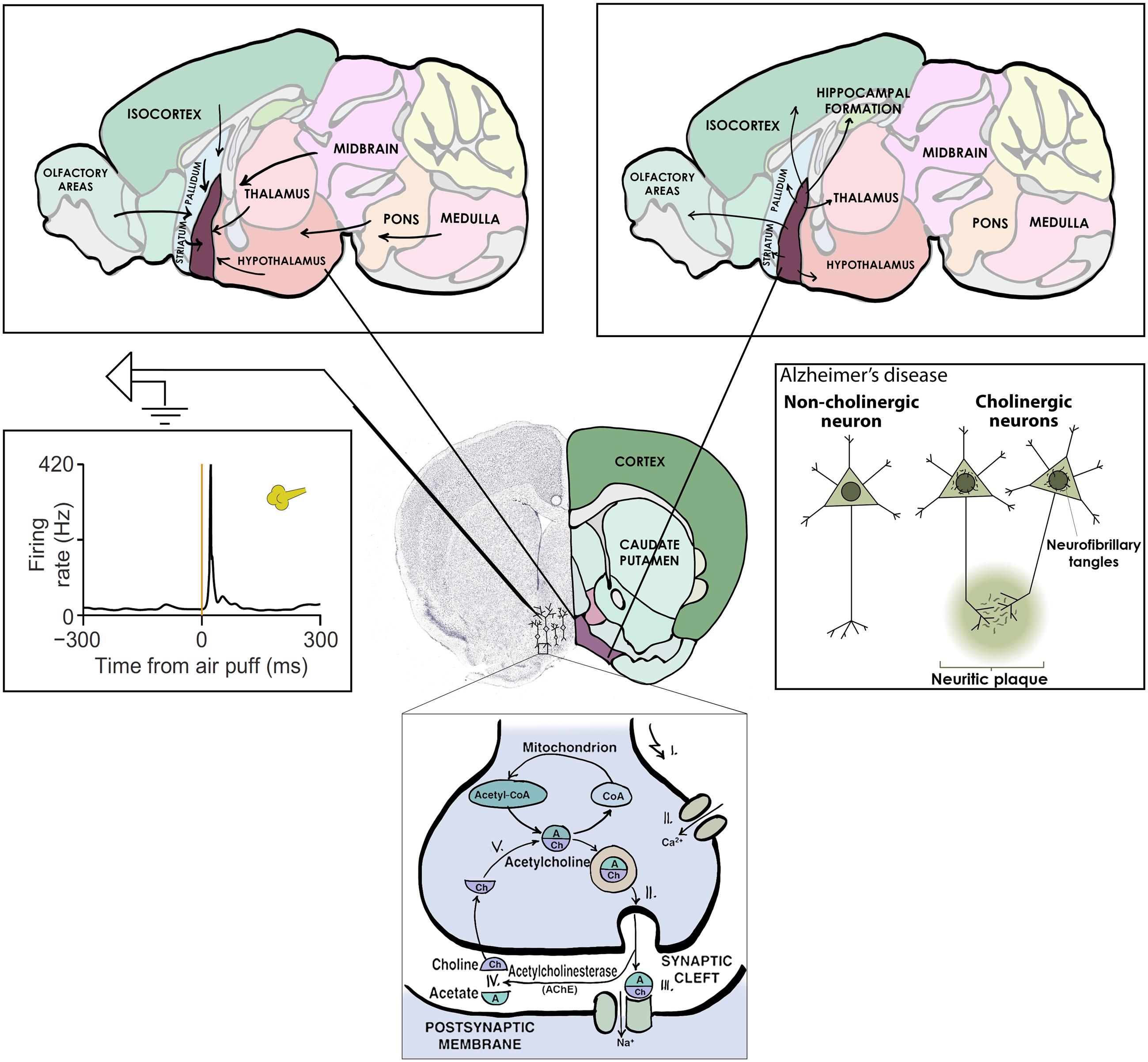
FIGURE 2. Central cholinergic neurons as an example collection to study. Basal forebrain cholinergic neurons release acetylcholine as neurotransmitter (bottom), share afferent (top left) and efferent (top right) connectivity, display uniform activation kinetics in response to aversive air puffs (middle left) and show cell type specific degeneration in Alzheimer’s disease (middle right).
Unique Activity Patterns of Cholinergic Neurons Hint at Functions in Cognition
We and others set out to tackle the long-unanswered question of when cholinergic neurons fire, to reveal how their activity patterns may support various aspects of normal and diseased cognition (Hangya et al., 2015; Harrison et al., 2016). To simultaneously probe aspects of learning and attention, the two leading theories of cholinergic function, we trained mice to respond to unpredictable quiet tones embedded in loud noise – a difficult sensory detection task that involves associative learning and demands sustained attention. To crack the identity of basal forebrain neurons, we turned to optogenetics: we rendered cholinergic neurons light-sensitive by combining the ChAT-Cre mouse line in which Cre-recombinase is expressed in cholinergic neurons with viral vectors that allow Cre-dependent expression of the light-sensitive channelrhodopsin. We placed microelectrodes into the basal forebrain, home to the cholinergic neurons, along with an optical fiber capable of delivering laser light into brain tissue. In our mice, cholinergic neurons, and only those, were activated by blue light, revealing their neurochemical identity. Finding the cholinergic cells still proved to be difficult, as over 90% of the neurons in the sparsely inhabited basal forebrain are non-cholinergic (Gritti et al., 2006).
Previous studies suggested that cholinergic neurons might be involved in controlling attention. First, lesions to basal forebrain cholinergic neurons caused impairments in tasks that require sustained attention like the five-choice serial reaction time task, during which rodents have to detect and respond to visual cues (Everitt and Robbins, 1997; Turchi and Sarter, 1997; Wrenn and Wiley, 1998; McGaughy et al., 2002; Dalley et al., 2004). Second, measures of acetylcholine release suggested that choline-transients appear in association with cue-detections in attention tasks (Parikh et al., 2007; Hasselmo and Sarter, 2011; Howe et al., 2013; Sarter et al., 2014). Third, cholinergic inputs to sensory cortices influence receptive field properties and stimulus tuning of sensory neurons, which may serve as a basis for attentional functions of the cholinergic system (Kilgard and Merzenich, 1998; Disney et al., 2007; Froemke et al., 2007; Herrero et al., 2008; Thiele et al., 2012). If cholinergic neurons controlled attention at a fast, trial-by-trial time scale, we would expect them to fire vigorously when mice anticipate the tone stimuli, that is, when they have to pay the most attention. Furthermore if these neurons indeed regulated attention levels, their stronger activity should foretell faster or more accurate behavioral responses. However, we found that cholinergic neurons, as a collection, did not show these patterns. Nevertheless, we observed gradual changes in the firing rate of cholinergic neurons throughout behavioral sessions, suggesting that attentional regulation by the cholinergic system demonstrated in previous studies may be mediated by slower modulation of cholinergic firing (Lee et al., 2005; Paolone et al., 2012; Teles-Grilo Ruivo et al., 2017).
On the other hand, basal forebrain cholinergic neurons were promptly activated by reward and punishment, with unexpected speed and precision (Figure 2). This finding is consistent with three studies in which calcium imaging or voltammetry was used to track cholinergic responses (Lovett-Barron et al., 2014; Harrison et al., 2016; Teles-Grilo Ruivo et al., 2017); however, the low temporal resolution of these methods prevented the appreciation of the speed of cholinergic firing. Our results are in line with an elegant recent report on the dissociation of tonic and phasic release of acetylcholine using choline-sensitive biosensors (Teles-Grilo Ruivo et al., 2017).
Moreover, we found that the extent of cholinergic activation was proportional to the unexpectedness, or ‘surprise,’ of the behavioral feedback: cells fired more to reward if it was delivered after an ambiguous auditory cue. Such activation patterns support the role of the cholinergic system in controlling learning: after behavioral feedback such as reward and punishment, there is a unique opportunity to form associations between the stimulus perceived, the action performed and the outcome received. This aspect of cholinergic neurons is notably similar to midbrain dopaminergic neurons, which represent reward prediction errors important for reinforcement learning (Schultz et al., 1997; Bayer and Glimcher, 2005). Nevertheless, reward prediction error is defined in the context of cued outcome tasks, therefore a difference in the behavioral paradigms studied prevents a direct comparison of the two cell types. We expect that understanding how these and other neuromodulatory cell types are coordinated to support learning will be an area of intense research in the near future (Ogawa et al., 2014; Varazzani et al., 2015; Matias et al., 2017).
Conclusion
These are only the first steps toward understanding how functional collections of neurons may contribute to behavior. Nevertheless, cholinergic neurons can serve as an instructive example of a genetically defined cell type that broadcasts a computationally tractable behavioral variable. As a next step, it will be important to understand how such ‘low-level’ information coded by cell types is combined into behaviorally relevant complex information, probably mediated by ensembles composed of the appropriate cell types and interconnections. Eventually, the study of genetically defined cell types and cell type-ensembles may lead to one of those elusive clues for future clinical investigations of neurodegenerative diseases.
Author Contributions
BH developed the idea, BH and KS conceived the manuscript, BH wrote the text and KS generated the figures.
Funding
This work was supported by the “Lendület” Program of the Hungarian Academy of Sciences (LP2015-2/2015).
Conflict of Interest Statement
The authors declare that the research was conducted in the absence of any commercial or financial relationships that could be construed as a potential conflict of interest.
Acknowledgments
We are grateful to Sachin P. Ranade, Maja Lorenc, and Adam Kepecs with whom BH conducted his studies on the basal forebrain cholinergic system. We thank Robert A. A. Campbell, Péter Csermely, and Ágnes Drosztmér for comments on the manuscript.
References
Arendt, T., and Bigl, V. (1986). Alzheimer plaques and cortical cholinergic innervation. Neuroscience 17, 277–279.
Atallah, B. V., Bruns, W., Carandini, M., and Scanziani, M. (2012). Parvalbumin-expressing interneurons linearly transform cortical responses to visual stimuli. Neuron 73, 159–170. doi: 10.1016/j.neuron.2011.12.013
Avila, I., and Lin, S.-C. (2014). Distinct neuronal populations in the basal forebrain encode motivational salience and movement. Front. Behav. Neurosci. 8:421. doi: 10.3389/fnbeh.2014.00421
Bayer, H. M., and Glimcher, P. W. (2005). Midbrain dopamine neurons encode a quantitative reward prediction error signal. Neuron 47, 129–141. doi: 10.1016/j.neuron.2005.05.020
Boyden, E. S., Zhang, F., Bamberg, E., Nagel, G., and Deisseroth, K. (2005). Millisecond-timescale, genetically targeted optical control of neural activity. Nat. Neurosci. 8, 1263–1268.
Courtin, J., Chaudun, F., Rozeske, R. R., Karalis, N., Gonzalez-Campo, C., Wurtz, H., et al. (2013). Prefrontal parvalbumin interneurons shape neuronal activity to drive fear expression. Nature 505, 92–96. doi: 10.1038/nature12755
Dalley, J. W., Theobald, D. E., Bouger, P., Chudasama, Y., Cardinal, R. N., and Robbins, T. W. (2004). Cortical cholinergic function and deficits in visual attentional performance in rats following 192 IgG-saporin-induced lesions of the medial prefrontal cortex. Cereb. Cortex 14, 922–932. doi: 10.1093/cercor/bhh052
Dautan, D., Souza, A. S., Huerta-ocampo, I., Valencia, M., Assous, M., Witten, I. B., et al. (2016). Segregated cholinergic transmission modulates dopamine neurons integrated in distinct functional circuits. Nat. Neurosci. 19, 1–14. doi: 10.1038/nn.4335
Dayan, P. (2012). Twenty-five lessons from computational neuromodulation. Neuron 76, 240–256. doi: 10.1016/j.neuron.2012.09.027
DeLong, M. R. (1969). Activity of pallidal neurons in the monkey during movement and sleep. Am. Physiol. Soc. Proc. 12, 207.
Dembrow, N. C., Chitwood, R. A., and Johnston, D. (2010). Projection-specific neuromodulation of medial prefrontal cortex neurons. J. Neurosci. 30, 16922–16937. doi: 10.1523/JNEUROSCI.3644-10.2010
Disney, A. A., Aoki, C., and Hawken, M. J. (2007). Gain modulation by nicotine in macaque v1. Neuron 56, 701–713. doi: 10.1016/j.neuron.2007.09.034
Do, J. P., Xu, M., Lee, S., Chang, W., Zhang, S., Chung, S., et al. (2016). Cell type-specific long-range connections of basal forebrain circuit. Elife 5, 1–17. doi: 10.7554/eLife.13214
Everitt, B. J., and Robbins, T. W. (1997). Central cholinergic systems and cognition. Annu. Rev. Psychol. 48, 649–684. doi: 10.1146/annurev.psych.48.1.649
Froemke, R. C., Merzenich, M. M., and Schreiner, C. E. (2007). A synaptic memory trace for cortical receptive field plasticity. Nature 450, 425–429. doi: 10.1038/nature06289
Gielow, M. R., and Zaborszky, L. (2017). The input-output relationship of the cholinergic basal forebrain. Cell Rep. 18, 1817–1830. doi: 10.1016/j.celrep.2017.01.060
Gratwicke, J., Jahanshahi, M., and Foltynie, T. (2015). Parkinson’s disease dementia: a neural networks perspective. Brain 138, 1454–1476. doi: 10.1093/brain/awv104
Gritti, I., Henny, P., Galloni, F., Mainville, L., Mariotti, M., and Jones, B. E. (2006). Stereological estimates of the basal forebrain cell population in the rat, including neurons containing choline acetyltransferase, glutamic acid decarboxylase or phosphate-activated glutaminase and colocalizing vesicular glutamate transporters. Neuroscience 143, 1051–1064. doi: 10.1016/j.neuroscience.2006.09.024
Hangya, B., Ranade, S. P., Lorenc, M., and Kepecs, A. (2015). Central cholinergic neurons are rapidly recruited by reinforcement feedback. Cell 162, 1155–1168. doi: 10.1016/j.cell.2015.07.057
Harrison, T. C., Pinto, L., Brock, J. R., and Dan, Y. (2016). Calcium imaging of basal forebrain activity during innate and learned behaviors. Front. Neural Circuits 10:36. doi: 10.3389/fncir.2016.00036
Hartwich, K., Pollak, T., and Klausberger, T. (2009). Distinct firing patterns of identified basket and dendrite-targeting interneurons in the prefrontal cortex during hippocampal theta and local spindle oscillations. J. Neurosci. 29, 9563–9574. doi: 10.1523/JNEUROSCI.1397-09.2009
Hasselmo, M. E., and Sarter, M. (2011). Modes and models of forebrain cholinergic neuromodulation of cognition. Neuropsychopharmacology 36, 52–73. doi: 10.1038/npp.2010.104
Herrero, J. L., Roberts, M. J., Delicato, L. S., Gieselmann, M. A., Dayan, P., and Thiele, A. (2008). Acetylcholine contributes through muscarinic receptors to attentional modulation in V1. Nature 454, 1110–1114. doi: 10.1038/nature07141
Howe, W. M., Berry, A. S., Francois, J., Gilmour, G., Carp, J. M., Tricklebank, M., et al. (2013). Prefrontal cholinergic mechanisms instigating shifts from monitoring for cues to cue-guided performance: converging electrochemical and fMRI evidence from rats and humans. J. Neurosci. 33, 8742–8752. doi: 10.1523/JNEUROSCI.5809-12.2013
Isomura, Y., Harukuni, R., Takekawa, T., Aizawa, H., and Fukai, T. (2009). Microcircuitry coordination of cortical motor information in self-initiation of voluntary movements. Nat. Neurosci. 12, 1586–1593. doi: 10.1038/nn.2431
Kilgard, M. P., and Merzenich, M. M. (1998). Cortical map reorganization enabled by nucleus basalis activity. Science 279, 1714–1718. doi: 10.1126/science.279.5357.1714
Kohlmeier, K. A., Tyler, C. J., Kalogiannis, M., Ishibashi, M., Kristensen, M. P., Gumenchuk, I., et al. (2013). Differential actions of orexin receptors in brainstem cholinergic and monoaminergic neurons revealed by receptor knockouts: implications for orexinergic signaling in arousal and narcolepsy. Front. Neurosci. 7:246. doi: 10.3389/fnins.2013.00246
Kvitsiani, D., Ranade, S., Hangya, B., Taniguchi, H., Huang, J. Z., and Kepecs, A. (2013). Distinct behavioural and network correlates of two interneuron types in prefrontal cortex. Nature 498, 363–366. doi: 10.1038/nature12176
Lagler, M., Ozdemir, A. T., Lagoun, S., Malagon-Vina, H., Borhegyi, Z., Hauer, R., et al. (2016). Divisions of identified parvalbumin-expressing basket cells during working memory-guided decision making. Neuron 91, 1390–1401. doi: 10.1016/j.neuron.2016.08.010
Lapray, D., Lasztoczi, B., Lagler, M., Viney, T. J., Katona, L., Valenti, O., et al. (2012). Behavior-dependent specialization of identified hippocampal interneurons. Nat. Neurosci. 15, 1265–1271. doi: 10.1038/nn.3176
Lee, M. G., Hassani, O. K., Alonso, A., and Jones, B. E. (2005). Cholinergic basal forebrain neurons burst with theta during waking and paradoxical sleep. J. Neurosci. 25, 4365–4369. doi: 10.1523/JNEUROSCI.0178-05.2005
Lee, S.-H., Kwan, A. C., Zhang, S., Phoumthipphavong, V., Flannery, J. G., Masmanidis, S. C., et al. (2012). Activation of specific interneurons improves V1 feature selectivity and visual perception. Nature 488, 379–383. doi: 10.1038/nature11312
Lin, L., Faraco, J., Li, R., Kadotani, H., Rogers, W., Lin, X., et al. (1999). The sleep disorder canine narcolepsy is caused by a mutation in the hypocretin (orexin) receptor 2 gene. Cell 98, 365–376.
Lin, S.-C., and Nicolelis, M. A. L. (2008). Neuronal ensemble bursting in the basal forebrain encodes salience irrespective of valence. Neuron 59, 138–149. doi: 10.1016/j.neuron.2008.04.031
Lovett-Barron, M., Kaifosh, P., Kheirbek, M. A., Danielson, N., Zaremba, J. D., Reardon, T. R., et al. (2014). Dendritic inhibition in the hippocampus supports fear learning. Science 343, 857–863. doi: 10.1126/science.1247485
Massi, L., Lagler, M., Hartwich, K., Borhegyi, Z., Somogyi, P., and Klausberger, T. (2012). Temporal dynamics of parvalbumin-expressing axo-axonic and basket cells in the rat medial prefrontal cortex in vivo. J. Neurosci. 32, 16496–16502. doi: 10.1523/JNEUROSCI.3475-12.2012
Matias, S., Lottem, E., Dugué, G. P., and Mainen, Z. F. (2017). Activity patterns of serotonin neurons underlying cognitive flexibility. Elife 6:e20552. doi: 10.7554/eLife.20552
McGaughy, J., Dalley, J. W., Morrison, C. H., Everitt, B. J., and Robbins, T. W. (2002). Selective behavioral and neurochemical effects of cholinergic lesions produced by intrabasalis infusions of 192 IgG-saporin on attentional performance in a five-choice serial reaction time task. J. Neurosci. 22, 1905–1913.
McNally, J. M., and McCarley, R. W. (2016). Gamma band oscillations: a key to understanding schizophrenia symptoms and neural circuit abnormalities. Curr. Opin. Psychiatry 29, 202–210. doi: 10.1097/YCO.0000000000000244
Moore, A. K., and Wehr, M. (2013). Parvalbumin-expressing inhibitory interneurons in auditory cortex are well-tuned for frequency. J. Neurosci. 33, 13713–13723. doi: 10.1523/JNEUROSCI.0663-13.2013
Ogawa, S. K., Cohen, J. Y., Hwang, D., Uchida, N., and Watabe-Uchida, M. (2014). Organization of monosynaptic inputs to the serotonin and dopamine neuromodulatory systems. Cell Rep. 8, 1105–1118. doi: 10.1016/j.celrep.2014.06.042
Otis, J. M., Namboodiri, V. M. K., Matan, A. M., Voets, E. S., Mohorn, E. P., Kosyk, O., et al. (2017). Prefrontal cortex output circuits guide reward seeking through divergent cue encoding. Nature 543, 103–107. doi: 10.1038/nature21376
Paolone, G., Lee, T. M., and Sarter, M. (2012). Time to pay attention: attentional performance time-stamped prefrontal cholinergic activation, diurnality, and performance. J. Neurosci. 32, 12115–12128. doi: 10.1523/JNEUROSCI.2271-12.2012
Parikh, V., Kozak, R., Martinez, V., and Sarter, M. (2007). Prefrontal acetylcholine release controls cue detection on multiple timescales. Neuron 56, 141–154. doi: 10.1016/j.neuron.2007.08.025
Parker, N. F., Cameron, C. M., Taliaferro, J. P., Lee, J., Choi, J. Y., Davidson, T. J., et al. (2016). Reward and choice encoding in terminals of midbrain dopamine neurons depends on striatal target. Nat. Neurosci. 19, 845–854. doi: 10.1038/nn.4287
Peyron, C., Faraco, J., Rogers, W., Ripley, B., Overeem, S., Charnay, Y., et al. (2000). A mutation in a case of early onset narcolepsy and a generalized absence of hypocretin peptides in human narcoleptic brains. Nat. Med. 6, 991–997. doi: 10.1038/79690
Pi, H.-J., Hangya, B., Kvitsiani, D., Sanders, J. I., Huang, Z. J., and Kepecs, A. (2013). Cortical interneurons that specialize in disinhibitory control. Nature 503, 521–524. doi: 10.1038/nature12676
Rossi, J., Balthasar, N., Olson, D., Scott, M., Berglund, E., Lee, C. E., et al. (2011). Melanocortin-4 receptors expressed by cholinergic neurons regulate energy balance and glucose homeostasis. Cell Metab. 13, 195–204. doi: 10.1016/j.cmet.2011.01.010
Sachidhanandam, S., Sermet, B. S., and Petersen, C. C. H. (2016). Parvalbumin-expressing GABAergic neurons in mouse barrel cortex contribute to gating a goal-directed sensorimotor transformation. Cell Rep. 15, 700–706. doi: 10.1016/j.celrep.2016.03.063
Saper, C. B. (1984). Organization of cerebral cortical afferent systems in the rat. II. Magnocellular basal nucleus. J. Comp. Neurol. 222, 313–342. doi: 10.1002/cne.902220302
Sarter, M., Lustig, C., Howe, W. M., Gritton, H., and Berry, A. S. (2014). Deterministic functions of cortical acetylcholine. Eur. J. Neurosci. 39, 1912–1920. doi: 10.1111/ejn.12515
Schultz, W., Dayan, P., and Montague, P. R. (1997). A neural substrate of prediction and reward. Science 275, 1593–1599. doi: 10.1126/science.275.5306.1593
Senn, V., Wolff, S. B. E., Herry, C., Grenier, F., Ehrlich, I., Gründemann, J., et al. (2014). Long-range connectivity defines behavioral specificity of amygdala neurons. Neuron 81, 428–437. doi: 10.1016/j.neuron.2013.11.006
Sohal, V. S., Zhang, F., Yizhar, O., and Deisseroth, K. (2009). Parvalbumin neurons and gamma rhythms enhance cortical circuit performance. Nature 459, 698–702. doi: 10.1038/nature07991
Teles-Grilo Ruivo, L. M., Baker, K. L., Conway, M. W., Kinsley, P. J., Gilmour, G., Phillips, K. G., et al. (2017). Coordinated acetylcholine release in prefrontal cortex and hippocampus is associated with arousal and reward on distinct timescales. Cell Rep. 18, 905–917. doi: 10.1016/j.celrep.2016.12.085
Thannickal, T. C., Moore, R. Y., Nienhuis, R., Ramanathan, L., Gulyani, S., Aldrich, M., et al. (2000). Reduced number of hypocretin neurons in human narcolepsy. Neuron 27, 469–474. doi: 10.1016/S0896-6273(00)00058-1
Thiele, A., Herrero, J. L., Distler, C., and Hoffmann, K.-P. (2012). Contribution of cholinergic and GABAergic mechanisms to direction tuning, discriminability, response reliability, and neuronal rate correlations in macaque middle temporal area. J. Neurosci. 32, 16602–16615. doi: 10.1523/JNEUROSCI.0554-12.2012
Turchi, J., and Sarter, M. (1997). Cortical acetylcholine and processing capacity: effects of cortical cholinergic deafferentation on crossmodal divided attention in rats. Brain Res. Cogn. Brain Res. 6, 147–158.
Varazzani, C., San-Galli, A., Gilardeau, S., and Bouret, S. (2015). Noradrenaline and dopamine neurons in the reward/effort trade-off: a direct electrophysiological comparison in behaving monkeys. J. Neurosci. 35, 7866–7877. doi: 10.1523/JNEUROSCI.0454-15.2015
Varga, C., Lee, S. Y., and Soltesz, I. (2010). Target-selective GABAergic control of entorhinal cortex output. Nat. Neurosci. 13, 822–824. doi: 10.1038/nn.2570
Viney, T. J., Lasztoczi, B., Katona, L., Crump, M. G., Tukker, J. J., Klausberger, T., et al. (2013). Network state-dependent inhibition of identified hippocampal CA3 axo-axonic cells in vivo. Nat. Neurosci. 16, 1802–1811. doi: 10.1038/nn.3550
Whitehouse, P. J., Price, D. L., Struble, R. G., Clark, A. W., Coyle, J. T., and Delon, M. R. (1982). Alzheimer’s disease and senile dementia: loss of neurons in the basal forebrain. Science 215, 1237–1239.
Wichmann, T., and Delong, M. R. (2002). “Neurocircuitry of Parkinson’s Disease,” in Neuropsychopharmacology: The Fifth Generation of Progress?: an Official Publication of the American College of Neuropsychopharmacology, eds K. L. Davis, D. Charney, J. T. Coyle, and C. Nemeroff (Philadelphia, PA: Lippincott Williams & Wilkins), 1761–1779.
Wichmann, T., and Delong, M. R. (2006). Deep brain stimulation for neurologic and neuropsychiatric disorders. Neuron 52, 197–204. doi: 10.1016/j.neuron.2006.09.022
Wilson, N. R., Runyan, C. A., Wang, F. L., and Sur, M. (2012). Division and subtraction by distinct cortical inhibitory networks in vivo. Nature 488, 343–348. doi: 10.1038/nature11347
Wrenn, C. C., and Wiley, R. G. (1998). The behavioral functions of the cholinergic basal forebrain: lessons from 192 IgG-saporin. Int. J. Dev. Neurosci. 16, 595–602.
Ye, L., Allen, W. E., Thompson, K. R., Tian, Q., Hsueh, B., Ramakrishnan, C., et al. (2016). Wiring and molecular features of prefrontal ensembles representing distinct experiences. Cell 165, 1776–1788. doi: 10.1016/j.cell.2016.05.010
Yu, A. J., and Dayan, P. (2005). Uncertainty, neuromodulation, and attention. Neuron 46, 681–692. doi: 10.1016/j.neuron.2005.04.026
Zaborszky, L., Csordas, A., Mosca, K., Kim, J., Gielow, M. R., Vadasz, C., et al. (2013). Neurons in the basal forebrain project to the cortex in a complex topographic organization that reflects corticocortical connectivity patterns: an experimental study based on retrograde tracing and 3d reconstruction. Cereb. Cortex 25, 118–137. doi: 10.1093/cercor/bht210
Keywords: cell types, optogenetics, connectivity, cholinergic, Alzheimer’s disease
Citation: Sviatkó K and Hangya B (2017) Monitoring the Right Collection: The Central Cholinergic Neurons as an Instructive Example. Front. Neural Circuits 11:31. doi: 10.3389/fncir.2017.00031
Received: 26 December 2016; Accepted: 10 April 2017;
Published: 27 April 2017.
Edited by:
Takao K. Hensch, Harvard University, USAReviewed by:
Kuan Hong Wang, National Institute of Mental Health, USAYa-Tang Li, California Institute of Technology, USA
Bruce Thomas Hope, National Institute on Drug Abuse, USA
Copyright © 2017 Sviatkó and Hangya. This is an open-access article distributed under the terms of the Creative Commons Attribution License (CC BY). The use, distribution or reproduction in other forums is permitted, provided the original author(s) or licensor are credited and that the original publication in this journal is cited, in accordance with accepted academic practice. No use, distribution or reproduction is permitted which does not comply with these terms.
*Correspondence: Balázs Hangya, aGFuZ3lhLmJhbGF6c0Brb2tpLm10YS5odQ==