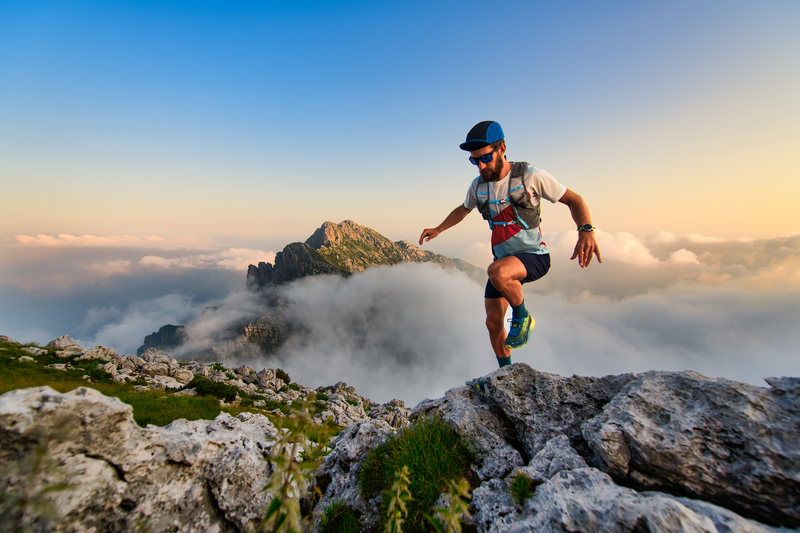
95% of researchers rate our articles as excellent or good
Learn more about the work of our research integrity team to safeguard the quality of each article we publish.
Find out more
REVIEW article
Front. Netw. Physiol.
Sec. Information Theory
Volume 5 - 2025 | doi: 10.3389/fnetp.2025.1551043
The final, formatted version of the article will be published soon.
You have multiple emails registered with Frontiers:
Please enter your email address:
If you already have an account, please login
You don't have a Frontiers account ? You can register here
Cerebral physiological signals embody complex neural, vascular, and metabolic processes that provide valuable insight into the brain's dynamic nature. Profound comprehension and analysis of these signals are essential for unraveling cerebral intricacies, enabling precise identification of patterns and anomalies. Therefore, the advancement of computational models in cerebral physiology is pivotal for exploring the links between measurable signals and underlying physiological states. This review provides a detailed explanation of computational models, including their mathematical formulations, and discusses their relevance to the analysis of cerebral physiology dynamics. It emphasizes the importance of linear multivariate statistical models, particularly autoregressive (AR) models and the Kalman filter, in time series modeling and prediction of cerebral processes. The review focuses on the analysis and operational principles of multivariate statistical models such as AR models and the Kalman filter. These models are examined for their ability to capture intricate relationships among cerebral parameters, offering a holistic representation of brain function. The use of multivariate statistical models enables the capturing of complex relationships among cerebral physiological signals. These models provide valuable insights into the dynamic nature of the brain by representing intricate neural, vascular, and metabolic processes. The review highlights the clinical implications of using computational models to understand cerebral physiology, while also acknowledging the inherent limitations, including the need for stationary data, challenges with high dimensionality, computational complexity, and limited forecasting horizons.
Keywords: Cerebral physiologic signals, Multivariate time-series analysis, computational neuroscience, brain function modeling, Statistical models, state-space models
Received: 07 Jan 2025; Accepted: 03 Apr 2025.
Copyright: © 2025 Vakitbilir, Sainbhi, Islam, Gomez, Stein, Froese, Bergmann, Mcclarty, Raj and Zeiler. This is an open-access article distributed under the terms of the Creative Commons Attribution License (CC BY). The use, distribution or reproduction in other forums is permitted, provided the original author(s) or licensor are credited and that the original publication in this journal is cited, in accordance with accepted academic practice. No use, distribution or reproduction is permitted which does not comply with these terms.
* Correspondence:
Nuray Vakitbilir, Faculty of Graduate Studies, University of Manitoba, Winnipeg, Canada
Disclaimer: All claims expressed in this article are solely those of the authors and do not necessarily represent those of their affiliated organizations, or those of the publisher, the editors and the reviewers. Any product that may be evaluated in this article or claim that may be made by its manufacturer is not guaranteed or endorsed by the publisher.
Research integrity at Frontiers
Learn more about the work of our research integrity team to safeguard the quality of each article we publish.