- 1Department of Neurosurgery, University of Maryland School of Medicine, Baltimore, MD, United States
- 2Surgical Neurology Branch, National Institute of Neurological Disorders, National Institutes of Health, Bethesda, MD, United States
- 3Department of Neurosurgery, Baylor College of Medicine, Houston, TX, United States
Epilepsy is one of the most common neurological disorders, affecting over 65 million people worldwide. Despite medical management with anti-seizure medications (ASMs), many patients fail to achieve seizure freedom, with over one-third of patients having drug-resistant epilepsy (DRE). Even with surgical management through resective surgery and/or neuromodulatory interventions, over 50
1 Introduction
Epilepsy is one of the most common neurological disorders, affecting over 65 million people worldwide (Ngugi et al., 2010). Although first-line management involves over 30 available options (Gunasekera et al., 2023). However, around one-third of patients will fail to achieve seizure control despite extensive pharmacotherapy (Löscher and Klein, 2021). If further workup is pursued in these patients, they typically undergo surgical evaluation in epilepsy centers to localize seizure foci for eventual surgical targeting (i.e., resection, neuromodulation) (Lerche, 2020). Yet, despite direct targeting of suspected epileptogenic zones, over 50
The past two decades have seen an increase in intracranial studies suggesting the presence of pathological seizure networks, see Table 1 (Spencer, 2002; Engel et al., 2013a; Lehnertz et al., 2023). These studies lend pivotal support to the network theory of epilepsy, first proposed in 2002 by Dr. Spencer. She defined seizure networks as functionally and structurally connected circuitry, whereby activity in any one area can affect the entire network (Spencer, 2002). Specifically, she proposed that seizure activity entrains neural networks as a whole, and that the classic thought of focal areas of ictal onset is misrepresentative of the true underlying mechanism of disease. Rather, strongly connected networks underlying seizure propagation result in observed seizure phenomena and the persistence of epileptic disorders. This switch in dogma was reflected in the ILAE’s (International League Against Epilepsy) recent revision to their classification system, now defining focal epilepsy as seizures arising in networks isolated in a single cerebral hemisphere, and generalized epilepsy as seizures that rapidly engage with bilaterally distributed networks (Berg et al., 2010). This understanding of seizures and the role of intracranial networks is supported by studies which show that measures outside of seizure onset localization are important in achieving seizure control (Spencer, 1996; Engel et al., 2013b; Andrews et al., 2019). However, despite this shift in understanding and evidence for seizure networks, we have yet to see this translate to improved treatment options and outcomes in patients with DRE.
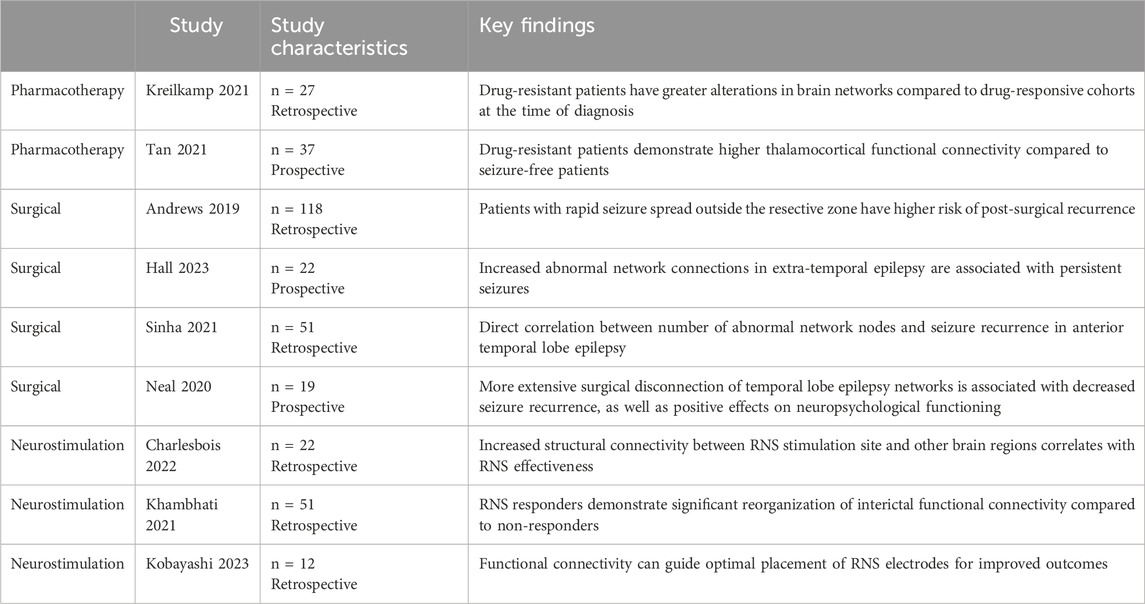
Table 1. This table summarizes studies which lend evidence to support the failure to address the underlying epileptic network as a potential mechanism for treatment failure.
Over the past few years, there have been significant technological advancements, shaping how we treat epilepsy. For example, in 2013, the United States Food and Drug Administration (FDA) approved the first closed-loop neuromodulation device, Responsive Neurostimulation (RNS) made by NeuroPace, that detects electrographic changes preceding seizures and applies counterstimulation to halt seizure activity (Boddeti et al., 2022). However, studies find that the therapeutic benefit seen in some patients is not necessarily due to the immediate seizure disruption, but can be more likely attributed to alterations to chronic network changes (Rao and Rolston, 2023). Although new therapeutic solutions are available, if they are applied using an outdated framework (i.e., epilepsy as a focal disorder), and do not adapt to target seizure networks, seizure outcomes may be suboptimal. Here, we present a review discussing the current state of DRE management, focusing on focal epilepsy due to the larger role of surgical management for focal epilepsy compared to generalized epilepsy (Bullinger et al., 2022). We present recent studies that provide strong evidence of seizure networks in people with epilepsy. Finally, we conclude by discussing how the network theory may guide future surgical epilepsy management.
2 Drug-resistant epilepsy management
As discussed above, patients with seizure disorders are typically managed with ASMs (Gunasekera et al., 2023). However, ASMs will fail to achieve seizure control in approximately one-third of patients, warranting a comprehensive tripartite workup elucidating focal targets for subsequent surgical intervention (Lerche, 2020; Kanner and Bicchi, 2022).
Phase 1 employs non-invasive monitoring through a variety of modalities to identify potential focal targets that may be concordant with a patient’s seizure semiology. These modalities include video scalp-electroencephalography (EEG), neuropsychological testing, and functional neuroimaging, such as positron emission tomography (PET), ictal single photon emission computed tomography (SPECT), and functional magnetic resonance imaging (fMRI) (Munakomi and Das, 2023). Workup progresses to phase 2 if non-invasive tests are inconclusive or identified intracranial regions of interest are discordant with seizure semiology. Phase 2 includes neurosurgical implantation of subdural electrode grids and/or stereotactic depth electrodes (sEEGs) to record directly from cortical/subcortical areas and localize seizure onset regions (Baumgartner et al., 2019). After electrode implantation, patients remain in the epilepsy monitoring unit (EMU) and are weaned off ASMs, to allow for recording of seizure activity that can be used to localize seizure targets and inform subsequent surgical treatment (Bagić et al., 2024).
2.1 Resective/ablative surgery
Surgical intervention is largely dependent on location of seizure onset. To date, clinical evidence strongly supports resective surgery as superior to medical management for focal epilepsy, especially in patients with DRE (Wiebe et al., 2001; Engel et al., 2012). One retrospective study of 284 patients with focal DRE found that 47
2.2 Neuromodulation
However, a subset of patients may be inappropriate candidates for surgical resection. This can be due to a myriad of factors, including seizure foci localizing near eloquent areas, patients presenting with medical comorbidities, or absence of discrete seizure foci, such as may be seen in generalized epilepsy (Rugg et al., 2020; Munakomi and Das, 2023). In these cases, interventions like neuromodulation devices provide effective alternatives to cortical resection (Guery and Rheims, 2021). A recent meta-analysis reported seizure reduction in 64.8
Despite the multitude of surgical interventions, and the success of these treatments, a significant proportion of patients do not achieve seizure freedom. Additionally, the mechanisms of treatment failure are still unclear, however commonly reported factors portending treatment failure are incongruent electrophysiology data, non-lesional epilepsy, and ill-defined epileptic focus (Zhang et al., 2013; Krucoff et al., 2017; Chen et al., 2023). These predictive factors suggest that treatment tends to fail when the seizure network either spatially extends or is functionally connected beyond the epileptic focus. Discordant electrophysiology data and an ill-defined epileptic focus suggests perhaps more than one epileptic focus, as would be seen in an epileptic network; non-lesional epilepsy suggests either a structural etiology at too small of a resolution or epileptic activity too diffusely distributed that there is no identifiable lesion on standard workup. Given that many patients continue to experience seizures despite aggressive surgical management, it is imperative to understand the underlying causes of treatment failure.
3 Mechanisms of treatment failure
All seizure therapies, from ASMs to surgical intervention, struggle to target the underlying epileptic network, acting either too broadly or too focally. Pharmacotherapy reduces seizure activity but fails to address underlying pathologic network alterations. In fact, the recent push towards defining these medications as “anti-seizure” rather than “anti-epileptic” stems from a recognition that these therapies provide symptomatic treatment only and have not been found to alter the disease course (Löscher and Klein, 2021). Further research aimed at understanding the molecular basis of network formation, as well as developing drugs that target these mechanisms directly, is certainly warranted. Prior studies have shown a correlation between pharmacoresistance and a higher number of alterations across widespread brain networks, compared to the drug-responsive cohort (Kreilkamp et al., 2021; Tan et al., 2021; Sinha et al., 2022). This suggests that patients with DRE have altered networks that persist beyond the management of the anti-seizure medication, though the order of causality - whether network changes worsen due to ineffective medication or networks are inherently unresponsive to the medication - is presently unknown. Network reorganization through adaptive neural processes can reinforce the formation of these abnormal networks (Loscher et al., 2020). Prior studies have shown that hippocampal sclerosis in focal temporal lobe epilepsy is associated with ASM resistance, suggesting that these pathological changes may contribute to ASM treatment failure by altering ASM targets in the brain, leading to reduced drug sensitivity (Loscher et al., 2020). Addressing the broader network alterations in drug-resistant patients may elucidate differences in disease pathophysiology or potential treatment targets avenues for improved outcomes.
In contrast to the broad effects of ASMs, resective surgery targets removal of defined epileptogenic foci. However, this focal approach can prove insufficient. One retrospective study of 57 patients with focal DRE found four major causes of treatment failure: erroneous identification of the epileptogenic foci during workup, intraoperative mistakes in identifying or guidance to the resection zone, generation of new epileptogenic areas, and incomplete resection due to functional limitations (González-Martínez et al., 2007). These findings can in part be explained by an epileptic network which is incompletely identified or targeted with resection. In 2019, Andrews et al. conducted a retrospective study of ECoG recordings obtained from patients with focal DRE during neuromonitoring (Andrews et al., 2019). They found that patients with temporal lobe epilepsy who experienced significantly poorer post-operative seizure outcomes had resections that did not include cortical areas of rapid seizure spread, determined by intracranial EEG (
4 Identifying and targeting seizure networks
In translating a network approach to clinical practice, the first challenge is to accurately identify the target epileptic network. Imaging to detect seizure networks can be broadly categorized as either structural or functional imaging. Structural imaging depicts the physical connections within a neural network, such as through tractography (Guo et al., 2024). Functional imaging reveals the temporal associations in neuronal activation between different regions of the brain that comprise a network; examples of this include EEG, fMRI, MEG, and PET (Guo et al., 2024). Each modality has advantages and disadvantages, and concerted use can compensate for limitations (Guo et al., 2024). One case report found sustained seizure control after a second resective procedure for non-lesional frontal lobe epilepsy. Analysis of network hubs - defined as regions that play crucial roles in network communication, identified by several different imaging modalities including sEEG, fMRI, and diffusion tensor imaging (DTI) - demonstrated that the second surgery’s resective zone included highly connected hubs that were spared by the first resection (Gong et al., 2024). This underscores the importance of adopting a multimodal approach to surgical planning to adequately identify significant nodes within a seizure network, thereby optimizing seizure reduction outcomes.
Current presurgical planning largely relies on capturing seizure events during invasive and/or noninvasive EEG recordings (Baumgartner et al., 2019). This approach requires significant recording time and may fail to identify suitable targets (Pondal-Sordo et al., 2007; Shah and Mittal, 2014). The recent evidence suggesting seizure activity on EEG can arise from any number of critical nodes in the seizure network may explain neuromonitoring findings that are, at times, discordant with a patient’s seizure semiology (McGonigal, 2020). This implies that a recording-based approach would require capture of many seizure events to characterize the full seizure network (Liu et al., 2022). On the other hand, network-based localization may compensate for these limitations. For example, studies have shown that interictal functional connectivity (FC), determined by EEG, can be used to characterize the seizure network (Lagarde et al., 2022; Shamas et al., 2022; Rijal et al., 2023). As such, it may be possible to identify seizure networks from baseline interictal recordings, obviating the need to capture multiple seizure events. Perhaps a network-based approach to presurgical planning may extend to include measurements of interictal functional connectivity, obtained during the phase 2 SEEG mapping, in order to better characterize the seizure network and aid in precise surgical targeting.
Building on these insights, recent studies have supported the use of additional imaging techniques to elucidate seizure networks to understand post-ablative or post-resective results. For instance, MRgLITT outcomes have been shown to depend on functional connectivity, as measured on resting-state fMRI, to the ablative region. Ablations leading to seizure freedom exhibit more localized functional connectivity compared to ablations with poorer outcomes in patients with focal epilepsy (Mithani et al., 2019). This study also found that the intrinsic connectivity, from resting-state fMRI, of the ablative region was more predictive of post-surgical outcome than the specific anatomical location of the ablative surgery (Mithani et al., 2019). This suggests the potential utility of network characterization for presurgical planning of ablative procedures to ensure sufficient disruption of the seizure network and consequently to improve outcomes.
Additionally, taking a network approach to imaging before neuromodulation may improve therapeutic efficacy (Piper et al., 2022). Currently, RNS targets the epileptic focus identified from pre-operative mapping studies. Studies have recently demonstrated that assessing preoperative seizure networks can prognosticate neurostimulation response, suggesting the possibility of using individual circuit dynamics to help tailor treatments more effectively (Fan et al., 2022; Scheid et al., 2022). One study looked at direct involvement of the network to assess the correlation with treatment response. They found that increased structural connectivity, measured from patient-specific DTI tractography based on diffusion MRI, between the stimulation site and other brain regions correlated with the effectiveness of RNS treatment for patients with focal epilepsy (Charlebois et al., 2022). This suggests that the success of neuromodulation may depend on how stimulation connects to and thereby influences brain networks linked to the stimulation site. Moreover, a recent study found that RNS responders demonstrated significant reorganization of interictal functional connectivity, as determined by intracranial EEG, within neural networks compared to non-responders, suggesting that better treatment response is associated with more effective changes to the network (Khambhati et al., 2021). Additionally, a new study looked at cortico-cortical evoked potentials from intracranial EEG in patients with focal epilepsy and RNS implants and showed that RNS outcomes correlated with placement of electrodes near receiver or projection nodes within the seizure network. This suggests that analysis of functional connectivity may help guide optimal placement of RNS electrodes to improve outcomes, rather than relying solely on ictal electrophysiological changes as is the current standard (Kobayashi et al., 2023).
In the context of deep brain stimulation (DBS), targeting the thalamus is thought to disrupt ictal propagation through prevention of seizure spread to upstream cortical sites, lending support for the notion that the broader network is an important target for epilepsy treatment (Li and Yang, 2017). Emphasizing DBS targets which most greatly impact the network - either due to the target region being highly connected to the rest of the network or the site where multiple circuits converge - may provide an avenue for potential new neuromodulatory targets (Li and Yang, 2017). It is worth noting that while thalamic DBS is intended as a network-based therapy, long-term clinical trials comparing thalamic DBS to RNS, with focal targets, have virtually identical outcomes (Yang et al., 2022). This inefficacy may perhaps be in part due to the lack of personalized seizure network identification, wherein the thalamic nucleus that best disrupts the network may vary between patients. Similarly, a network approach may serve to better understand and better utilize vagal nerve stimulation (VNS). Currently relegated to an adjuvant therapy in generalized DRE, VNS has been FDA approved for over 25 years yet still the mechanism of action remains unclear (Carron et al., 2023). It is believed that VNS modulates a complex network of communication between the brainstem and cerebrum, desynchronizing epileptiform activity (Hachem et al., 2018). Studies have demonstrated that modulation of specific network connections, such as thalamocortical connections, are associated with higher response rates to VNS, underscoring the importance of targeting the entire seizure network for an effective therapeutic response. These studies underline the need to assess the epileptic network and tailor the stimulation site of neuromodulation to effectively perturb the personalized network at large.
Modifying current pre-surgical evaluations may be the next step in a network-guided approach. For instance, current sEEG mapping involves detecting a seizure focus, but instead, efforts should be shifted more broadly to emphasize identification of the network (Piper et al., 2022). One recent paper reported using sEEG data to construct a seizure network model to identify network-relevant surgical targets for RNS, LITT, and resection in patients with focal epilepsy (Peng et al., 2023). They defined seizure network nodes as the highest ranked sEEG contact points by linear discriminant analysis, a supervised machine learning algorithm. They then described the seizure network as the connections between these nodes, as calculated by DTF-based edge assignment. This model was tested on 10 patients retrospectively, demonstrating both consistency between the model’s recommendations and the clinical decisions that were made. This supports the use of network models to aid in identification of relevant network nodes to augment therapeutic efficacy. Recent studies have supported the use of novel modality combinations, such as hybrid PET/MRI/MEG, to improve the detection of epileptic foci in patients with focal DRE (Guo et al., 2024). Additionally, using advanced techniques, such as graph theory, might unveil particular nodes of significance within the network (Piper et al., 2022). Graph theory utilizes mathematical techniques to characterize structural and functional connections of brain regions at multiple topographical levels (Bernhardt et al., 2015). While few studies have explored the clinical applicability of graph theory analyses based on ECoG recordings to characterize the seizure network, preliminary studies have found evidence of the power of connectivity techniques in predicting post-surgical outcomes for patients with focal epilepsy (Wilke et al., 2011). This suggests the potential utility of structural and functional connectivity analyses which may be able to determine not only areas of connectivity to epileptogenic zones but also the dynamics of how these regions influence one another in the broader context of the network (Figure 1). A multimodal imaging and analytical approach may provide additional insights into the epileptogenic network and may be useful for pre-surgical planning in the future. Once a comprehensive picture of the seizure network is identified, surgical targets may shift from the removal of the seizure onset zone, and with it the entire lobe or implicated region of the brain, and instead towards removal of designated seizure network nodes, or locations that are crucial to sustaining the pathologic network. In this way, network-based epilepsy surgery may minimize damage to healthy brain tissue while simultaneously improving patient outcomes by specifically targeting the critical nodes within the network that propagate seizures.
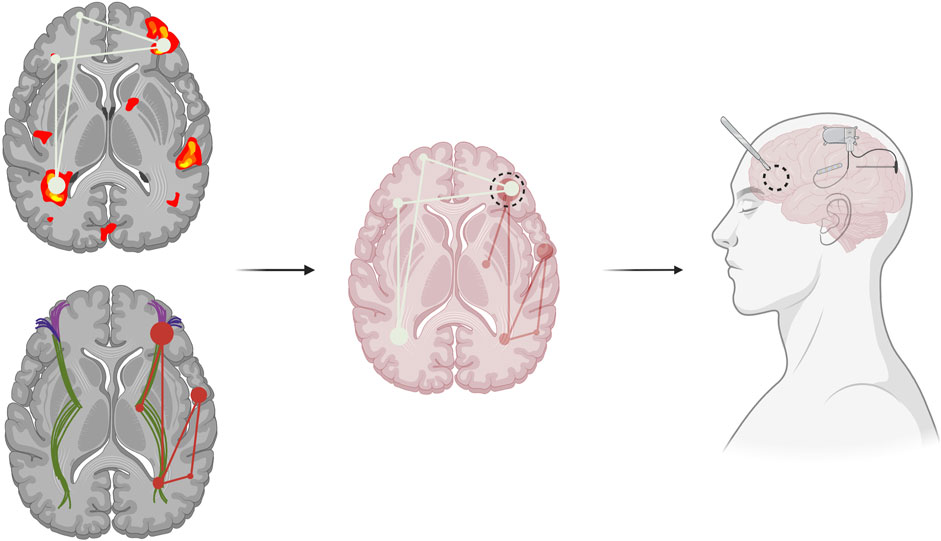
Figure 1. Functional (top left) and structural (bottom left) connectivity can identify seizure networks. Combining various imaging modalities can help locate relevant seizure network hubs, central areas of seizure network activity or communication, which can inform pre-surgical epilepsy planning (middle). Modification of current surgical techniques to more precisely target the identified epileptic network, such as through combined resection with neuromodulation, including the use of the Responsive Neurostimulation System (RNS), of additional seizure network foci (right).
Advances in surgical techniques may augment network-based surgical targeting. For instance, while resective surgery remains the primary treatment modality for focal epilepsy, patients with multiple seizure foci may warrant a complementary approach with responsive neurostimulation in these critical brain regions inadequately impacted by resection (Salama et al., 2024). One retrospective study has found an improved seizure reduction of 81
5 Conclusion
Epilepsy research over the last few decades has featured a paradigm shift in the pathophysiological understanding of epilepsy from a focal to a network-based disorder. Despite increasing evidence supporting the concept of seizure networks, these findings have not translated to adaptation of current medical and surgical management. Recent studies have implicated a failure to sufficiently modulate the epileptic network as a potential reason for the inefficacy of both pharmacotherapeutic and surgical treatments. Identifying new ways to elucidate the seizure network, such as through combinations of imaging techniques to pinpoint critical nodes, may be useful to help guide presurgical planning. Modification of current surgical techniques with a network-based approach may offer significant strides in seizure freedom rates and improved quality of life for patients with drug-resistant epilepsy.
Author contributions
JL: Writing–original draft, Writing–review and editing, Conceptualization. UB: Conceptualization, Writing–original draft, Writing–review and editing. MK: Writing–original draft, Writing–review and editing. ZK: Writing–original draft, Writing–review and editing. IR: Writing–review and editing. MB: Supervision, Writing–review and editing. AK: Supervision, Writing–review and editing, Conceptualization.
Funding
The author(s) declare that no financial support was received for the research, authorship, and/or publication of this article.
Conflict of interest
The authors declare that the research was conducted in the absence of any commercial or financial relationships that could be construed as a potential conflict of interest.
Publisher’s note
All claims expressed in this article are solely those of the authors and do not necessarily represent those of their affiliated organizations, or those of the publisher, the editors and the reviewers. Any product that may be evaluated in this article, or claim that may be made by its manufacturer, is not guaranteed or endorsed by the publisher.
References
Andrews, J. P., Gummadavelli, A., Farooque, P., Bonito, J., Arencibia, C., Blumenfeld, H., et al. (2019). Association of seizure spread with surgical failure in epilepsy. JAMA neurol. 76, 462–469. issn: 2168-6157. doi:10.1001/jamaneurol.2018.4316
Bagić, A. I., Ahrens, S. M., Chapman, K. E., Bai, S., Clarke, D. F., Eisner, M., et al. (2024). Epilepsy monitoring unit practices and safety among NAEC epilepsy centers: a census survey. Epilepsy & Behav. 150, 109571. issn: 1525-5050. doi:10.1016/j.yebeh.2023.109571
Barba, C., Rheims, S., Minotti, L., Guénot, M., Hoffmann, D., Chabardès, S., et al. (2016). Temporal plus epilepsy is a major determinant of temporal lobe surgery failures. Brain 139, 444–451. issn: 0006-8950. doi:10.1093/brain/awv372
Baumgartner, C., Koren, J. P., Britto-Arias, M., Zoche, L., and Pirker, S. (2019). Presurgical epilepsy evaluation and epilepsy surgery. F1000Research 8, F1000 Faculty Rev-1818. issn: 20461402. doi:10.12688/f1000research.17714.1
Berg, A. T., Berkovic, S. F., Brodie, M. J., Buchhalter, J., Cross, J. H., van Emde Boas, W., et al. (2010). Revised terminology and concepts for organization of seizures and epilepsies: report of the ILAE Commission on Classification and Terminology, 2005–2009. Epilepsia 51, 676–685. issn: 1528-1167. doi:10.1111/j.1528-1167.2010.02522.x
Bernhardt, B. C., Bonilha, L., and Gross, D. W. (2015). Network analysis for a network disorder: the emerging role of graph theory in the study of epilepsy. Epilepsy & Behav. 50, 162–170. issn: 1525-5050. doi:10.1016/j.yebeh.2015.06.005
Boddeti, U., McAfee, D., Khan, A., Bachani, M., and Ksendzovsky, A. (2022). Responsive neurostimulation for seizure control: current status and future directions. Biomedicines 10, 2677. issn: 2227-9059. doi:10.3390/biomedicines10112677
Bullinger, K. L., Alwaki, A., and Gross, R. E. (2022). Surgical treatment of drug-resistant generalized epilepsy. Curr. neurology Neurosci. Rep. 22, 459–465. issn: 1534-6293. doi:10.1007/s11910-022-01210-w
Carron, R., Roncon, P., Lagarde, S., Dibué, M., Zanello, M., and Bartolomei, F. (2023). Latest views on the mechanisms of action of surgically implanted cervical vagal nerve stimulation in epilepsy. Neuromodulation Technol. A. T. Neural Interface 26, 498–506. issn: 1094-7159. doi:10.1016/j.neurom.2022.08.447
Charlebois, C. M., Anderson, D. N., Johnson, K. A., Philip, B. J., Davis, T. S., Newman, B. J., et al. (2022). Patient-specific structural connectivity informs outcomes of responsive neurostimulation for temporal lobe epilepsy. Epilepsia 63, 2037–2055. issn: 1528-1167. doi:10.1111/epi.17298
Chen, J. S., Lamoureux, A. A., Shlobin, N. A., Elkaim, L. M., Wang, A., Ibrahim, G. M., et al. (2023). Magnetic resonance-guided laser interstitial thermal therapy for drug-resistant epilepsy: a systematic review and individual participant data meta-analysis. Epilepsia 64, 1957–1974. issn: 1528-1167. doi:10.1111/epi.17560
Engel, J., McDermott, M. P., Wiebe, S., Langfitt, J. T., Stern, J. M., Dewar, S., et al. (2012). Early surgical therapy for drug-resistant temporal lobe epilepsy: a randomized trial. JAMA 307, 922–930. issn: 1538-3598. doi:10.1001/jama.2012.220
Engel, J., Thompson, P. M., Stern, J. M., Staba, R. J., Bragin, A., and Mody, I. (2013a). Connectomics and epilepsy. Curr. Opin. Neurology 26, 186–194. issn: 13507540. doi:10.1097/WCO.0b013e32835ee5b8
Engel, J., Wiebe, S., Radhakrishnan, K., Chitra, S., and Palmini, A. (2013b). Surgical treatment for epilepsy. Neurol. (Wien) 12, 2223–0629.
Fan, J. M., Lee, A. T., Kudo, K., Ranasinghe, K. G., Morise, H., Findlay, A. M., et al. (2022). Network connectivity predicts effectiveness of responsive neurostimulation in focal epilepsy. Brain Commun. 4, fcac104. issn: 26321297. doi:10.1093/braincomms/fcac104
Gong, R., Bickel, S., Tostaeva, G., Lado, F. A., Metha, A. D., Kuzniecky, R. I., et al. (2024). Optimizing surgical planning for epilepsy patients with multimodal neuroimaging and neurophysiology integration: a case study. J. Clin. neurophysiology 41, 317–321. doi:10.1097/WNP.0000000000001071
González-Martínez, J. A., Srikijvilaikul, T., Nair, D., and Bingaman, W. E. (2007). Long-term seizure outcome in reoperation after failure of epilepsy surgery. Neurosurgery 60, 873–880. issn. doi:10.1227/01.NEU.0000255438.13871.FA
Guery, D., and Rheims, S. (2021). Clinical management of drug resistant epilepsy: a review on current strategies. Neuropsychiatric Dis. Treat. 17, 2229–2242. issn: 1176-6328. doi:10.2147/NDT.S256699
Gunasekera, C. L., Sirven, J. I., and Feyissa, A. M. (2023). The evolution of antiseizure medication therapy selection in adults: is artificial intelligence -assisted antiseizure medication selection ready for prime time? J. central Nerv. Syst. Dis. 15, 11795735231209209. doi:10.1177/11795735231209209
Guo, Y., Lin, Z., Fan, Z., and Tian, X. (2024). Epileptic brain network mechanisms and neuroimaging techniques for the brain network. Neural Regen. Res. 19, 2637–2648. issn: 18767958. doi:10.4103/1673-5374.391307
Hachem, L. D., Wong, S. M., and Ibrahim, G. M. (2018). The vagus afferent network: emerging role in translational connectomics. Neurosurg. focus 45, E2. issn: 1092-0684. doi:10.3171/2018.6.FOCUS18216
Hall, G. R., Hutchings, F., Horsley, J., Simpson, C. M., Wang, Y., de Tisi, J., et al. (2023). Epileptogenic networks in extra temporal lobe epilepsy. Netw. Neurosci. 7, 1351–1362. issn: 24721751. doi:10.1162/netn_a_00327
Haneef, Z., and Skrehot, H. C. (2023). Neurostimulation in generalized epilepsy: a systematic review and meta-analysis. Epilepsia 64, 811–820. issn: 1528-1167. doi:10.1111/epi.17524
Kanner, A. M., and Bicchi, M. M. (2022). Antiseizure medications for adults with epilepsy: a review. JAMA 327, 1269–1281. issn: 1538-3598. doi:10.1001/jama.2022.3880
Khambhati, A. N., Shafi, A., Rao, V. R., and Chang, E. F. (2021). Long-term brain network reorganization predicts responsive neurostimulation outcomes for focal epilepsy. Sci. Transl. Med. 13 (608), eabf6588. doi:10.1126/scitranslmed.abf6588
Kobayashi, K., Taylor, K. N., Shahabi, H., Krishnan, B., Joshi, A., Mackow, M. J., et al. (2023). Effective connectivity relates seizure outcome to electrode placement in responsive neurostimulation. Brain Commun. 6, fcae035. issn: 26321297. doi:10.1093/braincomms/fcae035
Kreilkamp, B. A., McKavanagh, A., Alonazi, B., Bryant, L., Das, K., Wieshmann, U. C., et al. (2021). Altered structural connectome in non-lesional newly diagnosed focal epilepsy: relation to pharmacoresistance. NeuroImage. Clin. 29, 102564. doi:10.1016/j.nicl.2021.102564
Krucoff, M. O., Chan, A. Y., Harward, S. C., Rahimpour, S., Rolston, J. D., Muh, C., et al. (2017). Rates and predictors of success and failure in repeat epilepsy surgery: a meta-analysis and systematic review. Epilepsia 58, 2133–2142. issn: 1528-1167. doi:10.1111/epi.13920
Lagarde, S., Bénar, C. G., Wendling, F., and Bartolomei, F. (2022). Interictal functional connectivity in focal refractory epilepsies investigated by intracranial EEG. Brain connect. 12, 850–869. issn: 2158-0022. doi:10.1089/brain.2021.0190
Lehnertz, K., Bröhl, T., and von Wrede, R. (2023). Epileptic-network-based prediction and control of seizures in humans. Neurobiol. Dis. 181, 106098. issn: 0969-9961. doi:10.1016/j.nbd.2023.106098
Lerche, H. (2020). Drug-resistant epilepsy — time to target mechanisms. Nat. Rev. Neurol. 16 (11), 595–596. issn: 1759-4766 (11 Oct. 2020). doi:10.1038/s41582-020-00419-y
Li, D. H., and Yang, X. F. (2017). Remote modulation of network excitability during deep brain stimulation for epilepsy. Seizure - Eur. J. Epilepsy 47, 42–50. issn: 1059-1311. doi:10.1016/j.seizure.2017.02.016
Liu, X., Hu, L., Wang, S., and Shen, J. (2022). Localization of seizure onset zone with epilepsy propagation networks based on graph convolutional network. Biomed. Signal Process. Control 74, 103489. issn: 1746-8094. doi:10.1016/j.bspc.2022.103489
Löscher, W., and Klein, P. (2021). The pharmacology and clinical efficacy of antiseizure medications: from bromide salts to cenobamate and beyond. CNS Drugs 35, 935–963. issn: 11791934 (9 Sept. doi:10.1007/s40263-021-00827-8
Loscher, W., Potschka, H., Sisodiya, S. M., and Vezzani, A. (2020). Drug resistance in epilepsy: clinical impact, potential mechanisms, and new innovative treatment options. Pharmacol. Rev. 72, 606–638. issn: 1521-0081. doi:10.1124/pr.120.019539
McGonigal, A. (2020). Semiology and epileptic networks. Neurosurg. Clin. N. Am. 31, 373–385. issn: 1558-1349. doi:10.1016/j.nec.2020.03.003
Mithani, K., Boutet, A., Germann, J., Elias, G. J. B., Weil, A. G., Shah, A., et al. (2019). Lesion network localization of seizure freedom following MR-guided laser interstitial thermal ablation. Sci. Rep. 9 (1), 18598. issn: 2045-2322 (1 Dec. 2019). doi:10.1038/s41598-019-55015-y
Mohan, M., Keller, S., Nicolson, A., Biswas, S., Smith, D., Osman Farah, J., et al. (2018). The long-term outcomes of epilepsy surgery. PLoS ONE 13 (5), e0196274. doi:10.1371/journal.pone.0196274
Munakomi, S., and Das, J. M. (2023). “Epilepsy surgery,” in StatPearls (Treasure Island (FL): StatPearls Publishing).
Nair, D. R., Laxer, K. D., Weber, P. B., Murro, A. M., Park, Y. D., Barkley, G. L., et al. (2020). Nine-year prospective efficacy and safety of brain-responsive neurostimulation for focal epilepsy. Neurology 95, E1244–E1256. issn. doi:10.1212/WNL.0000000000010154
Neal, E. G., Maciver, S., Schoenberg, M. R., and Vale, F. L. (2020). Surgical disconnection of epilepsy network correlates with improved outcomes. Seizure 76, 56–63. issn: 1059-1311. doi:10.1016/j.seizure.2020.01.018
Ngugi, A. K., Bottomley, C., Kleinschmidt, I., Sander, J. W., and Newton, C. R. (2010). Estimation of the burden of active and life-time epilepsy: a meta-analytic approach. Epilepsia 51, 883–890. issn: 1528-1167. doi:10.1111/j.1528-1167.2009.02481.x
Peng, G., Nourani, M., Dave, H., and Harvey, J. (2023). SEEG-based epileptic seizure network modeling and analysis for pre-surgery evaluation. Comput. Biol. Med. 167, 107692. issn: 0010-4825. doi:10.1016/j.compbiomed.2023.107692
Piper, R. J., Richardson, R. M., Worrell, G., Carmichael, D. W., Baldeweg, T., Litt, B., et al. (2022). Towards network-guided neuromodulation for epilepsy. Brain 145, 3347–3362. issn: 14602156. doi:10.1093/brain/awac234
Pondal-Sordo, M., Diosy, D., Téllez-Zenteno, J. F., Sahjpaul, R., and Wiebe, S. (2007). Usefulness of intracranial EEG in the decision process for epilepsy surgery. Epilepsy Res. 74, 176–182. issn: 0920-1211. doi:10.1016/j.eplepsyres.2007.03.011
Rao, V. R., and Rolston, J. D. (2023). Unearthing the mechanisms of responsive neurostimulation for epilepsy. Commun. Med. 3 (1 Nov), 166. doi:10.1038/s43856-023-00401-x
Rijal, S., Corona, L., Perry, M. S., Tamilia, E., Madsen, J. R., Stone, S. S. D., et al. (2023). Functional connectivity discriminates epileptogenic states and predicts surgical outcome in children with drug resistant epilepsy. Sci. Rep. 13 (1), 9622. issn: 2045-2322 (1 June 2023). doi:10.1038/s41598-023-36551-0
Rugg-Gunn, F., Miserocchi, A., and McEvoy, A. (2020). Epilepsy surgery. Pract. Neurol. 20, 4–14. issn: 1474-7758. doi:10.1136/practneurol-2019-002192
Salama, H., Salama, A., Oscher, L., Jallo, G. I., and Shimony, N. (2024). The role of neuromodulation in the management of drug-resistant epilepsy. Neurol. Sci., 1–26. issn: 1590-3478 (Apr. 2024). doi:10.1007/s10072-024-07513-9
Scheid, B. H., Bernabei, J. M., Khambhati, A. N., Mouchtaris, S., Jeschke, J., Bassett, D. S., et al. (2022). Intracranial electroencephalographic biomarker predicts effective responsive neurostimulation for epilepsy prior to treatment. Epilepsia 63, 652–662. issn: 1528-1167. doi:10.1111/epi.17163
Shah, A. K., and Mittal, S. (2014). Invasive electroencephalography monitoring: indications and presurgical planning. Ann. Indian Acad. Neurology 17, S89–S94. issn: 19983549 (Suppl 1. doi:10.4103/0972-2327.128668
Shah, P., Ashourvan, A., Mikhail, F., Pines, A., Kini, L., Oechsel, K., et al. (2019). Characterizing the role of the structural connectome in seizure dynamics. Brain 142, 1955–1972. issn: 14602156. doi:10.1093/brain/awz125
Shamas, M., Yeh, H. J., Fried, I., Engel, J., and Staba, R. (2022). Interictal gamma event connectivity differentiates the seizure network and outcome in patients after temporal lobe epilepsy surgery. eNeuro 9, ENEURO.0141–22.2022. issn: 2373-2822. doi:10.1523/ENEURO.0141-22.2022
Sinha, N., Johnson, G. W., Davis, K. A., and Englot, D. J. (2022). Integrating network neuroscience into epilepsy care: progress, barriers, and next steps. Epilepsy Curr. 22, 272–278. issn: 15357511 (5 Sept. doi:10.1177/15357597221101271
Sinha, N., Wang, Y., Moreira da Silva, N., Miserocchi, A., McEvoy, A. W., de Tisi, J., et al. (2021). Structural brain network abnormalities and the probability of seizure recurrence after epilepsy surgery. Neurology 96, e758–e771. issn: 1526632X. doi:10.1212/WNL.0000000000011315
Spencer, S. S. (2002). Neural networks in human epilepsy: evidence of and implications for treatment. Epilepsia 43, 219–227. issn: 0013-9580 (3. doi:10.1046/j.1528-1157.2002.26901.x
Spencer, S. S. (1996). Long-term outcome after epilepsy surgery. Epilepsia 37, 807–813. issn: 0013-9580. doi:10.1111/j.1528-1157.1996.tb00032.x
Tan, G., Li, X., Niu, R., Wang, H., Chen, D., Gong, Q., et al. (2021). Functional connectivity of the thalamocortical circuit in patients with seizure relapse after antiseizure medication withdrawal. Epilepsia 62, 2463–2473. issn: 1528-1167. doi:10.1111/epi.17014
Tonini, C., Beghi, E., Berg, A. T., Bogliun, G., Giordano, L., Newton, R. W., et al. (2004). Predictors of epilepsy surgery outcome: a meta-analysis. Epilepsy Res. 62, 75–87. issn: 0920-1211. doi:10.1016/j.eplepsyres.2004.08.006
Tran, D. K., Mnatsakayan, L., Lin, J., Hsu, F., and Vadera, S. (2020). Treatment of multi-focal epilepsy with resective surgery plus responsive neurostimulation (RNS): one institution’s experience. Front. Neurology 11, 545074. issn: 16642295. doi:10.3389/fneur.2020.545074
Wiebe, S., Blume, W. T., Girvin, J. P., and Eliasziw, M.Effectiveness and Efficiency of Surgery for Temporal Lobe Epilepsy Study Group (2001). A randomized, controlled trial of surgery for temporal-lobe epilepsy. N. Engl. J. Med. 345, 311–318. issn: 0028-4793. doi:10.1056/NEJM200108023450501
Wilke, C., Worrell, G., and He, B. (2011). Graph analysis of epileptogenic networks in human partial epilepsy. Epilepsia 52, 84–93. issn: 1528-1167. doi:10.1111/j.1528-1167.2010.02785.x
Yang, J. C., Bullinger, K. L., Dickey, A. S., Karakis, I., Alwaki, A., Cabaniss, B. T., et al. (2022). Anterior nucleus of the thalamus deep brain stimulation vs temporal lobe responsive neurostimulation for temporal lobe epilepsy. Epilepsia 63, 2290–2300. issn: 15281167. doi:10.1111/epi.17331
Yoon, H. H., Kwon, H. L., Mattson, R. H., Spencer, D. D., and Spencer, S. S. (2003). Long-term seizure outcome in patients initially seizure-free after resective epilepsy surgery. Neurology 61, 445–450. issn: 1526-632X. doi:10.1212/01.wnl.0000081226.51886.5b
Keywords: epilepsy, epilepsy networks, epilepsy surgery, neuromodulation, seizures, seizure networks, resection, ablation
Citation: Langbein J, Boddeti U, Kreinbrink M, Khan Z, Rampalli I, Bachani M and Ksendzovsky A (2024) Therapeutic approaches targeting seizure networks. Front. Netw. Physiol. 4:1441983. doi: 10.3389/fnetp.2024.1441983
Received: 31 May 2024; Accepted: 16 July 2024;
Published: 07 August 2024.
Edited by:
Klaus Lehnertz, University of Bonn, GermanyReviewed by:
Vikram Rao, University of California, San Francisco, United StatesRandi Von Wrede, University Hospital Bonn, Germany
Copyright © 2024 Langbein, Boddeti, Kreinbrink, Khan, Rampalli, Bachani and Ksendzovsky. This is an open-access article distributed under the terms of the Creative Commons Attribution License (CC BY). The use, distribution or reproduction in other forums is permitted, provided the original author(s) and the copyright owner(s) are credited and that the original publication in this journal is cited, in accordance with accepted academic practice. No use, distribution or reproduction is permitted which does not comply with these terms.
*Correspondence: Alexander Ksendzovsky, YWtzZW5kem92c2t5QHNvbS51bWFyeWxhbmQuZWR1
†These authors have contributed equally to this work and share first authorship
‡These authors contributed equally and share second authorship.