- 1Department of Control Theory, Lobachevsky State University of Nizhny Novgorod, Nizhny Novgorod, Russia
- 2Department of Mathematics and Statistics and Neuroscience Institute, Georgia State University, Atlanta, GA, United States
The dynamics of synaptic interactions within spiking neuron networks play a fundamental role in shaping emergent collective behavior. This paper studies a finite-size network of quadratic integrate-and-fire neurons interconnected via a general synaptic function that accounts for synaptic dynamics and time delays. Through asymptotic analysis, we transform this integrate-and-fire network into the Kuramoto-Sakaguchi model, whose parameters are explicitly expressed via synaptic function characteristics. This reduction yields analytical conditions on synaptic activation rates and time delays determining whether the synaptic coupling is attractive or repulsive. Our analysis reveals alternating stability regions for synchronous and partially synchronous firing, dependent on slow synaptic activation and time delay. We also demonstrate that the reduced microscopic model predicts the emergence of synchronization, weakly stable cyclops states, and non-stationary regimes remarkably well in the original integrate-and-fire network and its theta neuron counterpart. Our reduction approach promises to open the door to rigorous analysis of rhythmogenesis in networks with synaptic adaptation and plasticity.
1 Introduction
Cooperative rhythms play a pivotal role in brain functioning. Fully or partially synchronized oscillations, observed across various frequency bands, underlie fundamental processes such as perception, cognition, and motor control Churchland and Sejnowski, 1992; Mizuseki and Buzsaki, 2014; Kopell et al., 2000. Extensive research has focused on the emergence of cooperative rhythms in networks of spiking and bursting neurons, encompassing synchronization Kopell et al., 2000; Brunel, 2000; Börgers and Kopell, 2003; Somers and Kopell, 1993; Izhikevich, 2007; Belykh et al., 2005; Ermentrout and Terman, 2010, partial and cluster synchronization Achuthan and Canavier 2009; Shilnikov et al., 2008; Belykh and Hasler, 2011; Schöll, 2016; Berner et al., 2021a, neural bumps Laing and Chow, 2001; Gutkin et al., 2001, and chimera states Olmi et al., 2011; Omelchenko et al., 2013.
Networks of spiking neurons with fast synaptic connections are often modeled via pulsatile on-off coupling, which sharply activates upon the arrival of a spike from a pre-synaptic cell. Such interactions are conveniently represented by networks of quadratic integrate-and-fire (QIF) models particularly suitable for large-scale simulations and analysis of cooperative dynamics Gerstner and Kistler (2002). The macroscopic dynamics of QIF networks have received extensive attention through the reduction to low-dimensional model descriptions, especially in the thermodynamic limit of infinite-dimensional networks Montbrió et al., 2015; Pazó and Montbrió, 2016; Devalle et al., 2017; Esnaola-Acebes et al., 2017; Devalle et al., 2018; Schmidt et al., 2018; Pietras et al., 2019; Montbrió and Pazó 2020; Lin et al., 2020; Gast et al., 2020; Taher et al., 2020; Byrne et al., 2022; Clusella et al., 2022; Clusella and Montbrió 2024; Ratas and Pyragas, 2018; Pyragas and Pyragas 2022, 2023; Coombes 2023; Ferrara et al., 2023. Notably, Montbrió et al. (2015) derived exact macroscopic equations for QIF networks, uncovering an effective coupling between firing rate and mean membrane potential governing network dynamics. Pietras et al. (2023) offered an analytical description of QIF network macroscopic dynamics, extending beyond the Ott-Antosen ansatz Ott and Antonsen (2008) and exploring various fast synaptic pulse profile choices. The impact of synaptic time delay on the collective dynamics of integrate-and-fire networks with sharply activated synaptic coupling, modeled by the Dirac delta function, was also extensively explored (Ernst et al., 1995; Devalle et al., 2018; Ratas and Pyragas, 2018; Pyragas and Pyragas 2022, 2023). In particular, Devalle et al. (2018) reduced a QIF model with synaptic delay to a set of firing rate equations to analyze the existence and stability of partially synchronous states. Ratas and Pyragas (2018) employed a Lorenzian ansatz to characterize macroscopic oscillations of a QIF network with heterogeneous time-delayed delta function synapses. However, there is a lack of analytical studies on the role of slower synaptic activation, potentially in the presence of time delays, in controlling critical phase transitions in QIF networks. Nevertheless, since the seminal paper by Van Vreeswijk et al. (1994), it has been recognized that slow inhibitory and excitatory synapses can reverse their roles, with slow inhibition favoring synchronization Golomb and Rinzel 1993; Terman et al., 1998; Elson et al., 2002. While predicting the exact rates of synaptic activation inducing such critical transitions in conductance-based spiking models may be challenging, analytically tractable QIF networks offer promising avenues for such exploration.
Toward this goal, this paper investigates a finite-size network of QIF neurons globally connected via a general kernel function that governs both synaptic activation and synaptic time delay. We analytically illustrate how the shape of the kernel function impacts neuron interaction, significantly altering the microscopic and macroscopic behavior of QIF networks representing Type I neuron populations. This is achieved by reducing QIF networks and their phase analog, theta neuron networks, to the Kuramoto-Sakaguchi (KS) model. Here, oscillator frequencies, coupling strength, and the Sakaguchi phase lag parameter are determined by the pulse profile’s first and second terms in the Fourier expansion. We conduct this reduction under the weak coupling assumption, utilizing the intermediate step of representing the QIF network as a generalized Winfree model, subsequently reduced to the KS model.
In our recent study Munyayev et al. (2023), we elucidated the qualitative connection between the dynamics of QIF networks incorporating synaptic dynamics and neuronal refractoriness, and the second-order Kuramoto model with high-order mode coupling. Here, we use multi-scale analysis to derive exact relationships between the QIF network with an arbitrary synaptic activation function and the KS model. Specifically, we establish explicit conditions on the parameters of the general kernel function that lead to critical transitions, determining whether the coupling is attractive or repulsive. Consequently, these conditions dictate the emergence of stable synchronization or nonstationary generalized splay states Berner et al. (2021b) and cyclops states Munyayev et al. (2023). Our analysis reveals alternating stability regions for network synchronization, dependent on both the (slow) synaptic activation and time delay. With some important caveats, this finding can be interpreted as an analogous stability criterion for synchronization in time-delayed phase oscillator networks Earl and Strogatz (2003).
Our approach serves as a connecting link between two alternative methodologies for describing macroscopic dynamics: QIF networks and theta neurons, and Winfree-type models Pazó and Montbrió, 2014; Gallego et al., 2017; Montbrió and Pazó, 2018; Pazó et al., 2019; Pazó and Gallego 2020; Manoranjani et al., 2021; Bick et al., 2020. Our KS model reduction of the generalized Winfree model with a general synaptic activation function can be seen as an extension of the work Montbrió and Pazó (2018), where a two-population Kuramoto model was derived from a network of Winfree oscillators featuring a feedback loop between fast excitation and slow inhibition.
The structure of this paper is outlined as follows. Section 2 presents the QIF network model, its theta neuron equivalent, and the general synaptic activation function. Section 3 details transforming the theta neuron model into the generalized Winfree model. We expand the pulse profile as a Fourier series and further simplify the model to the KS model using weak coupling-enabled averaging techniques. Section 4 focuses on a specific example of synaptic activation, presenting a class of kernel functions. We establish exact conditions determining whether the synaptic coupling is attractive, promoting synchronization, or repulsive, favoring splay and cyclops states. Section 5 offers numerical validation of the derived conditions and presents a comparison between the dynamics of the QIF network, the theta neuron model, and the reduced KS model. We demonstrate that the KS model accurately predicts firing rates and times, capturing the emergence of synchronization, weakly stable cyclops states, and non-stationary regimes. Section 6 contains concluding remarks and discussions.
2 The general QIF network and its theta neuron representation
Physiologically, excitable neurons are commonly categorized into two types. We focus here on Type I neurons, a group encompassing cortical excitatory pyramidal neurons. When subjected to a sufficiently large input stimulus, these neurons exhibit action potentials at an arbitrarily low rate, signaling the disappearance of a resting state through a saddle-node bifurcation. The canonical model used to describe Type I neurons is the QIF neuron model, which characterizes neurons’ dynamics near the spiking threshold Izhikevich (2007).
This study investigates a globally coupled network of
Here,
The last term on the right-hand side of (Eq. 1) represents synaptic interactions characterized by the coupling strength
This equation accounts for relaxation processes and describes a specific type of neuron activation and its sensitivity to stimuli from other cells, including signal duration and post-spike latency. Here,
The QIF-neuron model (Eq. 1) describes the membrane potential
where
The last term on the right-hand side of (Eq. 3) accounts for chemical interactions among neurons. The coupling strength
where the function
determines the shape of the pulsatile chemical synapse. The positive integer parameter
where
Note that the limiting
In this work, we primarily focus on the pulse shape defined by (Eq. 5), originally proposed in Ariaratnam and Strogatz (2001) and widely adopted in recent studies of pulse-coupled phase oscillators O’Keeffe and Strogatz 2016; Pazó and Montbrió, 2014; Gallego et al., 2017; Pazó et al., 2019; Bick et al., 2020 and populations of theta neurons Luke et al., 2013; So et al., 2014; Laing 2014, Laing 2015; Montbrió et al., 2015; Pazó and Montbrió, 2016; Chandra et al., 2017; Goel and Ermentrout 2002; Bick et al., 2020; Pietras et al., 2023. However, our approach is directly applicable to alternative pulse shapes satisfying common properties such as unimodality, normalization, symmetry, and localization around
In the following, we explore how the shape of the kernel function
3 Deriving the KS model from the theta-neuron model: an asymptotic analysis
We begin by assuming that each neuron operates within an oscillatory regime in the absence of interaction, i.e.,
where
The transformation (Eq. 7) transitions the model (Eqs 3, 4) to an alternative phase representation:
where
This representation remains consistent with the original description; notably,
For further analysis, it is convenient to expand the symmetric pulse
The coefficients
where
Noteworthy, in the limit
We proceed by assuming that the synaptic coupling is weak, allowing us to express it as
These assumptions enable a multiple-time scale analysis. To facilitate this analysis, we introduce a separation of time scales:
and represent each phase variable,
Substituting the series (Eq. 15) with times (Eq. 14) and (Eq. 11) into (Eqs 8, 9), and considering the zeroth order in
and, taking into account (Eq. 16), for
where each corresponding complex coefficient
In (Eq. 16), the first term
In accordance with the averaging procedure after substituting expression (Eq. 17) into (Eq. 8), the next step of our asymptotic approach involves considering all terms that are
To eliminate the secular terms that grow without bounds as
This yields a solution for
where
To determine the unknown parameter
This choice of the optimal value of parameter
where the complex coefficient
Note that oscillator frequencies
4 The role of synaptic profile: a combined effect of activation, deactivation, and time delays
To demonstrate the important effects arising from the specific selection of the shape of a “low-pass filter” in synaptic activation, we examine the following class of kernel functions:
where
The source term on the right-hand side of (Eq. 26) is presented in three interchangeable forms corresponding to the QIF model (Eqs 1, 2), theta neuron (Eqs 3, 4), and their averaged representation through the KS model (Eq. 20). This term can be interpreted as the population firing rate, which induces a post-synaptic current in response to the arrival of spikes. In the limit
To describe the adaption dynamics of
In the case
For
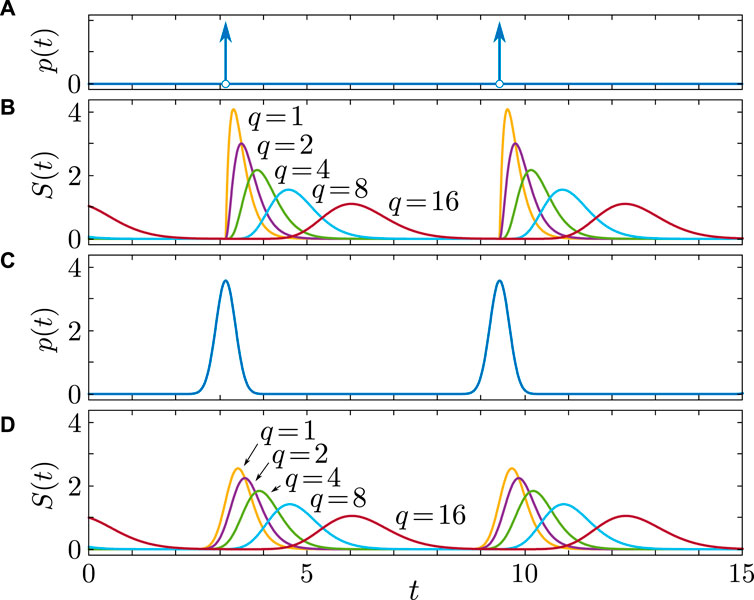
Figure 1. Synaptic dynamics
We extend the argument to arbitrary
Our choice of the kernel function
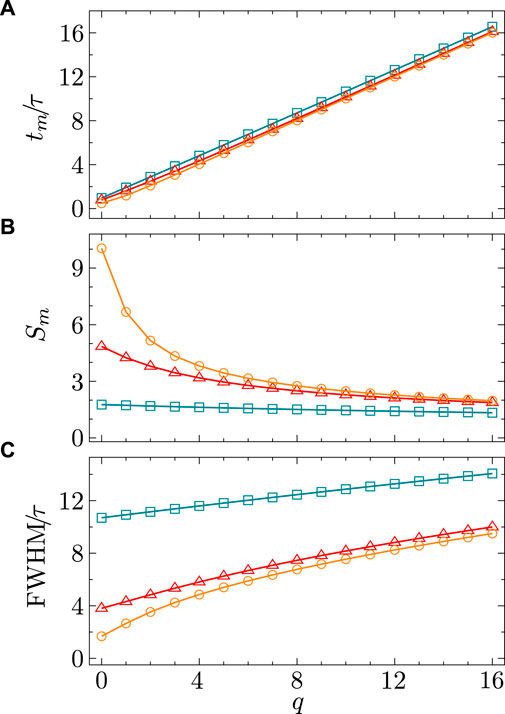
Figure 2. Characteristics of the synaptic dynamic profile for
Towards our objective of deriving explicit conditions for the attractiveness or repulsiveness of synaptic coupling governed by (Eq. 24) with the kernel function (Eq. 25), we calculate the complex coefficient
where
Solving the inequality (Eq. 28), we obtain the following
where
Figure 3 displays the regions, as defined by (Eq. 29), where the coupling in the KS model is attractive (blue) or repulsive (red). These regions exhibit an alternating pattern as functions of the synaptic time constant
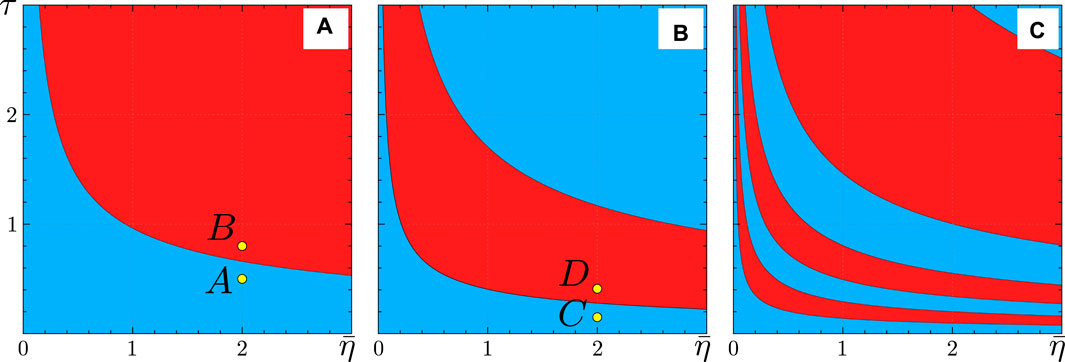
Figure 3. Regions of attractive (blue) and repulsive (red) coupling in the theta neuron model (Eqs 3, 4), corresponding to the KS model regions defined by (Eq. 29). The colors represent the coupling strength
Figure 4 provides further insight into how the synchronization properties of the KS model, critically controlled by the coupling strength
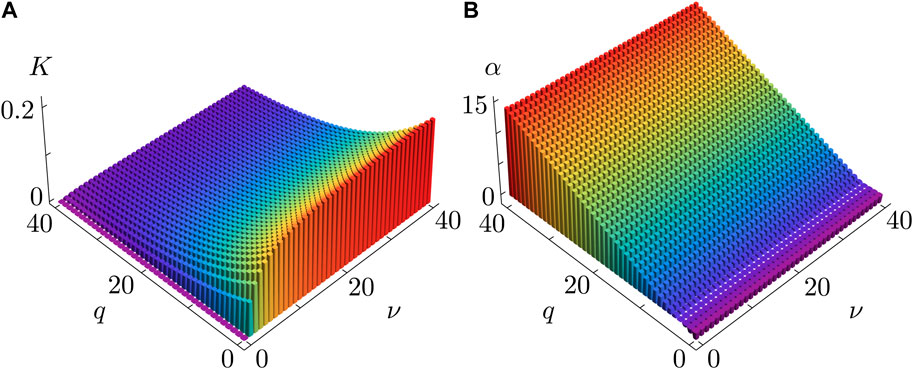
Figure 4. Coupling strength
In the following, we offer additional evidence supporting the predictive power of the derived KS model. We show numerically that it effectively captures the emergence of robust dynamical regimes like synchronization and more intricate partially synchronized dynamics such as weakly stable cyclops states and non-stationary generalized splay states in both the QIF and theta neuron models.
5 Dynamical equivalence of the models: numerical validation
We conduct numerical computations using a widely accepted fifth-order Runge–Kutta method with a fixed time step of 0.01, providing additional validation for our analytical findings and predictions.
To characterize the dynamical regimes, we utilize both microscopic measures (pairwise phase differences and firing times) and macroscopic indicators such as the first- and second-order complex Kuramoto parameters Daido 1992; Skardal et al., 2011:
where
To identify the time steps corresponding to neuron spike events in both the QIF-neuron model and the theta neuron model, we monitored the sign changes of
Figures 5, 6 illustrate the perfect correspondence between the emergence of full synchronization and non-stationary generalized splay states in the theta neuron model (Eqs 3, 4) and the KS model (Eq. 20) within the range of attractive coupling (point A in Figure 3) and repulsive coupling (point B in Figure 3), respectively. In the case of full synchronization (Figure 5), the first-order and second-order scalar parameters (Eq. 30),
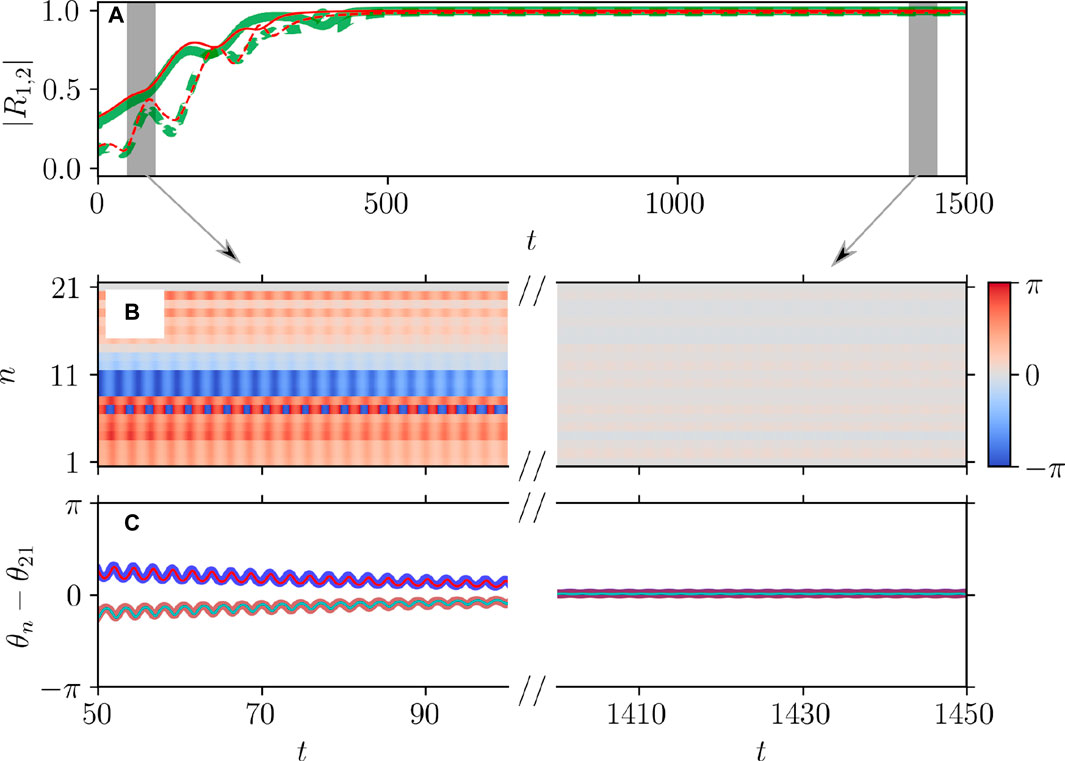
Figure 5. Dynamical equivalence between the theta neuron (Eqs 3, 4) and KS models (Eq. 20), demonstrated via the onset of full synchronization. (A) The evolution of the first
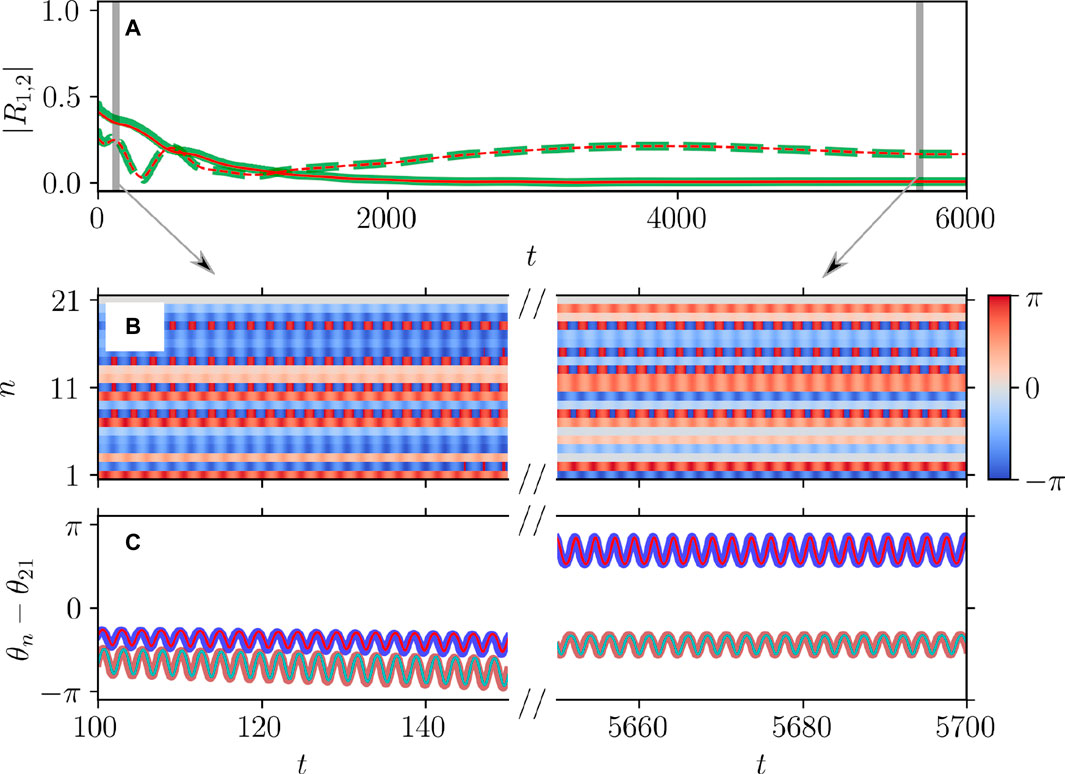
Figure 6. Dynamical equivalence between the theta neuron (Eqs 3, 4) and KS models (Eq. 20), demonstrated via the onset of non-stationary generalized splay state with an oscillating
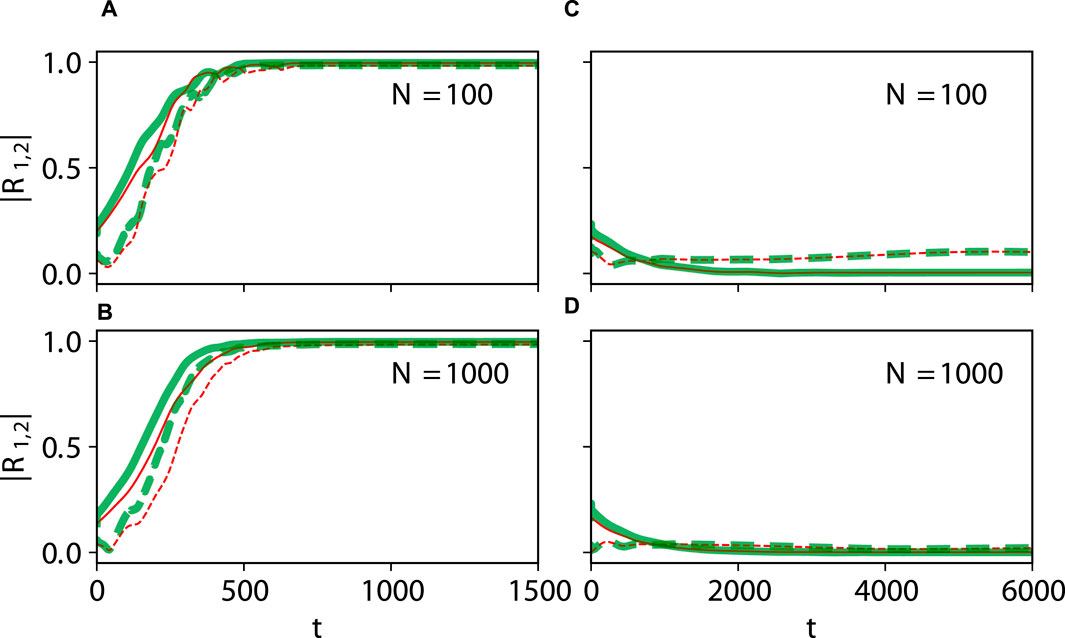
Figure 7. Large-size networks. Dynamical equivalence between the theta neuron (Eqs 3, 4) and KS models (Eq. 20), demonstrated via the onset of full synchronization (A,B) and non-stationary generalized splay state with an oscillating
Figures 8–10 illustrate the remarkable agreement between cooperative dynamics in the QIF and KS models. Specifically, Figure 8 depicts the onset of full synchronization, as evidenced by synchronized firing rates and times determined via (Eq. 31). The slight discrepancy in the firing times between the QIF and KS models may stem from various sources, such as accumulated numerical errors and the approximate calculation of the frequency parameter
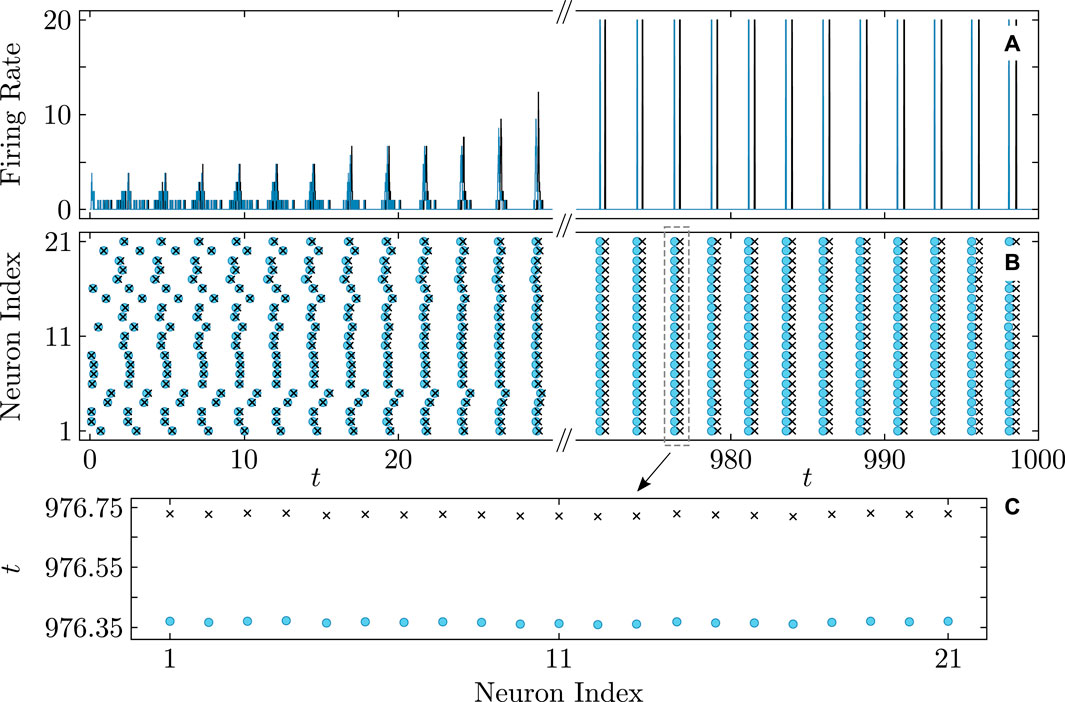
Figure 8. Onset of full synchronization in the QIF (Eq. 1) and KS models (Eq. 20). (A) Firing rate and (B) firing times of QIF neurons (cyan curves and round markers) and oscillators of the KS model (black curves and cross markers) with the firing times recorded at
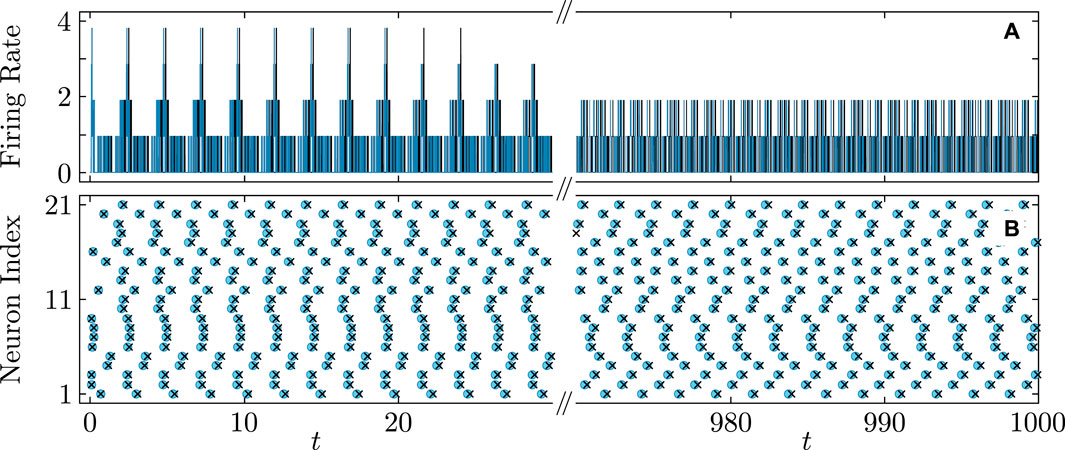
Figure 9. Diagram similar to Figure 8 showing a nearly perfect match for asynchronous firing rate (A) and firing times (B) in the QIF network (cyan curves and round markers) and the KS model (black curves and cross markers). Parameters:
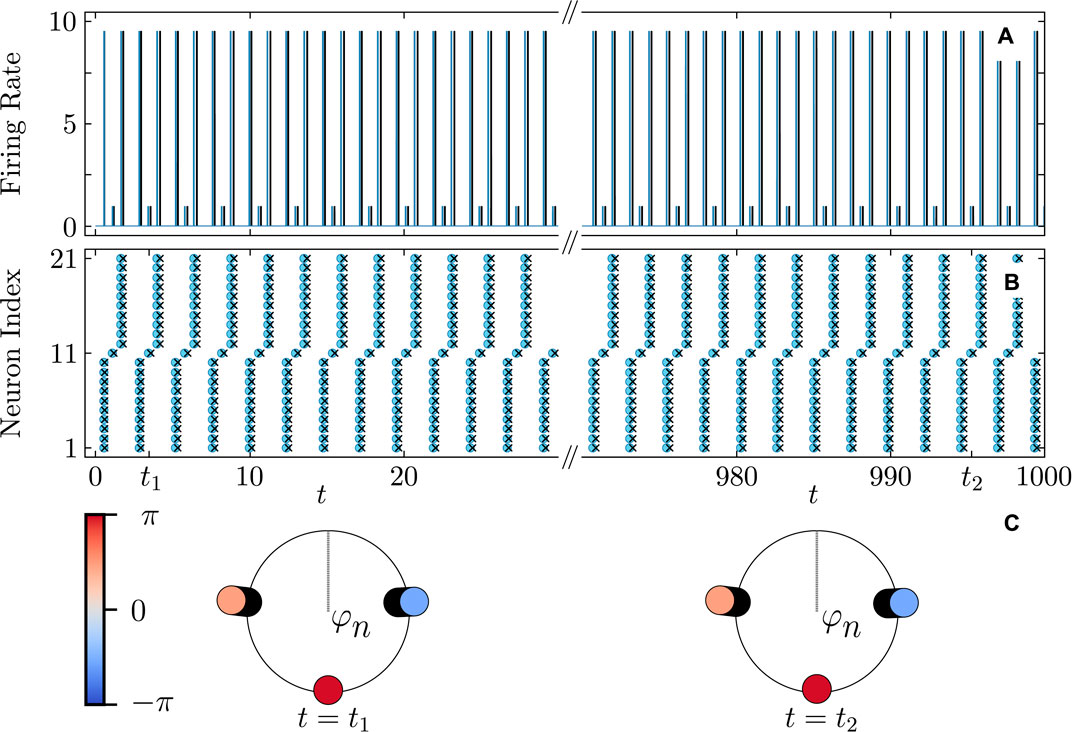
Figure 10. Firing rate (A) and firing times (B) of a three-cluster cyclops state in the QIF network (cyan curves and round markers) and the KS model (black curves and cross markers). (C) Snapshots of the cyclops state phase distributions
In the numerical calculations of relatively small-size networks of QIF neurons presented in Figures 8–10, we employed the fourth–order Runge–Kutta method using the procedure for identifying the spike events described above. To ensure better consistency between the QIF-neuron model and the theta neuron model, we used sufficiently large values of
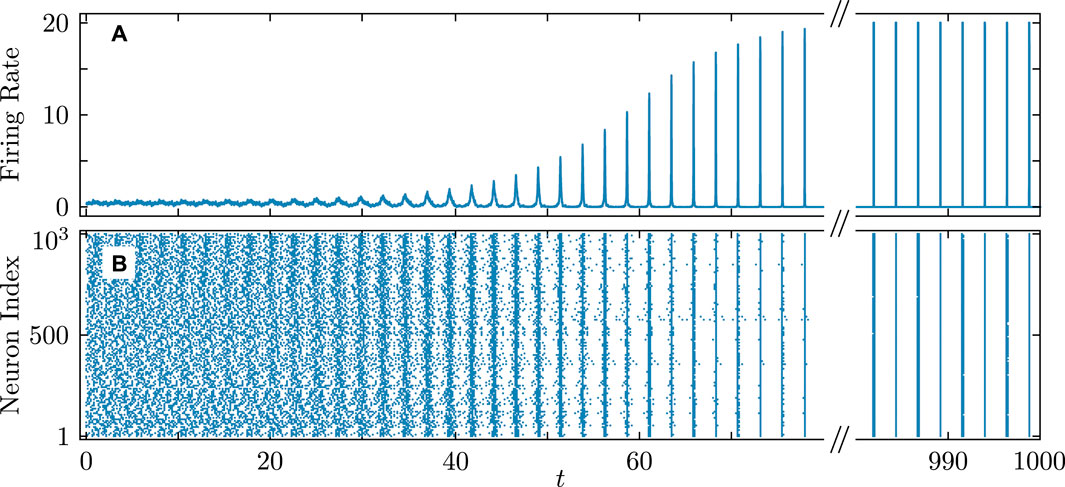
Figure 11. Firing rate (A) and firing times (B) in the QIF network demonstrating the transition to full synchronization starting from random initial conditions. Parameters
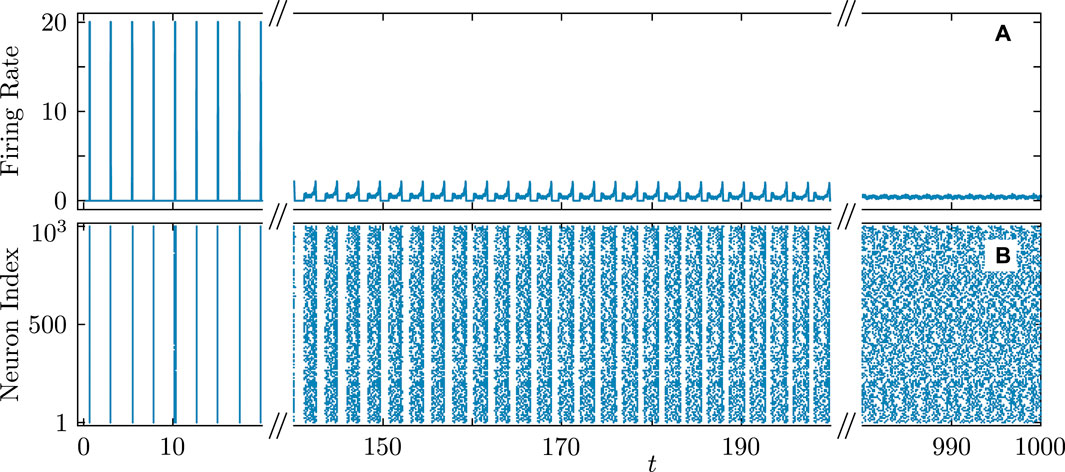
Figure 12. Firing rate (A) and firing times (B) in the QIF network accompanying the transition from synchronous to asynchronous dynamics. Parameters
6 Conclusions
Understanding the influence of synaptic dynamics, including activation rates, deactivation processes, and latency, on collective dynamics in neuronal networks is of significant importance. Considerable advancements have been made in analyzing the role of fast or time-delayed synapses in integrate-and-fire neuron networks. However, there remains a scarcity of analytical studies exploring the influence of slower synaptic dynamics, potentially in the presence of time delays, on controlling critical phase transitions in neuronal networks.
In this paper, we have made substantial contributions to advancing analytical methods in this field. We studied a finite-size network of QIF neurons globally interconnected via a generalized kernel function governing both synaptic activation and time delay. Our analytical exploration demonstrated how the shape of the kernel function profoundly affects neuron interaction, thereby significantly modifying the microscopic and macroscopic behavior of QIF networks. To achieve this, we reduced the QIF and theta neuron network models to the Kuramoto–Sakaguchi model. In this model, oscillator frequencies, coupling strength, and the Sakaguchi phase lag parameter are determined by the Fourier terms of the pulse profile series expansion.
We established exact conditions determining whether synaptic coupling is attractive, fostering synchronization, or repulsive, promoting splay and cyclops states. Furthermore, we demonstrated a remarkable correspondence between the dynamics of the derived KS model and the original QIF and theta neuron models. Specifically, the KS model accurately predicted firing rates and times, capturing the emergence of synchronization, weakly stable cyclops states, and non-stationary regimes in the QIF model. Our reduction approach complements the work by Ratas and Pyragas (2018), which assumed a Lorentzian distribution of the input currents and employed the thermodynamic limit to reduce the QIF model with time-delayed coupling to macroscopic equations that characterize the mean membrane potential, the spiking rate, and the mean synaptic current. The bifurcation analysis of these macroscopic equations, performed in Ratas and Pyragas (2018), revealed alternating parameter regions where the QIF network exhibits macroscopic self-oscillations as a function of input current heterogeneity and time-delayed coupling. While sharing some similarities and goals, our approach is fundamentally different. It reduces finite-size QIF networks with an arbitrary current distribution and arbitrary synaptic activation function
Data availability statement
The original contributions presented in the study are included in the article/supplementary material, further inquiries can be directed to the corresponding author.
Author contributions
LAS: Conceptualization, Formal Analysis, Investigation, Methodology, Visualization, Writing–review and editing. VOM: Formal Analysis, Investigation, Methodology, Visualization, Writing–review and editing. MIB: Formal Analysis, Investigation, Methodology, Visualization, Writing–review and editing. GVO: Conceptualization, Investigation, Methodology, Supervision, Writing–review and editing. IB: Conceptualization, Investigation, Methodology, Supervision, Writing–original draft.
Funding
The author(s) declare that financial support was received for the research, authorship, and/or publication of this article. This work was supported by the Ministry of Science and Higher Education of the Russian Federation under project No. 0729-2020-0036 (to GO and MB.), the Russian Science Foundation under project No. 22-12-00348 (to VM and LS), the Georgia State University Brains and Behavior Program, the National Science Foundation (United States) under Grants No. CMMI-2009329 and CMMI-1953135 (to IB).
Conflict of interest
The authors declare that the research was conducted in the absence of any commercial or financial relationships that could be construed as a potential conflict of interest.
Publisher’s note
All claims expressed in this article are solely those of the authors and do not necessarily represent those of their affiliated organizations, or those of the publisher, the editors and the reviewers. Any product that may be evaluated in this article, or claim that may be made by its manufacturer, is not guaranteed or endorsed by the publisher.
References
Achuthan, S., and Canavier, C. C. (2009). Phase-resetting curves determine synchronization, phase locking, and clustering in networks of neural oscillators. J. Neurosci. 29, 5218–5233. doi:10.1523/JNEUROSCI.0426-09.2009
Afifurrahman, A., Ullner, E., and Politi, A. (2021). Collective dynamics in the presence of finite-width pulses. Chaos An Interdiscip. J. Nonlinear Sci. 31, 043135. doi:10.1063/5.0046691
Afifurrahman, , Ullner, E., and Politi, A. (2020). Stability of synchronous states in sparse neuronal networks. Nonlinear Dyn. 102, 733–743. doi:10.1007/s11071-020-05880-4
Ariaratnam, J. T., and Strogatz, S. H. (2001). Phase diagram for the Winfree model of coupled nonlinear oscillators. Phys. Rev. Lett. 86, 4278–4281. doi:10.1103/PhysRevLett.86.4278
Belykh, I., De Lange, E., and Hasler, M. (2005). Synchronization of bursting neurons: what matters in the network topology. Phys. Rev. Lett. 94, 188101. doi:10.1103/PhysRevLett.94.188101
Belykh, I., and Hasler, M. (2011). Mesoscale and clusters of synchrony in networks of bursting neurons. Chaos An Interdiscip. J. Nonlinear Sci. 21, 016106. doi:10.1063/1.3563581
Berner, R., Vock, S., Schöll, E., and Yanchuk, S. (2021a). Desynchronization transitions in adaptive networks. Phys. Rev. Lett. 126, 028301. doi:10.1103/PhysRevLett.126.028301
Berner, R., Yanchuk, S., Maistrenko, Y., and Scholl, E. (2021b). Generalized splay states in phase oscillator networks. Chaos An Interdiscip. J. Nonlinear Sci. 31, 073128. doi:10.1063/5.0056664
Bick, C., Goodfellow, M., Laing, C. R., and Martens, E. A. (2020). Understanding the dynamics of biological and neural oscillator networks through exact mean-field reductions: a review. J. Math. Neurosci. 10 (9), 9. doi:10.1186/s13408-020-00086-9
Bolotov, M., Osipov, G., and Pikovsky, A. (2016). Marginal chimera state at cross-frequency locking of pulse-coupled neural networks. Phys. Rev. E 93, 032202. doi:10.1103/PhysRevE.93.032202
Börgers, C., and Kopell, N. (2003). Synchronization in networks of excitatory and inhibitory neurons with sparse, random connectivity. Neural comput. 15, 509–538. doi:10.1162/089976603321192059
Brunel, N. (2000). Dynamics of sparsely connected networks of excitatory and inhibitory spiking neurons. J. Comput. Neurosci. 8, 183–208. doi:10.1023/a:1008925309027
Byrne, Á., Ross, J., Nicks, R., and Coombes, S. (2022). Mean-field models for EEG/MEG: from oscillations to waves. Brain Topogr. 35, 36–53. doi:10.1007/s10548-021-00842-4
Chandra, S., Hathcock, D., Crain, K., Antonsen, T. M., Girvan, M., and Ott, E. (2017). Modeling the network dynamics of pulse-coupled neurons. Chaos An Interdiscip. J. Nonlinear Sci. 27, 033102. doi:10.1063/1.4977514
Chen, B., Engelbrecht, J. R., and Mirollo, R. (2017). Cluster synchronization in networks of identical oscillators with α-function pulse coupling. Phys. Rev. E 95, 022207. doi:10.1103/PhysRevE.95.022207
Clusella, P., and Montbrió, E. (2024). Exact low-dimensional description for fast neural oscillations with low firing rates. Phys. Rev. E 109, 014229. doi:10.1103/PhysRevE.109.014229
Clusella, P., Pietras, B., and Montbrió, E. (2022). Kuramoto model for populations of quadratic integrate-and-fire neurons with chemical and electrical coupling. Chaos An Interdiscip. J. Nonlinear Sci. 32, 013105. doi:10.1063/5.0075285
Coombes, S. (2023). Next generation neural population models. Front. Appl. Math. Statistics 9, 1128224. doi:10.3389/fams.2023.1128224
Daido, H. (1992). Order function and macroscopic mutual entrainment in uniformly coupled limit-cycle oscillators. Prog. Theor. Phys. 88, 1213–1218. doi:10.1143/ptp.88.1213
Devalle, F., Montbrió, E., and Pazó, D. (2018). Dynamics of a large system of spiking neurons with synaptic delay. Phys. Rev. E 98, 042214. doi:10.1103/physreve.98.042214
Devalle, F., Roxin, A., and Montbrió, E. (2017). Firing rate equations require a spike synchrony mechanism to correctly describe fast oscillations in inhibitory networks. PLoS Comput. Biol. 13, e1005881. doi:10.1371/journal.pcbi.1005881
Earl, M. G., and Strogatz, S. H. (2003). Synchronization in oscillator networks with delayed coupling: a stability criterion. Phys. Rev. E 67, 036204. doi:10.1103/PhysRevE.67.036204
Elson, R. C., Selverston, A. I., Abarbanel, H. D., and Rabinovich, M. I. (2002). Inhibitory synchronization of bursting in biological neurons: dependence on synaptic time constant. J. Neurophysiology 88, 1166–1176. doi:10.1152/jn.2002.88.3.1166
Ermentrout, B., and Kopell, N. (1986). Parabolic bursting in an excitable system coupled with a slow oscillation. SIAM J. Appl. Math. 46, 233–253. doi:10.1137/0146017
Ermentrout, G. B., and Terman, D. H. (2010). Mathematical foundations of neuroscience, 35. Springer Science and Business Media.
Ernst, U., Pawelzik, K., and Geisel, T. (1995). Synchronization induced by temporal delays in pulse-coupled oscillators. Phys. Rev. Lett. 74, 1570–1573. doi:10.1103/PhysRevLett.74.1570
Esnaola-Acebes, J. M., Roxin, A., Avitabile, D., and Montbrió, E. (2017). Synchrony-induced modes of oscillation of a neural field model. Phys. Rev. E 96, 052407. doi:10.1103/PhysRevE.96.052407
Ferrara, A., Angulo-Garcia, D., Torcini, A., and Olmi, S. (2023). Population spiking and bursting in next-generation neural masses with spike-frequency adaptation. Phys. Rev. E 107, 024311. doi:10.1103/PhysRevE.107.024311
Gallego, R., Montbrió, E., and Pazó, D. (2017). Synchronization scenarios in the Winfree model of coupled oscillators. Phys. Rev. E 96, 042208. doi:10.1103/PhysRevE.96.042208
Gast, R., Schmidt, H., and Knösche, T. R. (2020). A mean-field description of bursting dynamics in spiking neural networks with short-term adaptation. Neural Comput. 32, 1615–1634. doi:10.1162/neco_a_01300
Gerstner, W., and Kistler, W. M. (2002). Spiking neuron models: single neurons, populations, plasticity. Cambridge University Press.
Goel, P., and Ermentrout, B. (2002). Synchrony, stability, and firing patterns in pulse-coupled oscillators. Phys. D. Nonlinear Phenom. 163, 191–216. doi:10.1016/s0167-2789(01)00374-8
Golomb, D., and Rinzel, J. (1993). Dynamics of globally coupled inhibitory neurons with heterogeneity. Phys. Rev. E 48, 4810–4814. doi:10.1103/physreve.48.4810
Gutkin, B. S., Laing, C. R., Colby, C. L., Chow, C. C., and Ermentrout, G. B. (2001). Turning on and off with excitation: the role of spike-timing asynchrony and synchrony in sustained neural activity. J. Comput. Neurosci. 11, 121–134. doi:10.1023/a:1012837415096
Kopell, N., Ermentrout, G., Whittington, M. A., and Traub, R. D. (2000). Gamma rhythms and beta rhythms have different synchronization properties. Proc. Natl. Acad. Sci. 97, 1867–1872. doi:10.1073/pnas.97.4.1867
Laing, C. R. (2014). Derivation of a neural field model from a network of theta neurons. Phys. Rev. E 90, 010901. doi:10.1103/PhysRevE.90.010901
Laing, C. R. (2015). Exact neural fields incorporating gap junctions. SIAM J. Appl. Dyn. Syst. 14, 1899–1929. doi:10.1137/15m1011287
Laing, C. R., and Chow, C. C. (2001). Stationary bumps in networks of spiking neurons. Neural Comput. 13, 1473–1494. doi:10.1162/089976601750264974
Lin, L., Barreto, E., and So, P. (2020). Synaptic diversity suppresses complex collective behavior in networks of theta neurons. Front. Comput. Neurosci. 14, 44. doi:10.3389/fncom.2020.00044
Luke, T. B., Barreto, E., and So, P. (2013). Complete classification of the macroscopic behavior of a heterogeneous network of theta neurons. Neural Comput. 25, 3207–3234. doi:10.1162/NECO_a_00525
Manoranjani, M., Gopal, R., Senthilkumar, D., and Chandrasekar, V. (2021). Role of phase-dependent influence function in the Winfree model of coupled oscillators. Phys. Rev. E 104, 064206. doi:10.1103/PhysRevE.104.064206
Mizuseki, K., and Buzsaki, G. (2014). Theta oscillations decrease spike synchrony in the hippocampus and entorhinal cortex. Philosophical Trans. R. Soc. B Biol. Sci. 369, 20120530. doi:10.1098/rstb.2012.0530
Mohanty, P., and Politi, A. (2006). A new approach to partial synchronization in globally coupled rotators. J. Phys. A Math. General 39, L415–L421. doi:10.1088/0305-4470/39/26/l01
Montbrió, E., and Pazó, D. (2018). Kuramoto model for excitation-inhibition-based oscillations. Phys. Rev. Lett. 120, 244101. doi:10.1103/PhysRevLett.120.244101
Montbrió, E., and Pazó, D. (2020). Exact mean-field theory explains the dual role of electrical synapses in collective synchronization. Phys. Rev. Lett. 125, 248101. doi:10.1103/PhysRevLett.125.248101
Montbrió, E., Pazó, D., and Roxin, A. (2015). Macroscopic description for networks of spiking neurons. Phys. Rev. X 5, 021028. doi:10.1103/physrevx.5.021028
Munyayev, V. O., Bolotov, M. I., Smirnov, L. A., Osipov, G. V., and Belykh, I. (2023). Cyclops states in repulsive Kuramoto networks: the role of higher-order coupling. Phys. Rev. Lett. 130, 107201. doi:10.1103/PhysRevLett.130.107201
O’Keeffe, K. P., and Strogatz, S. H. (2016). Dynamics of a population of oscillatory and excitable elements. Phys. Rev. E 93, 062203. doi:10.1103/PhysRevE.93.062203
Olmi, S., Politi, A., and Torcini, A. (2011). Collective chaos in pulse-coupled neural networks. Europhys. Lett. 92, 60007. doi:10.1209/0295-5075/92/60007
Omelchenko, I., Omel’chenko, E., Hövel, P., and Schöll, E. (2013). When nonlocal coupling between oscillators becomes stronger: patched synchrony or multichimera states. Phys. Rev. Lett. 110, 224101. doi:10.1103/PhysRevLett.110.224101
Ott, E., and Antonsen, T. M. (2008). Low dimensional behavior of large systems of globally coupled oscillators. Chaos An Interdiscip. J. Nonlinear Sci. 18, 037113. doi:10.1063/1.2930766
Pazó, D., and Gallego, R. (2020). The Winfree model with non-infinitesimal phase-response curve: Ott–Antonsen theory. Chaos An Interdiscip. J. Nonlinear Sci. 30, 073139. doi:10.1063/5.0015131
Pazó, D., and Montbrió, E. (2014). Low-dimensional dynamics of populations of pulse-coupled oscillators. Phys. Rev. X 4, 011009. doi:10.1103/physrevx.4.011009
Pazó, D., and Montbrió, E. (2016). From quasiperiodic partial synchronization to collective chaos in populations of inhibitory neurons with delay. Phys. Rev. Lett. 116, 238101. doi:10.1103/PhysRevLett.116.238101
Pazó, D., Montbrió, E., and Gallego, R. (2019). The Winfree model with heterogeneous phase-response curves: analytical results. J. Phys. A Math. Theor. 52, 154001. doi:10.1088/1751-8121/ab0b4c
Pietras, B., Cestnik, R., and Pikovsky, A. (2023). Exact finite-dimensional description for networks of globally coupled spiking neurons. Phys. Rev. E 107, 024315. doi:10.1103/PhysRevE.107.024315
Pietras, B., Devalle, F., Roxin, A., Daffertshofer, A., and Montbrió, E. (2019). Exact firing rate model reveals the differential effects of chemical versus electrical synapses in spiking networks. Phys. Rev. E 100, 042412. doi:10.1103/PhysRevE.100.042412
Pyragas, V., and Pyragas, K. (2022). Mean-field equations for neural populations with q-Gaussian heterogeneities. Phys. Rev. E 105, 044402. doi:10.1103/PhysRevE.105.044402
Pyragas, V., and Pyragas, K. (2023). Effect of cauchy noise on a network of quadratic integrate-and-fire neurons with non-cauchy heterogeneities. Phys. Lett. A 480, 128972. doi:10.1016/j.physleta.2023.128972
Ratas, I., and Pyragas, K. (2018). Macroscopic oscillations of a quadratic integrate-and-fire neuron network with global distributed-delay coupling. Phys. Rev. E 98, 052224. doi:10.1103/physreve.98.052224
Schmidt, H., Avitabile, D., Montbrió, E., and Roxin, A. (2018). Network mechanisms underlying the role of oscillations in cognitive tasks. PLoS Comput. Biol. 14, e1006430. doi:10.1371/journal.pcbi.1006430
Schöll, E. (2016). Synchronization patterns and chimera states in complex networks: interplay of topology and dynamics. Eur. Phys. J. Special Top. 225, 891–919. doi:10.1140/epjst/e2016-02646-3
Shilnikov, A., Gordon, R., and Belykh, I. (2008). Polyrhythmic synchronization in bursting networking motifs. Chaos An Interdiscip. J. Nonlinear Sci. 18, 037120. doi:10.1063/1.2959850
Skardal, P. S., Ott, E., and Restrepo, J. G. (2011). Cluster synchrony in systems of coupled phase oscillators with higher-order coupling. Phys. Rev. E 84, 036208. doi:10.1103/PhysRevE.84.036208
So, P., Luke, T. B., and Barreto, E. (2014). Networks of theta neurons with time-varying excitability: macroscopic chaos, multistability, and final-state uncertainty. Phys. D. Nonlinear Phenom. 267, 16–26. doi:10.1016/j.physd.2013.04.009
Somers, D., and Kopell, N. (1993). Rapid synchronization through fast threshold modulation. Biol. Cybern. 68, 393–407. doi:10.1007/BF00198772
Taher, H., Torcini, A., and Olmi, S. (2020). Exact neural mass model for synaptic-based working memory. PLoS Comput. Biol. 16, e1008533. doi:10.1371/journal.pcbi.1008533
Terman, D., Kopell, N., and Bose, A. (1998). Dynamics of two mutually coupled slow inhibitory neurons. Phys. D. Nonlinear Phenom. 117, 241–275. doi:10.1016/s0167-2789(97)00312-6
Van Vreeswijk, C., Abbott, L., and Bard Ermentrout, G. (1994). When inhibition not excitation synchronizes neural firing. J. Comput. Neurosci. 1, 313–321. doi:10.1007/BF00961879
Zillmer, R., Livi, R., Politi, A., and Torcini, A. (2007). Stability of the splay state in pulse-coupled networks. Phys. Rev. E 76, 046102. doi:10.1103/PhysRevE.76.046102
Keywords: network physiology, integrate-and-fire models, theta neurons, Kuramoto model, synaptic activation, time delay, synchronization, partial synchronization
Citation: Smirnov LA, Munyayev VO, Bolotov MI, Osipov GV and Belykh I (2024) How synaptic function controls critical transitions in spiking neuron networks: insight from a Kuramoto model reduction. Front. Netw. Physiol. 4:1423023. doi: 10.3389/fnetp.2024.1423023
Received: 25 April 2024; Accepted: 16 July 2024;
Published: 09 August 2024.
Edited by:
Eckehard Schöll, Technical University of Berlin, GermanyReviewed by:
Ernest Montbrio, Pompeu Fabra University, SpainSimona Olmi, National Research Council (CNR), Italy
Copyright © 2024 Smirnov, Munyayev, Bolotov, Osipov and Belykh. This is an open-access article distributed under the terms of the Creative Commons Attribution License (CC BY). The use, distribution or reproduction in other forums is permitted, provided the original author(s) and the copyright owner(s) are credited and that the original publication in this journal is cited, in accordance with accepted academic practice. No use, distribution or reproduction is permitted which does not comply with these terms.
*Correspondence: Igor Belykh, aWJlbHlraEBnc3UuZWR1