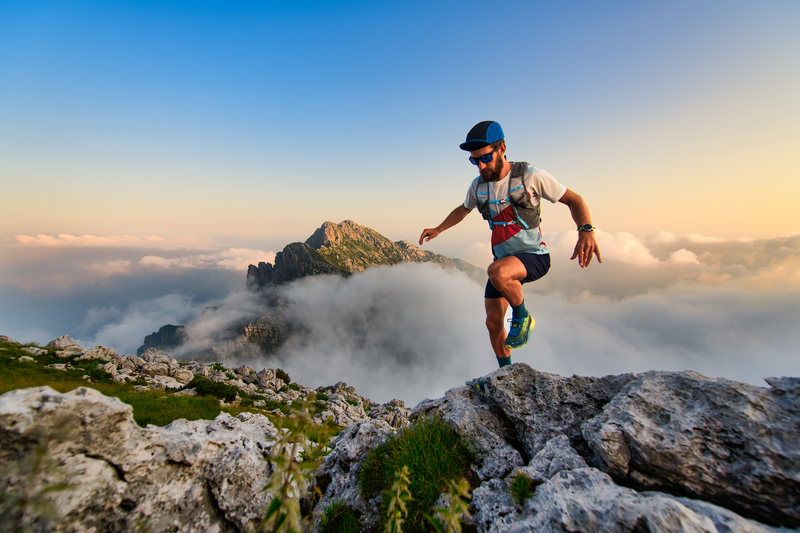
94% of researchers rate our articles as excellent or good
Learn more about the work of our research integrity team to safeguard the quality of each article we publish.
Find out more
OPINION article
Front. Netw. Physiol. , 19 May 2023
Sec. Network Physiology of Exercise
Volume 3 - 2023 | https://doi.org/10.3389/fnetp.2023.1190355
This article is part of the Research Topic Insights in Network Physiology of Exercise View all articles
On and off the sports field, athletes are confronted with numerous stressors. These stressors may reflect daily hassles, heavy training sessions, or occasionally major life events like losing a loved one (Den Hartigh et al., 2022). To prevent injuries or declines in performance and psychological wellbeing, athletes constantly need to demonstrate resilience following these stressors. While some scholars propose that resilience may refer to resisting, recovering from, or growing from a stressor’s negative impact (Masten and Powell, 2003), it has been pointed out that these are distinct concepts and that resilience most closely resembles a recovery-from-stressors process (e.g., Den Hartigh & Hill, 2022; Layne et al., 2008; Layne et al., 2021; Lozano Nasi et al., 2023; Taleb & West, 2023). Therefore, we proceed from the following definition of resilience: “the dynamic process by which a biopsychosocial system returns to the previous level of functioning, following a perturbation caused by a stressor” (Hill et al., 2018b, p. 367).
Defining resilience from such a dynamical perspective in sports implies that resilience emerges from continuously changing interactions between multiple psychological and physiological variables, and that it cannot be reduced to a single set of fixed factors (Hill et al., 2018a; Hill et al., 2018b; Hill et al., 2021). Practically, this means that strategies to successfully deal with a stressor in one situation, may not be effective in another situation. Moreover, changes among the factors do not yield proportional outcomes at the observable level (Kelso, 1995; Nowak & Vallacher, 1998). That is, relatively large changes in various factors may have virtually no impact on whether an athlete can demonstrate resilience, whereas relatively small changes of the same factors close to a tipping point may induce injuries or significant performance and wellbeing declines (cf. Pol et al., 2019). Thus, the way in which different factors dynamically interact and change over time needs to be clarified (Hill et al., 2018a).
In this article, we argue that the dynamic process of resilience in sports provides a logical fit with network structures (Pincus & Metten, 2010). Specifically, we demonstrate how networks a) fit with the contemporary conceptualization of resilience and b) can be studied to provide insights into resilience. Finally, new avenues for future research leveraging network analyses will be provided.
A network represents a collection of interconnected variables or nodes that exchange information with each other (Balagué et al., 2020; Bartsch et al., 2015; Bashan et al., 2012; Pincus & Metten, 2010). Networks can be used to model small-scaled systems, such as neurons within the brain, as well as large-scaled systems like societies where each node represents a person. In any network, the nodes and their interaction patterns may change over time (see Figure 1; Balagué et al., 2020; Bartsch et al., 2015; Bashan et al., 2012; Den Hartigh and Hill, 2022; Den Hartigh et al., 2018). These so-called intrinsic dynamics (Vallacher et al., 2015; Gernigon et al., 2022) allow a network to reorganize itself in response to an external perturbation (Pincus & Metten, 2010; Bashan et al., 2012; Kiefer et al., 2018). In terms of resilience, a network structure may be perturbed by a stressor, but restores its previous configuration over time. Furthermore, due to their dynamic interactions, networks also allow for the nonlinear influences of the constituent nodes (Bashan et al., 2012; Bartsch et al., 2015). That is, the very structure of a network may either amplify or dampen the perturbation of a stressor (Gao et al., 2016). Therefore, network structures and how they change over time in response to perturbations can provide valuable insights into the dynamic process of resilience. Accordingly, recent advances in the domain of physiology have applied network analyses to capture critical transitions on the level of behavior and movement (Kerkman et al., 2020; Garcia-Retortillo and Ivanov, 2022).
FIGURE 1. Example of a network with sport-specific psychological (red), and physiological (blue) nodes that changes over time due to intrinsic dynamics. The specific nodes can include motivation and perceived stress on the psychological side as well as training load and muscle tension on the physiological side, but likely differ between individuals (Hill et al., 2021). The nodes change in strength (indicated by their size) and how they are connected to each other. At T1, the individual nodes are relatively loosely connected, but become relatively densely connected across time, which is expressed in the increase in connections as well as the changes in the levels (size) of the variables at T2. As a hypothetical example, due to high motivation, an athlete may invest successively more time and energy into their training, causing the load (and other associated physiological parameters, such as muscular strength) to grow as well. However, once the training load cannot be increased anymore, the connection between motivation and training load may start to dissolve. Instead, the athlete may use their high levels of motivation to focus on mental skills training, leading to the formation of new connections between motivation and arousal management. Note that changes in the nodes and connectivity may also occur following an external perturbation. Whether structural changes in the connectivity are beneficial for an athlete depends on the previous state of the network. Increasing connectivity may be beneficial when the connectivity was too low previously, but problematic when the network becomes too densely connected and therefore rigid (e.g., Scheffer et al., 2012). The figure was created using the free software Loopy (https://ncase.me/loopy/).
In order to prevent injuries or declines in performance and wellbeing in athletes, providing predictions about an athlete’s capacity to demonstrate resilience is an important avenue (Den Hartigh et al., 2022). These predictions may become possible by capturing the structures of relevant networks (Gao et al., 2016). For example, according to Scheffer and colleagues (2012), a network that consists of many tightly coupled nodes may be more prone to collapse following a perturbation compared to a network that shows more heterogeneity in its connectivity. That is, networks with too many nodes characterized by high in-degrees (i.e., a measure of connectivity, Jia and Barabasi, 2013) become increasingly fragile because the perturbation spreads through the entire system causing a “domino effect” (Ghoshal and Barabasi, 2011; Bashan et al., 2012; Scheffer et al., 2012).
The spread of a perturbation throughout a network may further be enhanced or dampened by specific variables that are associated with resilience. For example, protective factors may reduce the perturbation of a stressor and contribute to resistance, while promotive factors may facilitate the reorganization process following a perturbation (Layne et al., 2008; 2021). Simulation studies from the domain of clinical psychology showed that when a risk factor represents a central node with high connectivity, it may enhance the spread of a perturbation throughout a symptom network (Lunansky et al., 2021). These findings have also been verified with empirical data of personality network structures in response to stress (Papageorgiou et al., 2019). In contrast, a central protective factor dampens the perturbation caused by a stressor and avoids the spread from one symptom to another (Kalisch et al., 2019; Papageorgiou et al., 2020). Because several outcomes are relevant for athletes (i.e., physical health, performance, and psychological wellbeing), different protective and risk factors may be specified for different levels of functioning. However, it should be noted that due to the intrinsic dynamics, the role of these nodes may change over time. Therefore, central issues like injury prevention may not only be a question of monitoring the right variables over time (Den Hartigh et al., 2022), but also understanding the changing interconnectivity of these variables (Andrews et al., 2022; Balagué et al., 2022).
Compared to the notion that network structures can explain resilience, the structural changes that networks undergo to become resilient are relatively unexplored (e.g., Pincus & Metten, 2010). In exercise physiology, the general interest in tracking how interactions between organ systems (e.g., brain, heart, skeletal muscles) change in response to fatigue and training through the assessment of “network-based biomarkers” has, however, already gained traction (e.g., Balague et al., 2022). Regarding the concept of resilience, researchers suggested that a system’s underlying structure may change in response to a perturbation to become more rigid and stable or more flexible without losing its functionality (Pincus & Metten, 2010). Specifically, following a perturbation, new connections between individual nodes may be formed (i.e., integration tendency, Kiefer et al., 2018), which allows a system to preserve its stability. In contrast, to avoid becoming trapped in dysfunctional states, connections between specific nodes may be dissolved (i.e., segregation tendency, Kiefer et al., 2018). Thus, while on a superordinate level (i.e., performance or health), we observe a recovery trajectory, the underlying network structure could have undergone changes (Bashan et al., 2012; Bartsch et al., 2015). These changes may help an athlete to bounce back more quickly when similar perturbations occur in the future.1 Therefore, the changes in the underlying network structure may explain how resilience in superordinate variables in sports, such as performance or physical and psychological wellbeing, may be improved. For example, when an athlete experiences psychological problems, dissolving tight connectivity between physiological and psychological nodes may be beneficial for an athlete to prevent physical injuries. Conversely, increasing the connectivity may be functional when either psychological or physiological nodes can help buffer against external perturbations (cf. Balagué et al., 2020).
Network structures may also provide insight into resilience losses. Previous research has shown that successive stressors can destabilize a system and reduce the capacity for resilience (Scheffer et al., 2012). In athletes, such resilience losses may be marked by a slowing down in the recovery rate to the previous state (i.e., “critical slowing down”), and can ultimately lead to sudden declines in the athletes’ performance or wellbeing (e.g., Hill et al., 2018a; 2020; Hill et al., 2021; Den Hartigh et al., 2022). This means that the network would require increasingly more time to restore its previous structure.2 Mapping the stress-response as well as the time it takes for an athlete to return to the previous state can provide valuable insights into when preventive measures need to be taken (Hill et al., 2018a). Additionally, by gaining a better understanding of the individual-specific configuration of a network, the timed interventions may be targeted at the risk factors at hand (Lunansky et al., 2022). For example, if the recovery in the physical state and wellbeing of an athlete slows down following normal training loads, the training load may be temporarily reduced (e.g., taking a day off) before negative transitions to physical or psychological problems occur.
In order to identify changes in the network structures that underlie resilience, daily measurements of multiple variables would need to be collected. Such data collections may be conducted around the daily training sessions of athletes and can include (but are not limited to) variables like recovery from the previous sessions, motivation to train, training load, or enjoyment of the training session (Den Hartigh et al., 2022). Note that specific analytic strategies for this kind of data are beyond the scope of this paper. Therefore, we refer readers to Pincus and Metten (2010) for different examples of resilience-specific network analyses (see also Blanken et al., 2019), and Hasselman (2022) for a state-of-the-art multiplex recurrence network technique.3
Because the above outlined data collection protocols can be time-consuming or unavailable to researchers, alternative approaches may focus on simulation studies. Dynamic network modelling has already been applied to the domain of sports. For example, Den Hartigh and colleagues (2018) used a model based on coupled differential equations to determine talent development in sports. Interestingly, these simulations also contained a perturbation which needed to be overcome in the form of transitioning from the youth to the senior level. Such models can be 1) varied with regards to what variables should be included to represent the nodes and how the interactions between the nodes may change over time, and 2) examined for their specific structure and changes to identify when external stressors cause stronger or weaker perturbations (Gao et al., 2016). Therefore, stimulation studies may be particularly promising when adequate timeseries data collection is limited.
In this article, we discussed the potential of network analyses to provide more in-depth insights into the dynamic process of resilience in sports. We argued that networks yield a striking resemblance with the key properties of resilience and provide a logical fit as a level of analysis. Specifically, the intrinsic dynamics and interaction-dominance of networks may account for nonlinear changes of resilience and explain how the process unfolds over time. We therefore propose that network analyses can provide a powerful future avenue for studying resilience in sports, because they may not only indicate what makes an athlete resilient, but also how resilience in athletes changes over time. From our perspective, the field can make major advances by either closely monitoring and analyzing network structures of physiological and psychological variables of athletes or engaging in simulation studies of how networks respond to perturbations over time.
Both YH and RD conceived the idea for the paper, wrote, and edited the manuscript. Both authors contributed equally to this work. All authors listed have made a substantial, direct, and intellectual contribution to the work and approved it for publication.
The authors declare that the research was conducted in the absence of any commercial or financial relationships that could be construed as a potential conflict of interest.
All claims expressed in this article are solely those of the authors and do not necessarily represent those of their affiliated organizations, or those of the publisher, the editors and the reviewers. Any product that may be evaluated in this article, or claim that may be made by its manufacturer, is not guaranteed or endorsed by the publisher.
1Note that if a stressor is resisted and no perturbation occurs, the functional reorganization of the network may not occur (Den Hartigh & Hill, 2022).
2The same functional output may also be achieved from different network configurations (i.e., degeneracy, Edelman & Gally, 2001). Therefore, a precise definition of the level of functioning to which the system returns is warranted.
3Inspirations for additional sophisticated approaches for network-based analyses can also be inferred from recent publications in the domain of network physiology (e.g., Bashan et al., 2012; Bartsch et al., 2015; Rizzo et al., 2020; Rizzo et al., 2022; Garcia-Retortillo et al., 2023).
Andrews, E., Jildeh, T. R., Abbas, M. J., Lindsay-Rivera, K., Berguson, J., and Okoroha, K. R. (2022). Concussions in the national hockey league: Analysis of incidence, return to play, and performance. Orthop. J. Sports Med. 10 (1), 23259671211052069. doi:10.1177/23259671211052069
Balagué, N., Hristovski, R., Almarcha, M. D. C., Garcia-Retortillo, S., and Ivanov, P. C. (2020). Network physiology of exercise: Vision and perspectives. Front. Physiol. 11, 611550. doi:10.3389/fphys.2020.611550
Balagué, N., Hristovski, R., Almarcha, M., Garcia-Retortillo, S., and Ivanov, P. C. (2022). Network physiology of exercise: Beyond molecular and omics perspectives. Sports Med. Open 8 (1), 119. doi:10.1186/s40798-022-00512-0
Bartsch, R. P., Liu, K. K., Bashan, A., and Ivanov, P. C. (2015). Network physiology: How organ systems dynamically interact. PloS One 10 (11), e0142143. doi:10.1371/journal.pone.0142143
Bashan, A., Bartsch, R. P., Kantelhardt, J. W., Havlin, S., and Ivanov, P. C. (2012). Network physiology reveals relations between network topology and physiological function. Nat. Commun. 3, 702. doi:10.1038/ncomms1705
Blanken, T. F., Van Der Zweerde, T., Van Straten, A., Van Someren, E. J., Borsboom, D., and Lancee, J. (2019). Introducing network intervention analysis to investigate sequential, symptom-specific treatment effects: A demonstration in co-occurring insomnia and depression. Psychother. Psychosom. 88 (1), 52–54. doi:10.1159/000495045
Den Hartigh, R. J. R., and Hill, Y. (2022). Conceptualizing and measuring psychological resilience: What can we learn from physics? New Ideas Psychol. 66, 100934. doi:10.1016/j.newideapsych.2022.100934
Den Hartigh, R. J. R., Hill, Y., and Van Geert, P. L. C. (2018). The development of talent in sports: A dynamic network approach. Complexity., 2018, 1. 13. doi:10.1155/2018/9280154
Den Hartigh, R. J. R., Meerhoff, L. R. A., Van Yperen, N. W., Neumann, N. D., Brauers, J. J., Frencken, W. G. P., et al. (2022). Resilience in sports: A multidisciplinary, dynamic, and personalized perspective. Int. Rev. Sport Exerc. Psychol., 1–23. –23. doi:10.1080/1750984X.2022.2039749
Edelman, G. M., and Gally, J. A. (2001). Degeneracy and complexity in biological systems. Proc. Natl. Acad. Sci. 98 (24), 13763–13768. doi:10.1073/pnas.231499798
Gao, J., Barzel, B., and Barabási, A. L. (2016). Universal resilience patterns in complex networks. Nature 530 (7590), 307–312. doi:10.1038/nature16948
Garcia-Retortillo, S., Gomez, C. R., and Ivanov, P. (2023). Functional networks of muscle fibers facilitate inter-muscular coordination. ResearchSquare. doi:10.21203/rs.3.rs-2671338/v1
Garcia-Retortillo, S., and Ivanov, P. C. (2022). Inter-muscular networks of synchronous muscle fiber activation. Front. Netw. Physiol. 2, 1059793. doi:10.3389/fnetp.2022.1059793
Gernigon, C., den Hartigh, R. J. R., Vallacher, R. R., and van Geert, P. (2022). How the complexity of psychological processes reframes the issue of reproducibility in psychological science. PsyArXiv.
Ghoshal, G., and Barabási, A. L. (2011). Ranking stability and super-stable nodes in complex networks. Nat. Comm. 2 (1), 394. doi:10.1038/ncomms1396
Hasselman, F. (2022). Early warning signals in phase space: Geometric resilience loss indicators from multiplex cumulative recurrence networks. Front. Physiol. 13, 859127. doi:10.3389/fphys.2022.859127
Hill, Y., Den Hartigh, R. J. R., Cox, R. F., De Jonge, P., and Van Yperen, N. W. (2020). Predicting resilience losses in dyadic team performance. Nonlinear Dyn. Psychol. Life Sci. 24 (3), 327–351.
Hill, Y., Den Hartigh, R. J. R., Meijer, R. R., De Jonge, P., and Van Yperen, N. W. (2018a). Resilience in sports from a dynamical perspective. Sport exerc. Perform. Psychol. 7 (4), 333–341. doi:10.1037/spy0000118
Hill, Y., Den Hartigh, R. J. R., Meijer, R. R., De Jonge, P., and Van Yperen, N. W. (2018b). The temporal process of resilience. Sport exerc. Perform. Psychol. 7 (4), 363–370. doi:10.1037/spy0000143
Hill, Y., Meijer, R. R., Van Yperen, N. W., Michelakis, G., Barisch, S., and Den Hartigh, R. J. R. (2021). Nonergodicity in protective factors of resilience in athletes. Sport exerc. Perform. Psychol. 10 (2), 217–223. doi:10.1037/spy0000246
Hill, Y., Van Yperen, N. W., and Den Hartigh, R. J. R. (2021). Facing repeated stressors in a motor task: Does it enhance or diminish resilience? J. Mot. Behav. 52 (6), 717–726. doi:10.1080/00222895.2020.1852155
Jia, T., and Barabási, A. L. (2013). Control capacity and a random sampling method in exploring controllability of complex networks. Sci. Rep. 3 (1), 2354–2356. doi:10.1038/srep02354
Kalisch, R., Cramer, A. O., Binder, H., Fritz, J., Leertouwer, I., Lunansky, G., et al. (2019). Deconstructing and reconstructing resilience: A dynamic network approach. Perspect. Psychol. Sci. 14 (5), 765–777. doi:10.1177/1745691619855637
Kerkman, J. N., Bekius, A., Boonstra, T. W., Daffertshofer, A., and Dominici, N. (2020). Muscle synergies and coherence networks reflect different modes of coordination during walking. Front. Physiol. 11, 751. doi:10.3389/fphys.2020.00751
Kiefer, A. W., Silva, P. L., Harrison, H. S., and Araújo, D. (2018). Antifragility in sport: Leveraging adversity to enhance performance. Sport exerc. Perform. Psychol. 7 (4), 342–350. doi:10.1037/spy0000130
Layne, C. M., Beck, C. J., Rimmasch, H., Southwick, J. S., Moreno, M. A., and Hobfoll, S. E. (2008). “Promoting “resilient” posttraumatic adjustment in childhood and beyond: “Unpacking” life events, adjustment trajectories, resources, and interventions,” in Treating traumatized children: Risk, resilience, and recovery. Editors D. Brom, R. Pat-Horenczyk, and J. Ford (London, United Kingdom: Routledge), 13–49.
Layne, C. M., Ruzek, J. I., and Dixon, K. (2021). From resilience and restoration to resistance and resource caravans: A developmental framework for advancing the disaster field. Psychiatry 84 (4), 393–409. doi:10.1080/00332747.2021.2005444
Lozano Nasi, V., Jans, L., and Steg, L. (2023). Can we do more than “bounce back”? Transilience in the face of climate change risks. J. Environ. Psychol. 86, 101947. doi:10.1016/j.jenvp.2022.101947
Lunansky, G., Naberman, J., van Borkulo, C. D., Chen, C., Wang, L., and Borsboom, D. (2022). Intervening on psychopathology networks: Evaluating intervention targets through simulations. Methods 204, 29–37. doi:10.1016/j.ymeth.2021.11.006
Lunansky, G., Van Borkulo, C. D., Haslbeck, J. M., Van der Linden, M. A., Garay, C. J., Etchevers, M. J., et al. (2021). The mental health ecosystem: Extending symptom networks with risk and protective factors. Front. Psychiatry 12, 640658. doi:10.3389/fpsyt.2021.640658
Masten, A. S., and Powell, J. L. (2003). “A resilience framework for research, policy, and practice,” in Resilience and vulnerabilities: Adaptation in the context of childhood adversities. Editor S. S. Luthar (New York: Cambridge University Press), 1–25.
Papageorgiou, K. A., Benini, E., Bilello, D., Gianniou, F. M., Clough, P. J., and Costantini, G. (2019). Bridging the gap: A network approach to dark triad, mental toughness, the big five, and perceived stress. J. Pers. 87 (6), 1250–1263. doi:10.1111/jopy.12472
Papageorgiou, K. A., Likhanov, M., Costantini, G., Tsigeman, E., Zaleshin, M., Budakova, A., et al. (2020). Personality, behavioral strengths and difficulties and performance of adolescents with high achievements in science, literature, art and sports. Pers. Indiv. Differ. 160, 109917. doi:10.1016/j.paid.2020.109917
Pincus, D., and Metten, A. (2010). Nonlinear dynamics in biopsychosocial resilience. Nonlinear Dyn. Psychol. Life Sci. 14, 353–380.
Pol, R., Hristovski, R., Medina, D., and Balague, N. (2019). From microscopic to macroscopic sports injuries. Applying the complex dynamic systems approach to sports medicine: A narrative review. Br. J. Sports Med. 53 (19), 1214–1220. doi:10.1136/bjsports-2016-097395
Rizzo, R., Garcia-Retortillo, S., and Ivanov, P. C. (2022). Dynamic networks of physiologic interactions of brain waves and rhythms in muscle activity. Hum. Mov. Sci. 84, 102971. doi:10.1016/j.humov.2022.102971
Rizzo, R., Zhang, X., Wang, J. W., Lombardi, F., and Ivanov, P. C. (2020). Network physiology of cortico–muscular interactions. Front. Physiol. 11, 558070. doi:10.3389/fphys.2020.558070
Scheffer, M., Carpenter, S. R., Lenton, T. M., Bascompte, J., Brock, W., Dakos, V., et al. (2012). Anticipating critical transitions. Science 338 (6105), 344–348. doi:10.1126/science.1225244
Taleb, N. N., and West, J. (2023). Working with convex responses: Antifragility from finance to oncology. Entropy 25 (2), 343. doi:10.3390/e25020343
Keywords: dynamical systems (DS), growth, mental wellbeing, resistance, performance, physiology, psychology
Citation: Hill Y and Den Hartigh RJR (2023) Resilience in sports through the lens of dynamic network structures. Front. Netw. Physiol. 3:1190355. doi: 10.3389/fnetp.2023.1190355
Received: 20 March 2023; Accepted: 12 May 2023;
Published: 19 May 2023.
Edited by:
Ginés Viscor, University of Barcelona, SpainReviewed by:
Maxim Likhanov, State Key Laboratory of Cognitive Neuroscience and Learning of Beijing Normal University, Bejing, CNCopyright © 2023 Hill and Den Hartigh. This is an open-access article distributed under the terms of the Creative Commons Attribution License (CC BY). The use, distribution or reproduction in other forums is permitted, provided the original author(s) and the copyright owner(s) are credited and that the original publication in this journal is cited, in accordance with accepted academic practice. No use, distribution or reproduction is permitted which does not comply with these terms.
*Correspondence: Yannick Hill, eS5oaWxsQHZ1Lm5s
Disclaimer: All claims expressed in this article are solely those of the authors and do not necessarily represent those of their affiliated organizations, or those of the publisher, the editors and the reviewers. Any product that may be evaluated in this article or claim that may be made by its manufacturer is not guaranteed or endorsed by the publisher.
Research integrity at Frontiers
Learn more about the work of our research integrity team to safeguard the quality of each article we publish.