- 1National Medical Research Center for Therapy and Preventive Medicine, Moscow, Russia
- 2Smart Sleep Laboratory, Saratov State University, Saratov, Russia
- 3Laboratory of Nonlinear Dynamics Modeling, Saratov Branch of Kotelnikov Institute of Radio Engineering and Electronics of Russian Academy of Sciences, Saratov, Russia
- 4Institute of Cardiological Research, Saratov State Medical University, Saratov, Russia
- 5Interdisciplinary Sleep Medicine Center, Charité—Universitätsmedizin Berlin, Berlin, Germany
Cardiorespiratory interactions are important, both for understanding the fundamental processes of functioning of the human body and for development of methods for diagnostics of various pathologies. The properties of cardiorespiratory interaction are determined by the processes of autonomic control of blood circulation, which are modulated by the higher nervous activity. We study the directional couplings between the respiration and the process of parasympathetic control of the heart rate in the awake state and different stages of sleep in 96 healthy subjects from different age groups. The detection of directional couplings is carried out using the method of phase dynamics modeling applied to experimental RR-intervals and the signal of respiration. We reveal the presence of bidirectional couplings between the studied processes in all age groups. Our results show that the coupling from respiration to the process of parasympathetic control of the heart rate is stronger than the coupling in the opposite direction. The difference in the strength of bidirectional couplings between the considered processes is most pronounced in deep sleep.
Introduction
The study of interaction between the human cardiac and respiratory systems attracts a lot of attention. The most studied types of cardiorespiratory interaction are the respiratory sinus arrhythmia (Angelone and Coulter, 1964; Schäfer et al., 1998; Song and Lehrer, 2003), which explains the variation of the heart rate within a breathing cycle, and the cardiorespiratory phase synchronization (Rosenblum et al., 1998; Schäfer et al., 1998; Schäfer et al., 1999; Mrowka et al., 2000; Prokhorov et al., 2003), which is defined as the occurrence of heartbeats in certain phases of the respiratory cycles. The methods based on calculation of cross-spectral coherence (White and Boashash, 1990; Quian Quiroga et al., 2002) and detection of synchronization (Rosenblum et al., 1998; Schäfer et al., 1999; Mrowka et al., 2000; Pikovsky et al., 2001; Rosenblum et al., 2001; Rosenblum and Pikovsky, 2001; Schelter et al., 2006a; Karavaev et al., 2009) helped to understand the cardiorespiratory interaction from a physiological point of view (Ivanov et al., 1998; Kantelhardt et al., 2002; Keener and Sneyd, 2009; Schumann et al., 2010). It has been shown that characteristics of the cardiorespiratory interaction change during sleep (Bunde et al., 2000; Kantelhardt et al., 2003; Bartsch et al., 2007; Schmitt et al., 2009; Schumann et al., 2010; Müller et al., 2014; Riedl et al., 2014; Karavaev et al., 2021) and during healthy aging (Bartsch et al., 2012; Ponomarenko et al., 2021), differ in newborns (Mrowka et al., 2000), and depend on the gender of the subjects (Shiogai et al., 2010). They can be used for predicting complications of cardiovascular diseases (Dougherty and Burr, 1992; Hohnloser et al., 1994; Ishbulatov et al., 2020) and help to understand the mechanism of neural control of the cardiovascular and respiratory systems (Sayers, 1973; Lown and Verrier, 1976; Akselrod et al., 1981; Task Force of the European Society of Cardiology and the North American Society of Pacing and Electrophysiology, 1996; Malberg et al., 2002; Prokhorov et al., 2005a; Karavaev et al., 2013; Ishbulatov et al., 2020).
In order to get a more detailed understanding of the mechanism of cardiorespiratory coupling, many authors study the driver-response (causal) relationships, or directionality of coupling using the methods based on Granger causality (Baccala et al., 1998; Baccala and Sameshima, 2001; Kaminski et al., 2001; Porta et al., 2002; Faes et al., 2004; Astolfi et al., 2006; Schelter et al., 2006b; Faes et al., 2006; Baccala et al., 2007; Faes and Nollo, 2010; Milde et al., 2011; Porta et al., 2012; Schulz et al., 2013; Faes et al., 2015), entropy (Schreiber, 2000; Hoyer et al., 2002; Kaiser and Schreiber, 2002; Bhattacharya et al., 2003; Ancona et al., 2004; Verdes, 2005; Bahraminasab et al., 2008; Faes et al., 2011), and modeling of phase dynamics (Schäfer et al., 1998; Censi et al., 2002; Rosenblum et al., 2002; Bartsch et al., 2007). It has been shown that in healthy infants, the direction of coupling between cardiovascular and respiratory systems evolves from approximately symmetric coupling during the first days of life to nearly unidirectional (from respiration to the cardiovascular system) after 6 months of age (Rosenblum et al., 2002). For a large database of healthy subjects, it has been shown that the intensity of influence is much stronger from respiration to heart than in the opposite direction and the direction of coupling from respiration to the main heart rhythm is dominant throughout life (Faes et al., 2004; Faes and Nollo, 2010; Porta et al., 2012) and does not depend on the subject’s gender (Mrowka et al., 2003; Bartsch et al., 2007; Bahraminasab et al., 2008; Shiogai et al., 2010) or sleep stage (Mrowka et al., 2003). However, the intensity of influence from respiration to heart decreases with age (Shiogai et al., 2010) and during active standing or head-up tilt protocols (Nollo et al., 2005; Faes et al., 2011; Porta et al., 2012; Faes et al., 2015) and changes under anesthesia (Stankovski et al., 2016). On the other hand, the intensity of influence from heart to respiration remains constant with age (Iatsenko et al., 2013). In spontaneously breathing patients under general anesthesia (Galletly and Larsen, 1999) and in the case of so-called dynamic diseases such as apnea, the mechanisms of cardiorespiratory interaction and feedback between heart rate and respiration are disrupted, leading to an increase in the directional coupling from the main heart rhythm to respiration (Schreiber, 2000; Kaiser and Schreiber, 2002; Bhattacharya et al., 2003; Ancona et al., 2004; Verdes, 2005).
Causal relationships between the human cardiac and respiratory systems were studied mainly between the main heart rhythm with a frequency of about 1 Hz and respiration whose frequency is usually around 0.25 Hz. Another aspect of the cardiorespiratory interaction is associated with the relationships between the respiration and fluctuations of the heart rate in the high-frequency (HF) range 0.15–0.4 Hz. The occurrence of fluctuations in the sequence of RR-intervals in the HF range is associated with a number of factors, including the parasympathetic control of the heart rate (Task Force of the European Society of Cardiology and the North American Society of Pacing and Electrophysiology, 1996; Porges, 1995; Lewis et al., 2006; Prokhorov et al., 2021), intrathoracic pressure changes, and indirect influence of interaction between central generators of cardiorespiratory rhythms and peripheral factors (tonic and phasic baroreceptor and chemoreceptor reflexes, cardiac and pulmonary stretch reflexes, local chemical and metabolic factors, etc.) (Berntson et al., 1993). Recently, we have shown the decrease of coherence between the respiration and parasympathetic control of the heart rate with aging in healthy subjects (Ponomarenko et al., 2021). The coherence between these processes depends on the stage of sleep (Ponomarenko et al., 2021).
In this paper, we study the directional couplings between the respiration and the process of parasympathetic control of the heart rate in healthy subjects. We investigate whether these directional couplings depend on age and the stages of sleep.
Materials and methods
Study participants
Our study included 96 healthy subjects (59 females and 37 males), who were divided into four groups depending on age. The first group included 36 subjects aged 20–34 years, the second group included 23 subjects aged 35–49 years, the third group included 17 subjects aged 50–64 years, and the fourth group included 20 subjects in ages 65 and older. The data were recorded at the sleep laboratories within the European Union project SIESTA (Klösch et al., 2001). The study was approved by the local institutional review boards of the sleep centers involved. All study participants provided written informed consent. Exclusion criteria subjects for the healthy group were obstructive apnea and hypopnea and identified pathologies of the respiratory, cardiovascular, and neural system.
Data preprocessing
The signals of respiration and electrocardiogram (ECG) were simultaneously recorded within 8 h at night for each subject. The respiratory signal was recorded with a sampling frequency of 20 Hz using a thermistor oronasal respiration flow sensor. The ECG signal was recorded with a sampling frequency of 200 Hz. We detected the epochs of wakefulness, rapid eye movement (REM) sleep, light sleep S2 (LS), and deep sleep S3 (DS) in accordance with the classification (Rechtschaffen and Kales, 1968). We analyzed the first 5-min segments of the detected epochs without artifacts in ECG and respiratory signals.
From the ECG signal, we extracted a sequence of RR-intervals, i.e., a series of time intervals between the two successive R peaks, in accordance with the standards of heart rate variability (HRV) measurement (Task Force of the European Society of Cardiology the North American Society of Pacing Electrophysiology, 1996). To obtain equidistant time series from not equidistant sequence of RR-intervals we approximated it with cubic splines and resampled with a frequency of 20 Hz.
To extract the high-frequency (HF) component of HRV associated with the process of parasympathetic control of the heart rate, we filtered the sequence of RR-intervals using a rectangular digital filter with the bandpass of 0.15–0.50 Hz. In a similar way, we filtered the respiratory signal with the same bandpass filter. The filtered signals of respiration and RR-intervals are denoted as x1(t) and x2(t), respectively.
Indices of directional coupling
Using the filtered signals x1(t) and x2(t) we calculated the indices of directional coupling between the respiration and parasympathetic control of the heart rate during sleep and wakefulness in each subject. To calculate these indices, we used the method based on modeling the phase dynamics (Rosenblum and Pikovsky, 2001; Rosenblum et al., 2002; Smirnov and Bezruchko, 2003). The main idea of this method is to estimate how strongly the future evolution of the phase of the first (second) system depends on the current value of the phase of the second (first) system.
First, from time series of the signals x1(t) and x2(t), we obtain the time series of their instantaneous phases φ1(t) and φ2(t), respectively, using the Hilbert transform (Gabor, 1946; Panter, 1965; Pikovsky et al., 1997). Then, we construct stochastic differential equations modeling the phase dynamics of oscillatory processes:
where
where
The intensity of influence of the second system on the first one,
The derivatives
This normalization has disadvantages, since if the variances
The normalized index
For each subject in the awake state and different stages of sleep, we varied the trial delay time Δ from −5 to 5 s and calculated the indices
Statistical significance of estimated indices
To estimate a statistical significance of indices
To evaluate a statistical significance of differences in the estimates of calculated indices in different groups of subjects, we used the Mann-Whitney U-test (Mann and Whitney, 1947).
Results
Figure 1 shows short fragments of typical experimental signals for a healthy young subject in the awake state. The signal of respiration is presented in Figure 1A and the sequence of RR-intervals is presented in Figure 1B. Figures 1C,D show the time series of the signals x1(t) and x2(t), respectively, obtained by bandpass filtering of the signal of respiration and RR-intervals, respectively, in the 0.15–0.50 Hz band.
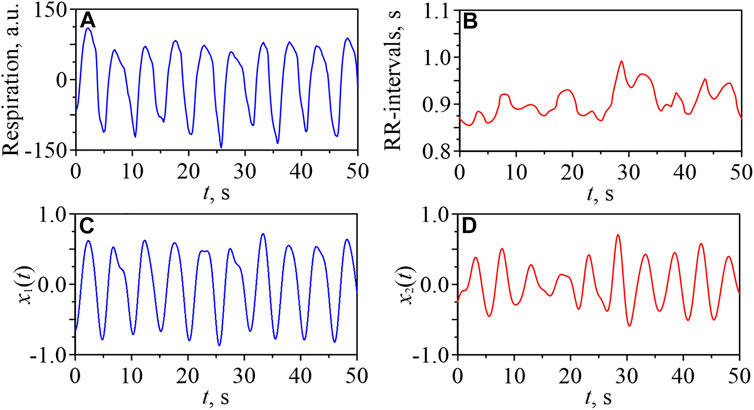
FIGURE 1. Fragments of a respiratory signal (A) and a sequence of RR-intervals (B) for one of the young subjects in the awake state. Filtered signals x1(t) (C) and x2(t) (D).
For each subject in the awake state, LS, DS, and REM sleep, we calculated the indices
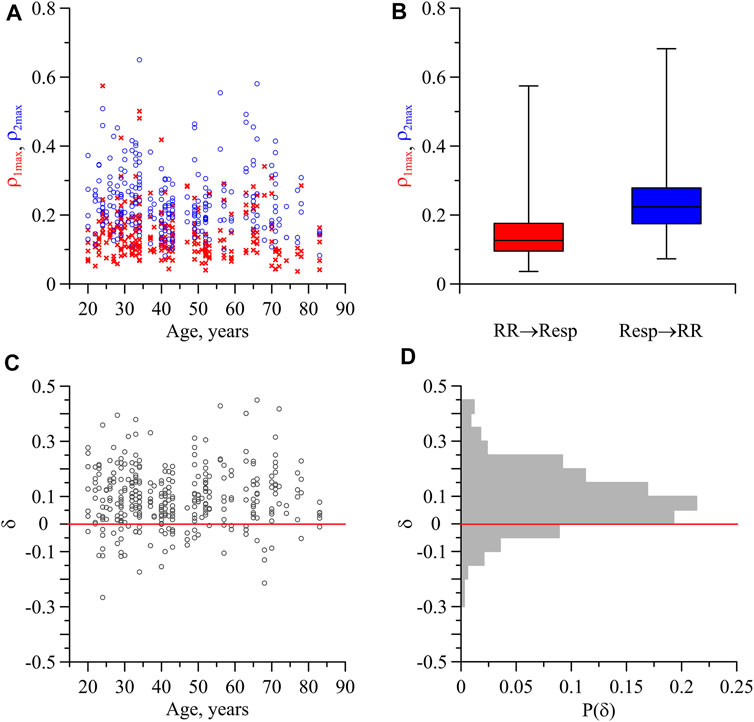
FIGURE 2. Indices of directional coupling between the respiration and the process of parasympathetic control of the heart rate. (A) Values of
In Figure 2B, the box-and-whisker diagrams for the indices
Figure 2C shows the index δ characterizing the difference between the directional coupling indices
Figure 3A shows the statistically significant (p = 0.05) indices
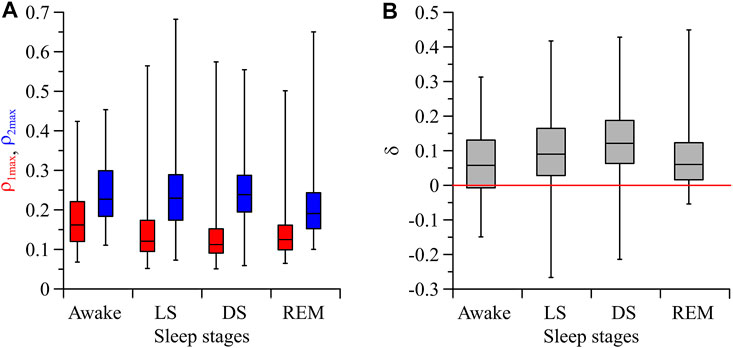
FIGURE 3. (A) Box-and-whisker diagrams for the values of
Figure 4A shows the indices of directional coupling
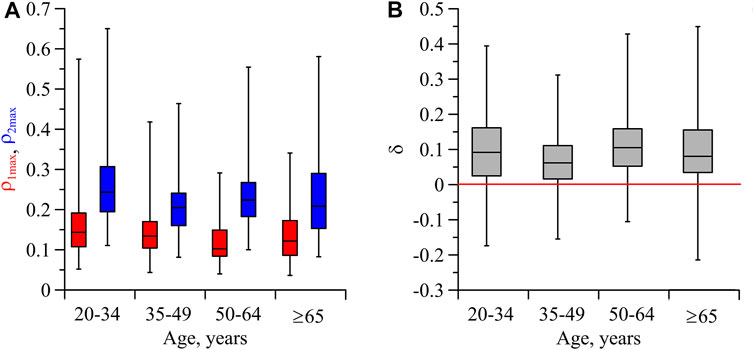
FIGURE 4. (A) Box-and-whisker diagrams for the values of
Discussion
In the present study, we analyzed the directional couplings between the respiration and the process of parasympathetic control of the heart rate in healthy subjects. It is known that parasympathetic fibers innervate the smooth muscle tone of the respiratory tract, providing regulation of microvasculature in the respiratory tract and realizing a direct directional coupling from parasympathetic regulation to the respiration. We understand that the respiratory tract is complex and involves several different muscle groups along upper airways, and for respiratory work such as the diaphragm. At the same time, feedback loops from the pulmonary stretch receptors and arterial baroreceptors act through the nucleus tractus solitarii on the Bötzinger complex located in the pontomedullary region of the pons, which provides regulation of the cardiovagal parasympathetic outflow by the respiratory pattern generator (Guyenet, 2014). Besides, the heart rate also responds to intrathoracic pressure changes caused by the respiration cycle (Berntson et al., 1993).
Taking into account the complex structure of interactions between the elements involved in the cardiorespiratory interaction, the obtained results can be interpreted as the presence of a dominant influence of respiration on the set of factors that form oscillations in the HF range of RR-intervals (in particular, vagal activity). The influence in the opposite direction is less pronounced. Moreover, it turns out that the degree of asymmetry in these directional couplings depends on the subject’s psychophysical state, which changes in different stages of sleep.
The obtained results are consistent with the results of studies, in which the dominant direction of coupling from respiration to the main heart rhythm was observed for healthy subjects of different ages (Faes et al., 2004; Faes and Nollo, 2010; Porta et al., 2012; Iatsenko et al., 2013) and it was found to be independent of subject gender (Mrowka et al., 2003; Bartsch et al., 2007; Bahraminasab et al., 2008; Shiogai et al., 2010) or sleep stage (Mrowka et al., 2003).
Moreover, the asymmetry in the coupling is more pronounced in LS and DS compared to the awake state and REM-sleep. This indicates the influence of the sympatho-vagal balance on the direction of coupling between the studied processes. The mean value of the index
At the same time, it was shown that under certain conditions (e.g., anesthesia) the dominant direction of coupling could be from the heart to respiration (Galletly and Larsen, 1999). Although this work considered a different frequency range, associated mainly with the main frequency of the heart rate, such conclusions indicate the relationship between the dominant direction of the cardiorespiratory interaction and the psychophysical state of the subject.
To calculate the indices
We detected the bidirectional coupling between the respiration and parasympathetic control of the heart rate in healthy subjects at different ages both during sleep and wakefulness. This result is consistent with the results of the studies (Galletly and Larsen, 1999; Iatsenko et al., 2013), which reported the presence of bidirectional interaction between the main heart rhythm and respiration. However, our result contradicts the hypothesis that the coupling between the respiratory and cardiovascular systems is unidirectional, i.e., the respiratory rhythm affects the heart rate through stimulation of the vagus nerve (Guyton, 1991) and direct mechanical action on the sinus node (Bernardi et al., 1990; Faes and Nollo, 2010), while the influence in the opposite direction is absent. However, the influence of the cardiovascular system on the respiratory system was reported in newborns (Rosenblum et al., 2002) and in subjects with apnea (Schreiber, 2000; Bhattacharya et al., 2003; Verdes, 2005).
In our study, we found that the direction of coupling from respiration to the process of parasympathetic control of the heart rate is dominant in all age groups of subjects. Moreover, the values of the directional coupling indices in different age groups take close values. It should be noted that a decrease in cardiorespiratory phase synchronization has been found in elderly subjects (Bliwise, 1993; Shiogai et al., 2010; Bartsch et al., 2012) and a decrease in coherence between the respiration and parasympathetic control of the heart rate with aging has been reported (Ponomarenko et al., 2021). Our results indicate that the mentioned effects of decrease in coherence and synchronization of the cardiac and respiratory systems during aging occur for reasons unrelated to the values of indices of directional coupling between the respiration and parasympathetic control of the heart rate.
To the best of our knowledge, there are no special studies which indicate the presence of time delays in couplings between the respiration and HF oscillations in RR-intervals. However, there is a number of indirect evidence of the possible presence of such delays. In particular, in experiments with direct stimulation of the sympathetic and parasympathetic nerves innervating the heart, there was a delay of tens and hundreds of milliseconds in the response of the cardiovascular system to such stimulation (Warner and Russell, 1969; Somsen et al., 1985; Fagius et al., 1987; Salata and Zipes, 1991; Berntson et al., 1993).
We used the surrogate data analysis to test the hypothesis that the indices of directional coupling calculated from experimental data are significantly different from zero. Surrogate data were generated by random choice of pairs of signals from different subjects, which were not coupled by default, but had similar characteristics. Note that for each state and each direction of coupling, its own 95%-threshold was formed, above which the indices of directional coupling were considered significant.
Using the Mann-Whitney U-test, we tested the null-hypothesis about the equality of
Conclusion
We have revealed the presence of bidirectional coupling between the respiration and the process of parasympathetic control of the heart rate during wakefulness and different stages of sleep in healthy subjects. It is found that in all age groups of subjects, the direction of coupling from respiration to the process of parasympathetic control of the heart rate is dominant. The asymmetry in coupling between the considered processes is most pronounced during deep sleep. This supports the fact that deep sleep is most important for physical restoration with energy saving behavior of physiological systems.
The obtained results provide useful additional information about the features of the cardiorespiratory interaction associated with the modulation of regulatory processes by the higher nervous activity. Furthermore, the considered indices of directional coupling can be useful in sleep studies as an additional tool for classifying sleep stages without registration of electroencephalograms.
Data availability statement
The data analyzed in this study is subject to the following licenses/restrictions: these are data which belong to medical faculties and are not publicly available. Requests to access these datasets should be directed to TP, dGhvbWFzLnBlbnplbEBjaGFyaXRlLmRl.
Ethics statement
The studies involving human participants were reviewed and approved by the Ethics Committee of Klinikum der Philipps-Universität Marburg, Germany. The patients/participants provided their written informed consent to participate in this study.
Author contributions
All authors listed have made a substantial, direct, and intellectual contribution to the work and approved it for publication.
Funding
This work was supported by the Russian Ministry of Health as part of the scientific work No. 122013100209-5, performed at the National Medical Research Center for Therapy and Preventive Medicine in 2022–2024 (development of algorithms and physiological interpretation of results), the Project of RF Government, Grant No. 075-15-2022-1094 (sleep studies), and the Grant MK-2325.2021.1.2 (analysis of directional couplings).
Conflict of interest
The authors declare that the research was conducted in the absence of any commercial or financial relationships that could be construed as a potential conflict of interest.
The handling editor RB declared a past co-authorship with the author TP.
Publisher’s note
All claims expressed in this article are solely those of the authors and do not necessarily represent those of their affiliated organizations, or those of the publisher, the editors and the reviewers. Any product that may be evaluated in this article, or claim that may be made by its manufacturer, is not guaranteed or endorsed by the publisher.
References
Akselrod, S., Gordon, D., Ubel, F., Shannon, D., Berger, A., and Cohen, R. J. (1981). Power spectrum analysis of heart rate fluctuation: A quantitative probe of beat-to-beat cardiovascular control. Science 213 (4504), 220–222. doi:10.1126/science.6166045
Ancona, N., Marinazzo, D., and Stramaglia, S. (2004). Radial basis function approach to nonlinear Granger causality of time series. Phys. Rev. E Stat. Nonlin. Soft Matter Phys. 70, 056221. doi:10.1103/PhysRevE.70.056221
Angelone, A., and Coulter, N. A. (1964). Respiratory sinus arrhythmia: A frequency dependent phenomenon. J. Appl. Physiol. 19, 479–482. doi:10.1152/jappl.1964.19.3.479
Anrep, G. V., Pascual, W., and Rössler, R. (1936). Respiratory variations of the heart rate. I.-the reflex mechanism of the respiratory arrhythmia. Proc. R. Soc. B Biol. Sci. 119, 191–217.
Astolfi, L., Cincotti, F., Mattia, D., Marciani, M. G., Baccala, L. A., De Vico, F. F., et al. (2006). Assessing cortical functional connectivity by partial directed coherence: Simulations and application to real data. IEEE Trans. Biomed. Eng. 53 (9), 1802–1812. doi:10.1109/TBME.2006.873692
Baccala, L. A., Sameshima, K., Ballester, G., Valle, A. C., and Timo-Iaria, C. (1998). Studying the interaction between brain structures via directed coherence and Granger causality. Appl. Sig. Process. 5 (1), 40–48. doi:10.1007/s005290050005
Baccala, L. A., and Sameshima, K. (2001). Partial directed coherence: A new concept in neural structure determination. Biol. Cybern. 84 (6), 463–474. doi:10.1007/PL00007990
Baccala, L. A., Sameshima, K., and Takahashi, D. Y. (2007). “Generalized partial directed coherence”. 15th International Conference on Digital Signal Processing, (Cardiff, UK, July 1-4) 2007 163–166. doi:10.1109/ICDSP.2007.4288544
Bahraminasab, A., Ghasemi, F., Stefanovska, A., McClintock, P., and Kantz, H. (2008). Direction of coupling from phases of interacting oscillators: A permutation information approach. Phys. Rev. Lett. 100 (8), 084101. doi:10.1103/PhysRevLett.100.084101
Bartsch, R. P., Kantelhardt, J., Penzel, T., and Havlin, S. (2007). Experimental evidence for phase synchronization transitions in the human cardiorespiratory system. Phys. Rev. Lett. 98 (5), 054102. doi:10.1103/PhysRevLett.98.054102
Bartsch, R. P., Schumann, A. Y., Kantelhardt, J. W., Penzel, T., and Ivanov, P. Ch. (2012). Phase transitions in physiologic coupling. Proc. Natl. Acad. Sci. U. S. A. 109 (26), 10181–10186. doi:10.1073/pnas.1204568109
Bernardi, L., Salvucci, F., Suardi, R., Solda, P. L., Calciati, A., Perlini, S., et al. (1990). Evidence for an intrinsic mechanism regulating heart-rate variability in the transplanted and the intact heart during submaximal dynamic exercise. Cardiovasc. Res. 24 (12), 969–981. doi:10.1093/cvr/24.12.969
Berntson, G. G., Cacioppo, J. T., and Quigley, K. S. (1993). Respiratory sinus arrhythmia: Autonomic origins, physiological mechanisms, and psychophysiological implications. Psychophysiology 30, 183–196. doi:10.1111/j.1469-8986.1993.tb01731.x
Bhattacharya, J., Pereda, E., and Petsche, H. (2003). Effective detection of coupling in short and noisy bivariate data. IEEE Trans. Syst. Man. Cybern. B Cybern. 33 (1), 85–95. doi:10.1109/tsmcb.2003.808175
Bliwise, D. L. (1993). Sleep in normal aging and dementia. Sleep 16 (1), 40–81. doi:10.1093/sleep/16.1.40
Bunde, A., Havlin, S., Kantelhardt, J. W., Penzel, T., Peter, J. H., and Voigt, K. (2000). Correlated and uncorrelated regions in heart-rate fluctuations during sleep. Phys. Rev. Lett. 85 (17), 3736–3739. doi:10.1103/PhysRevLett.85.3736
Censi, F., Calcagnini, G., and Cerutti, S. (2002). Coupling patterns between spontaneous rhythms and respiration in cardiovascular variability signals. Comput. Methods Programs Biomed. 68 (1), 37–47. doi:10.1016/S0169-2607(01)00158-4
Dougherty, C. M., and Burr, R. L. (1992). Comparison of heart rate variability in survivors and nonsurvivors of sudden cardiac arrest. Am. J. Cardiol. 70 (4), 441–448. doi:10.1016/0002-9149(92)91187-9
Faes, L., and Nollo, G. (2010). Extended causal modeling to assess Partial Directed Coherence in multiple time series with significant instantaneous interactions. Biol. Cybern. 103 (5), 387–400. doi:10.1007/s00422-010-0406-6
Faes, L., Nollo, G., and Porta, A. (2011). Non-uniform multivariate embedding to assess the information transfer in cardiovascular and cardiorespiratory variability series. Comput. Biol. Med. 42, 290–297. doi:10.1016/j.compbiomed.2011.02.007
Faes, L., Porta, A., Cucino, R., Cerutti, S., Antolini, R., and Nollo, G. (2004). Causal transfer function analysis to describe closed loop interactions between cardiovascular and cardiorespiratory variability signals. Biol. Cybern. 90 (6), 390–399. doi:10.1007/s00422-004-0488-0
Faes, L., Porta, A., and Nollo, G. (2015). Information decomposition in bivariate systems: Theory and application to cardiorespiratory dynamics. Entropy 17 (1), 277–303. doi:10.3390/e17010277
Faes, L., Widesott, L., Del Greco, M., Antolini, R., and Nollo, G. (2006). Causal cross-spectral analysis of heart rate and blood pressure variability for describing the impairment of the cardiovascular control in neurally mediated syncope. IEEE Trans. Biomed. Eng. 53 (1), 65–73. doi:10.1109/TBME.2005.859788
Fagius, J., Sundlof, G., and Wallin, B. G. (1987). Variation of sympathetic reflex latency in man. J. Auton. Nerv. Syst. 21, 157–165. doi:10.1016/0165-1838(87)90018-x
Gabor, D. J. (1946). Theory of communication. Part 1: The analysis of information. IEE Lond. 93 (26), 429–441. doi:10.1049/ji-3-2.1946.0074
Galletly, D., and Larsen, P. (1999). Ventilatory frequency variability in spontaneously breathing anaesthetized subjects. Br. J. Anaesth. 83, 552–563. doi:10.1093/bja/83.4.552
Guyenet, P. G. (2014). Regulation of breathing and autonomic outflows by chemoreceptors. Compr. Physiol. 4 (4), 1511–1562. doi:10.1002/cphy.c140004
Hohnloser, S. H., Klingenheben, T., Van de Loo, A., Hablawetz, E., Just, H., Schwartz, P. J., et al. (1994). Reflex versus tonic vagal activity as a prognostic parameter in patients with sustained ventricular tachycardia or ventricular fibrillation. Circulation 89 (3), 1068–1073. doi:10.1161/01.cir.89.3.1068
Hoyer, D., Leder, U., Hoyer, H., Pompe, B., Sommer, M., and Zwiener, U. (2002). Mutual information and phase dependencies: Measures of reduced nonlinear cardiorespiratory interactions after myocardial infarction. Med. Eng. Phys. 24 (1), 33–43. doi:10.1016/S1350-4533(01)00120-5
Iatsenko, D., Bernjak, A., Stankovski, T., Shiogai, Y., Owen-Lynch, P. J., Clarkson, P. B. M., et al. (2013). Evolution of cardiorespiratory interactions with age. Philos. Trans. A Math. Phys. Eng. Sci. 371 (1997), 20110622. doi:10.1098/rsta.2011.0622
Ishbulatov, Y. M., Karavaev, A. S., Kiselev, A. R., Simonyan, M. A., Prokhorov, M. D., Ponomarenko, V. I., et al. (2020). Mathematical modeling of the cardiovascular autonomic control in healthy subjects during a passive head-up tilt test. Sci. Rep. 10 (1), 16525. doi:10.1038/s41598-020-71532-7
Ivanov, P. C., Nunes Amaral, L. A., Goldberger, A. L., and Stanley, H. E. (1998). Stochastic feedback and the regulation of biological rhythms. Europhys. Lett. 43 (4), 363–368. doi:10.1209/epl/i1998-00366-3
Kaiser, A., and Schreiber, T. (2002). Information transfer in continuous processes. Phys. D. Nonlinear Phenom. 166 (1-2), 43–62. doi:10.1016/S0167-2789(02)00432-3
Kaminski, M., Ding, M., Truccolo, W. A., and Bressler, S. L. (2001). Evaluating causal relations in neural systems: Granger causality, directed transfer function and statistical assessment of significance. Biol. Cybern. 85 (2), 145–157. doi:10.1007/s004220000235
Kantelhardt, J. W., Ashkenazy, Y., Ivanov, P. Ch., Bunde, A., Havlin, S., Penzel, T., et al. (2002). Characterization of sleep stages by correlations in the magnitude and sign of heartbeat increments. Phys. Rev. E Stat. Nonlin. Soft Matter Phys. 65 (5), 051908. doi:10.1103/PhysRevE.65.051908
Kantelhardt, J. W., PenzelRostigBecker, T. S. H. F., Havlin, S., and Bunde, A. (2003). Breathing during REM and non-REM sleep: Correlated versus uncorrelated behaviour. Phys. A Stat. Mech. its Appl. 319 (C), 447–457. doi:10.1016/S0378-4371(02)01502-9
Karavaev, A. S., Ishbulatov, Y. M., Prokhorov, M. D., Ponomarenko, V. I., Kiselev, A. R., Runnova, A. E., et al. (2021). Simulating dynamics of circulation in the awake state and different stages of sleep using non-autonomous mathematical model with time delay. Front. Physiol. 11, 612787. doi:10.3389/fphys.2020.612787
Karavaev, A. S., Ishbulatov, Yu. M., Ponomarenko, V. I., Bezruchko, B. P., Kiselev, A. R., and Prokhorov, M. D. (2019). Autonomic control is a source of dynamical chaos in the cardiovascular system. Chaos 29 (12), 121101. doi:10.1063/1.5134833
Karavaev, A. S., Kiselev, A. R., Gridnev, V. I., Borovkova, E. I., Prokhorov, M. D., Posnenkova, O. M., et al. (2013). Phase and frequency locking of 0.1 Hz oscillations in heart rhythm and baroreflex control of arterial pressure by respiration with linearly varying frequency in healthy subjects. Fiziol. Cheloveka 39 (4), 93–104. doi:10.7868/S0131164613010049
Karavaev, A. S., Prokhorov, M. D., Ponomarenko, V. I., Kiselev, A. R., Gridnev, V. I., Ruban, E. I., et al. (2009). Synchronization of low-frequency oscillations in the human cardiovascular system. Chaos 19, 033112. doi:10.1063/1.3187794
Keener, J., and Sneyd, J. (2009). Mathematical Physiology II: Systems Physiology. New York: Springer.
Klösch, G., Kemp, B., Penzel, T., Schlögl, A., Rappelsberger, P., Trenker, E., et al. (2001). The SIESTA project polygraphic and clinical database. IEEE Eng. Med. Biol. Mag. 20 (3), 51–57. doi:10.1109/51.932725
Lewis, M. J., Short, A. L., and Lewis, K. E. (2006). Autonomic nervous system control of the cardiovascular and respiratory systems in asthma. Respir. Med. 100, 1688–1705. doi:10.1016/j.rmed.2006.01.019
Lombardi, F. (2000). Chaos theory, heart rate variability, and arrhythmic mortality. Circulation 101 (1), 8–10. doi:10.1161/01.cir.101.1.8
Lown, B., and Verrier, R. L. (1976). Neural activity and ventricular fibrillation. N. Engl. J. Med. 294 (21), 1165–1170. doi:10.1056/NEJM197605202942107
Malberg, H., Wessel, N., Hasart, A., Osterziel, K. J., and Voss, A. (2002). Advanced analysis of spontaneous baroreflex sensitivity, blood pressure and heart rate variability in patients with dilated cardiomyopathy. Clin. Sci. (Lond). 102 (4), 465–473. doi:10.1042/cs20010106
Mann, H. B., and Whitney, D. R. (1947). On a test of whether one of two random variables is stochastically larger than the other. Ann. Math. Stat. 18 (1), 50–60. doi:10.1214/aoms/1177730491
Milde, T., Schwab, K., Walther, M., Eiselt, M., Schelenz, C., Voss, A., et al. (2011). Time-variant partial directed coherence in analysis of the cardiovascular system. A methodological study. Physiol. Meas. 32 (11), 1787–1805. doi:10.1088/0967-3334/32/11/S06
Mrowka, R., Cimponeriu, L., Patzak, A., and Rosenblum, M. G. (2003). Directionality of coupling of physiological subsystems: Age-related changes of cardiorespiratory interaction during different sleep stages in babies. Am. J. Physiol. Regul. Integr. Comp. Physiol. 285 (6), R1395–R1401. doi:10.1152/ajpregu.00373.2003
Mrowka, R., Patzak, A., and Rosenblum, M. (2000). Quantitative analysis of cardiorespiratory synchronization in infants. Int. J. Bifurc. Chaos 10 (11), 2479–2488. doi:10.1142/S0218127400001754
Müller, A., Riedl, M., Penzel, T., Kurths, J., and Wessel, N. (2014). Ereignisbasierte Charakterisierung kardiovaskulärer Interaktionen während des Schlafs. Somnologie 18 (4), 243–251. doi:10.1007/s11818-014-0688-3
Musizza, B., Stefanovska, A., McClintock, P. V. E., Palus, M., Petrovcic, J., Ribaric, S., et al. (2007). Interactions between cardiac, respiratory and EEG-delta oscillations in rats during anaesthesia. J. Physiol. 580 (1), 315–326. doi:10.1113/jphysiol.2006.126748
Nollo, G., Faes, L., Porta, A., Antolini, R., and Ravelli, F. (2005). Exploring directionality in spontaneous heart period and systolic pressure variability interactions in humans: Implications in the evaluation of baroreflex gain. Am. J. Physiol. Heart Circ. Physiol. 288 (4), H1777–H1785. doi:10.1152/ajpheart.00594.2004
Panter, P. F. (1965). Modulation, noise and spectral analysis, applied to information transmission. New York: McGraw-Hill.
Pikovsky, A., Rosenblum, M., and Kurths, J. (2001). Synchronization: A universal concept in nonlinear sciences. Cambridge: Cambridge University Press.
Pikovsky, A. S., Rosenblum, M. G., Osipov, G. V., and Kurths, J. (1997). Phase synchronization of chaotic oscillators by external driving. Phys. D. Nonlinear Phenom. 104 (3-4), 219–238. doi:10.1016/S0167-2789(96)00301-6
Ponomarenko, V. I., Karavaev, A. S., Borovkova, E. I., Hramkov, A. N., Kiselev, A. R., Prokhorov, M. D., et al. (2021). Decrease of coherence between the respiration and parasympathetic control of the heart rate with aging. Chaos 31 (7), 073105. doi:10.1063/5.0056624
Porges, S. W. (1995). Cardiac vagal tone: A physiological index of stress. Neurosci. Biobehav. Rev. 19 (2), 225–233. doi:10.1016/0149-7634(94)00066-a
Porta, A., Bassani, T., Bari, V., Pinna, G. D., Maestri, R., and Guzzetti, S. (2012). Accounting for respiration is necessary to reliably infer Granger causality from cardiovascular variability series. IEEE Trans. Biomed. Eng. 59 (3), 832–841. doi:10.1109/TBME.2011.2180379
Porta, A., Furlan, R., Rimoldi, O., Pagani, M., Malliani, A., and Van de Borne, P. (2002). Quantifying the strength of the linear causal coupling in closed loop interacting cardiovascular variability signals. Biol. Cybern. 86 (3), 241–251. doi:10.1007/s00422-001-0292-z
Prokhorov, M. D., Bespyatov, A. B., Bodrov, M. B., and Gridnev, V. I. (2005a). Deriving main rhythms of the human cardiovascular system from the heartbeat time series and detecting their synchronization. Chaos, Solit. Fractals 23 (4), 1429–1438. doi:10.1016/s0960-0779(04)00399-6
Prokhorov, M. D., Karavaev, A. S., Ishbulatov, Y. M., Ponomarenko, V. I., Kiselev, A. R., and Kurths, J. (2021). Interbeat interval variability versus frequency modulation of heart rate. Phys. Rev. E 103 (4), 042404. doi:10.1103/PhysRevE.103.042404
Prokhorov, M. D., Ponomarenko, V. I., Bodrov, M. B., Bespyatov, A. B., and Gridnev, V. I. (2005b). Rhythm synchronization in human cardiovascular system from the time sequence of R-R intervals. Biophysics 50 (5), 795–799.
Prokhorov, M. D., Ponomarenko, V. I., Gridnev, V. I ., Bodrov, M. B., and Bespyatov, A. B. (2003). Synchronization between main rhythmic processes in the human cardiovascular system. Phys. Rev. E Stat. Nonlin. Soft Matter Phys. 68 (1), 041913. doi:10.1103/PhysRevE.68.041913
Quian Quiroga, R., Kraskov, A., Kreuz, T., and Grassberger, P. (2002). Performance of different synchronization measures in real data: A case study on electroencephalographic signals. Phys. Rev. E Stat. Nonlin. Soft Matter Phys. 65, 041903. doi:10.1103/PhysRevE.65.041903
Rechtschaffen, A., and Kales, A. (1968). A manual of standardized terminology, techniques and scoring system of sleep stages in human subjects. Los Angeles: UCLA Brain Information Service/Brain Research Institute.
Riedl, M., Müller, A., Kraemer, J. F., Penzel, T., Kurths, J., and Wessel, N. (2014). Cardio-respiratory coordination increases during sleep apnea. PLoS ONE 9 (4), e93866. doi:10.1371/journal.pone.0093866
Rosenblum, M. G., Cimponeriu, L., Bezerianos, A., Patzak, A., and Mrowka, R. (2002). Identification of coupling direction: Application to cardiorespiratory interaction. Phys. Rev. E Stat. Nonlin. Soft Matter Phys. 65 (4), 041909. doi:10.1103/PhysRevE.65.041909
Rosenblum, M. G., Kurths, J., Pikovsky, A., Schafer, C., Tass, P., and Abel, H.-H. (1998). Synchronization in noisy systems and cardiorespiratory interaction. IEEE Eng. Med. Biol. Mag. 17 (6), 46–53. doi:10.1109/51.731320
Rosenblum, M. G., and Pikovsky, A. S. (2001). Detecting direction of coupling in interacting oscillators. Phys. Rev. E Stat. Nonlin. Soft Matter Phys. 64 (2), 045202. doi:10.1103/PhysRevE.64.045202
Rosenblum, M. G., Pikovsky, A. S., Kurths, J., Schafer, C., and Tass, P. A. (2001). Phase synchronization: From theory to data analysis. Handb. Biol. Phys. 4, 279–321.
Salata, J. J., and Zipes, D. P. (1991). “Autonomic nervous system control of heart rate and atrioventricular nodal conduction,” in The reflex control of circulation. Editors I. H. Zucker, and J. P. Gilmore (Boca Raton, FL: CRC Press), 69–101.
Sayers, B. M. (1973). Analysis of heart rate variability. Ergonomics 16 (1), 17–32. doi:10.1080/00140137308924479
Schäfer, C., Rosenblum, M. G., Abel, H.-H., and Kurths, J. (1999). Synchronization in the human cardiorespiratory system. Phys. Rev. E Stat. Phys. Plasmas Fluids Relat. Interdiscip. Top. 60 (1), 857–870. doi:10.1103/physreve.60.857
Schäfer, C., Rosenblum, M. G., Kurths, J., and Abel, H. H. (1998). Heartbeat synchronized with ventilation. Nature 392, 239–240. doi:10.1038/32567
Schelter, B., Winterhalder, M., Dahlhaus, R., Kurths, J., and Timmer, J. (2006a). Partial phase synchronization for multivariate synchronizing systems. Phys. Rev. Lett. 96 (20), 208103. doi:10.1103/PhysRevLett.96.208103
Schelter, B., Winterhalder, M., Eichler, M., Peifer, M., Hellwig, B., Guschlbauer, B., et al. (2006b). Testing for directed influences among neural signals using partial directed coherence. J. Neurosci. Methods 152 (1-2), 210–219. doi:10.1016/j.jneumeth.2005.09.001
Schmitt, D. T., Stein, P. K., and Ivanov, P. Ch. (2009). Stratification pattern of static and scale-invariant dynamic measures of heartbeat fluctuations across sleep stages in young and elderly. IEEE Trans. Biomed. Eng. 56 (5), 1564–1573. doi:10.1109/TBME.2009.2014819
Schreiber, T. (2000). Measuring information transfer. Phys. Rev. Lett. 85 (2), 461–464. doi:10.1103/PhysRevLett.85.461
Schreiber, T., and Schmitz, A. (2000). Surrogate time series. Phys. D. Nonlinear Phenom. 142 (3-4), 346–382. doi:10.1016/S0167-2789(00)00043-9
Schulz, S., Adochiei, F.-C., Edu, I.-R., Schroeder, R., Costin, H., Bar, K.-J., et al. (2013). Cardiovascular and cardiorespiratory coupling analyses: A review. Philos. Trans. A Math. Phys. Eng. Sci. 371, 20120191. doi:10.1098/rsta.2012.0191
Schumann, A. Y., Bartsch, R. P., Penzel, T., Ivanov, P. C., and Kantelhardt, J. W. (2010). Aging effects on cardiac and respiratory dynamics in healthy subjects across sleep stages. Sleep 33 (7), 943–955. doi:10.1093/sleep/33.7.943
Shiogai, Y., Stefanovska, A., and McClintock, P. V. E. (2010). Nonlinear dynamics of cardiovascular ageing. Phys. Rep. 488 (2-3), 51–110. doi:10.1016/j.physrep.2009.12.003
Sidak, E. V., Smirnov, D. A., and Bezruchko, B. P. (2017). Estimation of the time delay of coupling between oscillators from time realizations of oscillation phases for different properties of phase dynamics. J. Commun. Technol. Electron. 62 (3), 241–250. doi:10.1134/S1064226917030196
Smirnov, D. A., and Bezruchko, B. P. (2003). Estimation of interaction strength and direction from short and noisy time series. Phys. Rev. E Stat. Nonlin. Soft Matter Phys. 68 (4), 046209. doi:10.1103/PhysRevE.68.046209
Smirnov, D. A., Sidak, E. V., and Bezruchko, B. P. (2011). Interval estimates of coupling delay using time series of oscillators. Tech. Phys. Lett. 37 (1), 30–33. doi:10.1134/S1063785011010147
Somsen, J. M., Jennings, J. R., and Van der Molen, M. W. (1985). “Cardiac cycle time effects: Information processing and the latencies involved,” in Psychophysiology of cardiovascular control: Models, methods, and data (New York: Plenum Press), 533–548. doi:10.1111/j.1469-8986.2004.00241.x
Song, H. S., and Lehrer, P. M. (2003). The effects of specific respiratory rates on heart rate and heart rate variability. Appl. Psychophysiol. Biofeedback 28 (1), 13–23. doi:10.1023/a:1022312815649
Stankovski, T., Petkoski, S., Raeder, J., Smith, A. F., McClintock, P. V. E., and Stefanovska, A. (2016). Alterations in the coupling functions between cortical and cardio-respiratory oscillations due to anaesthesia with propofol and sevoflurane. Philos. Trans. A Math. Phys. Eng. Sci. 374 (2067), 20150186. doi:10.1098/rsta.2015.0186
Task Force of the European Society of Cardiology and the North American Society of Pacing and Electrophysiology (1996). Heart rate variability: Standards of measurement, physiological interpretation, and clinical use. Circulation 93 (5), 1043–1065. doi:10.1161/01.cir.93.5.1043
Verdes, P. F. (2005). Assessing causality from multivariate time series. Phys. Rev. E Stat. Nonlin. Soft Matter Phys. 72 (2), 026222. doi:10.1103/physreve.72.026222
Warner, H. R., and Russell, R. O. (1969). Effect of combined sympathetic and vagal stimulation on heart rate in the dog. Circ. Res. 24, 567–573. doi:10.1161/01.res.24.4.567
Keywords: cardiovascular system, respiration, parasympathetic control of the heart rate, sleep studies, directional couplings
Citation: Borovkova EI, Prokhorov MD, Kiselev AR, Hramkov AN, Mironov SA, Agaltsov MV, Ponomarenko VI, Karavaev AS, Drapkina OM and Penzel T (2022) Directional couplings between the respiration and parasympathetic control of the heart rate during sleep and wakefulness in healthy subjects at different ages. Front. Netw. Physiol. 2:942700. doi: 10.3389/fnetp.2022.942700
Received: 12 May 2022; Accepted: 15 August 2022;
Published: 06 September 2022.
Edited by:
Ronny P. Bartsch, Bar-Ilan University, IsraelReviewed by:
Tomislav Stankovski, Saints Cyril and Methodius University of Skopje, North MacedoniaPhilip Thomas Clemson, University of Liverpool, United Kingdom
Copyright © 2022 Borovkova, Prokhorov, Kiselev, Hramkov, Mironov, Agaltsov, Ponomarenko, Karavaev, Drapkina and Penzel. This is an open-access article distributed under the terms of the Creative Commons Attribution License (CC BY). The use, distribution or reproduction in other forums is permitted, provided the original author(s) and the copyright owner(s) are credited and that the original publication in this journal is cited, in accordance with accepted academic practice. No use, distribution or reproduction is permitted which does not comply with these terms.
*Correspondence: Mikhail D. Prokhorov, bWRwcm9raG9yb3ZAeWFuZGV4LnJ1