- 1Network Physiology Laboratory, Division of Medicine, University College London, London, United Kingdom
- 2Institute for Liver and Digestive Health, Division of Medicine, University College London, London, United Kingdom
- 3Department of Medicine, University of Padova, Padova, Italy
Background: Liver cirrhosis involves multiple organ systems and has a high mortality. A network approach to complex diseases often reveals the collective system behaviours and intrinsic interactions between organ systems. However, mapping the functional connectivity for each individual patient has been challenging due to the lack of suitable analytical methods for assessment of physiological networks. In the present study we applied a parenclitic approach to assess the physiological network of each individual patient from routine clinical/laboratory data available. We aimed to assess the value of the parenclitic networks to predict survival in patients with cirrhosis.
Methods: Parenclitic approach creates a network from the perspective of an individual subject in a population. In this study such an approach was used to measure the deviation of each individual patient from the existing network of physiological interactions in a reference population of patients with cirrhosis. 106 patients with cirrhosis were retrospectively enrolled and followed up for 12 months. Network construction and analysis were performed using data from seven clinical/laboratory variables (serum albumin, bilirubin, creatinine, ammonia, sodium, prothrombin time and hepatic encephalopathy) for calculation of parenclitic deviations. Cox regression was used for survival analysis.
Result: Initial network analysis indicated that correlation between five clinical/laboratory variables can distinguish between survivors and non-survivors in this cohort. Parenclitic deviations along albumin-bilirubin (Hazard ratio = 1.063, p < 0.05) and albumin-prothrombin time (Hazard ratio = 1.138, p < 0.05) predicted 12-month survival independent of model for end-stage liver disease (MELD). Combination of MELD with the parenclitic measures could predict survival better than MELD alone.
Conclusion: The parenclitic network approach can predict survival of patients with cirrhosis and provides pathophysiologic insight on network disruption in chronic liver disease.
Introduction
The liver is the physiological hub for multiple homeostatic, metabolic, synthetic and immune functions. Thus, patients with liver failure exhibit various neural, renal, cardiovascular, endocrine, and metabolic manifestations. Cirrhosis is a complex disease caused by alcohol, chronic viral hepatitis, fatty liver or other causes (Asrani et al., 2019) and involves multiple organ-systems and functions. Thus, interpretation of organ dysfunction without consideration of the whole system is illogical. This is evident in the relative difficulty in the management of complications of cirrhosis whereby targeting a single organ dysfunction may lead to the dysregulation of other tightly balanced pathways (Harrison, 2018). This makes prediction of treatment response and prognosis especially challenging and further complicates prioritization of liver transplantation (Dutkowski et al., 2015; Jadlowiec and Taner, 2016). The introduction of several prognostic scores and models such as Child-Pugh, MELD, UKELD amongst others is in direct response to the complexity of decompensated cirrhosis and while these models have been useful, various limitations continue to surface (Biselli et al., 2010).
The future of disease diagnosis, management and prognosis will likely benefit from a network physiology approach providing a more global or holistic view of the changes in the physiological interactome leading to disrupted states. Network physiology focuses on complex interactions among diverse organ systems in health and disease (Bashan et al., 2012) and provides a viable alternative to the conventional scoring methods and facilitate the evaluation of organ systems interaction in complex disorders such as cirrhosis. Early work by Asada et al., on critically ill patients in the intensive care unit showed disrupted network of organ systems interaction in non-survivors. In Asada et al. (2016) study, the degree of organ systems interaction was assessed by calculating the correlation between biomarkers (e.g., correlation between creatinine and bilirubin as biomarkers for renal and hepatic function respectively). Then a network was mapped using individual biomarkers as its nodes and correlation coefficients as the edges of the network. Their results showed that in a cohort of critically ill patients, survivors consistently exhibited a higher number of edges and clusters compared to non-survivors in their organ connectivity network structures (Asada et al., 2016). In a recent report, we used a similar approach and showed that functional connectivity of organ systems is significantly disrupted in patients with cirrhosis who did not survive during 12-month follow up (Tan et al., 2020). However, the methodology of these studies is based on correlation analysis of a population of patients and cannot be used for mapping the network connectivity at the level of individual patients. Hence, these reports provide insight about the pathophysiology in general but doesn’t allow clinical application to individual subjects (Asada et al., 2016).
The parenclitic network analysis was proposed by Zanin et al. (2014) to create a network from the perspective of an individual subject in a population. Instead of looking at the network of connections in a population, this approach provides a method for mapping a network for each subject, where nodes represent features and links are weighted according to the deviation between a subject’s features and their corresponding typical relationship within a studied population (“Parenclitic” mean “deviation” in Greek) (Zanin et al., 2014). In its simplest form, the model can be a simple linear regression between all possible pairs of features in the population, followed by the calculation of deviations between values of a particular subject and pre-constructed reference models (Figure 1). A network map is then constructed for individual subjects whereby each feature represents a node and deviation from the reference model is defined as edges between the nodes. The topological characteristics of the resulting network of individual subject can be used to extract important information about the relationships of the system. Since its first description, parenclitic network analysis has been used both in genetic mapping of cancer (Zanin, 2016; Karsakov et al., 2017; Whitwell et al., 2018), Down syndrome (Krivonosov, 2020), aging (Whitwell et al., 2020) and even criminology (Zanin, 2018) and continues to open up new insights in complex systems.
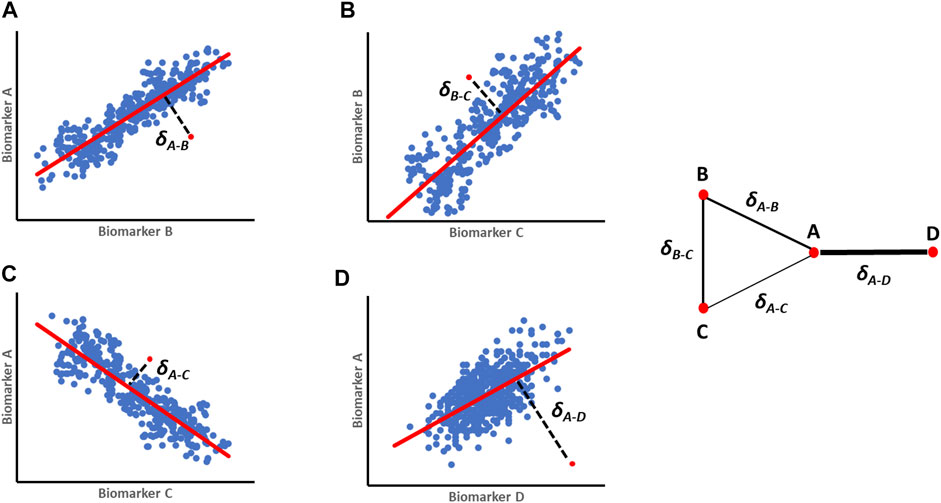
FIGURE 1. A schematic representation of orthogonal residuals (δ) calculation and translation into parenclitic network. (A–D) First regression models are built for pairs of variables (A-B; B-C; A-C and A-D) from a reference population (e.g., survivors, treatment responders etc.). The blue dots represent individual reference data, the red regression lines represent the expected relationship models, while the red dots are individual data of patients being studied. The black lines represent the deviation values (δ). The resulting parenclitic network map of nodes A, B, C and D is presented with edges weighted (in terms of thickness) according to the magnitude of deviations from the models.
In the present study we applied a parenclitic approach using standard clinical/laboratory data to map the physiological network of individual patients with cirrhosis. We then tested whether this approach could predict survival independent of current measures of severity of cirrhosis such as MELD and Child-Pugh scores.
Methods
Ethics
The study protocol was approved by the Padova Hospital Ethics Committee. All participants provided written informed consent. This study was conducted according to the Declaration of Helsinki (Hong Kong Amendment) and Good Clinical Practice (European) guidelines.
Patients Cohorts
The study population consists of 106 patients diagnosed with cirrhosis referred to the tertiary referral liver centre of the Clinica Medica V, Padova University Hospital, for formal hepatic encephalopathy assessment. Patients were enrolled between 2009 and 2018 according to the inclusion/exclusion criteria [for more information about patients’ recruitment see Formentin et al. (2021)].
Inclusion/Exclusion Criteria
Inclusion criteria include confirmed diagnosis of cirrhosis based on clinical manifestations and/or liver imaging. Exclusion criteria includes age under 16 or over 80, hepatocellular carcinoma, severe co-morbidity with short prognosis per se, a history of cirrhosis on a transplanted liver, significant head injury, neurological or psychiatric disease other than hepatic encephalopathy, active alcohol misuse or acute infection.
Follow Up
Patients meeting above inclusion and exclusion criteria were studied retrospectively and further separated into survivor and non-survivor groups by the survival status during the follow-up periods (12 months). Patients who were transplanted due to liver failure were classed as non-survivors as they were in immediate need of a new liver and would not survive without transplantation (Oyelade et al., 2020).
Clinical Laboratory Variables
Seven standard clinical variables representing unique physiological functions or clinical feature were collected (serum albumin, ALB; total bilirubin, Bil; prothrombin time, PT; serum creatinine, Cr; ammonia, NH4; serum sodium, Na; and hepatic encephalopathy, HE) based on a previous study (Tan et al., 2020). HE was classified as unimpaired, minimal and overt HE according to Montagnese et al. (Montagnese et al., 2004; Vilstrup et al., 2014).
Network Generation in the Population
The patients were grouped into two classes based on their survival status after 12 months of follow-up periods and a network map based on simple linear regression was constructed for both classes for visualisation (Tan et al., 2020). Nodes within network graphs represent clinical variables and the correlation between pairs of nodes are represented as edges. Spearman’s correlation was computed with pair matching to correct for missing data and the level of significance was based on a Bonferroni-corrected p-value (Dunn, 1961). An edge was formed between a pair of variables if the correlation between them is significant (p ≤ 0.0024: i.e., Bonferroni-corrected p-value). Pairs of clinical variables that did not meet the threshold for significant correlation in the correlation coefficient analysis were excluded from further analysis. The correlation network maps for survivors and non-survivors after a 12-month follow-up time are presented in Figures 2A,B.
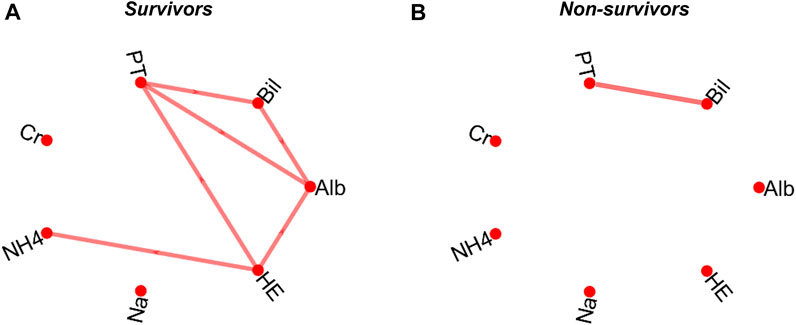
FIGURE 2. Correlation network map of survivors (A) and non-survivors (B) following a 12-month follow-up period. The map is based on a pairwise Spearman’s correlation’s correlation based on a Bonferroni-corrected significant level (p = 0.0024). serum albumin, Alb; total bilirubin, Bil; prothrombin time, PT; serum creatinine, Cr; ammonia, NH4; serum sodium, Na; and hepatic encephalopathy, HE.
Parenclitic Network
Deviation Value Computation (δ)
The data of patients that survived after a 12-month follow-up period were used as reference in each follow-up time to construct a regression model based on the significantly correlated pairs of clinical variables above. As shown in Figure 2A, there is a significant correlation between 6 of the 21 computed pair of correlations in the survivor group. There is only one significant correlation between biomarkers in the non-survivor group (Figure 2B). The deviations between the data of all individual patients (survived and non-survived) from the pre-constructed reference model were then calculated as orthogonal residuals of the regression lines of each pair of correlated variables (Figures 1A–D).
Network Topology Analysis
Network topology analysis describes the underlying dynamics of a connected system. The network topology analysis of physiological functions have been shown to provide information on the adaptability and dynamic flexibility of organ systems to changes in the environmental conditions (Bashan et al., 2012). Several network topology metrics weighed by parenclitic deviations were used to assess the changes in physiological connectedness in patients with cirrhosis. These include network in-degree centrality, shortest path length, global diameter, and efficiency. Supplementary Table S1 presents the definitions and mathematical formulae of these indices.
Software Development
The software for computing the parenclitic network outputs was written in-house using MATLAB build R2021a (MATLAB, 2021) according to the originally described technique (Zanin et al., 2014). In summary, the software extracts and uses the data of the survivors to compute a Bonferroni-corrected regression model for all pairs of physiological variables (e.g., Na-Alb, Alb-Bil). The parameters (slope and intercepts) of significantly correlated pairs were used to compute the vertical and horizontal residuals (y and x respectively) which were then used to find the orthogonal residuals (delta, ∂) for all patients (survivors and non-survivors) as follows:
The computed ∂’s were used as the weight of the connections between all correlated pairs of variables for all patients. Further, the individual ∂-weighted parenclitic network graphs are then used to perform the global network topology analyses. All computed results were combined into an output table which is labelled with the combined names of the variable pairs for the ∂’s and the computed network topology indices. The table is then written into a named, dated output saved in the workspace as a single excel file for further statistical analysis. The software is available in the GitHub repositor; https://github.com/topeoyelade.
Statistical Analysis
Statistical analysis was performed using both MATLAB build R2021a (MATLAB, 2021) and SPSS Statistics 26 (IBM Corp., Armonk, New York) (Corp I.B.M., 2019). Initially, a Receiver Operating Curve (ROC) analysis was performed and the Area Under the Curve (AUC) was used to generate cut-off values that combines optimum sensitivity and selectivity in differentiation between the survivors and non-survivors for all computed output variables. Mann-Whitney U-test was used to compare the means of all output variables (∂’s and computed network topology indices) between the survivors and non-survivors. We performed Kaplan-Meier and log-rank (Mantel-Cox) test to assess whether the cut-offs from the ROC analysis can distinguish the groups. Further, bivariate Cox regression was computed to assess whether the significantly different variables with survival prediction can predict mortality independent of MELD and Child-Pugh scores. The combined prognostic index (e.g., MELD-∂) was calculated using the regression coefficients according to the following equation:
Results
Study Population
Overall, 106 patients diagnosed with cirrhosis were followed up for 12 months. During the follow up periods 17 deaths were recorded; 14 patients underwent transplantation due to liver failure or associated complications and were recorded as dead as they were considered to need a new liver to survive. The demography and clinical characteristics of the studied population is described in Table 1. Baseline biomarkers as well as MELD and Child-Pugh scores are presented in Supplementary Table S2 which shows a significant difference in most baseline biomarkers and MELD/Child-Pugh scores between survivors and non-survivors.
Parenclitic Deviation (∂’s) of Survivors and Non-Survivors
Parenclitic deviation were compared between survivors and non-survivors and the results are shown in Tables 2. Based on Mann-Whitney U-test, there was increased parenclitic deviations in Alb-Bil (p < 0.001) and Alb-PT (p = 0.004) and Alb-HE (p = 0.034) axes compared with the non-survivors (Table 2).
Parenclitic Deviations in Predicting Survival
Univariate Cox regression showed significant link between higher risk of mortality and parenclitic deviations along the Alb-Bil, Bil-PT, and the Ammonia-HE axes (Table 3). Higher deviation in the Alb-PT axis resulted in 20% increased risk of 12-month mortality (95% CI, 6%–35%, p < 0.001). Finally, deviation in the Alb-HE axis was linked with 3-fold increased risk of mortality after 12-month follow-up period (95% CI, 5% - 7-fold, p = 0.004: Table 3). A complete set of hazard ratios for all parenclitic deviations are shown in Supplementary Table S4.
Independence of Parenclitic Deviations in Predicting Survival
To assess whether the ability of the parenclitic deviations to significantly predict survival is independent of the index of liver disease severity (MELD), we performed bivariate Cox regressions for parenclitic deviations with MELD as covariate. The parenclitic deviation along the Alb-Bil (Hazard Ratio, 95% CI = 1.063, 1.000–1.129; p = 0.048) and Alb-PT (Hazard Ratio, 95% CI = 1.138, 1.012–1.280; p = 0.031) axes predicted 12-month survival independent of MELD (Table 4). To study this further, we looked at the independence of parenclitic deviations from Child-Pugh score, a classic measure for severity of hepatic dysfunction. Our results showed that parenclitic deviation of the Alb-Bil, Alb-PT and Bil-PT predicted 12-month survival independent of Child-Pugh scores (Supplementary Table S3).
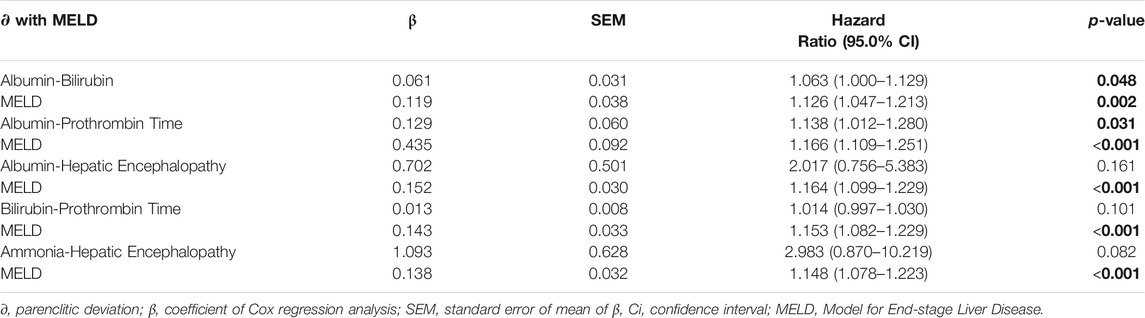
TABLE 4. – The prognosis effects of parenclitic deviations independent of MELD using bivariate Cox regression analysis.
Receiver Operating Characteristics Curves of Parenclitic Deviations
ROC curves were computed for the parenclitic deviations for 12-month follow-up periods that predicted survival independent of MELD (Table 5). The deviation along the Alb-Bil axis showed similar AUC in comparison with MELD (0.762 versus 0.792). As shown in Figure 3 and Table 5, addition of parenclitic deviation of Alb-Bil and Alb-PT axes could increase the AUC for MELD from 0.792 to 0.835 and 0.824 respectively (p < 0.001).
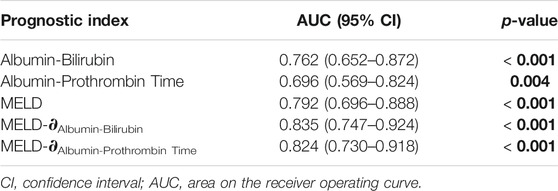
TABLE 5. Area under the ROC curves (AUC) of parenclitic deviations (∂), MELD and combined MELD-∂ during 12-month follow-up periods.
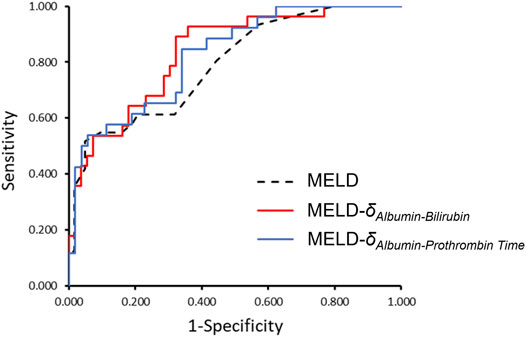
FIGURE 3. The ROC curves comparing MELD alone with MELD-δAlb-Bil and MELD- δAlb-PT in classifying patients as survivor or non-survivor. Addition of parenclitic deviation of Alb-Bil and Alb-PT axes could increase the AUC for MELD from 0.792 (95% CI, 0.696–0.888) to 0.835 (0.747–0.924) and 0.824 (0.730–0.918) respectively (p < 0.001 for all curves).
Kaplan-Meier Graphs of Parenclitic Deviations
For the parenclitic deviations that were significantly predictive of survival independent of MELD, cut-offs with the optimum sensitivity and specificity were generated from their ROC curves (i.e., optimum sensitivity and specificity for prediction of survival). The deduced cut-offs were then used to group the patients into group “predicted non-survivor” if the patients’ parenclitic deviations are higher than or equal to the corresponding cut-off values or “predicted survivor” if otherwise. The binary output was then used to generate Kaplan-Meier graphs to assess the prognostic value. Figure 4 indicates that both Alb-Bil and Alb-PT deviations can predict 12 months survival with a statistically significant log-ranked test (Chi-square 19.03 and 7.81 respectively). Furthermore, addition of Alb-Bil or Alb-PT deviations to MELD in a bivariate Cox regression model enhances the prognostic value of MELD alone (Figure 5).
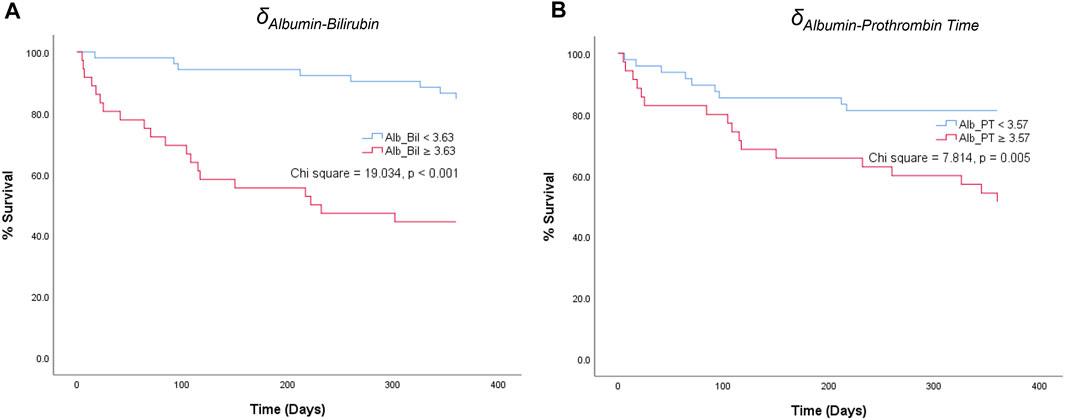
FIGURE 4. Kaplan-Meier graphs showing 12-month survival predictions of parenclitic deviations along the (A) Albumin-Bilirubin (Alb_Bil) and (B) Albumin-Prothrombin Time (Alb_PT) axes based on the cut-off values of 3.63 and 3.57 respectively [Log-rank (Mantel-Cox) test, Chi square = 19.034, p < 0.001 and 7.814, p = 0.005 respectively].
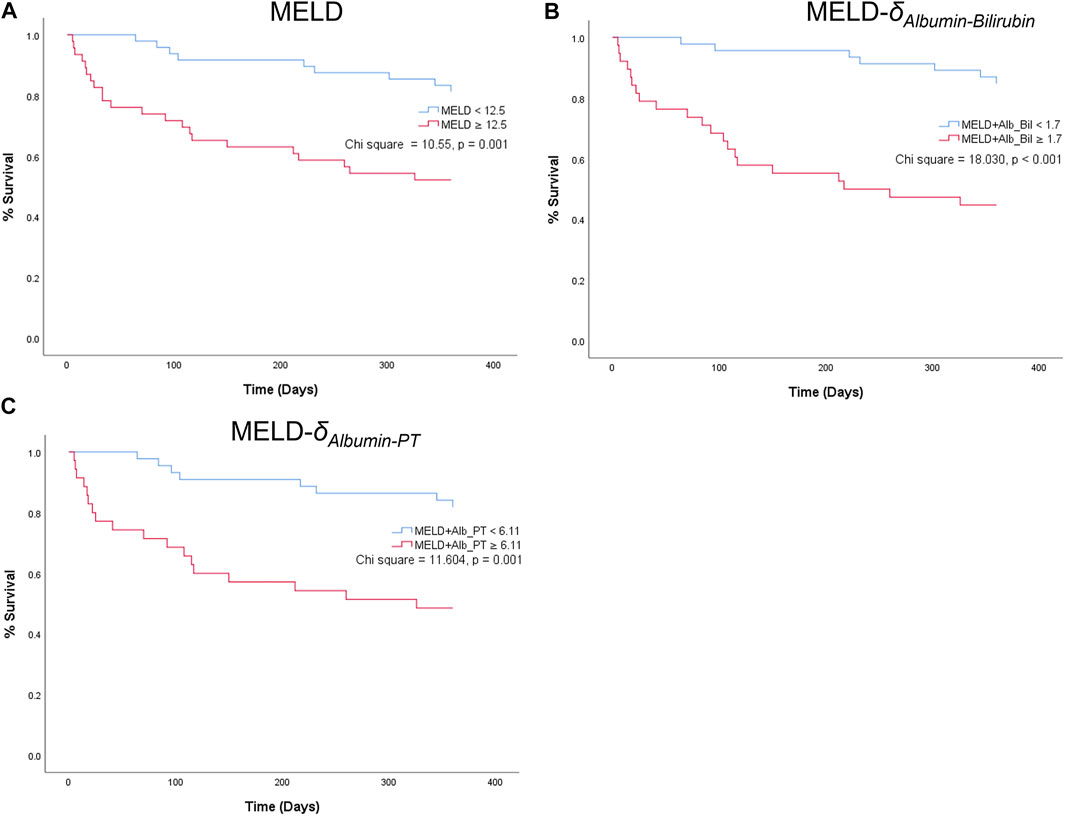
FIGURE 5. Kaplan-Meier graphs showing 12-month survival predictions of MELD (A) and two combined indices: MELD-δAlbumin-Bilirubin (MELD-Alb_Bil) (B) and MELD--δAlbumin-PT (MELD-Alb_PT) (C).
Network Topology Indices and Prediction of Survival in Patients With Cirrhosis
A shown in Supplementary Table S4, there was significant increase in standard deviation of centrality between the survivors and non-survivor group (p = 0.038). Other topology indices did not exhibit statistically significant difference. Cox regression analysis was performed to determine the relationship between network topology indices and survival. Higher standard deviation of centrality increased the risk of mortality with a hazard ratio of 1.054 (95% CI, 1.026–1.083, p < 0.001). Furthermore, the standard deviation of centrality was able to predict survival independent of Child-Pugh score (Supplementary Table S5).
Discussion
In this study, a parenclitic approach was used to map the physiological network of patients with cirrhosis from routine clinical/laboratory data. By using the data of survivors to construct a reference model, deviations for each patient’s pairs of variables from the reference model were calculated and used for prognosis calculation. We found that increased parenclitic deviations and reduced connectedness in the Ammonia-HE axis is associated with ∼4-fold increase in the risk of mortality. Reduced connectedness along the Alb-Bil, Alb-PT and Bil-PT axes were also linked with increased risk of mortality independent of routine prognostic indices such as MELD and Child-Pugh. Higher parenclitic deviations shown by non-survivors suggests a digression from the expected connection along various physiological axes and can be interpreted as significant network disruption between organ systems (i.e., more parenclitic deviation = less organ systems connectivity).
Furthermore, we analysed the network topology indices characterising the parenclitic networks defined by weighted deviations. This gives quantitative measure of the network and evaluates the deviations and their collective relationships. From a set of topological indices, standard deviation of centrality was significantly higher in the non-survivors than survivors and showed significant association with 12-month survival. This global index was also observed to predict survival independent of Child-Pugh. Put together, these results show that parenclitic network analysis can detect certain functional dynamics not picked up by the current models used for prognostication in cirrhosis. These results highlight the significance of interrelationships between clinical variables such as Alb-Bil, Alb-PT and Ammonia-HE in reflecting the pathological stage of cirrhosis and provides insight into complex interactions between extrahepatic complications manifested in multiple organ systems and how they may exacerbate the prognosis of patients with cirrhosis.
A network approach to complex disease such as liver failure has potential to transform the landscape of assessing prognosis. The present study indicates that a parenclitic approach with routine laboratory tests (e.g., albumin, bilirubin, PT) may increase the accuracy of current prognostic factors and be used in conjunction with MELD to ultimately increase the number of lives saved. This is in line with previous research revealing other physio-markers such as EEG or heart rate variability (HRV) in conjunction with MELD to increase the accuracy of prognostication (Montagnese et al., 2015; Chan et al., 2017; Bhogal et al., 2019; Oyelade, 2021). However, while analysis of EEG or HRV requires suitable recording equipment and analytical expertise, the parenclitic approach introduced in this study uses routine laboratory tests that is available in all clinical settings. This is an advantage for this approach and can be extended in future multi-centre prospective clinical investigations. Such a network approach also has potential to be used in other complex illnesses such as sepsis and multiple organ failure for survival modelling as well as providing novel insight about the pathophysiology. If organ systems network disruption plays an important role in critically ill patients (Asada et al., 2016), novel therapies may target enhanced levels of connectivity of organ systems rather than treating functional systems in isolation using pharmacological antagonists.
Our results indicate that parenclitic deviation from albumin-bilirubin, albumin-PT and ammonia-HE axes provide useful information for prognostication. Hepatic encephalopathy is a spectrum of neurophysiological disturbances that occurs in the background of acute or chronic liver failure (Aldridge et al., 2015). Although classically linked with hyperammonaemia, systemic inflammation is known to precipitate or cause exacerbation of HE (Shawcross et al., 2011; Tranah et al., 2013). While the exact link between systemic inflammation, ammonia and HE remain unclear, systemic inflammation (due to endotoxemia, or bacterial translocation) may increase the susceptibility of the brain to hyperammonaemia thereby derailing the correlation between increased serum ammonia and HE. While there was a positive correlation between ammonia and HE in survivors (r = 0.469, p = 0.002), the severity of HE was not significantly associated with ammonia in non-survivors (r = −0.027, p = 0.911). This show that factor(s) other than ammonia may be contributing to HE in non-survivors. Indeed, various studies have linked systemic inflammation with increased severity and poorer prognosis of HE (Rolando, 2000; Vaquero et al., 2003; Shawcross et al., 2004; Shawcross et al., 2007; Sharifi, 2008). Thus, the increased parenclitic deviation along the Ammonia-HE axis may reflect the contribution of a secondary physiological factor which predisposes an increased mortality from cirrhosis. This can be easily analysed using a parenclitic approach as described here or more traditional statistical methods such as analysis of covariance.
Our analysis showed that the correlation between albumin and bilirubin is lost in non-survivors. There was a sharp reduction in serum albumin with increased bilirubin in survivors compared to non-survivors (Supplementary Figure S1). The reason for this disruption is not well clear. However, we hypothesis that; 1) The relatively high albumin observed even at significantly elevated bilirubin level in non-survival may be due to clinical infusion which may not improve the effective systemic albumin or prognosis (Solà et al., 2018; Fernández et al., 2020) but may be associated with increased serious adverse events as was recently reported in the ATTIRE study (China et al., 2021); 2) The half-life of albumin is comparatively higher at about 3 weeks (Peters, 1995) compared to bilirubin which remains in circulation for about 6 min (Reed et al., 1988). In addition, the half-life of albumin might be altered in critically ill patients due impaired microcirculation compared with healthier patients (Vincent et al., 2014) a factor that may contribute to difference in albumin-bilirubin correlation or survivors and non-survivors.
Albumin-PT was another axis that differentiated survivors from non-survivors in our study. The liver produces most procoagulant and anticoagulant proteins, responsible for maintaining haemostasis. In cirrhotic patients, the production of clotting factors and their inhibitors decreases, results in either a “rebalanced” haemostatic equilibrium or a prothrombotic state due to systemic inflammation (Baccouche et al., 2017). Increased bleeding risk has traditionally been regarded as the most significant haemostatic complication in patients with liver dysfunction, especially in the context of elevated international normalized ratio (INR) (Flores et al., 2017). However, the predictive value of INR in indicating the risk of haemorrhagic event has been contradicted in literature and remains unclear (Lisman and Leebeek, 2007; Lisman and Porte, 2010). On the contrary, there is an increasing recognition of hypercoagulability in some patients with cirrhosis where the risk of thrombotic events (e.g., portal vein thrombosis) might be higher than haemorrhage (Tripodi et al., 2011; Tripodi et al., 2017; Talon et al., 2020). Portal vein thromboses and clotting of extracorporeal circuits are common in cirrhosis despite elevated INR values, while elevated bleeding tendency has been suggested to be associated with sepsis, hepatorenal syndrome, hypotension, and endothelial dysfunction instead of isolated liver dysfunction (Harrison, 2018). Indeed, venous thromboembolism (VTE) is an underdiagnosed and serious medical condition that occurs at a relative risk of >2% in cirrhotic patients and associated with greater mortality in higher Child-Pugh stages (Buresi et al., 2012; Yang et al., 2012). Also, low serum albumin has been found to be strongly predictive of increased risk of VTE, independent of INR or platelet account (Northup et al., 2006). It is hypothesized that lower serum albumin concentration is a surrogate for decreased protein synthesis by the liver and therefore correlated with decreased production of endogenous anti-coagulant factors such as Protein C and S. Our results share similar findings (Supplementary Figure S2), that the albumin levels are generally lower in non-survivors and remain low despite increase in PT. While in survivors, albumin levels present positive linear increase with PT. In cirrhosis, coagulopathy involves a complicated network of haemostatic factors, with the risks of thrombotic and haemorrhagic events reported to be independent of current markers or scores (Harrison, 2018). Therefore, a parenclitic approach to relationship between albumin and PT might pave the way for assessment of this relationship in routine clinical practice.
This study validates the feasibility of parenclitic network-based approach for predicting the survival status of patients with liver cirrhosis, and its independence from Child-Pugh and MELD scores, indicating that including the correlation between biomarkers improves current prognostic indices and may help improve the accuracy of prognostication. This suggests that a parenclitic approach has potential in complementing current prognostic scoring systems for liver cirrhosis. However, there are some limitations. Firstly, the data of survivors was used as reference for measurement of deviations is limited in size and from a single referral medical centre. Future studies need to look at a more diverse multicentre cohort of patients with cirrhosis. Full applicability of the parenclitic method in survival modelling in cirrhosis requires further validation by applying the reference parameters developed in the current study to an external dataset of patients with cirrhosis. Alternatively, constructing parenclitic networks in bootstrap replica of the data may provide further information on reliability of this approach. Further studies can investigate validation of such a network approach in a larger and more clinically diverse patient population. Another limitation of this study is that the relationship models of different clinical variables were based on linear regression, which assumes a correlative linear relationship between all pairs of variables. More sophisticated methods such as the 2-dimensional kernel density estimation (Whitwell et al., 2018) could potentially serve as a better approach, as it provides compatibility of categorical and continuous data. Further, various variables such as inflammatory biomarkers (e.g., IL-6) and physiological markers (e.g., heart rate variability, heart rate turbulence and temperature variability indices), that were shown to predict mortality in cirrhosis patients (Mani et al., 2009; Bottaro et al., 2020) could be included in the analysis to widen the scope and improve the prognostic value of the parenclitic method. In addition, the results of this study might not be extendable to all subgroup of patients with cirrhosis as data were selected from patients referred to a tertiary referral clinic for evaluation of HE. For example, the parenclitic network may exhibit a different pattern in patients with acute-on-chronic liver failure (ACLF) compared with other forms of decompensation. This may give insight about the mechanism of decompensation and organ failure in cirrhosis. Future studies can focus on more diverse, and clinically relevant subgroups of patients with cirrhosis to provide a more comprehensive picture of organ systems network disruption in individual patients with cirrhosis. The present study also lacks a time-dependent approach in predicting outcome using the parenclitic networks. Assessment of network structure over time can provide useful information on the trajectory of alterations in physiological processes involved in decompensation and might be of significant value for prognosis evaluation.
In conclusion, this is the first study to use the parenclitic network analysis of routine clinical data to assess organ system disruption and predict survival in individual patients with cirrhosis. Potential application of this method includes the prediction of treatments response or patients likely to develop serious adverse events due to certain treatments. For example, patients with decompensated cirrhosis indicated for vasoconstrictors and/or albumin treatment who may not respond (Cavallin et al., 2015; Wang et al., 2018; Moore et al., 2020) or those likely to develop respiratory failure (Wong et al., 2021) or other side effects (Martín–Llahí, 2008; Neri et al., 2008; Sanyal et al., 2008; Gluud et al., 2010). The holistic approach of the parenclitic network analysis may prove to be a better prognostic method and can provide novel pathophysiologic insight for understanding complex diseases such as chronic liver failure.
Data Availability Statement
The raw data supporting the conclusion of this article will be made available by the authors, without undue reservation.
Ethics Statement
The studies involving human participants were reviewed and approved by the Padova Hospital Ethics Committee. The patients/participants provided their written informed consent to participate in this study.
Author Contributions
HZ, TO, and AM contributed to the conceptualization. SM, AM, and TO contributed to the data curation. HZ, TO, and AM contributed to the formal analysis, software, and the writing of the original draft. KM and AM contributed to the supervision. HZ, TO, KM, SM, and AM contributed to the writing—review and editing. All authors contributed to the article and approved the submitted version.
Conflict of Interest
The authors declare that the research was conducted in the absence of any commercial or financial relationships that could be construed as a potential conflict of interest.
Publisher’s Note
All claims expressed in this article are solely those of the authors and do not necessarily represent those of their affiliated organizations, or those of the publisher, the editors and the reviewers. Any product that may be evaluated in this article, or claim that may be made by its manufacturer, is not guaranteed or endorsed by the publisher.
Supplementary Material
The Supplementary Material for this article can be found online at: https://www.frontiersin.org/articles/10.3389/fnetp.2022.833119/full#supplementary-material
References
Aldridge, D. R., Tranah, E. J., and Shawcross, D. L. (2015). Pathogenesis of Hepatic Encephalopathy: Role of Ammonia and Systemic Inflammation. J. Clin. Exp. Hepatol. 5 (Suppl. 1), S7–s20. doi:10.1016/j.jceh.2014.06.004
Asada, T., Aoki, Y., Sugiyama, T., Yamamoto, M., Ishii, T., Kitsuta, Y., et al. (2016). Organ System Network Disruption in Nonsurvivors of Critically Ill Patients. Crit. Care Med. 44 (1), 83–90. doi:10.1097/CCM.0000000000001354
Asrani, S. K., Devarbhavi, H., Eaton, J., and Kamath, P. S. (2019). Burden of Liver Diseases in the World. J. Hepatol. 70 (1), 151–171. doi:10.1016/j.jhep.2018.09.014
Baccouche, H., Labidi, A., Fekih, M., Mahjoub, S., Kaabi, H., Hmida, S., et al. (2017). Haemostatic Balance in Cirrhosis. Blood Coagul. Fibrinolysis 28 (2), 139–144. doi:10.1097/mbc.0000000000000561
Bashan, A., Bartsch, R. P., Kantelhardt, J. W., Havlin, S., and Ivanov, P. C. (2012). Network Physiology Reveals Relations between Network Topology and Physiological Function. Nat. Commun. 3 (1), 702. doi:10.1038/ncomms1705
Bhogal, A. S., De Rui, M., Pavanello, D., El-Azizi, I., Rowshan, S., Amodio, P., et al. (2019). Which Heart Rate Variability index Is an Independent Predictor of Mortality in Cirrhosis? Dig. Liver Dis. 51 (5), 695–702. doi:10.1016/j.dld.2018.09.011
Biselli, M., Gitto, S., Gramenzi, A., Di Donato, R., Brodosi, L., Ravaioli, M., et al. (2010). Six Score Systems to Evaluate Candidates with Advanced Cirrhosis for Orthotopic Liver Transplant: Which Is the winner? Liver Transpl. 16 (8), 964–973. doi:10.1002/lt.22093
Bottaro, M., Abid, N. U., El-Azizi, I., Hallett, J., Koranteng, A., Formentin, C., et al. (2020). Skin Temperature Variability Is an Independent Predictor of Survival in Patients with Cirrhosis. Physiol. Rep. 8 (12), e14452. doi:10.14814/phy2.14452
Buresi, M., Hull, R., and Coffin, C. S. (2012). Venous Thromboembolism in Cirrhosis: a Review of the Literature. Can. J. Gastroenterol. 26 (12), 905–908. doi:10.1155/2012/175849
Cavallin, M., Kamath, P. S., Merli, M., Fasolato, S., Toniutto, P., Salerno, F., et al. (2015). Terlipressin Plus Albumin versus Midodrine and Octreotide Plus Albumin in the Treatment of Hepatorenal Syndrome: a Randomized Trial. Hepatology 62 (2), 567–574. doi:10.1002/hep.27709
Chan, K.-C., Yeh, J.-R., and Sun, W.-Z. (2017). The Role of Autonomic Dysfunction in Predicting 1-year Mortality after Liver Transplantation. Liver Int. 37 (8), 1239–1248. doi:10.1111/liv.13364
China, L., Freemantle, N., Forrest, E., Kallis, Y., Ryder, S. D., Wright, G., et al. (2021). A Randomized Trial of Albumin Infusions in Hospitalized Patients with Cirrhosis. N. Engl. J. Med. 384 (9), 808–817. doi:10.1056/nejmoa2022166
Dunn, O. J. (1961). Multiple Comparisons Among Means. J. Am. Stat. Assoc. 56 (293), 52–64. doi:10.1080/01621459.1961.10482090
Dutkowski, P., Linecker, M., DeOliveira, M. L., Müllhaupt, B., and Clavien, P.-A. (2015). Challenges to Liver Transplantation and Strategies to Improve Outcomes. Gastroenterology 148 (2), 307–323. doi:10.1053/j.gastro.2014.08.045
Fernández, J., Angeli, P., Trebicka, J., Merli, M., Gustot, T., Alessandria, C., et al. (2020). Efficacy of Albumin Treatment for Patients with Cirrhosis and Infections Unrelated to Spontaneous Bacterial Peritonitis. Clin. Gastroenterol. Hepatol. 18 (4), 963–973. doi:10.1016/j.cgh.2019.07.055
Flores, B., D Trivedi, H., C Robson, S., and Bonder, A. (2017). Hemostasis, Bleeding and Thrombosis in Liver Disease. J. Transl Sci. 3 (3), 1000182. doi:10.15761/JTS.1000182
Formentin, C., Zarantonello, L., Mangini, C., Frigo, A. C., Montagnese, S., and Merkel, C. (2021). Clinical, Neuropsychological and Neurophysiological Indices and Predictors of Hepatic Encephalopathy (HE). Liver Int. 41 (5), 1070–1082. doi:10.1111/liv.14785
Gluud, L. L., Christensen, K., Christensen, E., and Krag, A. (2010). Systematic Review of Randomized Trials on Vasoconstrictor Drugs for Hepatorenal Syndrome. Hepatology 51 (2), 576–584. doi:10.1002/hep.23286
Harrison, M. F. (2018). The Misunderstood Coagulopathy of Liver Disease: A Review for the Acute Setting. West. J. Emerg. Med. 19 (5), 863–871. doi:10.5811/westjem.2018.7.37893
Jadlowiec, C. C., and Taner, T. (2016). Liver Transplantation: Current Status and Challenges. Wjg 22 (18), 4438–4445. doi:10.3748/wjg.v22.i18.4438
Karsakov, A., Bartlett, T., Ryblov, A., Meyerov, I., Ivanchenko, M., and Zaikin, A. (2017). Parenclitic Network Analysis of Methylation Data for Cancer Identification. PloS one 12 (1), e0169661. doi:10.1371/journal.pone.0169661
Krivonosov, M. (2020). DNA Methylation Changes with Age as a Complex System: A Parenclitic Network Approach to a Family-Based Cohort of Patients with Down Syndrome. bioRxiv, 2020.03.10.986505. doi:10.1101/2020.03.10.986505
Lisman, T., and Leebeek, F. W. G. (2007). Hemostatic Alterations in Liver Disease: A Review on Pathophysiology, Clinical Consequences, and Treatment. Dig. Surg. 24 (4), 250–258. doi:10.1159/000103655
Lisman, T., and Porte, R. J. (2010). Rebalanced Hemostasis in Patients with Liver Disease: Evidence and Clinical Consequences. Blood 116 (6), 878–885. doi:10.1182/blood-2010-02-261891
Mani, A. R., Montagnese, S., Jackson, C. D., Jenkins, C. W., Head, I. M., Stephens, R. C., et al. (2009). Decreased Heart Rate Variability in Patients with Cirrhosis Relates to the Presence and Degree of Hepatic Encephalopathy. Am. J. Physiology-Gastrointestinal Liver Physiol. 296 (2), G330–G338. doi:10.1152/ajpgi.90488.2008
Martín–Llahí, M. (2008). Terlipressin and Albumin vs Albumin in Patients with Cirrhosis and Hepatorenal Syndrome: a Randomized Study. Gastroenterology 134 (5), 1352–1359.
Montagnese, S., Amodio, P., and Morgan, M. Y. (2004). Methods for Diagnosing Hepatic Encephalopathy in Patients with Cirrhosis: A Multidimensional Approach. Metab. Brain Dis. 19 (3), 281–312. doi:10.1023/b:mebr.0000043977.11113.2a
Montagnese, S., De Rui, M., Schiff, S., Ceranto, E., Valenti, P., Angeli, P., et al. (2015). Prognostic Benefit of the Addition of a Quantitative Index of Hepatic Encephalopathy to the MELD Score: the MELD-EEG. Liver Int. 35 (1), 58–64. doi:10.1111/liv.12490
Moore, K., Jamil, K., Verleger, K., Luo, L., Kebede, N., Heisen, M., et al. (2020). Real-world Treatment Patterns and Outcomes Using Terlipressin in 203 Patients with the Hepatorenal Syndrome. Aliment. Pharmacol. Ther. 52 (2), 351–358. doi:10.1111/apt.15836
Neri, S., Pulvirenti, D., Malaguarnera, M., Cosimo, B. M., Bertino, G., Ignaccolo, L., et al. (2008). Terlipressin and Albumin in Patients with Cirrhosis and Type I Hepatorenal Syndrome. Dig. Dis. Sci. 53 (3), 830–835. doi:10.1007/s10620-007-9919-9
Northup, P. G., McMahon, M. M., Ruhl, A. P., Altschuler, S. E., Volk-Bednarz, A., Caldwell, S. H., et al. (2006). Coagulopathy Does Not Fully Protect Hospitalized Cirrhosis Patients from Peripheral Venous Thromboembolism. Am. J. Gastroenterol. 101 (7), 1524–1528. doi:10.1111/j.1572-0241.2006.00588.x
Oyelade, T., Canciani, G., Bottaro, M., Zaccaria, M., Formentin, C., Moore, K., et al. (2020). Heart Rate Turbulence Predicts Survival Independently from Severity of Liver Dysfunction in Patients with Cirrhosis. Front. Physiol. 11, 602456. doi:10.3389/fphys.2020.602456
Oyelade, T. (2021). Heart Rate Variability in Patients with Cirrhosis: A Systematic Review and Meta-Analysis. Physiol. Meas. 42 (5), 055003. doi:10.1088/1361-6579/abf888
Peters, T. (1995). All about Albumin: Biochemistry, Genetics, and Medical Applications. Academic Press.
Reed, R. G., Davidson, L. K., Burrington, C. M., and Peters, T. (1988). Non-resolving Jaundice: Bilirubin Covalently Attached to Serum Albumin Circulates with the Same Metabolic Half-Life as Albumin. Clin. Chem. 34 (10), 1992–1994. doi:10.1093/clinchem/34.10.1992
Rolando, N. (2000). The Systemic Inflammatory Response Syndrome in Acute Liver Failure. Hepatology 32 (4), 734–739. doi:10.1053/jhep.2000.17687
Sanyal, A. J., Boyer, T., Garcia–Tsao, G., Regenstein, F., Rossaro, L., Appenrodt, B., et al. (2008). A Randomized, Prospective, Double-Blind, Placebo-Controlled Trial of Terlipressin for Type 1 Hepatorenal Syndrome. Gastroenterology 134 (5), 1360–1368. doi:10.1053/j.gastro.2008.02.014
Sharifi, Y. (2008). Defining the Outcomes of Severe Hepatic Encephalopathy in Cirrhosis: Inflammation Is the Key. Liver Int. 28 (5). doi:10.1111/j.1478-3231.2008.01738.x
Shawcross, D. L., Davies, N. A., Williams, R., and Jalan, R. (2004). Systemic Inflammatory Response Exacerbates the Neuropsychological Effects of Induced Hyperammonemia in Cirrhosis. J. Hepatol. 40 (2), 247–254. doi:10.1016/j.jhep.2003.10.016
Shawcross, D. L., Sharifi, Y., Canavan, J. B., Yeoman, A. D., Abeles, R. D., Taylor, N. J., et al. (2011). Infection and Systemic Inflammation, Not Ammonia, Are Associated with Grade 3/4 Hepatic Encephalopathy, but Not Mortality in Cirrhosis. J. Hepatol. 54 (4), 640–649. doi:10.1016/j.jhep.2010.07.045
Shawcross, D. L., Wright, G., Olde Damink, S. W. M., and Jalan, R. (2007). Role of Ammonia and Inflammation in Minimal Hepatic Encephalopathy. Metab. Brain Dis. 22 (1), 125–138. doi:10.1007/s11011-006-9042-1
Solà, E., Solé, C., Simón-Talero, M., Martín-Llahí, M., Castellote, J., Garcia-Martínez, R., et al. (2018). Midodrine and Albumin for Prevention of Complications in Patients with Cirrhosis Awaiting Liver Transplantation. A Randomized Placebo-Controlled Trial. J. Hepatol. 69 (6), 1250–1259. doi:10.1016/j.jhep.2018.08.006
Talon, L., Sinegre, T., Lecompte, T., Pereira, B., Massoulié, S., Abergel, A., et al. (2020). Hypercoagulability (Thrombin Generation) in Patients with Cirrhosis Is Detected with ST‐Genesia. J. Thromb. Haemost. 18 (9), 2177–2190. doi:10.1111/jth.14963
Tan, Y. Y., Montagnese, S., and Mani, A. R. (2020). Organ System Network Disruption Is Associated with Poor Prognosis in Patients with Chronic Liver Failure. Front. Physiol. 11, 983. doi:10.3389/fphys.2020.00983
Tranah, T. H., Vijay, G. K. M., Ryan, J. M., and Shawcross, D. L. (2013). Systemic Inflammation and Ammonia in Hepatic Encephalopathy. Metab. Brain Dis. 28 (1), 1–5. doi:10.1007/s11011-012-9370-2
Tripodi, A., Anstee, Q. M., Sogaard, K. K., Primignani, M., and Valla, D. C. (2011). Hypercoagulability in Cirrhosis: Causes and Consequences1. J. Thromb. Haemost. 9 (9), 1713–1723. doi:10.1111/j.1538-7836.2011.04429.x
Tripodi, A., Primignani, M., Mannucci, P. M., and Caldwell, S. H. (2017). Changing Concepts of Cirrhotic Coagulopathy. Official J. Am. Coll. Gastroenterol. ACG 112 (2), 274–281. doi:10.1038/ajg.2016.498
Vaquero, J., Polson, J., Chung, C., Helenowski, I., Schiodt, F. V., Reisch, J., et al. (2003). Infection and the Progression of Hepatic Encephalopathy in Acute Liver Failure. Gastroenterology 125 (3), 755–764. doi:10.1016/s0016-5085(03)01051-5
Vilstrup, H., Amodio, P., Bajaj, J., Cordoba, J., Ferenci, P., Mullen, K. D., et al. (2014). Hepatic Encephalopathy in Chronic Liver Disease: 2014 Practice Guideline by the American Association for the Study of Liver Diseases and the European Association for the Study of the Liver. Hepatology 60 (2), 715–735. doi:10.1002/hep.27210
Vincent, J.-L., Russell, J. A., Jacob, M., Martin, G., Guidet, B., Wernerman, J., et al. (2014). Albumin Administration in the Acutely Ill: what Is New and where Next? Crit. Care 18 (4), 231. doi:10.1186/cc13991
Wang, H., Liu, A., Bo, W., Feng, X., and Hu, Y. (2018). Terlipressin in the Treatment of Hepatorenal Syndrome. Medicine (Baltimore) 97 (16), e0431. doi:10.1097/md.0000000000010431
Whitwell, H. J., Bacalini, M. G., Blyuss, O., Chen, S., Garagnani, P., Gordleeva, S. Y., et al. (2020). The Human Body as a Super Network: Digital Methods to Analyze the Propagation of Aging. Front. Aging Neurosci. 12, 136. doi:10.3389/fnagi.2020.00136
Whitwell, H. J., Blyuss, O., Menon, U., Timms, J. F., and Zaikin, A. (2018). Parenclitic Networks for Predicting Ovarian Cancer. Oncotarget 9 (32), 22717–22726. doi:10.18632/oncotarget.25216
Wong, F., Pappas, S. C., Curry, M. P., Reddy, K. R., Rubin, R. A., Porayko, M. K., et al. (2021). Terlipressin Plus Albumin for the Treatment of Type 1 Hepatorenal Syndrome. N. Engl. J. Med. 384 (9), 818–828. doi:10.1056/nejmoa2008290
Yang, Z. J., Costa, K. A., Novelli, E. M., and Smith, R. E. (2012). Venous Thromboembolism in Cirrhosis. Clin. Appl. Thromb. Hemost. 20 (2), 169–178. doi:10.1177/1076029612461846
Zanin, M., Alcazar, J. M., Carbajosa, J. V., Paez, M. G., Papo, D., Sousa, P., et al. (2014). Parenclitic Networks: Uncovering New Functions in Biological Data. Sci. Rep. 4 (1), 5112–5116. doi:10.1038/srep05112
Zanin, M. (2018). Credit Card Fraud Detection through Parenclitic Network Analysis. Complexity 2018, 64370. doi:10.1155/2018/5764370
Keywords: cirrhosis, MELD, network physiology, parenclitic, prognosis, survival
Citation: Zhang H, Oyelade T, Moore KP, Montagnese S and Mani AR (2022) Prognosis and Survival Modelling in Cirrhosis Using Parenclitic Networks. Front. Netw. Physiol. 2:833119. doi: 10.3389/fnetp.2022.833119
Received: 10 December 2021; Accepted: 07 February 2022;
Published: 21 February 2022.
Edited by:
Paul Bogdan, University of Southern California, Los Angeles, United StatesReviewed by:
Vasilios E. Papaioannou, Democritus University of Thrace, GreeceSalvatore Micciche’, University of Palermo, Italy
Christian Jansen, University of Bonn, Germany
Toshifumi Asada, Mitsui Memorial Hospital, Japan
Copyright © 2022 Zhang, Oyelade, Moore, Montagnese and Mani. This is an open-access article distributed under the terms of the Creative Commons Attribution License (CC BY). The use, distribution or reproduction in other forums is permitted, provided the original author(s) and the copyright owner(s) are credited and that the original publication in this journal is cited, in accordance with accepted academic practice. No use, distribution or reproduction is permitted which does not comply with these terms.
*Correspondence: Ali R. Mani, a.r.mani@ucl.ac.uk
†These authors have contributed equally to this work