- 1Department of Epileptology, University of Bonn Medical Centre, Bonn, Germany
- 2Helmholtz Institute for Radiation and Nuclear Physics, University of Bonn, Bonn, Germany
- 3Interdisciplinary Center for Complex Systems, University of Bonn, Bonn, Germany
Electroencephalography (EEG) is a widely employed tool for exploring brain dynamics and is used extensively in various domains, ranging from clinical diagnosis via neuroscience, cognitive science, cognitive psychology, psychophysiology, neuromarketing, neurolinguistics, and pharmacology to research on brain computer interfaces. EEG is the only technique that enables the continuous recording of brain dynamics over periods of time that range from a few seconds to hours and days and beyond. When taking long-term recordings, various endogenous and exogenous biological rhythms may impinge on characteristics of EEG signals. While the impact of the circadian rhythm and of ultradian rhythms on spectral characteristics of EEG signals has been investigated for more than half a century, only little is known on how biological rhythms influence characteristics of brain dynamics assessed with modern EEG analysis techniques. At the example of multiday, multichannel non-invasive and invasive EEG recordings, we here discuss the impact of biological rhythms on temporal changes of various characteristics of human brain dynamics: higher-order statistical moments and interaction properties of multichannel EEG signals as well as local and global characteristics of EEG-derived evolving functional brain networks. Our findings emphasize the need to take into account the impact of biological rhythms in order to avoid erroneous statements about brain dynamics and about evolving functional brain networks.
1 Introduction
The human brain is an open, dissipative, and adaptive dynamical system that can be described as a complex network of networks of interacting subsystems. It is an inherently nonstationary system, whose complicated spatial-temporal dynamics is still poorly understood. In order to gain deeper insights, various measurement techniques are employed to record—on different spatial scales and with different levels of invasiveness—time series of observables related to e.g. electric and/or magnetic fields or thermodynamic and chemical properties. Among these measurement techniques, electroencephalography (EEG) is the only technique that allows for the continuous multichannel recording of time series of macro-scale brain dynamics over extended periods of time (days to weeks and beyond (Niedermeyer and Lopes da Silva, 2005; Weisdorf et al., 2019; Viana et al., 2021)). In case of brain pathologies (such as epilepsy), invasive electroencephalography provides additional access to the meso- (≈105 neurons) and the micro-scale (single neurons) (Engel et al., 2005; Chang, 2015). Electroencephalography allows to capture a wide spectrum of physiological and pathophysiological activities on various time scales.
Access to brain dynamics using EEG can be gained through active perturbations or passive observations. In the first case, evoked or event-related potentials (EP/ERP) are assumed to reflect the synchronized neuronal relaxation dynamics of specific brain regions elicited by motor, sensory, or cognitive tasks. On a more global level, cortical excitability can be probed with e.g. electrical or magnetic stimulation (Hallett, 2007; Yang et al., 2021). With these approaches, the recorded relaxation dynamics is typically confined to time scales ranging from a few milliseconds to a few seconds. In the second case, ongoing (i.e., non-triggered) EEG signals are recorded e.g. during sleep, states of wakefulness (daily life activities), or during specific neuropsychological tasks that control sensory inputs and/or higher cognitive functions. Such recordings capture brain dynamics on time scales that range from a few seconds to hours and days and beyond. Both these cases require special time series analysis techniques. Modern EEG analysis techniques allow investigation of various linear and nonlinear aspects of ongoing brain dynamics of single brain regions as well as of properties of interactions (strength, direction, coupling functions) between the dynamics of two or more brain regions. Together with graph-theoretical concepts these analysis techniques provide a means to characterize the dynamical evolution of brain networks (for an overview, see e.g. Niedermeyer and Lopes da Silva (2005), Pereda et al. (2005), Stam (2005), Lehnertz et al. (2009), Lehnertz (2011), Sakkalis (2011), Greenblatt et al. (2012), Lehnertz et al. (2014), Lehnertz et al. (2017).
Like many other physiologic observables, EEG signals are influenced by various endogenous and exogenous biological rhythms (Aschoff, 1981; Rensing et al., 1987). Among these rhythms, the circadian rhythm—a roughly 24-h cycle (range: 20—28 h; see Figure 1)—is probably the best investigated rhythm (Mills, 1966; Halberg, 1969; Folkard et al., 1983; Spengler et al., 2000; Cermakian and Boivin, 2003; Bell-Pedersen et al., 2005; Iskra-Golec, 2006; Czeisler and Gooley, 2007; Franken and Dijk, 2009; Mohawk et al., 2012; Gillette, 2013; Ly et al., 2016; Duboc et al., 2020; Foster, 2020; Garbarino et al., 2020; Hablitz et al., 2020; Lananna and Musiek, 2020; Matenchuk et al., 2020). Ultradian rhythms have shorter periods than the circadian rhythm’s period, and periods are often defined to be shorter than 20 h but longer than 1 h. These rhythms are often not directly related to environment cycles which renders their interpretation difficult. Prominent examples include the 90—120 min cycling of the sleep stages (Dement and Kleitman, 1957) and the basic rest-activity cycle (Klein and Armitage, 1979; Lavie and Kripke, 1981; Kleitman, 1982). Infradian rhythms have longer periods than the circadian rhythm’s period, i.e., longer than 28 h. Prominent examples include the circaseptan rhythm (weekly rhythm, 7 ± 3 days), the circatrigintan rhythm (monthly rhythm, 30 ± 5 days, e.g. menstruation), and the circannual rhythm (yearly rhythm, 1 year ±2 months). Interactions between the various rhythms are not fully understood (Laje et al., 2018).
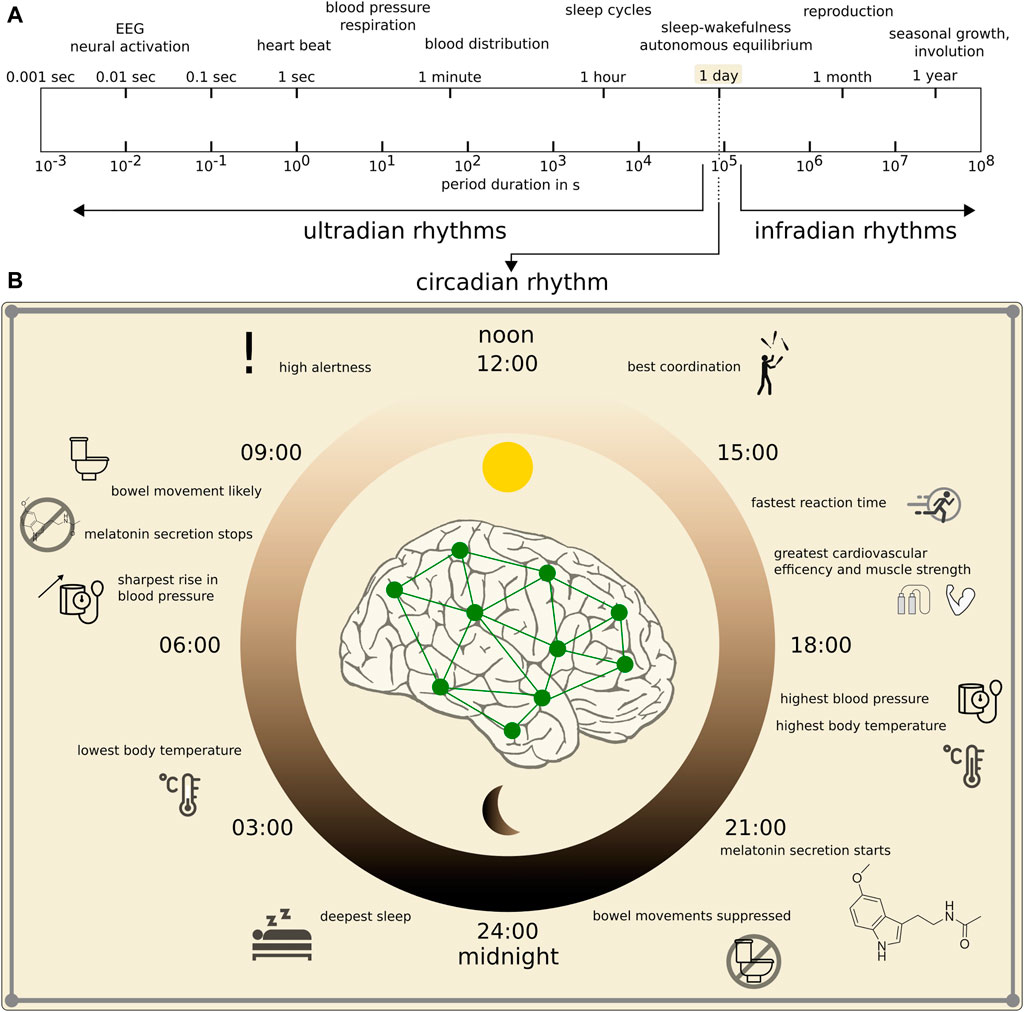
FIGURE 1. Spectrum of main biological rhythms in humans. (A): Logarithmic presentation of period durations of rhythms (modified after Hildebrandt (1991)). (B): Zoom into human circadian rhythm with some behavioral and physiological functions within the 24 h cycle that impact on the dynamics of the brain and other organ systems.
The impact of particularly the circadian rhythm and of ultradian rhythms on EEG signals is known for more than 50 years (for circadian rhythms, see, e.g. Frank et al. (1966); Gundel and Witthöft (1983); Machleidt (1980); Borbély et al. (1981); Torsvall and Åkerstedt (1987); Cacot et al. (1995); Borbély and Achermann (1999); Aeschbach et al. (1999); Dijk and Duffy (1999); for ultradian rhythms, see, e.g. Manseau and Broughton (1984); Oken and Chiappa (1988); Tsuji and Kobayashi (1988); Hayashi et al. (1994); Kaiser (2008); Piarulli et al. (2016)). Many seminal studies, however, were based on EEG recordings that covered time periods ranging from a few seconds to a few hours and/or captured the dynamics of only a few brain regions. Moreover, most studies concentrated on the rhythms’ impact on spectral characteristics of EEG signals, i.e., on changes of spectral power in the well-known alpha-, beta-, theta-, and delta-frequency band. Given recent technological developments that enable ultra-long (days to weeks and beyond) scalp (Casson, 2019), sub-scalp (Weisdorf et al., 2019; Duun-Henriksen et al., 2020), and intracortical (Zaer et al., 2021) EEG recordings in diverse applications (Aricò et al., 2018; Abiri et al., 2019; Cinel et al., 2019; Lohani et al., 2019; Alsuradi et al., 2020; Dehais et al., 2020; Rashid et al., 2020) even beyond clinical ones, it is important to understand the impact of endogenous and exogenous rhythms on characteristics of brain dynamics assessed with the aforementioned modern EEG analysis techniques.
2 From Local to Global: Impact of Biological Rhythms
In the following, we highlight some important aspects of this impact by discussing findings that we obtained from analyses of exemplary continuous multiday, multichannel EEG signals recorded non-invasively and invasively from two subjects. We here concentrate on exemplary characteristics of brain dynamics and of so called functional brain networks (Bullmore and Sporns, 2009) that we estimated from EEG signals with various analysis techniques using a moving-window approach (window length: 20 s; non-overlapping window; demeaned artifact-free data). The chosen window length can be regarded as a compromise between the required statistical accuracy for the calculation of the various characteristics and approximate stationarity of EEG signals within a window’s duration (Isaksson et al., 1981; Blanco et al., 1995; Rieke et al., 2003).
For each window and each sampled brain region, we estimated the respective dynamics’ statistical moments (Press et al., 2007): standard deviation σ, skewness s, and (excess) kurtosis k. For each window and each (non-redundant) pair of sampled brain regions, we characterized their strength of interaction employing a phase-based (mean phase coherence R (Mormann et al., 2000)) and an amplitude-based estimator (absolute value of the linear correlation coefficient ρ (Press et al., 2007)). Both these estimators are often used to derive a functional brain network, whose vertices are associated with the sampled brain regions and whose edges represent the strength of interaction between pairs of vertices (often referred to as functional connectivity; see Bastos and Schoffelen (2016) for an overview). We here proceeded in that way and estimated the network’s clustering coefficient CX as well as eigenvector centrality for vertices
2.1 Impact on Temporal Changes of Dynamics’ Characteristics of Single Brain Regions
We begin with discussing the impact of various rhythms on the temporal changes of statistical moments estimated for the dynamics of 19 brain regions recorded non-invasively with scalp EEG (nEEG) (Niedermeyer and Lopes da Silva, 2005) (Figure 2A). A large fraction of the temporal variability of the nEEG signals’ standard deviation, skewness, and kurtosis can be attributed to the circadian rhythm with a period length at about 24 h. Interestingly, both the rhythm’s intensity and its period length vary for the different recording sites, which would indicate that the dynamics of the different brain regions are differently affected by this rhythm. Similar observations can be made for ultradian rhythms with period lengths at about 12, 8, 6, 4 h, 90, and 60 min but with comparably smaller intensities. Topographically, these rhythms are most pronounced in fronto-central areas, and these areas are known to reflect, for instance, the dynamics of the wake-dependent, or homeostatic component of sleep regulation (Cajochen et al., 2002; Croce et al., 2018). Another important aspect of the rhythms’ impact can be identified from the circadian distribution of nEEG signals’ statistical moments. Within the circadian cycle, the standard deviation attains highest values during the nighttime, as expected (Hjorth, 1970, 1973). Notably, the nEEG signals’ third and fourth statistical moment indicate a clear deviation from Gaussianity for data recorded during the daytime.
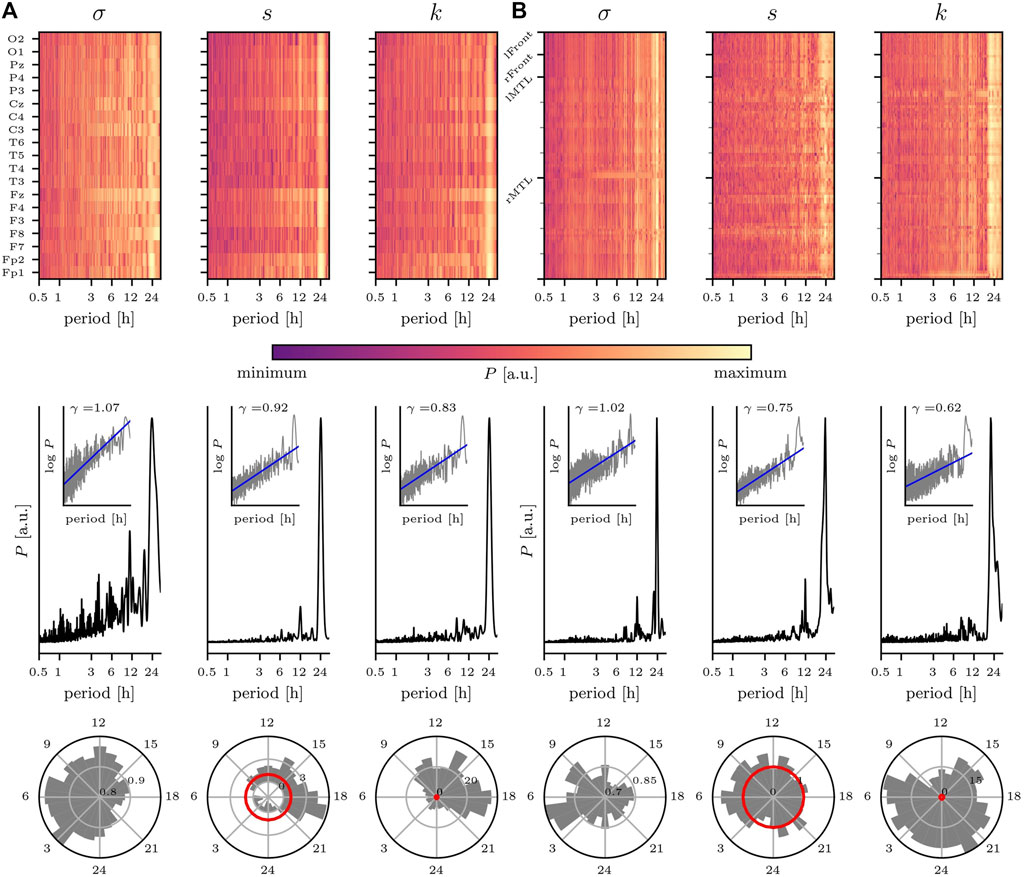
FIGURE 2. Impact of circadian rhythm and of ultradian rhythms on temporal changes of statistical moments of brain dynamics. Exemplary findings for (A) non-invasive EEG (nEEG) recording lasting 7 days (recording sites shown on y-axis; data from a male subject (81 y) with cognitive impairment under CNS drugs admitted for evaluation of epilepsy risk) and (B) intracranial EEG (iEEG) recording lasting 14 days (sampled brain regions (left/right mesial temporal lobe (MTL) and left/right frontal areas) shown on y-axis; data from a male subject (55 y) with epilepsy under CNS drugs admitted for presurgical evaluation). Top: relative power spectral densities (P; color coded) of time series of standard deviation σ, skewness s, and kurtosis k from each recording site. Middle: averaged relative power spectral densities (mean over all sampled brain regions). Insets show log-log plots of data (grey) together with linear least squares lines (blue, log P = γ log π, where π denotes period (range: 30 min to 32 h) and γ denotes the scaling exponent). Bottom: circadian distribution of statistical moments (24 h bins; mean over all sampled brain regions). Note that for Gaussian distributed data, skewness and (excess) kurtosis would be zero with their respective standard deviation indicated by the red circle. For σ, the outermost circle indicates the maximum value and inner circles the relative percentage. For s and k, the grey/black circles indicate the factor by which data deviates from the standard deviation of Gaussian distributed data (red circle). EEG data sampled at 256 Hz (A) 250 Hz (B); 16 bit ADC; bandwidth 1—45 Hz; notch filter at line frequency (50 Hz).
We observe a similarly pronounced impact of the circadian rhythm on statistical moments of intracranially recorded brain dynamics (Figure 2B). Remarkably, for the iEEG signals’ standard deviation σ, the 24 h peak in the corresponding periodogram is rather narrow, and one might speculate that this can be related to the narrow spatial sampling of circumscribed brain regions. Apart from rhythms with period lengths at about 12, 8 and 4 h, other ultradian rhythms contribute only to a small extent to the temporal variability of the iEEG signals’ statistical moments. Within the circadian cycle, the standard deviation again attains highest values during the nighttime, while skewness and kurtosis here indicate a clear deviation from Gaussianity mostly independent of the time of day.
It is important to note that the observed deviations from Gaussianity—seen for both nEEG and iEEG signals and that differentially depend on the time of day—may strongly impact on both the design of EEG-based investigations of various physiological and pathophysiological phenomena and the suitability and reliability of various EEG analysis techniques that assume the data to be Gaussian distributed. In the same manner, the observed 1/f-like temporal fluctuations of the EEG signals’ statistical moments (as indicated by the scaling exponents in the range
2.2 Impact on Temporal Changes of Interactions Between Brain Regions
We proceed with discussing the impact of the various biological rhythms on the temporal changes of interactions between the dynamics of the sampled brain regions (Figure 3). For both, the phase-based and the amplitude-based estimator for the strength of interaction and independent of the type of recording, we observe—on average—a pronounced impact of the circadian rhythm. Note, however, that interactions are not equally affected by this rhythm; its impact may vary for short-ranged (nearest neighbor brain regions), intermediate-ranged (regions within same hemisphere), and long-ranged (across hemispheres) interactions (cf. Kreuz et al. (2004) and Lehnertz et al. (2017)). Moreover, for some of these interactions we observe additional contributions with period lengths around 17–19 h and around 27–30 h, which lead to either a triplett-like structure together with the circadian peak or to a broadening of that peak in the averaged periodogram.
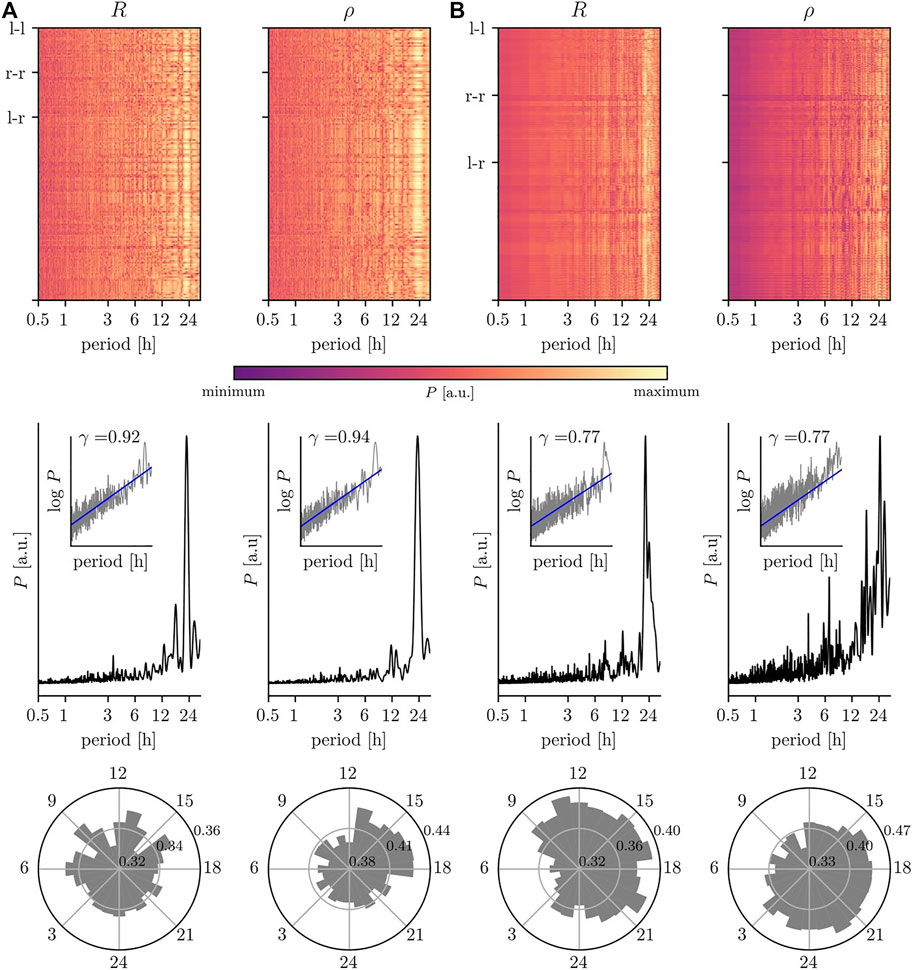
FIGURE 3. Impact of circadian rhythm and of ultradian rhythms on temporal changes of interaction properties of brain dynamics. Same EEG data as in Figure 2: (A) scalp recording; (B) intracranial recording. Top: relative power spectral densities (color coded) of time series of estimates of the strength of interaction between each (non-redundant) pair of sampled brain regions (estimates based on mean phase coherence R and on linear correlation coefficient ρ). Intrahemispheric interactions are labeled l–l/r–r and interhemispheric interactions l–r. Middle: averaged relative power spectral densities (mean over all non-redundant pairs of sampled brain regions). Insets show log-log plots of data (grey) together with linear least squares lines (blue, see Figure 2 for details). Bottom: circadian distribution of estimated interaction properties (24 h bins; mean over all non-redundant pairs of sampled brain regions).
Different ultradian rhythms appear to impact on temporal changes of the strength of interactions estimated from nEEG signals (Figure 3A) and from iEEG signals (Figure 3B) but with comparably smaller intensities. For the former, we observe contributions at period lengths around 3.5 and 12 h, while for the latter period lengths around 5 and 8 h or around 4 and 7 h can be identified depending on the employed estimator for the strength of interactions (R or ρ) together with an additional contribution at 12 h (cf. Porz et al. (2014)). A differential impact of these biological rhythms on the strength of interactions, estimated with either R or with ρ, can also be observed within the circadian cycle. Independent of the type of recording, the strengths of interactions are comparably higher during the daytime, which would point to a decreased level of synchronization between brain regions during the nighttime (Steriade and Hobson, 1976; Horovitz et al., 2009; Lazar et al., 2015; Mizrahi-Kliger et al., 2018; Nguyen et al., 2018). Interestingly, the daytime amplitude-based contributions (estimated with ρ) appear to be delayed by 3–6 h to the phase-based contributions (estimated with R). We can not yet provide an explanation for such a time delay, but it would need to be taken into account in comparative studies of e.g. functional connectivity or (patho-)physiologic synchronization phenomena.
In line with a number of previous studies (see, e.g., Gong et al. (2003); Stam and De Bruin (2004); Botcharova et al. (2014); Racz et al. (2018); Daffertshofer et al. (2018); Stylianou et al. (2020); La Rocca et al. (2021)), we observe 1/f-like temporal fluctuations of characteristics of brain interactions (as indicated by the scaling exponents
2.3 Impact on Temporal Changes of Characteristics of Evolving Functional Brain Networks
Eventually, we discuss the impact of the various biological rhythms on temporal changes of exemplary global (clustering coefficient (CR and Cρ)) and local characteristics (centralities of vertices (
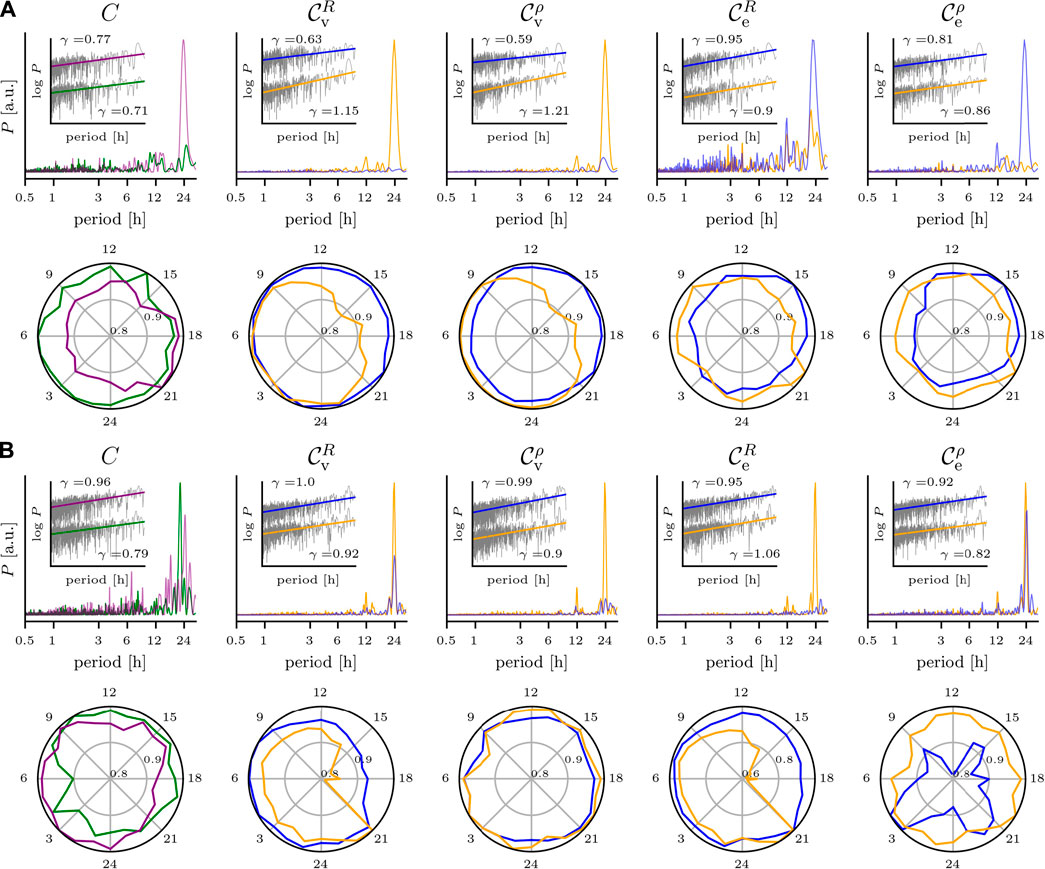
FIGURE 4. Impact of circadian rhythm and of ultradian rhythms on temporal changes of global and local characteristics of evolving brain networks. Same EEG data as in Figure 2. (A) Scalp recording; upper row, from left to right: relative power spectral densities of time series of the networks’ clustering coefficient C (derivation of network edges based on mean phase coherence R (green) or on linear correlation coefficient ρ (purple)), of eigenvector centralities
Clustering Coefficient
The temporal changes of Cρ of nEEG-based evolving networks (Figure 4A) are strongly dominated by the circadian rhythm with only minor contributions from ultradian rhythms with period lengths ranging from 1 h to about 14 h. In contrast, for the same networks but with edges derived via R, the circadian rhythm’s impact on CR compares to the ones seen for the aforementioned ultradian rhythms. Within the circadian cycle, fluctuations of both CR and Cρ amount to a few percent.
For the clustering coefficient of iEEG-based evolving networks (Figure 4B), the dependence of the rhythms’ impact on the estimator used to derive edges is less pronounced. The circadian rhythm appears to be decomposed into quartett-like structures with contributions at period lengths 22, 24, 19, and 27 h (descending order of intensities) for CR and at 25, 28, 21, and 19 h for Cρ. For CR, we observe contributions of ultradian rhythms with period lengths at about 15, 12, 8, and 3 h but with comparably small intensities. For Cρ, there are contributions with higher intensities and with period lengths at about 17.5, 15, and 16 h (triplett-like structure) as well as at 13, 8.5, 6.5, and 3.8 h. As with the nEEG-based evolving networks, fluctuations within the circadian cycle of clustering coefficients amount to a few percent. Comparable findings were reported for the clustering coefficient as well as for other global characteristics of binary evolving brain networks derived from continuous, long-term, multichannel iEEG (Kuhnert et al., 2010; Geier et al., 2015; Lehnertz et al., 2017) and nEEG data (Mitsis et al., 2020) recorded from a larger group of epilepsy patients. Note that the temporal fluctuations of the clustering coefficients of the nEEG-based and iEEG-based evolving brain networks comply with a power-law with a scaling exponent γ in the range
Vertex and Edge Centralities
For the nEEG-based evolving networks (Figure 4A), we concentrate on centrality (
Now, for temporal changes of edge centrality (
For the iEEG-based evolving networks (Figure 4B), we concentrate on centrality (
For temporal changes of edge centrality (
Not taking into account dependencies of network characteristics on biological rhythms can lead to erroneous statements about evolving functional brain networks.
3 Conclusion
We illustrated the impact of various biological rhythms on temporal changes of characteristics of human brain dynamics at the example of multiday, multichannel non-invasive and invasive EEG recordings from two subjects. We here considered statistical moments and interaction properties of multichannel brain dynamics as well as local and global characteristics of EEG-derived evolving functional brain networks.
We observed the circadian rhythm (with a period length around 24 h) to exert the strongest influence on almost all investigated characteristics. Its impact varied locally, and for characteristics of functional brain networks its influence strongly depended on the approach used to derive networks. For some characteristics, we observed triplett- and/or quartett-like structures accompanying the circadian peak in the respective periodograms. This points to a time-dependent period length of this biological rhythm that is captured by some though not all characteristics. We also observed various ultradian rhythms to impact on temporal changes of the investigated characteristics. Period lengths of these rhythms ranged from 1 to 12 h, and their impact varied locally and strongly differed for the investigated characteristics.
So far, only a few studies related time-dependent fluctuations of EEG signal characteristics (other than those related to power spectral density estimates) to biological rhythms. Ngamga et al. (2016) observed various recurrence-quantification-analysis-based complexity measures for continuous multiday, multichannel invasive EEG recordings from five subjects with epilepsy to fluctuate differently within the circadian cycle, and fluctuations differed for the investigated brain regions. Croce et al. (2018) reported various fractal characteristics of non-invasive EEG recordings from 21 healthy volunteers to be modulated by the circadian rhythm. Modulations varied locally and paralleled changes in alertness and performance. Wilkat et al. (2019) reported the circadian rhythm and ultradian rhythms with period length larger than 4 h to strongly impact on lag-1 autocorrelation and the (unbiased sample) variance of continuous multiday, multichannel invasive EEG recordings from 28 subjects with epilepsy. Since these characteristics are often used in studies that aim at identifying generic early warning signals for critical transitions (e.g., precursors of epileptic seizures), omitting their dependence on biological rhythm renders the reliability of these characteristics problematic. Kurth et al. (2021) reported stochastic qualifiers of brain dynamics to be strongly affected by rhythms acting on time scales that range from hours to days. These findings indicate that biological rhythms even impact on the choice of the stochastic model that may better describe brain dynamics depending on time of day. Kreuz et al. (2004), Porz et al. (2014), and Lehnertz et al. (2017) reported various phase-based estimators for the strength of interactions to be differentially influenced by ultradian and circadian rhythms. Likewise, only a few studies made use of continuous multiday, multichannel EEG recordings from larger groups of subjects with epilepsy to demonstrate fluctuations of local and global characteristics of EEG-derived evolving functional brain networks that can be related to various biological rhythms (Kuhnert et al., 2010; Geier et al., 2015; Geier and Lehnertz, 2017; Lehnertz et al., 2017; Rings et al., 2019).
We are not aware of studies that investigated the impact of infradian rhythms (circaseptan, circatrigintan, or circannual rhythms) on temporal changes of characteristics of human brain dynamics. Nevertheless, when considering time scales of years, a number of studies reported on—at times nontrivial—age-dependencies of characteristics of brain dynamics (see, e.g. (Lindsley, 1939; Duffy et al., 1984; Dustman et al., 1985, 1993; Klass and Brenner, 1995; Anokhin et al., 1996; Polich, 1997; Stam, 2005; Fernández et al., 2012; McIntosh et al., 2014; Sleimen-Malkoun et al., 2015; Zappasodi et al., 2015; Ishii et al., 2017; Knyazeva et al., 2018) and more recently of characteristics of EEG-derived functional brain networks (see, e.g. (Vecchio et al., 2017; Nobukawa et al., 2019; Helmstaedter et al., 2021). In addition to the impact of the various biological rhythms, such age-dependencies would need to be taken into account in order to avoid erroneous statements about brain dynamics and about evolving functional brain networks.
Data Availability Statement
The original contributions presented in the study are included in the article/supplementary files, further inquiries can be directed to the corresponding author.
Ethics Statement
The studies involving human participants were reviewed and approved by the Ethics committee of the University of Bonn. The patients/participants provided their written informed consent to participate in this study.
Author Contributions
All authors listed have made a substantial, direct, and intellectual contribution to the work and approved it for publication.
Funding
This work was supported by the Verein zur Foerderung der Epilepsieforschung (e.V.).
Conflict of Interest
The authors declare that the research was conducted in the absence of any commercial or financial relationships that could be construed as a potential conflict of interest.
Publisher’s Note
All claims expressed in this article are solely those of the authors and do not necessarily represent those of their affiliated organizations, or those of the publisher, the editors and the reviewers. Any product that may be evaluated in this article, or claim that may be made by its manufacturer, is not guaranteed or endorsed by the publisher.
Acknowledgments
We thank Randi von Wrede, Theresa Wilkat, Christoph Helmstaedter, and Chittaranjan Hens for fruitful discussions.
References
Abiri, R., Borhani, S., Sellers, E. W., Jiang, Y., and Zhao, X. (2019). A Comprehensive Review of EEG-Based Brain-Computer Interface Paradigms. J. Neural Eng. 16, 011001. doi:10.1088/1741-2552/aaf12e
Aeschbach, D., Matthews, J. R., Postolache, T. T., Jackson, M. A., Giesen, H. A., and Wehr, T. A. (1999). Two Circadian Rhythms in the Human Electroencephalogram during Wakefulness. Am. J. Physiology-Regulatory, Integr. Comp. Physiol. 277, R1771–R1779. doi:10.1152/ajpregu.1999.277.6.r1771
Alsuradi, H., Park, W., and Eid, M. (2020). EEG-based Neurohaptics Research: A Literature Review. IEEE Access 8, 49313–49328. doi:10.1109/access.2020.2979855
Anokhin, A. P., Birbaumer, N., Lutzenberger, W., Nikolaev, A., and Vogel, F. (1996). Age Increases Brain Complexity. Electroencephalography Clin. Neurophysiol. 99, 63–68. doi:10.1016/0921-884x(96)95573-3
Aricò, P., Borghini, G., Di Flumeri, G., Sciaraffa, N., and Babiloni, F. (2018). Passive BCI beyond the Lab: Current Trends and Future Directions. Physiol. Meas. 39, 08TR02. doi:10.1088/1361-6579/aad57e
Bastos, A. M., and Schoffelen, J.-M. (2016). A Tutorial Review of Functional Connectivity Analysis Methods and Their Interpretational Pitfalls. Front. Syst. Neurosci. 9, 175. doi:10.3389/fnsys.2015.00175
Baud, M. O., Schindler, K., and Rao, V. R. (2021). Under-sampling in Epilepsy: Limitations of Conventional EEG. Clin. Neurophysiol. Pract. 6, 41–49. doi:10.1016/j.cnp.2020.12.002
Bédard, C., Kröger, H., and Destexhe, A. (2006). Does the 1/f Frequency Scaling of Brain Signals Reflect Self-Organized Critical States. Phys. Rev. Lett. 97, 118102. doi:10.1103/physrevlett.97.118102
Beggs, J. M., and Timme, N. (2012). Being Critical of Criticality in the Brain. Front. Physiol. 3, 163. doi:10.3389/fphys.2012.00163
Bell-Pedersen, D., Cassone, V. M., Earnest, D. J., Golden, S. S., Hardin, P. E., Thomas, T. L., et al. (2005). Circadian Rhythms from Multiple Oscillators: Lessons from Diverse Organisms. Nat. Rev. Genet. 6, 544–556. doi:10.1038/nrg1633
Blanco, S., Garcia, H., Quiroga, R. Q., Romanelli, L., and Rosso, O. A. (1995). Stationarity of the EEG Series. IEEE Eng. Med. Biol. Mag. 14, 395–399. doi:10.1109/51.395321
Borbély, A. A., and Achermann, P. (1999). Sleep Homeostasis and Models of Sleep Regulation. J. Biol. Rhythms 14, 559–570. doi:10.1177/074873099129000894
Borbély, A. A., Baumann, F., Brandeis, D., Strauch, I., and Lehmann, D. (1981). Sleep Deprivation: Effect on Sleep Stages and EEG Power Density in Man. Electroencephalography Clin. Neurophysiol. 51, 483–493. doi:10.1016/0013-4694(81)90225-x
Botcharova, M., Farmer, S. F., and Berthouze, L. (2014). Markers of Criticality in Phase Synchronization. Front. Syst. Neurosci. 8, 176. doi:10.3389/fnsys.2014.00176
Bröhl, T., and Lehnertz, K. (2019). Centrality-based Identification of Important Edges in Complex Networks. Chaos 29, 033115. doi:10.1063/1.5081098
Bullmore, E., and Sporns, O. (2009). Complex Brain Networks: Graph Theoretical Analysis of Structural and Functional Systems. Nat. Rev. Neurosci. 10, 186–198. doi:10.1038/nrn2575
Cacot, P., Tesolin, B., and Sebban, C. (1995). Diurnal Variations of EEG Power in Healthy Adults. Electroencephalography Clin. Neurophysiol. 94, 305–312. doi:10.1016/0013-4694(94)00298-y
Cajochen, C., Wyatt, J. K., Czeisler, C. A., and Dijk, D. J. (2002). Separation of Circadian and Wake Duration-dependent Modulation of EEG Activation during Wakefulness. Neuroscience 114, 1047–1060. doi:10.1016/s0306-4522(02)00209-9
Calhoun, V. D., Miller, R., Pearlson, G., and Adalı, T. (2014). The Chronnectome: Time-Varying Connectivity Networks as the Next Frontier in fMRI Data Discovery. Neuron 84, 262–274. doi:10.1016/j.neuron.2014.10.015
Casson, A. J. (2019). Wearable EEG and beyond. Biomed. Eng. Lett. 9, 53–71. doi:10.1007/s13534-018-00093-6
Cermakian, N., and Boivin, D. B. (2003). A Molecular Perspective of Human Circadian Rhythm Disorders. Brain Res. Rev. 42, 204–220. doi:10.1016/s0165-0173(03)00171-1
Chang, E. F. (2015). Towards Large-Scale, Human-Based, Mesoscopic Neurotechnologies. Neuron 86, 68–78. doi:10.1016/j.neuron.2015.03.037
Chialvo, D. R. (2010). Emergent Complex Neural Dynamics. Nat. Phys. 6, 744–750. doi:10.1038/nphys1803
Cinel, C., Valeriani, D., and Poli, R. (2019). Neurotechnologies for Human Cognitive Augmentation: Current State of the Art and Future Prospects. Front. Hum. Neurosci. 13, 13. doi:10.3389/fnhum.2019.00013
Croce, P., Quercia, A., Costa, S., and Zappasodi, F. (2018). Circadian Rhythms in Fractal Features of EEG Signals. Front. Physiol. 9, 1567. doi:10.3389/fphys.2018.01567
Czeisler, C. A., and Gooley, J. J. (2007). Sleep and Circadian Rhythms in Humans. Cold Spring Harbor symposia quantitative Biol. 72, 579–597. doi:10.1101/sqb.2007.72.064
Daffertshofer, A., Ton, R., Kringelbach, M. L., Woolrich, M., and Deco, G. (2018). Distinct Criticality of Phase and Amplitude Dynamics in the Resting Brain. Neuroimage 180, 442–447. doi:10.1016/j.neuroimage.2018.03.002
Dehais, F., Karwowski, W., and Ayaz, H. (2020). Brain at Work and in Everyday Life as the Next Frontier: Grand Field Challenges for Neuroergonomics. Front. Neuroergonomics 1, 583733. doi:10.3389/fnrgo.2020.583733
Dement, W., and Kleitman, N. (1957). Cyclic Variations in EEG during Sleep and Their Relation to Eye Movements, Body Motility, and Dreaming. Electroencephalography Clin. Neurophysiol. 9, 673–690. doi:10.1016/0013-4694(57)90088-3
Dijk, D.-J., and Duffy, J. F. (1999). Circadian Regulation of Human Sleep and Age-Related Changes in its Timing, Consolidation and EEG Characteristics. Ann. Med. 31, 130–140. doi:10.3109/07853899908998789
Duboc, H., Coffin, B., and Siproudhis, L. (2020). Disruption of Circadian Rhythms and Gut Motility. J. Clin. Gastroenterol. 54, 405–414. doi:10.1097/mcg.0000000000001333
Duffy, F. H., Albert, M. S., McAnulty, G., and Garvey, A. J. (1984). Age-related Differences in Brain Electrical Activity of Healthy Subjects. Ann. Neurol. 16, 430–438. doi:10.1002/ana.410160403
Dustman, R. E., LaMarche, J. A., Cohn, N. B., Shearer, D. E., and Talone, J. M. (1985). Power Spectral Analysis and Cortical Coupling of EEG for Young and Old normal Adults. Neurobiol. Aging 6, 193–198. doi:10.1016/0197-4580(85)90049-1
Dustman, R. E., Shearer, D. E., and Emmerson, R. Y. (1993). EEG and Event-Related Potentials in normal Aging. Prog. Neurobiol. 41, 369–401. doi:10.1016/0301-0082(93)90005-d
Duun-Henriksen, J., Baud, M., Richardson, M. P., Cook, M., Kouvas, G., Heasman, J. M., et al. (2020). A new era in Electroencephalographic Monitoring? Subscalp Devices for Ultra-long-term Recordings. Epilepsia 61, 1805–1817. doi:10.1111/epi.16630
Engel, A. K., Moll, C. K. E., Fried, I., and Ojemann, G. A. (2005). Invasive Recordings from the Human Brain: Clinical Insights and beyond. Nat. Rev. Neurosci. 6, 35–47. doi:10.1038/nrn1585
Facer-Childs, E. R., Campos, B. M., Middleton, B., Skene, D. J., and Bagshaw, A. P. (2019). Circadian Phenotype Impacts the Brain's Resting-State Functional Connectivity, Attentional Performance, and Sleepiness. Sleep 42, zsz033. doi:10.1093/sleep/zsz033
Fernández, A., Zuluaga, P., Abásolo, D., Gómez, C., Serra, A., Méndez, M. A., et al. (2012). Brain Oscillatory Complexity across the Life Span. Clin. Neurophysiol. 123, 2154–2162. doi:10.1016/j.clinph.2012.04.025
Folkard, S., Wever, R. A., and Wildgruber, C. M. (1983). Multi-oscillatory Control of Circadian Rhythms in Human Performance. Nature 305, 223–226. doi:10.1038/305223a0
Foster, R. G. (2020). Sleep, Circadian Rhythms and Health. Interf. Focus. 10, 20190098. doi:10.1098/rsfs.2019.0098
Frank, G., Halberg, F., Harner, R., Matthews, J., Johnson, E., Gravem, H., et al. (1966). Circadian Periodicity, Adrenal Corticosteroids, and the EEG of normal Man. J. Psychiatr. Res. 4, 73–86. doi:10.1016/0022-3956(66)90020-3
Franken, P., and Dijk, D.-J. (2009). Circadian Clock Genes and Sleep Homeostasis. Eur. J. Neurosci. 29, 1820–1829. doi:10.1111/j.1460-9568.2009.06723.x
Garbarino, S., Lanteri, P., Sannita, W. G., Bragazzi, N. L., and Scoditti, E. (2020). Circadian Rhythms, Sleep, Immunity, and Fragility in the Elderly: The Model of the Susceptibility to Infections. Front. Neurol. 11, 558417. doi:10.3389/fneur.2020.558417
Geier, C., Lehnertz, K., and Bialonski, S. (2015). Time-dependent Degree-Degree Correlations in Epileptic Brain Networks: from Assortative to Dissortative Mixing. Front. Hum. Neurosci. 9, 462. doi:10.3389/fnhum.2015.00462
Geier, C., and Lehnertz, K. (2017). Long-term Variability of Importance of Brain Regions in Evolving Epileptic Brain Networks. Chaos 27, 043112. doi:10.1063/1.4979796
Gong, P., Nikolaev, A. R., and Van Leeuwen, C. (2003). Scale-invariant Fluctuations of the Dynamical Synchronization in Human Brain Electrical Activity. Neurosci. Lett. 336, 33–36. doi:10.1016/s0304-3940(02)01247-8
Greenblatt, R. E., Pflieger, M. E., and Ossadtchi, A. E. (2012). Connectivity Measures Applied to Human Brain Electrophysiological Data. J. Neurosci. Methods 207, 1–16. doi:10.1016/j.jneumeth.2012.02.025
Gundel, A., and Witthöft, H. (1983). Circadian Rhythm in the EEG of Man. Int. J. Neurosci. 19, 287–292. doi:10.3109/00207458309148662
Hablitz, L. M., Plá, V., Giannetto, M., Vinitsky, H. S., Stæger, F. F., Metcalfe, T., et al. (2020). Circadian Control of Brain Glymphatic and Lymphatic Fluid Flow. Nat. Commun. 11, 4411. doi:10.1038/s41467-020-18115-2
Hagemann, A., Wilting, J., Samimizad, B., Mormann, F., and Priesemann, V. (2021). Assessing Criticality in Pre-seizure Single-Neuron Activity of Human Epileptic Cortex. Plos Comput. Biol. 17, e1008773. doi:10.1371/journal.pcbi.1008773
Halberg, F. (1969). Chronobiology. Annu. Rev. Physiol. 31, 675–726. doi:10.1146/annurev.ph.31.030169.003331
Hallett, M. (2007). Transcranial Magnetic Stimulation: a Primer. Neuron 55, 187–199. doi:10.1016/j.neuron.2007.06.026
Hayashi, M., Sato, K., and Hori, T. (1994). Ultradian Rhythms in Task Performance, Self-Evaluation, and EEG Activity. Percept Mot. Skills 79, 791–800. doi:10.2466/pms.1994.79.2.791
He, B. J. (2014). Scale-free Brain Activity: Past, Present, and Future. Trends Cogn. Sci. 18, 480–487. doi:10.1016/j.tics.2014.04.003
Helmstaedter, C., Rings, T., Buscher, L., Janssen, B., Alaeddin, S., Krause, V., et al. (2021). Assessing Improvement of Unresponsive Wakefulness Condition with Evolving Functional Brain Networks. Under review.
Hildebrandt, G. (1991). Reactive Modifications of the Autonomous Time Structure in the Human Organism. J. Physiol. Pharmacol. 42, 5–27.
Hjorth, B. (1970). EEG Analysis Based on Time Domain Properties. Electroencephalography Clin. Neurophysiol. 29, 306–310. doi:10.1016/0013-4694(70)90143-4
Hjorth, B. (1973). The Physical Significance of Time Domain Descriptors in EEG Analysis. Electroencephalography Clin. Neurophysiol. 34, 321–325. doi:10.1016/0013-4694(73)90260-5
Hodkinson, D. J., O'Daly, O., Zunszain, P. A., Pariante, C. M., Lazurenko, V., Zelaya, F. O., et al. (2014). Circadian and Homeostatic Modulation of Functional Connectivity and Regional Cerebral Blood Flow in Humans under normal Entrained Conditions. J. Cereb. Blood Flow Metab. 34, 1493–1499. doi:10.1038/jcbfm.2014.109
Horovitz, S. G., Braun, A. R., Carr, W. S., Picchioni, D., Balkin, T. J., Fukunaga, M., et al. (2009). Decoupling of the Brain's Default Mode Network during Deep Sleep. Proc. Natl. Acad. Sci. 106, 11376–11381. doi:10.1073/pnas.0901435106
Huang, N. E., Shen, Z., Long, S. R., Wu, M. C., Shih, H. H., Zheng, Q., et al. (1998). The Empirical Mode Decomposition and the Hilbert Spectrum for Nonlinear and Non-stationary Time Series Analysis. Proc. R. Soc. Lond. A. 454, 903–995. doi:10.1098/rspa.1998.0193
Isaksson, A., Wennberg, A., and Zetterberg, L. H. (1981). Computer Analysis of EEG Signals with Parametric Models. Proc. IEEE 69, 451–461. doi:10.1109/proc.1981.11988
Ishii, R., Canuet, L., Aoki, Y., Hata, M., Iwase, M., Ikeda, S., et al. (2017). Healthy and Pathological Brain Aging: from the Perspective of Oscillations, Functional Connectivity, and Signal Complexity. Neuropsychobiology 75, 151–161. doi:10.1159/000486870
Iskra-Golec, I., and Smith, L. (2006). Ultradian and Asymmetric Rhythms of Hemispheric Processing Speed. Chronobiol. Int. 23, 1229–1239. doi:10.1080/07420520601077922
Kaiser, D. A. (2008). Ultradian and Circadian Effects in Electroencephalography Activity. Biofeedback 36, 148.
Kantelhardt, J. W., Koscielny-Bunde, E., Rego, H. H. A., Havlin, S., and Bunde, A. (2001). Detecting Long-Range Correlations with Detrended Fluctuation Analysis. Physica A: Stat. Mech. its Appl. 295, 441–454. doi:10.1016/s0378-4371(01)00144-3
Karoly, P. J., Goldenholz, D. M., Freestone, D. R., Moss, R. E., Grayden, D. B., Theodore, W. H., et al. (2018). Circadian and Circaseptan Rhythms in Human Epilepsy: a Retrospective Cohort Study. Lancet Neurol. 17, 977–985. doi:10.1016/s1474-4422(18)30274-6
Klass, D. W., and Brenner, R. P. (1995). Electroencephalography of the Elderly. J. Clin. Neurophysiol. 12, 116–131. doi:10.1097/00004691-199503000-00002
Klein, R., and Armitage, R. (1979). Rhythms in Human Performance: 1 1/2-hour Oscillations in Cognitive Style. Science 204, 1326–1328. doi:10.1126/science.451541
Kleitman, N. (1982). Basic Rest-Activity Cycle-22 Years Later. Sleep 5, 311–317. doi:10.1093/sleep/5.4.311
Knyazeva, M. G., Barzegaran, E., Vildavski, V. Y., and Demonet, J.-F. (2018). Aging of Human Alpha Rhythm. Neurobiol. Aging 69, 261–273. doi:10.1016/j.neurobiolaging.2018.05.018
Kreuz, T., Andrzejak, R. G., Mormann, F., Kraskov, A., Stögbauer, H., Elger, C. E., et al. (2004). Measure Profile Surrogates: A Method to Validate the Performance of Epileptic Seizure Prediction Algorithms. Phys. Rev. E Stat. Nonlin Soft Matter Phys. 69, 061915. doi:10.1103/PhysRevE.69.061915
Kuhnert, M.-T., Elger, C. E., and Lehnertz, K. (2010). Long-term Variability of Global Statistical Properties of Epileptic Brain Networks. Chaos 20, 043126. doi:10.1063/1.3504998
Kuhnert, M.-T., Geier, C., Elger, C. E., and Lehnertz, K. (2012). Identifying Important Nodes in Weighted Functional Brain Networks: A Comparison of Different Centrality Approaches. Chaos 22, 023142. doi:10.1063/1.4729185
Kurth, J. G., Rings, T., and Lehnertz, K. (2021). Testing Jump-Diffusion in Epileptic Brain Dynamics: Impact of Daily Rhythms. Entropy 23, 309. doi:10.3390/e23030309
La Rocca, D., Wendt, H., van Wassenhove, V., Ciuciu, P., and Abry, P. (2021). Revisiting Functional Connectivity for Infraslow Scale-free Brain Dynamics Using Complex Wavelets. Front. Physiol. 11, 1651. doi:10.3389/fphys.2020.578537
Laje, R., Agostino, P. V., and Golombek, D. A. (2018). The Times of Our Lives: Interaction Among Different Biological Periodicities. Front. Integr. Neurosci. 12, 10. doi:10.3389/fnint.2018.00010
Lananna, B. V., and Musiek, E. S. (2020). The Wrinkling of Time: Aging, Inflammation, Oxidative Stress, and the Circadian Clock in Neurodegeneration. Neurobiol. Dis. 139, 104832. doi:10.1016/j.nbd.2020.104832
Lavie, P., and Kripke, D. F. (1981). Ultradian Circa Hours Rhythms: A Multioscillatory System. Life Sci. 29, 2445–2450. doi:10.1016/0024-3205(81)90698-6
Lazar, A. S., Lazar, Z. I., and Dijk, D.-J. (2015). Circadian Regulation of Slow Waves in Human Sleep: Topographical Aspects. Neuroimage 116, 123–134. doi:10.1016/j.neuroimage.2015.05.012
Leguia, M. G., Andrzejak, R. G., Rummel, C., Fan, J. M., Mirro, E. A., Tcheng, T. K., et al. (2021). Seizure Cycles in Focal Epilepsy. JAMA Neurol. 78, 454–463. doi:10.1001/jamaneurol.2020.5370
Lehnertz, K., Ansmann, G., Bialonski, S., Dickten, H., Geier, C., and Porz, S. (2014). Evolving Networks in the Human Epileptic Brain. Physica D: Nonlinear Phenomena 267, 7–15. doi:10.1016/j.physd.2013.06.009
Lehnertz, K. (2011). Assessing Directed Interactions from Neurophysiological Signals-An Overview. Physiol. Meas. 32, 1715–1724. doi:10.1088/0967-3334/32/11/R01
Lehnertz, K., Bialonski, S., Horstmann, M.-T., Krug, D., Rothkegel, A., Staniek, M., et al. (2009). Synchronization Phenomena in Human Epileptic Brain Networks. J. Neurosci. Methods 183, 42–48. doi:10.1016/j.jneumeth.2009.05.015
Lehnertz, K., Geier, C., Rings, T., and Stahn, K. (2017). Capturing Time-Varying Brain Dynamics. EPJ Nonlinear Biomed. Phys. 5, 2. doi:10.1051/epjnbp/2017001
Liao, H., Mariani, M. S., Medo, M., Zhang, Y.-C., and Zhou, M.-Y. (2017). Ranking in Evolving Complex Networks. Phys. Rep. 689, 1–54. doi:10.1016/j.physrep.2017.05.001
Lindsley, D. B. (1939). A Longitudinal Study of the Occipital Alpha Rhythm in normal Children: Frequency and Amplitude Standards. Pedagogical Seminary J. Genet. Psychol. 55, 197–213. doi:10.1080/08856559.1939.10533190
Lohani, M., Payne, B. R., and Strayer, D. L. (2019). A Review of Psychophysiological Measures to Assess Cognitive States in Real-World Driving. Front. Hum. Neurosci. 13, 57. doi:10.3389/fnhum.2019.00057
Lurie, D. J., Kessler, D., Bassett, D. S., Betzel, R. F., Breakspear, M., Kheilholz, S., et al. (2020). Questions and Controversies in the Study of Time-Varying Functional Connectivity in Resting fMRI. Netw. Neurosci. 4, 30–69. doi:10.1162/netn_a_00116
Ly, J. Q. M., Gaggioni, G., Chellappa, S. L., Papachilleos, S., Brzozowski, A., Borsu, C., et al. (2016). Circadian Regulation of Human Cortical Excitability. Nat. Commun. 7, 11828. doi:10.1038/ncomms11828
Machleidt, W. (1980). Die Periodik zirkadianer und diurnaler Rhythmen im EEG. Klin Neurophysiol. 11, 155–161. doi:10.1055/s-2008-1061148
Manseau, C., and Broughton, R. J. (1984). Bilaterally Synchronous Ultradian EEG Rhythms in Awake Adult Humans. Psychophysiology 21, 265–273. doi:10.1111/j.1469-8986.1984.tb02933.x
Matenchuk, B. A., Mandhane, P. J., and Kozyrskyj, A. L. (2020). Sleep, Circadian Rhythm, and Gut Microbiota. Sleep Med. Rev. 53, 101340. doi:10.1016/j.smrv.2020.101340
McIntosh, A. R., Vakorin, V., Kovacevic, N., Wang, H., Diaconescu, A., and Protzner, A. B. (2014). Spatiotemporal Dependency of Age-Related Changes in Brain Signal Variability. Cereb. Cort. 24, 1806–1817. doi:10.1093/cercor/bht030
Mills, J. N. (1966). Human Circadian Rhythms. Physiol. Rev. 46, 128–171. doi:10.1152/physrev.1966.46.1.128
Mitsis, G. D., Anastasiadou, M. N., Christodoulakis, M., Papathanasiou, E. S., Papacostas, S. S., and Hadjipapas, A. (2020). Functional Brain Networks of Patients with Epilepsy Exhibit Pronounced Multiscale Periodicities, Which Correlate with Seizure Onset. Hum. Brain Mapp. 41, 2059–2076. doi:10.1002/hbm.24930
Mizrahi-Kliger, A. D., Kaplan, A., Israel, Z., and Bergman, H. (2018). Desynchronization of Slow Oscillations in the Basal Ganglia during Natural Sleep. Proc. Natl. Acad. Sci. USA 115, E4274–E4283. doi:10.1073/pnas.1720795115
Mohawk, J. A., Green, C. B., and Takahashi, J. S. (2012). Central and Peripheral Circadian Clocks in Mammals. Annu. Rev. Neurosci. 35, 445–462. doi:10.1146/annurev-neuro-060909-153128
Mormann, F., Lehnertz, K., David, P., and E. Elger, C. (2000). Mean Phase Coherence as a Measure for Phase Synchronization and its Application to the EEG of Epilepsy Patients. Physica D: Nonlinear Phenomena 144, 358–369. doi:10.1016/S0167-2789(00)00087-7
Nakamura, K., Brown, R. A., Narayanan, S., Collins, D. L., and Arnold, D. L. (2015). Alzheimer’s Disease Neuroimaging Initiative, et al.Diurnal fluctuations in brain volume: statistical analyses of MRI from large populations. Neuroimage 118, 126–132. doi:10.1016/j.neuroimage.2015.05.077
Ngamga, E. J., Bialonski, S., Marwan, N., Kurths, J., Geier, C., and Lehnertz, K. (2016). Evaluation of Selected Recurrence Measures in Discriminating Pre-ictal and Inter-ictal Periods from Epileptic EEG Data. Phys. Lett. A 380, 1419–1425. doi:10.1016/j.physleta.2016.02.024
Nguyen, T., Babawale, O., Kim, T., Jo, H. J., Liu, H., and Kim, J. G. (2018). Exploring Brain Functional Connectivity in Rest and Sleep States: a fNIRS Study. Sci. Rep. 8, 16144. doi:10.1038/s41598-018-33439-2
Niedermeyer, E., and Lopes da Silva, F. (2005). Electroencephalography: Basic Principles, Clinical Applications, and Related Fields. Philadelphia: Lippincott Williams and Williams.
Nobukawa, S., Kikuchi, M., and Takahashi, T. (2019). Changes in Functional Connectivity Dynamics with Aging: a Dynamical Phase Synchronization Approach. Neuroimage 188, 357–368. doi:10.1016/j.neuroimage.2018.12.008
Oken, B. S., and Chiappa, K. H. (1988). Short-term Variability in EEG Frequency Analysis. Electroencephalography Clin. Neurophysiol. 69, 191–198. doi:10.1016/0013-4694(88)90128-9
Open Science Collaboration (2015). Estimating the Reproducibility of Psychological Science. Science 349, aac4716. doi:10.1126/science.aac4716
Orban, C., Kong, R., Li, J., Chee, M. W. L., and Yeo, B. T. T. (2020). Time of Day Is Associated with Paradoxical Reductions in Global Signal Fluctuation and Functional Connectivity. Plos Biol. 18, e3000602. doi:10.1371/journal.pbio.3000602
Papo, D. (2014). Functional Significance of Complex Fluctuations in Brain Activity: from Resting State to Cognitive Neuroscience. Front. Syst. Neurosci. 8, 112. doi:10.3389/fnsys.2014.00112
Papo, D. (2013). Time Scales in Cognitive Neuroscience. Front. Physiol. 4, 86. doi:10.3389/fphys.2013.00086
Pereda, E., Quiroga, R. Q., and Bhattacharya, J. (2005). Nonlinear Multivariate Analysis of Neurophysiological Signals. Prog. Neurobiol. 77, 1–37. doi:10.1016/j.pneurobio.2005.10.003
Piarulli, A., Bergamasco, M., Thibaut, A., Cologan, V., Gosseries, O., and Laureys, S. (2016). EEG Ultradian Rhythmicity Differences in Disorders of Consciousness during Wakefulness. J. Neurol. 263, 1746–1760. doi:10.1007/s00415-016-8196-y
Polich, J. (1997). EEG and ERP Assessment of normal Aging. Electroencephalography Clin. Neurophysiology/Evoked Potentials Section 104, 244–256. doi:10.1016/s0168-5597(97)96139-6
Porz, S., Kiel, M., and Lehnertz, K. (2014). Can Spurious Indications for Phase Synchronization Due to Superimposed Signals Be Avoided. Chaos 24, 033112. doi:10.1063/1.4890568
Press, W. H., and Rybicki, G. B. (1989). Fast Algorithm for Spectral Analysis of Unevenly Sampled Data. ApJ 338, 277–280. doi:10.1086/167197
Press, W. H., Teukolsky, S. A., Vetterling, W. T., and Flannery, B. P. (2007). Numerical Recipes: The Art of Scientific Computing. 3rd edn. Cambridge, UK: Cambridge University Press.
Preti, M. G., Bolton, T. A., and Van De Ville, D. (2017). The Dynamic Functional Connectome: State-Of-The-Art and Perspectives. Neuroimage 160, 41–54. doi:10.1016/j.neuroimage.2016.12.061
Racz, F. S., Stylianou, O., Mukli, P., and Eke, A. (2018). Multifractal Dynamic Functional Connectivity in the Resting-State Brain. Front. Physiol. 9, 1704. doi:10.3389/fphys.2018.01704
Rashid, M., Sulaiman, N., Abdul Majeed, A., Musa, R. M., Nasir, A. F., Bari, B. S., et al. (2020). Current Status, Challenges, and Possible Solutions of EEG-Based Brain-Computer Interface: A Comprehensive Review. Front. Neurorobot. 14, 25. doi:10.3389/fnbot.2020.00025
Rensing, L., an der Heiden, U., and Mackey, M. C. (1987). “Temporal Disorder in Human Oscillatory Systems,” : in Proceedings of an International Symposium University of Bremen, 8–13 September 1986 (Springer Science & Business Media).
Rieke, C., Mormann, F., Andrzejak, R. G., Kreuz, T., David, P., Elger, C. E., et al. (2003). Discerning Nonstationarity from Nonlinearity in Seizure-free and Preseizure EEG Recordings from Epilepsy Patients. IEEE Trans. Biomed. Eng. 50, 634–639. doi:10.1109/TBME.2003.810684
Rings, T., Mazarei, M., Akhshi, A., Geier, C., Tabar, M. R. R., and Lehnertz, K. (2019). Traceability and Dynamical Resistance of Precursor of Extreme Events. Sci. Rep. 9, 1744. doi:10.1038/s41598-018-38372-y
Sakkalis, V. (2011). Review of Advanced Techniques for the Estimation of Brain Connectivity Measured with EEG/MEG. Comput. Biol. Med. 41, 1110–1117. doi:10.1016/j.compbiomed.2011.06.020
Sleimen-Malkoun, R., Perdikis, D., Müller, V., Blanc, J. L., Huys, R., Temprado, J. J., et al. (2015). Brain Dynamics of Aging: Multiscale Variability of EEG Signals at Rest and during an Auditory Oddball Task. Eneuro. 2, e0067. doi:10.1523/ENEURO.0067-14.2015
Specht, K. (2020). Current Challenges in Translational and Clinical fMRI and Future Directions. Front. Psychiatry 10, 924. doi:10.3389/fpsyt.2019.00924
Spengler, C. M., Czeisler, C. A., and Shea, S. A. (2000). An Endogenous Circadian Rhythm of Respiratory Control in Humans. J. Physiol. 526, 683–694. doi:10.1111/j.1469-7793.2000.00683.x
Stam, C. J., and De Bruin, E. A. (2004). Scale-free Dynamics of Global Functional Connectivity in the Human Brain. Hum. Brain Mapp. 22, 97–109. doi:10.1002/hbm.20016
Stam, C. J. (2005). Nonlinear Dynamical Analysis of EEG and MEG: Review of an Emerging Field. Clin. Neurophysiol. 116, 2266–2301. doi:10.1016/j.clinph.2005.06.011
Steriade, M., and Hobson, J. A. (1976). Neuronal Activity during the Sleep-Waking Cycle. Prog. Neurobiol. 6, 157–376. doi:10.1016/0301-0082(76)90013-7
Stylianou, O., Racz, F. S., Eke, A., and Mukli, P. (2020). Scale-free Coupled Dynamics in Brain Networks Captured by Bivariate Focus-Based Multifractal Analysis. Front. Physiol. 11, 615961. doi:10.3389/fphys.2020.615961
Thomas, C., Sadeghi, N., Nayak, A., Trefler, A., Sarlls, J., Baker, C. I., et al. (2018). Impact of Time-Of-Day on Diffusivity Measures of Brain Tissue Derived from Diffusion Tensor Imaging. Neuroimage 173, 25–34. doi:10.1016/j.neuroimage.2018.02.026
Torsvall, L., and åAkerstedt, T. (1987). Sleepiness on the Job: Continuously Measured EEG Changes in Train Drivers. Electroencephalography Clin. Neurophysiol. 66, 502–511. doi:10.1016/0013-4694(87)90096-4
Tsuji, Y., and Kobayashi, T. (1988). Short and Long Ultradian EEG Components in Daytime Arousal. Electroencephalography Clin. Neurophysiol. 70, 110–117. doi:10.1016/0013-4694(88)90111-3
Uddin, L. Q. (2020). Bring the Noise: Reconceptualizing Spontaneous Neural Activity. Trends Cogn. Sci. 24, 734–746. doi:10.1016/j.tics.2020.06.003
Vecchio, F., Miraglia, F., and Maria Rossini, P. (2017). Connectome: Graph Theory Application in Functional Brain Network Architecture. Clin. Neurophysiol. Pract. 2, 206–213. doi:10.1016/j.cnp.2017.09.003
Viana, P. F., Duun‐Henriksen, J., Glasstëter, M., Dümpelmann, M., Nurse, E. S., Martins, I. P., et al. (2021). 230 Days of Ultra Long‐term Subcutaneous EEG: Seizure Cycle Analysis and Comparison to Patient Diary. Ann. Clin. Transl. Neurol. 8, 288–293. doi:10.1002/acn3.51261
Weisdorf, S., Duun‐Henriksen, J., Kjeldsen, M. J., Poulsen, F. R., Gangstad, S. W., and Kjær, T. W. (2019). Ultra‐long‐term Subcutaneous home Monitoring of Epilepsy-490 Days of EEG from Nine Patients. Epilepsia 60, 2204–2214. doi:10.1111/epi.16360
Wilkat, T., Rings, T., and Lehnertz, K. (2019). No Evidence for Critical Slowing Down Prior to Human Epileptic Seizures. Chaos 29, 091104. doi:10.1063/1.5122759
Yang, D., Shin, Y.-I., and Hong, K.-S. (2021). Systemic Review on Transcranial Electrical Stimulation Parameters and EEG/fNIRS Features for Brain Diseases. Front. Neurosci. 15, 274. doi:10.3389/fnins.2021.629323
Zaer, H., Deshmukh, A., Orlowski, D., Fan, W., Prouvot, P.-H., Glud, A. N., et al. (2021). An Intracortical Implantable Brain-Computer Interface for Telemetric Real-Time Recording and Manipulation of Neuronal Circuits for Closed-Loop Intervention. Front. Hum. Neurosci. 15, 36. doi:10.3389/fnhum.2021.618626
Zalesky, A., Fornito, A., Cocchi, L., Gollo, L. L., and Breakspear, M. (2014). Time-resolved Resting-State Brain Networks. Proc. Natl. Acad. Sci. 111, 10341–10346. doi:10.1073/pnas.1400181111
Keywords: electroencephalography, biological rhythms, brain dynamics, statistical moments, synchronization, functional brain networks, clustering coefficient, centrality
Citation: Lehnertz K, Rings T and Bröhl T (2021) Time in Brain: How Biological Rhythms Impact on EEG Signals and on EEG-Derived Brain Networks. Front. Netw. Physiol. 1:755016. doi: 10.3389/fnetp.2021.755016
Received: 07 August 2021; Accepted: 13 September 2021;
Published: 27 September 2021.
Edited by:
Michal Zochowski, University of Michigan, United StatesCopyright © 2021 Lehnertz, Rings and Bröhl. This is an open-access article distributed under the terms of the Creative Commons Attribution License (CC BY). The use, distribution or reproduction in other forums is permitted, provided the original author(s) and the copyright owner(s) are credited and that the original publication in this journal is cited, in accordance with accepted academic practice. No use, distribution or reproduction is permitted which does not comply with these terms.
*Correspondence: Klaus Lehnertz, a2xhdXMubGVobmVydHpAdWtib25uLmRl