- 1Keck Laboratory for Network Physiology, Department of Physics, Boston University, Boston, MA, United States
- 2Harvard Medical School and Division of Sleep Medicine, Brigham and Women’s Hospital, Boston, MA, United States
- 3Bulgarian Academy of Sciences, Institute of Solid State Physics, Sofia, Bulgaria
State-of-the-Art and Fundamental Questions
The human organism comprises various physiological and organ systems, each with its own structural organization and functional complexity, leading to complex, transient, fluctuating and nonlinear output dynamics (Ivanov et al., 1996, 1999a; Goldberger et al., 2002). Basic physiology and clinical medicine widely employ a reductionist approach, and consider health and disease through the prism of the structural organization and dynamics of individual organ systems. Further, physiological states and functions at the organism level are traditionally defined by the dynamics of organ systems, their modulation and changes in response to transitions in biochemical signaling and neuro-autonomic regulation due to internal, external and pathologic perturbations (Amaral et al., 1998; Ivanov et al., 1999b; Bunde et al., 2000; Kantelhardt et al., 2002; Karasik et al., 2002; Hu et al., 2004b; Ivanov et al., 2004; Schmitt et al., 2009; Schumann et al., 2010).
However, the human organism is an integrated network, where multi-component physiological systems, each with its own regulatory mechanism, continuously interact to coordinate their functions. Coordinated network interactions among organs are essential to generating distinct physiological states and maintaining health. Physiological interactions occur at multiple levels of integration and across spatiotemporal scales to optimize organ functions and synchronize their dynamics at the organism level. Often manifested as synchronized bursting activities with certain time delays, these interactions are mediated by various signaling pathways that work in parallel to facilitate stochastic and nonlinear feedbacks (Ivanov et al., 1998; Hausdorff et al., 2001) across scales leading to different coupling forms (Bartsch et al., 2014; Bartsch and Ivanov, 2014). Having structurally intact and functioning systems is not sufficient to maintain health.
In addition to the state of individual organ systems, coordinated network interactions among systems and sub-systems are essential to generate distinct physiologic states and behaviors at the organism level, such as wake, sleep and sleep stages, rest and exercise, stress and anxiety, cognition, consciousness and unconsciousness. Disrupting organ communications can lead to dysfunction of individual systems or trigger a cascade of failures leading to a breakdown and collapse of the entire organism, as observed under clinical conditions such as sepsis, coma and multiple organ failure (Buchman, 2006; Moorman et al., 2016; Shashikumar et al., 2017; Foreman et al., 2021). Yet, despite the vast progress and achievements in systems biology and integrative physiology in the last decades, and the importance to basic physiology and clinical practice, we do not know the principles and mechanisms through which diverse systems and sub-systems in the human body dynamically interact as a network and integrate their functions to generate physiological states in health and disease.
The new multi-disciplinary field of Network Physiology aims to address these fundamental questions (Bashan et al., 2012; Ivanov and Bartsch, 2014). In addition to defining health and disease through structural, dynamical and regulatory changes in individual physiological systems, the new conceptual framework of Network Physiology focuses on the coordination and network interactions among diverse organ systems and sub-systems as a hallmark of physiologic state and function.
A fundamental problem in physical, biological, and physiological systems is to understand phenomena where global behaviors across systems emerge out of networked interactions among dynamically changing entities with coupling forms that are often nonlinear and change as a function of time. Early attempts to study multiple sub-systems within the cardiovascular system (Guyton et al., 1972), later extended to other systems (Coleman and Randall, 1983), were modeled on electric circuit diagrams that simply sum up individual measurements from separate physiologic experiments and could not begin to account for the transient dynamics and emergent non-linear behaviors which are hallmarks of human physiology. Efforts in recent years to understand specific physiological interactions such as cardio-respiratory coupling (Bartsch et al., 2012; Angelone and Coulter, 1964; Rosenblum et al., 1996; Schäfer et al., 1998; Mrowka et al., 2000; Pikovsky et al., 2001; Song and Lehrer, 2003; Garcia-Retortillo et al., 2019) (e.g., we all know our hearts race when we breathe in) did not address the collective behavior of organ-to-organ interactions.
Identifying and quantifying these interactions is a major challenge due to the complex dynamics of organ systems (Ivanov et al., 2001; Suki et al., 2003; Hu et al., 2004a). Such complexity arises from intrinsic interactions of multi-component cellular and neuronal sub-systems that build and regulate each organ in the human body (Figure 1), leading to intermittent, scale-invariant and nonlinear output signals. This is further compounded by various coupling and feedback interactions between organ systems that continuously vary in time (Stankovski et al., 2015), the nature of which is not understood. In fact, it was recently discovered that two organ systems can communicate through several forms of coupling that simultaneously coexist (Bartsch et al., 2014; Bartsch and Ivanov, 2014). This poses a barrier to our understanding of how organs integrate their functions to generate emergent behavior of the human body as a single entity able to adapt to internal and external perturbations, and mantain homeostasis (Fossion et al., 2018). The framework of computational neuroscience and systems physiology, which focus on pathways of neuron-to-neuron signaling and on integration within organ systems, do not provide adequate tools and are not of help here (Lehnertz et al., 2020). Even the most recent advances in systems biology and integrative physiology (Large, 2011) continue to focus on how genetic/cellular interactions relate to function at the single tissue and organ level; occasionally, physiologists will leap directly from micro-level sub-cellular and cellular insights on genomic, proteomic and metabolic interactions to “macroscopic” epidemiological observations. There is a wide gap in research efforts and knowledge at the “mesoscopic” level of horizontal network interactions across organ systems and sub-systems essential to maintaining health (Figure 2). The new field of Network Physiology has emerged to fill this gap, and to address the fundamental question of how physiological systems synchronize and integrate their dynamics as a network to optimize functions and to maintain health.
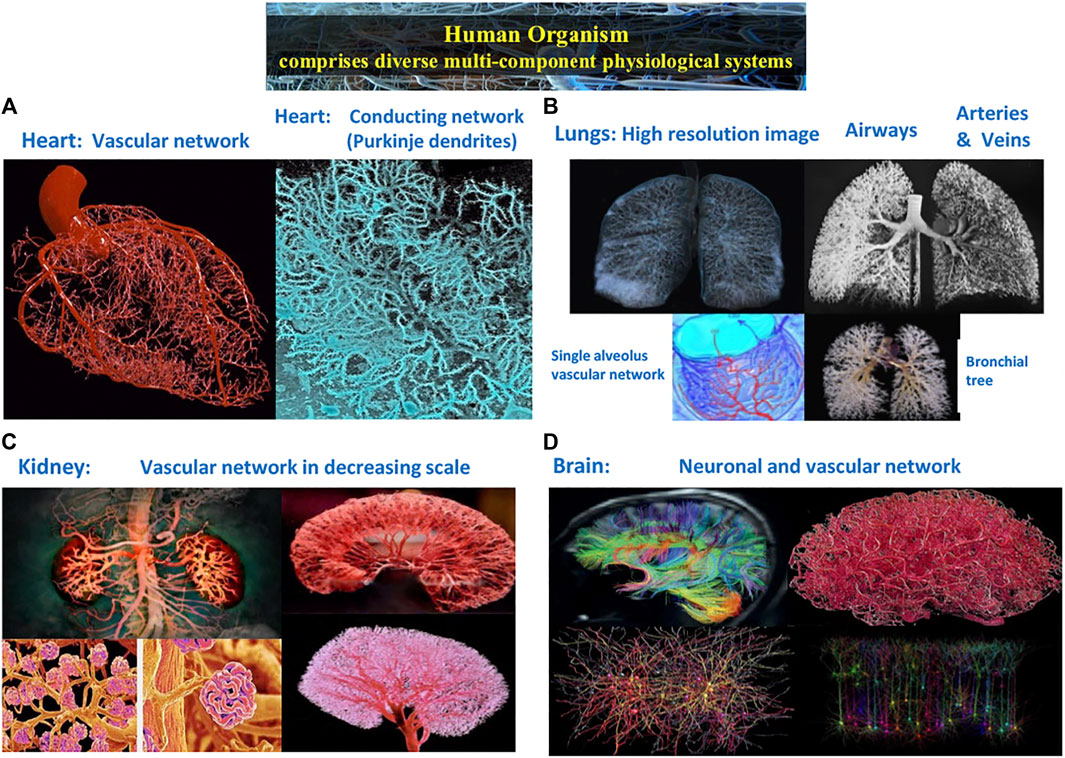
FIGURE 1. Complex structural and functional networks underlie the dynamics and mechanisms of regulation across spatial and temporal scales in multi-component physiological and organ systems. (A) Heart: vascular network and conducting network of Purkinje dendrites embedded in the myocardial muscle. (B) Lungs: airways and vascular networks from the bronchial tree to a single alveolus. (C) Kidney: vascular network revealing cortex structure at large scales, nephrons at intermediate scales and single glomeruli at small scales. (D) Brain: diffusion tensor image of connectivity micro-structure networks showing the location, orientation, and anisotropy of white matter tracts; vascular networks; and neuronal population networks representing levels of activation for individual neural cells from real time electron microscope imaging.
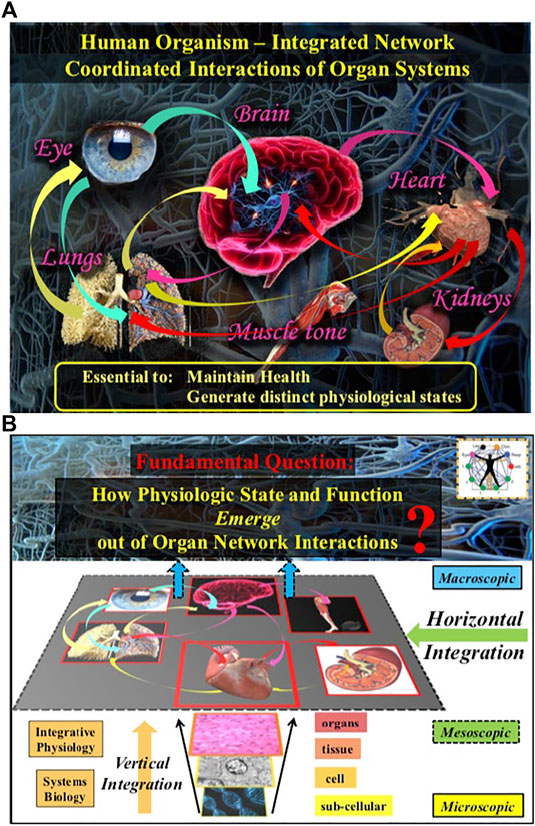
FIGURE 2. The human organism is an integrated network where diverse physiological and organ systems continuously interact to optimize and coordinate their functions. (A) Network interactions across spatial levels and temporal scales within systems and among systems are essential to generate various physiological states and to maintain health. (B) A fundamental question in Network Physiology is how physiological states and functions emerge out of vertical and horizontal network integration from the sub-cellular to the organism level.
Two major stumbling blocks hamper investigations in this direction: 1) long-term, continuous, parallel recordings from multiple organ systems are not readily available across different physiologic states and conditions (Finazzi et al., 2018); collecting such data in both ambulatory and clinical ICU/hospital environment is particularly problematic because medical devices are often not interoperable; 2) there are no well-established analytic methodology, computational tools, theoretical framework capable of probing organ interactions from continuous streams of data, that are simultaneously applicable to diverse organ systems with different output dynamics. The complex, multi-scale dynamics of organ systems make it extremely challenging to identify and quantify the network of organ interactions.
In dynamic networks of physiological interactions links represent coordination and synchronization between systems and sub-systems, and exhibit transient characteristics. A key question is how physiological states and functions emerge out of the collective network dynamics of integrated systems. While network structure may play a role in generating various states and functions, different global behaviors at the organism level can emerge from the same network topology due to changes in systems dynamics (network nodes) and modulations in the functional form of physiologic interactions (network links). This poses new challenges in developing generalized methodology adequate to quantify complex dynamics of networks where nodes represent systems with diverse dynamics, interacting through different forms of coupling that continuously change in time with transitions across states and conditions. Novel concepts and approaches derived from recent advances in network theory, coupled dynamical systems, statistical and computational physics, biomedical informatics, signal processing and biological engineering show promise to provide new insights into the complexity of physiological structure and function in health and disease, bridging across levels of integration sub-cellular signaling with inter-cellular interactions and communications among integrated organ systems and sub-systems. These advances form first building blocks in the methodological formalism and theoretical framework necessary to address the problems and challenges in the field of Network Physiology.
Network Physiology focuses on inferring coupling and dynamical interactions among organ systems based on continuous streams of synchronous recordings of key physiologic parameters and output signals from multiple systems. In contrast to traditional complex network theory, where edges/links are constant and represent static graphs of association, novel approaches in Network Physiology have to take into consideration 1) the complex dynamics of individual systems (network nodes), 2) dynamical aspects of network links representing organ communications in real time, 3) on the evolution of organ interactions with time and 4) emergence of collective network behavior in response to changes in physiologic states and conditions. This new field will integrate empirical and theoretical knowledge across disciplines with the aim to understand in different contexts, from extensive data analysis and modeling approaches to clinical practice, how diverse organs, physiological systems and sub-systems dynamically interact as a network from the cellular to the organism level to produce various physiological states and functions in health and disease.
In classical graph theory, network nodes and network links are static and represent statistical correlations and dependence rather than dynamical coupling. Dynamical aspects in classical network theory arise from removing/adding links or nodes and from diffusion processes of flow on a fixed network, where emphasis is given on the consequences of network topology and structure on networks function to transmit information. In contrast, in Network Physiology, links represent dynamical coupling and coordination between diverse systems and sub-systems and have transient characteristics. Changes in the dynamicsof physiological systems (network nodes) can propagate via ‘elastic’ time-varying links to affect the dynamics of other nodes, and thus, alter the behavior of the entire network. A fundamental question is how to quantify, predict and control emergent global behaviors in temporal multiplex networks of diverse dynamic systems interacting simultaneously through various functional forms of coupling. In such adaptive networks, markedly different global behaviors can emerge from the same network topology due to minor temporal changes in the dynamics of a node or in the functional form of a link (Ivanov and Bartsch, 2014). This directly relates to the question of how a variety of physiologic states and functions emerge out of the collective dynamics of integrated physiological and organ systems (Bartsch et al., 2015; Liu et al., 2015b; Rizzo et al., 2020; Ivanov et al., 2017, 2021b). This poses new challenges to further develop generalized methodology adequate to quantify complex dynamics of networks where nodes are not identical but represent diverse dynamical systems with diverse forms of coupling which continuously change in time. Such investigations are not simply an application of established concepts and approaches in complex networks theory to existing fields of biomedical research. Because of the new type of problems, the specificity of related challenges, and the necessity of new theoretical framework and interdisciplinary efforts, Network Physiology has developed into a new field of research (Figure 3).
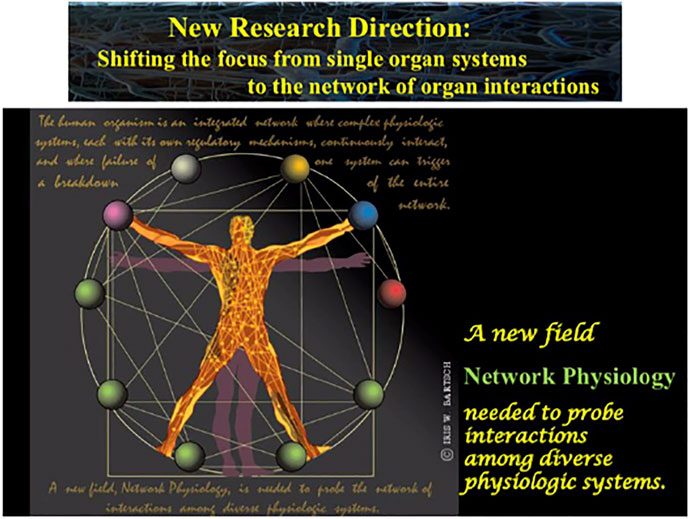
FIGURE 3. A new field, Network Physiology, has emerged, shifting the focus from single organ systems to the network of physiologic interactions with the aim to uncover basic laws of communication and principles of integration in networks of diverse physiological systems and their role in generating global behaviors at the organism level.
The scope of Network Physiology extends far beyond applying knowledge from one field (statistical physics, applied mathematics, informatics, network theory) to solve problems in another (systems biology, neuroscience, physiology and medicine). New computational and analytical approaches are needed to extract information from complex data, to infer transient interactions between dynamically changing systems, and to quantify global behavior at the organism level generated by networks of interactions that are function of time. In fact, in recent years, we have already witnessed the broad impact of introducing novel concepts and methods derived from modern statistical physics and network theory to biology and medicine, shifting the paradigm from reductionism to a new integrative framework essential to address fundamentally new problems in systems biology (Yao et al., 2019; Prats-Puig et al., 2020; Corkey and Deeney, 2020; Rizi et al., 2021; Barajas-Martínez et al., 2020), neuroscience (Castelluzzo et al., 2020; Pa¨eske et al., 2020; Fesce, 2020; Stramaglia et al., 2021), physiology (Podobnik et al., 2020; Zmazek et al., 2021), clinical medicine (Loscalzo and Barabasi, 2011; Delussi et al., 2020; Li et al., 2020; Liu et al., 2020; McNorgan et al., 2020; Tan et al., 2020; Haug et al., 2021; Liu et al., 2021) and even drug discovery (Hopkins, 2008). A central focus of research within this integrative framework is the interplay between structural connectivity and functional dependency, a key problem in neuroscience, brain research (Bullmore and Sporns, 2009; Gallos et al., 2012; Rothkegel and Lehnertz, 2014; Liu et al., 2015a; Bolton et al., 2020; Wang and Liu, 2020) and human physiology (Pereira-Ferrero et al., 2019; Lavanga et al., 2020; Barajas-Martínez et al., 2021; Gao et al., 2018; Balagué et al., 2020; Porta et al., 2017; Lioi et al., 2017; Jiang et al., 2021). As a result, new physical models have been motivated and proposed to investigate the dynamical consequences of adaptive networks (de Arcangelis et al., 2006; Millman et al., 2010; Gómez-Gardenes et al., 2011; Komarov and Pikovsky, 2013; Pecora et al., 2014; Zhang et al., 2015; Wellman et al., 2018; Wang et al., 2019; Lombardi et al., 2020; Masamura et al., 2020; Suki et al., 2020; Rowland Adams and Stefanovska, 2021; Polizzi et al., 2021), which in turn trigger more theoretical questions. These synergetic effects certainly establish Network Physiology as a new field in the landscape of contemporary biomedical and interdisciplinary research. Understanding the relationship, conceptual difference, the broad horizon and impact of Network Physiology is important to facilitate an active and productive dialog among physicists, biologists, physiologists, neuroscientists and medical clinicians.
The field of Network Physiology will draw on and facilitate the development of multiple areas of empirical and theoretical, basic and clinical research—from advanced methods for nonlinear dynamics and synchronization phenomena, theory of dynamical systems and adaptive networks, data-driven models of complex systems and their interactions, control theory in dynamic networks, information theory for coupling inference and causality for non-stationary and non-linear systems, new generation of data-intensive AI and machine learning algorithms for inference of network dynamics and function, biomedical engineering of sensors networks and human-machine interfaces—to numerous areas in basic physiology and clinical medicine, including proteomic and metabolic networks, networks of cell assembles, neuronal populations, networks of the autonomic and peripheral nervous systems, brain structural and functional networks, biomechanical networks in tissues, networks in the cardio-vascular and respiratory systems, network structures and dynamics in the kidneys and renal system, networks of skeletal muscle groups and muscle fibers, pairwise and network interactions of organ systems and sub-systems, and their manifestations in aging, exercise and sports, as well as in numerous clinical and pathological conditions with impact on multiple physiological systems in the human body, such as concussion and traumatic brain injury, cardiac arrest, sleep and neurodegenerative disorders, diabetes and obesity, maternal-fetal and neonatal care, sepsis, coma and multiple organ failure.
The field of Network Physiology also involves bioengineering research and development of novel biomedical device platforms for synchronized high-frequency recordings from multiple physiological systems both in the clinical ICU and hospital environment as well as networks of wearable sensors for continuous measurement of physiological parameters in free ambulatory conditions. Integrated networks of clinical monitoring devices and wearable sensors that provide high-precision, synchronous signals are essential to establish causality and pathways of dynamical interactions in networks of physiological systems, to track the evolution of these interactions across states and conditions, to develop new class of network-based markers for diagnosis and prognosis of clinical conditions and critical events. Thus, future developments in Network Physiology will lead to establishing a new kind of Big Data, the Human Physiolome (Figure 4), containing large-scale signals from multiple systems and an associated blueprint repository of hundreds of network maps representing physiological systems interactions for different states, conditions and diseases. New machine learning and AI algorithms trained to identify both topological characteristics as well as temporal dynamics of physiological networks, able to predict hierarchical re-organization and cascades of breakdown in dynamic networks, have to be developed to classify states, functions and conditions based on network physiology maps from large populations of subjects.
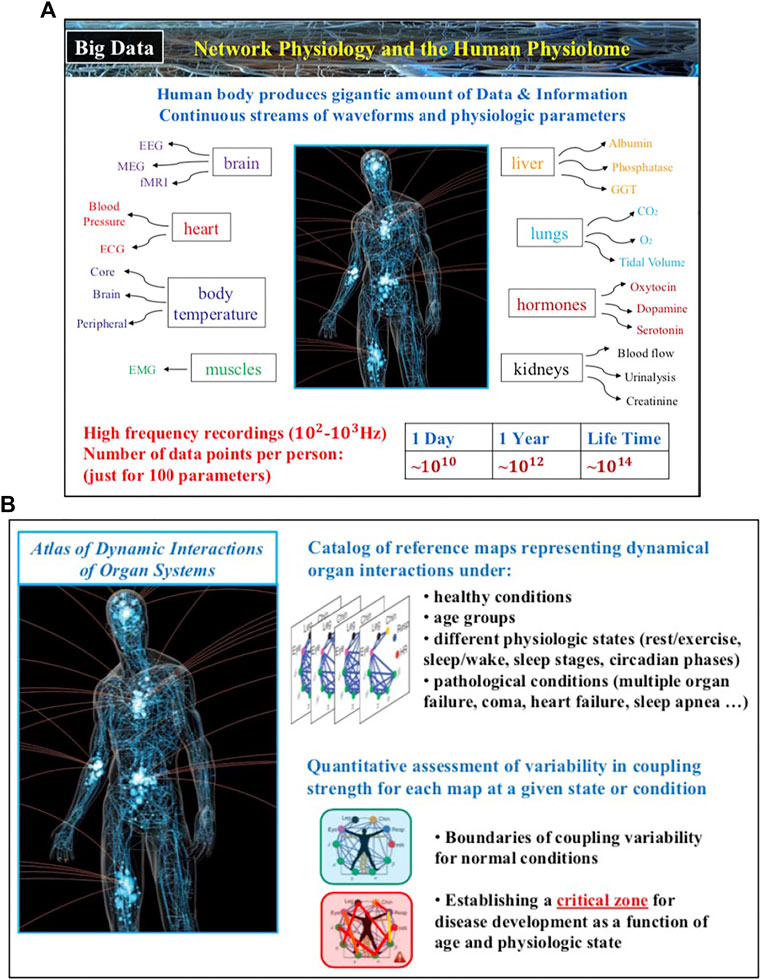
FIGURE 4. The human body generates continuous streams of physiological signals as output dynamics of various systems and physiological parameters that contain a wealth of information about the state of individual systems and the nature of their network interactions. (A) In 24 h just one hundred basic physiologic parameters recorded with 100 Hz generate 109–1010 data points, of the same order as the number of nucleotides in the human genome. (B) Novel methods and approaches within the framework of Network Physiology aim to establish associations between distinct physiologic states and pathological conditions with the structure and dynamics in physiological networks, and thus, lay the foundations of the Human Physiolome, a first of a kind Big Data of blueprint reference network maps representing states and conditions through network interactions across levels in the human body.
Major Challenges
Quantifying networks of physiological interactions poses major challenges. These challenges arise from several levels of complexity inherent to the dynamics of organ systems. Physiological systems exhibit non-stationary, intermittent, scale-invariant and nonlinear behaviors (Ivanov et al., 1999a; Ivanov et al., 2001). Their output dynamics transiently change in time with different physiologic states and under pathologic conditions in response to changes in the underlying control mechanisms. The structural and neural control networks that underlie each physiologic organ system include many individual components, connected through nonlinear interactions, that lead to high degree of freedom. This complexity is further compounded by various coupling and feedback interactions among different systems, the nature of which is not understood.
Moreover, physiological systems operate on a broad range of time scales from milliseconds to hours and exhibit different types of output dynamics—oscillatory, stochastic or mixed—and thus, earlier concepts of treating them as coupled chaotic oscillators need to be extended (Ashkenazy et al., 2002; Chen et al., 2006; Xu et al., 2006). Further, each integrated physiological system exhibits multiple simultaneous interactions and different forms of coupling with other systems, where interactions among systems vary in time (Bartsch and Ivanov, 2014; Bartsch et al., 2012; Bartsch et al., 2014). This leads to a transient multi-layer network structure consisting of distinct physiologic networks. Importantly, global network dynamics of the entire organismcannot be simply expressed as a sum of the behaviors of individual systems, and can be strongly influenced by minor changes in the behavior of one system and/or in the relative strength of interactions with other systems, even when network topology remains unchanged.
There are several fundamental and challenging questions in the field of Network Physiology. Physiological systems at the sub-cellular, tissue and organism level exhibit bursting dynamics (Ferrari et al., 2015) that result from molecular and cellular level signaling processes. A key question is whether synchronous bursts in systems dynamics can reveal fundamental information regarding the nature of network communications among systems. Specifically, what is the role of synchronized bursting dynamics in mediating neural control and flow of information between physiologically relevant brain rhythms and corresponding neuronal populations within and across brain areas, between cortical rhythms and other physiological systems, and among key organ systems. Can studies of transient synchronous bursts in systems dynamics reveal fundamental laws of network communications among systems. Can we uncover dynamical characteristics of brain- organ and organ-organ networks as a new signature of physiologic control, and can we establish robust associations of network structure and dynamics with physiologic states and functions at the organism level. Are there basic universal principles of integration in networks of diverse physiological systems that underly interactions between motifs, modules, sub-networks and networks formed by physiological systems at different levels and time scales. How physiological states and functions emerge from network interactions among diverse systems, what degree of coupling, link intensity distribution and coordination between systems dynamics is necessary to facilitate a physiological state at the organism level. How physiological networks hierarchically re-organize with transitions from one physiological state to another in response to changes in autonomic regulation, and what is the “critical zone” of variability in network interactions beyond which critical events occur leading to cascades of breakdown across the organism.
These fundamental questions pose challenges to developing new methodology and theoretical framework to identify and quantify dynamical interactions among systems with very different characteristics and signal outputs. There is an urgent need for adopting a cross-scale perspective to investigate the multi-scale regulatory mechanisms underlying the overall network physiology and its relation to physiological states and functions, and to address the heterogeneity, multi-modality and complexity of physiological processes. We need rigorous mathematical and algorithmic techniques that can extract causal interdependencies between systems across different scales while overcoming various noise sources. Progress in this direction will require new strategies to quantify time-varying information flow among diverse physiological processes across scales, and determine how it influences the global dynamics of complex physiological networks. Intrinsically related to future efforts on quantifying causal dependencies and control principles in biological and physiological networks, it will be essential to develop robust optimization algorithms capable to reconstruct or infer the structure and dynamics of complex interdependent networks while overcoming partial observability, noise induced defects and adversarial interventions caused by external perturbations, bacterial or viral infections.
Recent research efforts have focused on temporal networks (Holme and Saramäki, 2012), where traditional graph approaches to static network topology are extended to time-dependent structures, and are employed to investigate new phenomena related to changes in fundamental properties of networks, including the loss of transitivity and the emergence of time ordering of links (Holme and Saramäki, 2012). However, the inherent complexity of physiological systems and the problems that arise from network physiology are beyond the scope of the current-state-of-the-art in temporal networks. Specifically, current approaches to temporal networks do not account for the complex dynamics of individual physiological systems (network nodes) and for the heterogeneity of physiological networks comprised of diverse systems where coupling forms (individual network links) vary in time. Moreover, the current formalism employed in temporal networks requires a well-defined time-scale, which is not adequate for physiologic networks where scale-invariant dynamics and temporal feedbacks over a broad range of time scales are well-known hallmarks of integrated physiological systems. Currently, there is no established analytic instrumentarium and theoretical framework suitable to probe networks comprised of diverse systems with different output dynamics, operating on different time scales, and to quantify dynamic networks of organ interactions from continuous streams of noisy and transient signals.
Further, despite the increased need for smart healthcare sensing systems that monitor patients’ body balance, currently there is no coherent theory that facilitates the modeling of human physiological processes and the design and optimization of future healthcare cyber-physical systems (Bogdan and Marculescu, 2011; Xue and Bogdan, 2017; Bogdan, 2019). Utilizing new generation machine learning and AI algorithms, healthcare cyber-physical systems are expected to measure and mine the patient’s physiological state based on available continuous sensing, quantify risk indices corresponding to the onset of abnormality, signal the need for critical medical intervention in real-time by communicating patient’s medical information via a network from an individual to the hospital, and most importantly control (actuate) a network of vital health signals (e.g., cardiac pacing, insulin level, blood pressure) within personalized homeostasis.
It is also important to note current limitations, when one explores uncharted territory through the perspectives of Network Physiology. The progress towards a reliable network-based approach to disease is still limited by the incompleteness of the available data on protein–protein interactions, metabolic networks, information of biological regulatory pathways and organ interactions that are heavily relying on large scale biomedical experiments and streams of continuous physiological signals (Barabási et al., 2011; Cohen et al., 2021). Meanwhile, as research moves towards the dynamic interactome (Przytycka et al., 2010), it would certainly require new advances in temporal and adaptive networks to probe temporal variations in network topology and function. Network Physiology is still at an early stage (network building phase), where broad-scale empirical investigations are needed to establish a general framework to identify and define dynamical links among physiological systems, and to construct the specific physiological networks that dictate particular integrative functions. Since physiological systems communicate via complex mechanisms manifested through various functional forms of coupling, there is a need to integrate distinct forms of pair-wise physiologic interactions into a general framework that unites approaches from nonlinear dynamics, information theory and machine learning. Empirical investigations in Network Physiology will foster new development of data-driven modeling and theoretical approaches to provide mechanistic insights and elucidate principles of nonlinear control in physiological networks. This in turn will stimulate the development of new data-science methodology with broad impact on both basic biomedical research and clinical practice.
Current Progress
To address these challenges, recent nonlinear methods based on phase synchronization (Schäfer et al., 1998; Song et al., 1998; Pikovsky et al., 2001; Chen et al., 2006; Bartsch et al., 2012; Bartsch and Ivanov, 2014), coherence (Chorlian et al., 2009; McCraty et al., 2009; Bian et al., 2014; Kralemann et al., 2014; Piper et al., 2014; Kerkman et al., 2020), complex wavelets (La Rocca et al., 2021), mutual information (Faes et al., 2014; Valente et al., 2018; Antonacci et al., 2020), transfer entropy (Dumont et al., 2004; Faes et al., 2015; Valenza et al., 2016a; Valenza et al., 2016b; Lucchini et al., 2020) and Granger causality (Stramaglia et al., 2014) have been proposed to infer nonlinear interactions between pairs of dynamical systems. Efforts have focused on extending these methods to quantify direct or indirect interactions, the strength and directionality of links and the functional forms of coupling in physiological networks. A novel concept of time delay stability was introduced to extract information from synchronous bursting activity across systems, and to identify and quantify transient physiologic interactions among diverse organ systems with distinct output dynamics (Bashan et al., 2012; Liu et al., 2015b). In recent years, multi-disciplinary research efforts have made significant contributions that led to discoveries with potential for broad clinical applications, including: novel applications of complex networks theory to ask fundamentally new questions in systems biology; human disease and co-morbidity networks; new physics of synchronization phenomena in networks of oscillators; new insights in neural networks and brain structural and functional connectivity; innovative methods to probe complexity in physiological time series of individual systems and the impact of individual systems on the dynamics of the entire physiologic network; dynamical networks of organ systems and functional forms of coupling; and clinical applications derived from networks of physiological interactions.
Novel computational tools and analytic formalism recently developed in the field of Network Physiology have added new dimensions to our understanding of physiologic states and functions. The network physiology perspective has redefined physiologic states from point of view of dynamic networks of organ interactions. Utilizing this new perspective, recent studies have focused on 1) investigating brain-brain network interactions across distinct brain rhythms and locations, and their relation to new aspects of neural plasticity in response to changes in physiologic state; 2) characterizing dynamical features of brain-organ communications as a new signature of neuro-autonomic control; 3) establishing basic principles underlying coordinated organ-organ communications, and 4) constructing first dynamic maps of physiological systems and organ interactions across distinct physiologic states (Bashan et al., 2012; Bartsch et al., 2012; Bartsch and Ivanov, 2014; Ivanov and Bartsch, 2014; Liu et al., 2015a; Liu et al., 2015b; Bartsch et al., 2015; Lin et al., 2016; Ivanov et al., 2017, 2021b; Dvir et al., 2018; dos Santos Lima et al., 2019; Lin et al., 2020; Rizzo et al., 2020; Ivanov et al., 2021a; Balagué et al., 2020). Pioneering investigations have made first insights into structural and functional connectivity of physiologic networks underlying individual organ systems and their sub-systems (Tass et al., 1998; Bullmore and Sporns, 2009; Gallos et al., 2012; Liu et al., 2015a; Neufang and Akhrif, 2020; Cohen et al., 2021; Cook et al., 2021), and how global behaviors at the organism level, different physiologic states and functions arise out of networked interactions among organ systems to generate health or disease (Bashan et al., 2012; Ivanov and Bartsch, 2014; Karavaev et al., 2020; Pernice et al., 2020; Tecchio et al., 2020; Wood et al., 2020; Zavala et al., 2020; Angelova et al., 2021; Guillet et al., 2021; Morales et al., 2021; Mukli et al., 2021). This has led to identifying first associations of distinct physiologic states and conditions with specific network topology and temporal characteristics of organ interactions (Bashan et al., 2012; Ivanov and Bartsch, 2014). It was discovered that brain-organ interactions have preferred channels of communication (frequency bands) that are specific for each organ (Bartsch et al., 2015). Recent studies that focused on networks of brain–heart interactions identified new aspects of coupling and neuro-autonomic feedback mechanisms (Valenza et al., 2016b; Lin et al., 2016). By developing the theoretical framework necessary to uncover basic principles of 1) integration among diverse physiologic systems that leads to complex physiologic functions at the organism level, and of 2) hierarchical reorganization of physiological networks and their evolution across states and conditions, investigations in the field of Network Physiology provide first building blocks of an atlas of dynamic interactions among physiological systems in the human body and lay the foundation of the Human Physiolome.
Impacts and Future Development
The unique fundamental questions we address in Network Physiology will change the current paradigm of defining physiologic states, health and disease by shifting the focus from single organs to the network of physiologic interactions. Investigations in the field will help unravel the mystery of how health emerges as a result of network interactions among systems. Coordinated interdisciplinary research efforts in the field will establish basic principles of organ integration essential to generate emergent behaviors at the organism level, and to facilitate responses and adaptation to internal and external perturbations, and thus, will redefine physiological states and functions in health and disease through unique network maps of physiologic interactions.
Novel mathematical and computational methods will be developed to address the complexity of physiological systems, to facilitate empirical findings of physiological interactions, and to build the first theoretical framework for investigations of emerging global behaviors in networks of dynamical systems. This will directly impact areas of applied math, computer and data science, and network theory as 1) we develop new techniques for physiological data analyses, and 2) introduce new generation network models of dynamical systems with time-dependent interactions to uncover mechanisms of hierarchical integration, global network evolution across states and re-organization between distinct network modules, motifs, and communities of integrated physiological systems and sub-systems.
Future developments in Network Physiology will revolutionize our knowledge and understanding of the mechanisms that regulate and coordinate organ-to-organ interactions; establish first quantitative measures of the interactions between diverse organ systems and of their collective network behavior; uncover relations between physiologic states and patterns of organ network interactions; establish the hierarchical structure of physiological networks, the mechanism of network control and re-organization with states, conditions and disease; and thereby open entirely new areas of research at the interface of computational and data science, applied mathematics and physics, AI and bioengineering, physiology and medicine.
A number of potential basic science and medical innovations could follow (Figure 5): Novel methods tailored to infer coupling, causality and directionality of interactions among nonlinear systems with time-varying dynamics; New generation of AI and machine learning algorithms trained to simultaneously respond to both spatial and temporal features of dynamic networks; New class of data-driven network models to study mechanisms of emergent global network behaviors and phase transitions; Novel biomarkers based on organ network interactions for early diagnosis, age-related risk assessment and pathological conditions; Next-generation ICU monitoring/alert systems that incorporates maps of organ network interactions and AI algorithms to track real-time changes of states and conditions; New, comprehensive ways to assess the effects of medical treatment strategies and drugs, not just on a targeted organ system but on the coupling between organs; New network-based taxonomy of disease and co-morbidity networks; New kind of Big Data, the Human Physiolome (Figure 4), consisting of continuous long-term synchronous recordings from multiple physiological systems and the corresponding blueprint reference network maps (representing physiologic interactions across temporal and spatial scales from the sub-cellular to the organism level) that are associated with basic physiological states (wake and sleep, sleep stages, rest and exercise, stress and anxiety, cognition etc.), conditions (age groups, gender, race etc.), and disease (neurodegenerative and metabolic disorders, sleep and circadian disorders, cancer, diabetes and obesity, concussion and brain trauma, comma, cardiac arrest, sepsis, multiple organ failure etc.); New level of real-time personalized health monitoring; Establish the mathematical foundation and theoretical framework of the new interdisciplinary field of Network Physiology. Further, the uncovered laws of communication between organ systems, basic principles of integration in physiological networks and mechanisms of network control that lead to emergence of global network behaviors at the organism level will open new avenues of research and applications in the fields of bio-engineering, electronics and robotics, where next generation intelligent electronic and robotic systems, as well as swarms of bots, will implement algorithms derived from principles of interactions and network organization among physiological systems to execute versatile and complex tasks.
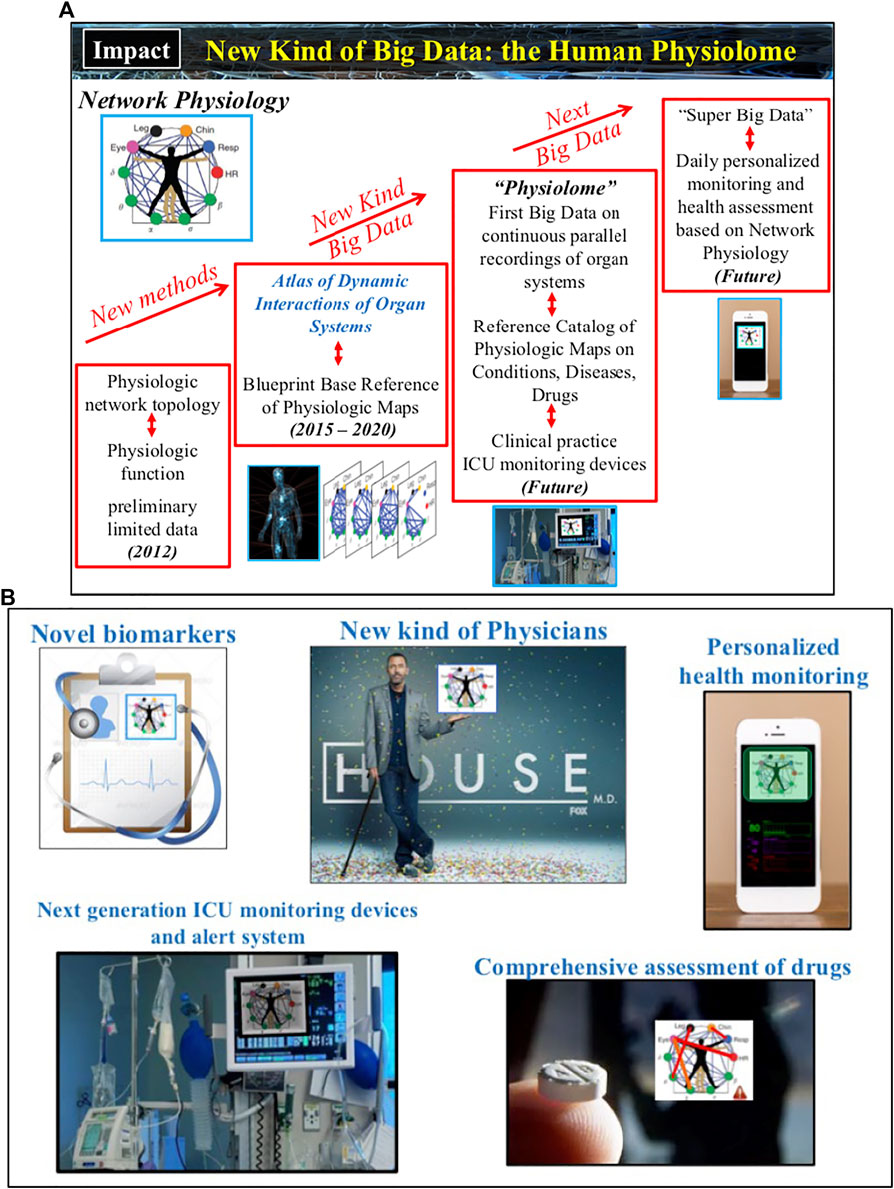
FIGURE 5. Developments in Network Physiology will revolutionize our knowledge and understanding of the principles underlying systems’ communications and their integration as a network, and the mechanisms that coordinate and control organ-to-organ interactions. (A) Current technological advances and findings of association between physiologic networks structure and dynamics with physiological function open the horizon to develop a new kind of Big Data and build the Human Physiolome — a dynamic atlas of network maps representing physiologic interactions across levels and systems in the human body under health and disease. (B) A broad range of applications will follow: novel network-based biomarkers and taxonomy of disease; next generation integrated biomedical devices and sensor networks to facilitate prediction of critical events and guide treatment strategies; comprehensive assessment of drugs effects not only on individual systems but also on the interactions among systems; personalized health monitoring; new educational and training tools for physicians and clinicians.
Multi-Disciplinary Community and a New Journal
The fundamental questions and challenges in the field of Network Physiology have drawn attention and have generated interest in a diverse community of research scientists across a broad range of disciplines and fields from applied mathematics, physics, data science and biomedical engineering to neuroscience, physiology and clinical medicine. Several collections of articles with focus on Network Physiology published in leading interdisciplinary journals, including New Journal of Physics (Ivanov, 2016), Physiological Measurement (Ivanov, 2017) and Frontiers in Physiology (Ivanov et al., 2019), have facilitated nucleation of ideas, identifying key problems, exchange of concepts and methodology, and helped outline new frontiers of synergetic research in the field. Conferences hosted by the International Summer Institute on Network Physiology at the Lake Como School for Advanced Studies (ISINP, 2017; ISINP, 2019) have served as a forum to present basic research and clinical studies, discuss challenges and future developments, train the next generation of young scientists, and foster collaborations among groups and institutions across countries, thus, establishing a world community working in this newly emerging field.
In April 2021, Frontiers, a leading open access publisher and open science platform, has launched Frontiers in Network Physiology, the first journal publishing rigorously peer-reviewed research and dedicated to furthering our understanding of network physiology. This multidisciplinary, open-access journal is at the forefront of communicating impactful scientific discoveries to academics and clinicians. The journal provides a platform for articles covering a range of physiological systems from the metabolic, sub-cellular and cellular level to integrated organ systems and the entire organism, and will publish cutting-edge empirical and theoretical works, discuss the challenges, current frontiers and future developments in the field of Network Physiology.
Frontiers in Network Physiology welcomes both basic research and clinical studies, and aims to promote data-driven discoveries of laws and control mechanisms that underlie physiologic network interactions under both health and pathological conditions. Of particular interest will be new approaches to identify and quantify forms of physiologic coupling as well as developing new and little-explored areas of network science of relevance to integrated physiological systems. The journal will also foster the development of research on next generation network-based diagnostic/prognostic markers and treatment strategies, as well as the development of new integrated biomedical engineering device platforms and sensory networks for multi-systems data recording and analysis. The scope of the journal encompasses a broad range of topics, including: Functional forms of physiologic coupling, time variation and effects of pair-wise interactions on the dynamics and control of individual systems; Network studies on structural and dynamical aspects of physiological sub-systems and systems that transcend space and time scales; Information flow on network topology in relation to cellular and neuronal assemblies and autonomic control of organ systems; Networks comprised of diverse physiological systems and associations between physiologic network structure and physiologic function; Basic principles of hierarchical network organization; Evolution of pair-wise coupling and network topology with transitions across physiologic states; Role of time-dependent network interactions for emergent transitions in network topology and function; Networks of physiological networks transcending interactions of sub-systems to interactions among organs; Manipulation, control and global dynamics of networks in response to clinical treatment; Cascades of failure across systems as encountered in critical care; Development of physiologically inspired AI algorithms, electronic and robotic systems based on the laws and principles of physiologic network interactions.
The conceptual framework of Network Physiology and the integrative approaches this new field offers to explore emerging cooperative phenomena and critical states in networks of diverse dynamical systems with nonlinear and time-varying interactions open new exciting horizons in both basic and applied sciences with broad impact on data science, biomedical technology and clinical practice. Advances in Network Physiology will revolutionize our understanding of health and disease. We invite the community to join this new multi-disciplinary science, and take part in an exciting journey of new discoveries and applications to build the Human Physiolome.
Author Contributions
The author confirms being the sole contributor of this work and has approved it for publication.
Funding
This work was supported by the W. M. Keck Foundation (http://www.wmkeck.org).
Conflict of Interest
The author declares that the research was conducted in the absence of any commercial or financial relationships that could be construed as a potential conflict of interest.
Acknowledgments
To all colleagues and friends for their support, inspiration and contributions to the field of Network Physiology.
References
Amaral, L. A. N., Goldberger, A. L., Ivanov, P. C., and Stanley, H. E. (1998). Scale-independent Measures and Pathologic Cardiac Dynamics. Phys. Rev. Lett. 81, 2388–2391. doi:10.1103/PhysRevLett.81.2388
Angelone, A., and Coulter, N. A. (1964). Respiratory Sinus Arrhythmia: a Frequency Dependent Phenomenon. J. Appl. Physiol. 19, 479–482. doi:10.1152/jappl.1964.19.3.479
Angelova, M., Holloway, P. M., Shelyag, S., Rajasegarar, S., and Rauch, H. (2021). Effect of Stress on Cardiorespiratory Synchronization of Ironman Athletes. Front. Physiol. 12, 189. doi:10.3389/fphys.2021.612245
Antonacci, Y., Astolfi, L., Nollo, G., and Faes, L. (2020). Information Transfer in Linear Multivariate Processes Assessed through Penalized Regression Techniques: Validation and Application to Physiological Networks. Entropy 22, 732. doi:10.3390/e22070732
Ashkenazy, Y., Hausdorff, J. M., Ivanov, P. C., and Stanley, H. E. (2002). A Stochastic Model of Human Gait Dynamics. Physica A: Stat. Mech. its Appl. 316, 662–670. doi:10.1016/s0378-4371(02)01453-x
Balagué, N., Hristovski, R., Almarcha, M., Garcia-Retortillo, S., and Ivanov, P. Ch. (2020). Network Physiology of Exercise: Vision and Perspectives. Front. Physiol 11, 611550.
Baraba´si, A.-L., Gulbahce, N., and Loscalzo, J. (2011). Network Medicine: a Network-Based Approach to Human Disease. Nat. Rev. Genet. 12, 56–68. doi:10.1038/nrg2918
Barajas-Martínez, A., Easton, J. F., Rivera, A. L., Martínez-Tapia, R., de la Cruz, L., Robles-Cabrera, A., et al. (2020). Metabolic Physiological Networks: The Impact of Age. Front. Physiol. 11, 1270. doi:10.3389/fphys.2020.587994
Barajas-Martínez, A., Ibarra-Coronado, E., Sierra-Vargas, M. P., Cruz-Bautista, I., Almeda-Valdes, P., Aguilar-Salinas, C. A., et al. (2021). Physiological Network from Anthropometric and Blood Test Biomarkers. Front. Physiol. 11, 1791.
Bartsch, R. P., and Ivanov, P. C. (2014). “Coexisting Forms of Coupling and Phase-Transitions in Physiological Networks,” in Nonlinear Dynamics of Electronic Systems. Editors V. M. Mladenov, and P. C. Ivanov. Communications in Computer and Information Science, 438, pp. 270–287. (Springer International Publishing). doi:10.1007/978-3-319-08672-9_33
Bartsch, R. P., Liu, K. K. L., Bashan, A., and Ivanov, P. C. (2015). Network Physiology: How Organ Systems Dynamically Interact. PloS one 10, e0142143. doi:10.1371/journal.pone.0142143
Bartsch, R. P., Liu, K. K., Ma, Q. D., and Ivanov, P. C. (2014). “Three Independent Forms of Cardio- Respiratory Coupling: Transitions across Sleep Stages,” in Computing in Cardiology 2014 (IEEE), 781–784.
Bartsch, R. P., Schumann, A. Y., Kantelhardt, J. W., Penzel, T., and Ivanov, P. C. (2012). Phase Transitions in Physiologic Coupling. Proc. Natl. Acad. Sci. 109, 10181–10186. doi:10.1073/pnas.1204568109
Bashan, A., Bartsch, R. P., Kantelhardt, J. W., Havlin, S., and Ivanov, P. C. (2012). Network Physiology Reveals Relations between Network Topology and Physiological Function. Nat. Commun. 3, 702. doi:10.1038/ncomms1705
Bian, Z., Li, Q., Wang, L., Lu, C., Yin, S., and Li, X. (2014). Relative Power and Coherence of Eeg Series Are Related to Amnestic Mild Cognitive Impairment in Diabetes. Front. Aging Neurosci. 6, 11. doi:10.3389/fnagi.2014.00011
Bogdan, P., and Marculescu, R. (2011). “Towards a Science of Cyber-Physical Systems Design,” in 2011 IEEE/ACM Second International Conference on Cyber-Physical Systems (IEEE), 99–108.
Bogdan, P. (2019). Taming the Unknown Unknowns in Complex Systems: Challenges and Opportunities for Modeling, Analysis and Control of Complex (Biological) Collectives. Front. Physiol. 10, 1452. doi:10.3389/fphys.2019.01452
Bolton, T. A. W., Wotruba, D., Buechler, R., Theodoridou, A., Michels, L., Kollias, S., et al. (2020). Triple Network Model Dynamically Revisited: Lower Salience Network State Switching in Pre-psychosis. Front. Physiol. 11, 66. doi:10.3389/fphys.2020.00066
Buchman, T. G. (2006). “Physiologic Failure: Multiple Organ Dysfunction Syndrome,” in Complex Systems Science in BioMedicine (Springer), 631–640.
Bullmore, E., and Sporns, O. (2009). Complex Brain Networks: Graph Theoretical Analysis of Structural and Functional Systems. Nat. Rev. Neurosci. 10, 186–198. doi:10.1038/nrn2575
Bunde, A., Havlin, S., Kantelhardt, J. W., Penzel, T., Peter, J.-H., and Voigt, K. (2000). Correlated and Uncorrelated Regions in Heart-Rate Fluctuations during Sleep. Phys. Rev. Lett. 85, 3736–3739. doi:10.1103/physrevlett.85.3736
Castelluzzo, M., Perinelli, A., Tabarelli, D., and Ricci, L. (2020). Dependence of Connectivity on the Logarithm of Geometric Distance in Brain Networks. Front. Physiol. 11, 1868. doi:10.3389/fphys.2020.611125
Chen, Z., Hu, K., Stanley, H. E., Novak, V., and Ivanov, P. C. (2006). Cross-correlation of Instantaneous Phase Increments in Pressure-Flow Fluctuations: Applications to Cerebral Autoregulation. Phys. Rev. E Stat. Nonlin. Soft Matter Phys. 73, 031915. doi:10.1103/PhysRevE.73.031915
Chorlian, D. B., Rangaswamy, M., and Porjesz, B. (2009). Eeg Coherence: Topography and Frequency Structure. Exp. Brain Res. 198, 59–83. doi:10.1007/s00221-009-1936-9
Cohen, A. A., Leblanc, S., and Roucou, X. (2021). Robust Physiological Metrics from Sparsely Sampled Networks. Front. Physiol. 12, 60. doi:10.3389/fphys.2021.624097
Coleman, T. G., and Randall, J. E. (1983). HUMAN. A Comprehensive Physiological Model. Physiologist 26, 15–21.
Cook, A. J., Pfeifer, K. J., and Tass, P. A. (2021). A Single Case Feasibility Study of Sensorimotor Rhythm Neurofeedback in Parkinson’s Disease. Front. Neurosci. 15, 65. doi:10.3389/fnins.2021.623317
Corkey, B. E., and Deeney, J. T. (2020). The Redox Communication Network as a Regulator of Metabolism. Front. Physiol. 11, 567796. doi:10.3389/fphys.2020.567796
de Arcangelis, L., Perrone-Capano, C., and Herrmann, H. J. (2006). Self-organized Criticality Model for Brain Plasticity. Phys. Rev. Lett. 96, 028107. doi:10.1103/PhysRevLett.96.028107
Delussi, M., Nazzaro, V., Ricci, K., and de Tommaso, M. (2020). Eeg Functional Connectivity and Cognitive Variables in Premanifest and Manifest huntington’s Disease: Eeg Low-Resolution Brain Electromagnetic Tomography (Loreta) Study. Front. Physiol. 11, 1655. doi:10.3389/fphys.2020.612325
dos Santos Lima, G. Z., Lobao-Soares, B., Corso, G., Belchior, H., Lopes, S. R., de Lima Prado, T., et al. (2019). Hippocampal and Cortical Communication Around Micro-arousals in Slow-Wave Sleep. Sci. Rep. 9, 5876. doi:10.1038/s41598-019-42100-5
Dumont, M., Jurysta, F., Lanquart, J.-P., Migeotte, P.-F., Van De Borne, P., and Linkowski, P. (2004). Interdependency between Heart Rate Variability and Sleep Eeg: Linear/non-Linear? Clin. Neurophysiol. 115, 2031–2040. doi:10.1016/j.clinph.2004.04.007
Dvir, H., Elbaz, I., Havlin, S., Appelbaum, L., Ivanov, P. C., and Bartsch, R. P. (2018). Neuronal Noise as an Origin of Sleep Arousals and its Role in Sudden Infant Death Syndrome. Sci. Adv. 4, eaar6277. doi:10.1126/sciadv.aar6277
Faes, L., Marinazzo, D., Jurysta, F., and Nollo, G. (2015). Linear and Non-linear Brain-Heart and Brain-Brain Interactions during Sleep. Physiol. Meas. 36, 683–698. doi:10.1088/0967-3334/36/4/683
Faes, L., Nollo, G., Jurysta, F., and Marinazzo, D. (2014). Information Dynamics of Brain-Heart Physiological Networks during Sleep. New J. Phys. 16, 105005. doi:10.1088/1367-2630/16/10/105005
Ferrari, F. A. S., Viana, R. L., Gomez, F., Lorimer, T., and Stoop, R. (2015). Macroscopic Bursting in Physiological Networks: Node or Network Property? New J. Phys. 17, 055024. doi:10.1088/1367-2630/17/5/055024
Fesce, R. (2020). Subjectivity as an Emergent Property of Information Processing by Neuronal Networks. Front. Neurosci. 14, 995. doi:10.3389/fnins.2020.548071
Finazzi, S., Mandelli, G., Garbero, E., Mondini, M., Trussardi, G., Giardino, M., et al. (2018). Data Collection and Research with Margheritatre. Physiol. Meas. 39, 084004. doi:10.1088/1361-6579/aad10f
Foreman, B., Lissak, I. A., Kamireddi, N., Moberg, D., and Rosenthal, E. S. (2021). Challenges and Opportunities in Multimodal Monitoring and Data Analytics in Traumatic Brain Injury. Curr. Neurol. Neurosci. Rep. 21, 1–9. doi:10.1007/s11910-021-01098-y
Fossion, R., Rivera, A. L., and Estañol, B. (2018). A Physicist's View of Homeostasis: How Time Series of Continuous Monitoring Reflect the Function of Physiological Variables in Regulatory Mechanisms. Physiol. Meas. 39, 084007. doi:10.1088/1361-6579/aad8db
Gallos, L. K., Makse, H. A., and Sigman, M. (2012). A Small World of Weak Ties Provides Optimal Global Integration of Self-Similar Modules in Functional Brain Networks. Proc. Natl. Acad. Sci. 109, 2825–2830. doi:10.1073/pnas.1106612109
Gao, X., Petricoin, E. F., Ward, K. R., Goldberg, S. R., Duane, T. M., Bonchev, D., et al. (2018). Network Proteomics of Human Dermal Wound Healing. Physiol. Meas. 39, 124002. doi:10.1088/1361-6579/aaee19
Garcia-Retortillo, S., Javierre, C., Hristovski, R., Ventura, J. L., and Balagué, N. (2019). Principal Component Analysis as a Novel Approach for Cardiorespiratory Exercise Testing Evaluation. Physiol. Meas. 40, 084002. doi:10.1088/1361-6579/ab2ca0
Goldberger, A. L., Amaral, L. A. N., Hausdorff, J. M., Ivanov, P. C., Peng, C.-K., and Stanley, H. E. (2002). Fractal Dynamics in Physiology: Alterations with Disease and Aging. Proc. Natl. Acad. Sci. 99, 2466–2472. doi:10.1073/pnas.012579499
Gómez-Gardenes, J., Gómez, S., Arenas, A., and Moreno, Y. (2011). Explosive Synchronization Transitions in Scale-free Networks. Phys. Rev. Lett. 106, 128701. doi:10.1103/PhysRevLett.106.128701
Guillet, A., Arneodo, A., and Argoul, F. (2021). Tracking Rhythms Coherence from Polysomnographic Records: a Time-Frequency Approach. Front. Appl. Math. Stat. 7, 17. doi:10.3389/fams.2021.624456
Guyton, A. C., Coleman, T. G., and Granger, H. J. (1972). Circulation: Overall Regulation. Annu. Rev. Physiol. 34, 13–44. doi:10.1146/annurev.ph.34.030172.000305
Haug, N., Sorger, J., Gisinger, T., Gyimesi, M., Kautzky-Willer, A., Thurner, S., et al. (2021). Decompression of Multimorbidity along the Disease Trajectories of Diabetes Mellitus Patients. Front. Physiol. 11, 1726. doi:10.3389/fphys.2020.612604
Hausdorff, J. M., Ashkenazy, Y., Peng, C.-K., Ivanov, P. C., Stanley, H. E., and Goldberger, A. L. (2001). When Human Walking Becomes Random Walking: Fractal Analysis and Modeling of Gait Rhythm Fluctuations. Physica A: Stat. Mech. its Appl. 302, 138–147. doi:10.1016/s0378-4371(01)00460-5
Holme, P., and Saramäki, J. (2012). Temporal Networks. Phys. Rep. 519, 97–125. doi:10.1016/j.physrep.2012.03.001
Hopkins, A. L. (2008). Network Pharmacology: the Next Paradigm in Drug Discovery. Nat. Chem. Biol. 4, 682–690. doi:10.1038/nchembio.118
Hu, K., Ivanov, P. C., Chen, Z., Hilton, M. F., Stanley, H. E., and Shea, S. A. (2004a). Non-random Fluctuations and Multi-Scale Dynamics Regulation of Human Activity. Physica A: Stat. Mech. its Appl. 337, 307–318. doi:10.1016/j.physa.2004.01.042
Hu, K., Ivanov, P. C., Hilton, M. F., Chen, Z., Ayers, R. T., Stanley, H. E., et al. (2004b). Endogenous Circadian Rhythm in an index of Cardiac Vulnerability Independent of Changes in Behavior. Proc. Natl. Acad. Sci. 101, 18223–18227. doi:10.1073/pnas.0408243101
ISINP (2017). First International Summer institute on Network Physiology. https://isinp.lakecomoschool.org.
ISINP (2019). Second International Summer institute on Network Physiology. https://isinp-2.lakecomoschool.org.
Ivanov, P. C., Amaral, L. A. N., Goldberger, A. L., Havlin, S., Rosenblum, M. G., Struzik, Z. R., et al. (1999a). Multifractality in Human Heartbeat Dynamics. Nature 399, 461–465. doi:10.1038/20924
Ivanov, P. C., Amaral, L. A. N., Goldberger, A. L., and Stanley, H. E. (1998). Stochastic Feedback and the Regulation of Biological Rhythms. Europhys. Lett. 43, 363–368. doi:10.1209/epl/i1998-00366-3
Ivanov, P. C., and Bartsch, R. P. (2014). “Network Physiology: Mapping Interactions between Networks of Physiologic Networks,” in Networks of Networks: The Last Frontier of Complexity. Editors G. D. Agostino, and A. Scala (Cham: Springer International Publishing), 203–222. doi:10.1007/978-3-319-03518-5_10
Ivanov, P. C., Bunde, A., Amaral, L. A. N., Havlin, S., Fritsch-Yelle, J., Baevsky, R. M., et al. (1999b). Sleep-wake Differences in Scaling Behavior of the Human Heartbeat: Analysis of Terrestrial and Long-Term Space Flight Data. Europhys. Lett. 48, 594–600. doi:10.1209/epl/i1999-00525-0
Ivanov, P. C., Chen, Z., Hu, K., and Stanley, H. E. (2004). Multiscale Aspects of Cardiac Control. Physica A: Stat. Mech. its Appl. 344, 685–704. doi:10.1016/j.physa.2004.08.016
Ivanov, P. C., Eke, A., and Sosnovtseva, O. (2019). The New Frontier of Network Physiology: From Temporal Dynamics to the Synchronization and Principles of Integration in Networks of Physiological Systems. Lausanne, Switzerland: Frontiers in Physiology. https://www.frontiersin.org/research-topics/10298.
Ivanov, P. C., Liu, K. K. L., and Bartsch, R. P. (2016). Focus on the Emerging New fields of Network Physiology and Network Medicine. New J. Phys. 18, 100201. doi:10.1088/1367-2630/18/10/100201 https://iopscience.iop.org/journal/1367-2630/page/Focus%20on% 20Network%20Physiology%20and%20Network%20Medicine
Ivanov, P. C., Liu, K. K. L., Lin, A., and Bartsch, R. P. (2017). “Network Physiology: From Neural Plasticity to Organ Network Interactions,” in Emergent Complexity from Nonlinearity, in Physics, Engineering and the Life Sciences. Editors G. Mantica, R. Stoop, and S. Stramaglia (Cham: Springer International Publishing), 145–165. doi:10.1007/978-3-319-47810-4_12
Ivanov, P. C., Nunes Amaral, L. A., Goldberger, A. L., Havlin, S., Rosenblum, M. G., Stanley, H. E., et al. (2001). From 1/f Noise to Multifractal Cascades in Heartbeat Dynamics. Chaos 11, 641–652. doi:10.1063/1.1395631
Ivanov, P. C., Rosenblum, M. G., Peng, C.-K., Mietus, J., Havlin, S., Stanley, H. E., et al. (1996). Scaling Behaviour of Heartbeat Intervals Obtained by Wavelet-Based Time-Series Analysis. Nature 383, 323–327. doi:10.1038/383323a0
Ivanov, P. C. (2017). The New Field of Network Physiology: Redefining Health and Disease through Networks of Physiological Interactions. Bristol, United Kingdom: Physiological Measurement. https://iopscience.iop.org/journal/0967-3334/page/.
Ivanov, P. C., Wang, J. W. J. L., Zhang, X., and Chen, B. (2021b). “The New Frontier of Network Physiology: Emerging Physiologic States in Health and Disease from Integrated Organ Network Interactions,” in 2019-20 MATRIX Annals. Editors D. R. Wood, J. de Gier, C. E. Praeger, and T. Tao (Springer International Publishing), 237–254. doi:10.1007/978-3-030-62497-2_12
Ivanov, P. C., Wang, J. W., and Zhang, X. (2021a). “Signal Processing in Network Physiology: Quantifying Network Dynamics of Organ Interactions,” in 2020 28th European Signal Processing Conference (EUSIPCO) (IEEE), 945–949.
Jiang, Y., Costello, J. T., Williams, T. B., Panyapiean, N., Bhogal, A. S., Tipton, M. J., et al. (2021). A Network Physiology Approach to Oxygen Saturation Variability during Normobaric Hypoxia. Exp. Physiol. 106, 151–159. doi:10.1113/ep088755
Kantelhardt, J. W., Ashkenazy, Y., Ivanov, P. C., Bunde, A., Havlin, S., Penzel, T., et al. (2002). Characterization of Sleep Stages by Correlations in the Magnitude and Sign of Heartbeat Increments. Phys. Rev. E Stat. Nonlin. Soft Matter Phys. 65, 051908. doi:10.1103/PhysRevE.65.051908
Karasik, R., Sapir, N., Ashkenazy, Y., Ivanov, P. C., Dvir, I., Lavie, P., et al. (2002). Correlation Differences in Heartbeat Fluctuations during Rest and Exercise. Phys. Rev. E Stat. Nonlin. Soft Matter Phys. 66, 062902. doi:10.1103/PhysRevE.66.062902
Karavaev, A. S., Ishbulatov Yu, Y. M., Prokhorov, M. D., Ponomarenko, V. I., Kiselev, A. R., Runnova, A. E., et al. (2020). Simulating Dynamics of Circulation in the Awake State and Different Stages of Sleep 610 Using Non-autonomous Mathematical Model with Time Delay. Front. Physiol. 11, 1656. doi:10.1142/s1793962320500142
Kerkman, J. N., Bekius, A., Boonstra, T. W., Daffertshofer, A., and Dominici, N. (2020). Muscle Synergies and Coherence Networks Reflect Different Modes of Coordination during Walking. Front. Physiol. 11, 751. doi:10.3389/fphys.2020.00751
Komarov, M., and Pikovsky, A. (2013). Dynamics of Multifrequency Oscillator Communities. Phys. Rev. Lett. 110, 134101. doi:10.1103/physrevlett.110.134101
Kralemann, B., Pikovsky, A., and Rosenblum, M. (2014). Reconstructing Effective Phase Connectivity of Oscillator Networks from Observations. New J. Phys. 16, 085013. doi:10.1088/1367-2630/16/8/085013
La Rocca, D., Wendt, H., van Wassenhove, V., Ciuciu, P., and Abry, P. (2021). Revisiting Functional Connectivity for Infraslow Scale-free Brain Dynamics Using Complex Wavelets. Front. Physiol. 620, 1651.
Large, W. A. (2011). Systems Biology in a Physiological World. J. Physiol. 589, 1001–1239. doi:10.1113/jphysiol.2011.205450
Lavanga, M., Bollen, B., Jansen, K., Ortibus, E., Naulaers, G., Van Huffel, S., et al. (2020). A Bradycardia-Based Stress Calculator for the Neonatal Intensive Care Unit: A Multisystem Approach. Front. Physiol. 11, 741. doi:10.3389/fphys.2020.00741
Lehnertz, K., Bröhl, T., and Rings, T. (2020). The Human Organism as an Integrated Interaction Network: Recent Conceptual and Methodological Challenges. Front. Physiol. 11, 1694. doi:10.3389/fphys.2020.598694
Li, Q., Gao, J., Zhang, Z., Huang, Q., Wu, Y., and Xu, B. (2020). Distinguishing Epileptiform Discharges from Normal Electroencephalograms Using Adaptive Fractal and Network Analysis: A Clinical Perspective. Front. Physiol. 11, 828. doi:10.3389/fphys.2020.00828
Lin, A., Liu, K. K. L., Bartsch, R. P., and Ivanov, P. C. (2020). Dynamic Network Interactions Among Distinct Brain Rhythms as a Hallmark of Physiologic State and Function. Commun. Biol. 3, 197. doi:10.1038/s42003-020-0878-4
Lin, A., Liu, K. K. L., Bartsch, R. P., and Ivanov, P. C. (2016). Delay-correlation Landscape Reveals Characteristic Time Delays of Brain Rhythms and Heart Interactions. Phil. Trans. R. Soc. A. 374, 20150182. doi:10.1098/rsta.2015.0182
Lioi, G., Bell, S. L., Smith, D. C., and Simpson, D. M. (2017). Directional Connectivity in the Eeg Is Able to Discriminate Wakefulness from Nrem Sleep. Physiol. Meas. 38, 1802–1820. doi:10.1088/1361-6579/aa81b5
Liu, K. K. L., Bartsch, R. P., Lin, A., Mantegna, R. N., and Ivanov, P. C. (2015a). Plasticity of Brain Wave Network Interactions and Evolution across Physiologic States. Front. Neural Circuits 9, 62. doi:10.3389/fncir.2015.00062
Liu, K. K. L., Bartsch, R. P., Ma, Q. D. Y., and Ivanov, P. C. (2015b). “Major Component Analysis of Dynamic Networks of Physiologic Organ Interactions,” in Journal of Physics: Conference Series (IOP Publishing), 640, 012013. doi:10.1088/1742-6596/640/1/012013J. Phys. Conf. Ser.
Liu, L., Shao, Z., Lv, J., Xu, F., Ren, S., Jin, Q., et al. (2020). Identification of Early Warning Signals at the Critical Transition Point of Colorectal Cancer Based on Dynamic Network Analysis. Front. Bioeng. Biotechnol. 8, 530. doi:10.3389/fbioe.2020.00530
Liu, M., Legault, V., Fulop, T., Cote, A.-M., Gravel, D., Blanchet, F. G., et al. (2021). Prediction of 646 Mortality in Hemodialysis Patients Using Moving Multivariate Distance. Front. Physiol. 12, 299–647. doi:10.3389/fphys.2021.612494
Lombardi, F., Gómez-Extremera, M., Bernaola-Galván, P., Vetrivelan, R., Saper, C. B., Scammell, T. E., et al. (2020). Critical Dynamics and Coupling in Bursts of Cortical Rhythms Indicate Non-homeostatic Mechanism for Sleep-Stage Transitions and Dual Role of VLPO Neurons in Both Sleep and Wake. J. Neurosci. 40, 171–190. doi:10.1523/jneurosci.1278-19.2019
Loscalzo, J., and Barabasi, A.-L. (2011). Systems Biology and the Future of Medicine. Wires Syst. Biol. Med. 3, 619–627. doi:10.1002/wsbm.144
Lucchini, M., Pini, N., Burtchen, N., Signorini, M. G., and Fifer, W. P. (2020). Transfer Entropy Modeling of Newborn Cardiorespiratory Regulation. Front. Physiol. 11, 1095. doi:10.3389/fphys.2020.01095
Masamura, S., Iwamoto, T., Sugitani, Y., Konishi, K., and Hara, N. (2020). Experimental Investigation of 656 Amplitude Death in Delay-Coupled Double-Scroll Circuits with Randomly Time-Varying Network Topology. Nonlinear Dyn. 657, 1–14. doi:10.1007/s11071-019-05461-0
McCraty, R., Atkinson, M., Tomasino, D., and Bradley, R. T. (2009). The Coherent Heart: Heart-Brain Interactions, Psychophysiological Coherence, and the Emergence of System-wide Order. Integral Review: A Transdisciplinary and Transcultural Journal for New Thought, Research, and Praxis 5 (2).
McNorgan, C., Judson, C., Handzlik, D., and Holden, J. G. (2020). Linking Adhd and Behavioral Assessment through Identification of Shared Diagnostic Task-Based Functional Connections. Front. Physiol. 663, 1595. doi:10.3389/fphys.2020.583005
Millman, D., Mihalas, S., Kirkwood, A., and Niebur, E. (2010). Self-organized Criticality Occurs in Non-conservative Neuronal Networks during ‘up' States. Nat. Phys. 6, 801–805. doi:10.1038/nphys1757
Moorman, J. R., Lake, D. E., and Ivanov, P. C. (2016). Early Detection of Sepsis-A Role for Network Physiology? Crit. Care Med. 44, e312–e313. doi:10.1097/ccm.0000000000001548
Morales, J., Borzée, P., Testelmans, D., Buyse, B., Van Huffel, S., and Varon, C. (2021). Linear and 669 Non-linear Quantification of the Respiratory Sinus Arrhythmia Using Support Vector Machines. Front. 670 Physiol. 12, 58. doi:10.3389/fphys.2021.623781
Mrowka, R., Patzak, A., and Rosenblum, M. (2000). Quantitative Analysis of Cardiorespiratory Synchronization in Infants. Int. J. Bifurcation Chaos 10, 2479–2488. doi:10.1142/s0218127400001754
Mukli, P., Nagy, Z., Racz, F. S., Portoro, I., Hartmann, A., Stylianou, O., et al. (2021). Two-tiered Response of Cardiorespiratory-Cerebrovascular Network to Orthostatic challenge. Front. Physiol. 12, 216. doi:10.3389/fphys.2021.622569
Neufang, S., and Akhrif, A. (2020). Regional Hurst Exponent Reflects Impulsivity-Related Alterations in Fronto-Hippocampal Pathways within the Waiting Impulsivity Network. Front. Physiol. 11, 827–677. doi:10.3389/fphys.2020.00827
Paëske, L., Hinrikus, H., Lass, J., Raik, J., and Bachmann, M. (2020). Negative Correlation between Functional Connectivity and Small-Worldness in the Alpha Frequency Band of a Healthy Brain. Front. Physiol. 11, 910. doi:10.3389/fphys.2020.00910
Pecora, L. M., Sorrentino, F., Hagerstrom, A. M., Murphy, T. E., and Roy, R. (2014). Cluster Synchronization and Isolated Desynchronization in Complex Networks with Symmetries. Nat. Commun. 5, 4079. doi:10.1038/ncomms5079
Pereira-Ferrero, V. H., Lewis, T. G., Ferrero, L. G. P., and Duarte, L. T. (2019). Complex Networks Models and Spectral Decomposition in the Analysis of Swimming Athletes' Performance at Olympic Games. Front. Physiol. 10, 1134. doi:10.3389/fphys.2019.01134
Pernice, R., Antonacci, Y., Zanetti, M., Busacca, A., Marinazzo, D., Faes, L., et al. (2020). Multivariate Correlation Measures Reveal Structure and Strength of Brain-Body Physiological Networks at Rest and during Mental Stress. Front. Neurosci. 14, 1427.
Pikovsky, A., Kurths, J., Rosenblum, M., and Kurths, J. (2001). Synchronization: A Universal Concept in Nonlinear Sciences. Cambridge University Press, 12.
Piper, D., Schiecke, K., Pester, B., Benninger, F., Feucht, M., and Witte, H. (2014). Time-variant Coherence between Heart Rate Variability and EEG Activity in Epileptic Patients: an Advanced Coupling Analysis between Physiological Networks. New J. Phys. 16, 115012. doi:10.1088/1367-2630/16/11/115012
Podobnik, B., Korošak, D., Skelin Klemen, M., Stožer, A., Dolenšek, J., Slak Rupnik, M., et al. (2020). β Cells Operate Collectively to Help Maintain Glucose Homeostasis. Biophysical J. 118, 2588–2595. doi:10.1016/j.bpj.2020.04.005
Polizzi, S., Arneodo, A., Pérez-Reche, F.-J., and Argoul, F. (2021). Emergence of Log-normal Type Distributions in Avalanche Processes in Living Systems: A Network Model. Front. Appl. Math. Stat. 6, 73. doi:10.3389/fams.2020.613962
Porta, A., Bari, V., De Maria, B., and Baumert, M. (2017). A Network Physiology Approach to the Assessment of the Link between Sinoatrial and Ventricular Cardiac Controls. Physiol. Meas. 38, 1472–1489. doi:10.1088/1361-6579/aa6e95
Prats-Puig, A., García-Retortillo, S., Puig-Parnau, M., Vasileva, F., Font-Lladó, R., Xargay-Torrent, S., et al. (2020). DNA Methylation Reorganization of Skeletal Muscle-specific Genes in Response to Gestational Obesity. Front. Physiol. 11, 938. doi:10.3389/fphys.2020.00938
Przytycka, T. M., Singh, M., and Slonim, D. K. (2010). Toward the Dynamic Interactome: It's about Time. Brief. Bioinformatics 11, 15–29. doi:10.1093/bib/bbp057
Rizi, A. K., Zamani, M., Shirazi, A., Jafari, G. R., and Kertész, J. (2021). Stability of Imbalanced Triangles in Gene Regulatory Networks of Cancerous and normal Cells. Front. Physiol. 11, 1792. doi:10.3389/fphys.2020.573732
Rizzo, R., Zhang, X., Wang, J. W. J. L., Lombardi, F., and Ivanov, P. C. (2020). Network Physiology of Cortico–Muscular Interactions. Front. Physiol. 11, 558070. doi:10.3389/fphys.2020.558070
Rosenblum, M. G., Pikovsky, A. S., and Kurths, J. (1996). Phase Synchronization of Chaotic Oscillators. Phys. Rev. Lett. 76, 1804–1807. doi:10.1103/physrevlett.76.1804
Rothkegel, A., and Lehnertz, K. (2014). Irregular Macroscopic Dynamics Due to Chimera States in Small-World Networks of Pulse-Coupled Oscillators. New J. Phys. 16, 055006. doi:10.1088/1367-2630/16/5/055006
Rowland Adams, J., and Stefanovska, A. (2021). Modeling Cell Energy Metabolism as Weighted Networks of Non-autonomous Oscillators. Front. Physiol. 11, 1845. doi:10.3389/fphys.2020.613183
Schäfer, C., Rosenblum, M. G., Kurths, J., and Abel, H. H. (1998). Heartbeat Synchronized with Ventilation. Nature 392, 239–240. doi:10.1038/32567
Schmitt, D. T., Stein, P. K., and Ivanov, P. C. (2009). Stratification Pattern of Static and Scale-Invariant Dynamic Measures of Heartbeat Fluctuations across Sleep Stages in Young and Elderly. IEEE Trans. Biomed. Eng. 56, 1564–1573. doi:10.1109/tbme.2009.2014819
Schumann, A. Y., Bartsch, R. P., Penzel, T., Ivanov, P. C., and Kantelhardt, J. W. (2010). Aging Effects on Cardiac and Respiratory Dynamics in Healthy Subjects across Sleep Stages. Sleep 33, 943–955. doi:10.1093/sleep/33.7.943
Shashikumar, S. P., Li, Q., Clifford, G. D., and Nemati, S. (2017). Multiscale Network Representation of Physiological Time Series for Early Prediction of Sepsis. Physiol. Meas. 38, 2235–2248. doi:10.1088/1361-6579/aa9772
Song, H.-S., and Lehrer, P. M. (2003). The Effects of Specific Respiratory Rates on Heart Rate and Heart Rate Variability. Appl. psychophysiology biofeedback 28, 13–23. doi:10.1023/a:1022312815649
Song, L. Z., Schwartz, G. E., and Russek, L. G. (1998). Heart-focused Attention and Heart-Brain Synchronization: Energetic and Physiological Mechanisms. Altern. Ther. Health Med. 4 (4), 44–62.
Stankovski, T., Ticcinelli, V., McClintock, P. V. E., and Stefanovska, A. (2015). Coupling Functions in Networks of Oscillators. New J. Phys. 17, 035002. doi:10.1088/1367-2630/17/3/035002
Stramaglia, S., M Cortes, J., and Marinazzo, D. (2014). Synergy and Redundancy in the granger Causal Analysis of Dynamical Networks. New J. Phys. 16, 105003. doi:10.1088/1367-2630/16/10/105003
Stramaglia, S., Scagliarini, T., Daniels, B. C., and Marinazzo, D. (2021). Quantifying Dynamical High-Order 736 Interdependencies from the O-Information: an Application to Neural Spiking Dynamics. Front. 737 Physiol. 11, 1784. doi:10.3389/fphys.2020.595736
Suki, B., Alencar, A. M., Frey, U., Ivanov, P. C., Buldyrev, S. V., Majumdar, A., et al. (2003). Fluctuations, Noise and Scaling in the Cardio-Pulmonary System. Fluct. Noise Lett. 03, R1–R25. doi:10.1142/s0219477503001142
Suki, B., Herrmann, J., and Bates, J. H. T. (2020). An Analytic Model of Tissue Self-Healing and its Network Implementation: Application to Fibrosis and Aging. Front. Physiol. 11, 1338. doi:10.3389/fphys.2020.583024
Tan, Y. Y., Montagnese, S., and Mani, A. R. (2020). Organ System Network Disruption Is Associated with Poor Prognosis in Patients with Chronic Liver Failure. Front. Physiol. 11, 983. doi:10.3389/fphys.2020.00983
Tass, P., Rosenblum, M. G., Weule, J., Kurths, J., Pikovsky, A., Volkmann, J., et al. (1998). Detection ofn:mPhase Locking from Noisy Data: Application to Magnetoencephalography. Phys. Rev. Lett. 81, 3291–3294. doi:10.1103/physrevlett.81.3291
Tecchio, F., Bertoli, M., Gianni, E., L’Abbate, T., Paulon, L., and Zappasodi, F. (2020). To Be Is to Become. Fractal Neurodynamics of the Body-Brain Control System. Front. Physiol. 11, 1650. doi:10.3389/fphys.2020.609768
Valente, M., Javorka, M., Porta, A., Bari, V., Krohova, J., Czippelova, B., et al. (2018). Univariate and Multivariate Conditional Entropy Measures for the Characterization of Short-Term Cardiovascular Complexity under Physiological Stress. Physiol. Meas. 39, 014002. doi:10.1088/1361-6579/aa9a91
Valenza, G., Greco, A., Gentili, C., Lanata, A., Sebastiani, L., Menicucci, D., et al. (2016a). Combining Electroencephalographic Activity and Instantaneous Heart Rate for Assessing Brain-Heart Dynamics during Visual Emotional Elicitation in Healthy Subjects. Phil. Trans. R. Soc. A. 374, 20150176. doi:10.1098/rsta.2015.0176
Valenza, G., Toschi, N., and Barbieri, R. (2016b). Uncovering Brain-Heart Information through Advanced Signal and Image Processing. Phil. Trans. R. Soc. A. 374, 20160020. doi:10.1098/rsta.2016.0020
Wang, J. W. J. L., Lombardi, F., Zhang, X., Anaclet, C., and Ivanov, P. C. (2019). Non-equilibrium Critical Dynamics of Bursts in θ and δ Rhythms as Fundamental Characteristic of Sleep and Wake Micro-architecture. Plos Comput. Biol. 15, e1007268. doi:10.1371/journal.pcbi.1007268
Wang, Z., and Liu, Z. (2020). A Brief Review of Chimera State in Empirical Brain Networks. Front. Physiol. 11, 724. doi:10.3389/fphys.2020.00724
Wellman, T. J., Mondoñedo, J. R., Davis, G. S., Bates, J. H. T., and Suki, B. (2018). Topographic Distribution of Idiopathic Pulmonary Fibrosis: a Hybrid Physics- and Agent-Based Model. Physiol. Meas. 39, 064007. doi:10.1088/1361-6579/aaca86
Wood, C., Bianchi, M. T., Yun, C.-H., Shin, C., and Thomas, R. J. (2020). Multicomponent Analysis of 769 Sleep Using Electrocortical, Respiratory, Autonomic and Hemodynamic Signals Reveals Distinct Features of 770 Stable and Unstable Nrem and Rem Sleep. Front. Physiol. 11, 1584. doi:10.3389/fphys.2020.592978
Xu, L., Chen, Z., Hu, K., Stanley, H. E., and Ivanov, P. C. (2006). Spurious Detection of Phase Synchronization in Coupled Nonlinear Oscillators. Phys. Rev. E Stat. Nonlin. Soft Matter Phys. 73, 065201. doi:10.1103/PhysRevE.73.065201
Xue, Y., and Bogdan, P. (2017). “Constructing Compact Causal Mathematical Models for Complex Dynamics,” in Proceedings of the 8th International Conference on Cyber-Physical Systems, 97–107. doi:10.1145/3055004.3055017
Yao, Y., Jiang, C., Wang, F., Yan, H., Long, D., Zhao, J., et al. (2019). Integrative Analysis of Mirna and Mrna Expression Profiles Associated with Human Atrial Aging. Front. Physiol. 10, 1226. doi:10.3389/fphys.2019.01226
Zavala, E., Voliotis, M., Zerenner, T., Tabak, J., Walker, J. J., Li, X. F., et al. (2020). Dynamic Hormone Control of Stress and Fertility. Front. Physiol. 11, 1457. doi:10.3389/fphys.2020.598845
Zhang, X., Boccaletti, S., Guan, S., and Liu, Z. (2015). Explosive Synchronization in Adaptive and Multilayer Networks. Phys. Rev. Lett. 114, 038701. doi:10.1103/PhysRevLett.114.038701
Keywords: network physiology, dynamic networks, complex systems, control, AI, sensory networks, big data, human physiolome
Citation: Ivanov PC (2021) The New Field of Network Physiology: Building the Human Physiolome. Front. Netw. Physiol. 1:711778. doi: 10.3389/fnetp.2021.711778
Received: 19 May 2021; Accepted: 21 May 2021;
Published: 30 June 2021.
Edited and reviewed by:
Francoise Argoul, Centre National de la Recherche Scientifique (CNRS), FranceCopyright © 2021 Ivanov. This is an open-access article distributed under the terms of the Creative Commons Attribution License (CC BY). The use, distribution or reproduction in other forums is permitted, provided the original author(s) and the copyright owner(s) are credited and that the original publication in this journal is cited, in accordance with accepted academic practice. No use, distribution or reproduction is permitted which does not comply with these terms.
*Correspondence: Plamen Ch. Ivanov, cGxhbWVuQGJ1cGh5LmJ1LmVkdQ==