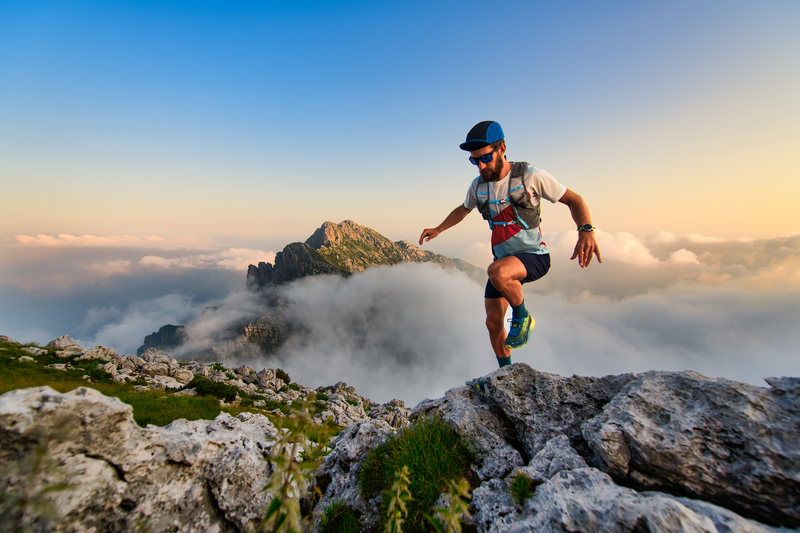
94% of researchers rate our articles as excellent or good
Learn more about the work of our research integrity team to safeguard the quality of each article we publish.
Find out more
ORIGINAL RESEARCH article
Front. Nephrol.
Sec. Blood Purification
Volume 5 - 2025 | doi: 10.3389/fneph.2025.1478367
This article is part of the Research Topic Bioimpedance Analysis: Lifelong Health, Disease, and Sport Applications View all 6 articles
The final, formatted version of the article will be published soon.
You have multiple emails registered with Frontiers:
Please enter your email address:
If you already have an account, please login
You don't have a Frontiers account ? You can register here
Objectives: To investigate the validity of bioelectrical impedance analysis (BIA)-derived phase angle (PhA) as a predictor of malnutrition in maintenance hemodialysis (MHD) patients.Methods: A single-center, cross-sectional study of 126 MHD patients was conducted. A diagnosis of malnutrition was based on the 7-point Subjective Global Assessment (7-p-SGA) criteria. A Bioelectrical Impedance Analyzer was used to determine the PhA, fat mass (FM), muscle mass, and extracellular water/total body water (ECW/TBW) ratio. Biochemical indices and anthropometric measurements were also assessed. Using 7-p-SGA criteria, the patients were categorized into two groups: well-nourished and malnourished. General patient characteristics and the PhA values were compared between the two groups. A correlation analysis examined the relationship between PhA and the nutritional index. Logistic regression models and receiver operating characteristic curve analyses were used to identify independent factors for predicting malnutrition and determining their respective cutoff values. The malnourished group had a significantly lower PhA than the well-nourished group (5.19° (5.81°, 4.09°) vs 6.13° (6.80°, 5.49°), P < 0.001). The PhA correlated positively with body mass index (BMI), albumin (Alb), and handgrip strength (HGS) (P < 0.05). However, there were no significant associations between PhA and FM or triceps skinfold thickness (TSF) (P > 0.05). Multivariate logistic regression analysis revealed that PhA, Alb, and BMI were independent predictors of malnutrition. Of these, BMI was the strongest predictor [odds ratio (OR) = 0.68; P < 0.001]. PhA also served as a secondary predictor of malnutrition (OR = 0.588; P = 0.035). Receiver operating characteristic curve analysis indicated that a PhA threshold value of approximately 5.78° was optimal for predicting malnutrition.PhA is a straightforward and reliable predictor of malnutrition in MHD patients, with an optimal cut-off value of 5.78° identified for diagnosing this condition.
Keywords: maintenance hemodialysis1, malnutrition2, bioelectrical impedance analysis3, Phase Angle4, 7-point-SGA5
Received: 09 Aug 2024; Accepted: 11 Feb 2025.
Copyright: © 2025 Xiao, Xie, Xiang, Cao, Xie, Liang and Su. This is an open-access article distributed under the terms of the Creative Commons Attribution License (CC BY). The use, distribution or reproduction in other forums is permitted, provided the original author(s) or licensor are credited and that the original publication in this journal is cited, in accordance with accepted academic practice. No use, distribution or reproduction is permitted which does not comply with these terms.
* Correspondence:
Qingxuan Xiao, Dongguan Tungwah Hospital, Dongguan, China
Xinyang Xiang, Dongguan Tungwah Hospital, Dongguan, China
Ting Cao, Dongguan Tungwah Hospital, Dongguan, China
Yingye Xie, Dongguan Tungwah Hospital, Dongguan, China
Xiang Liang, Dongguan Tungwah Hospital, Dongguan, China
Xiaoyan Su, Dongguan Tungwah Hospital, Dongguan, China
Disclaimer: All claims expressed in this article are solely those of the authors and do not necessarily represent those of their affiliated organizations, or those of the publisher, the editors and the reviewers. Any product that may be evaluated in this article or claim that may be made by its manufacturer is not guaranteed or endorsed by the publisher.
Research integrity at Frontiers
Learn more about the work of our research integrity team to safeguard the quality of each article we publish.