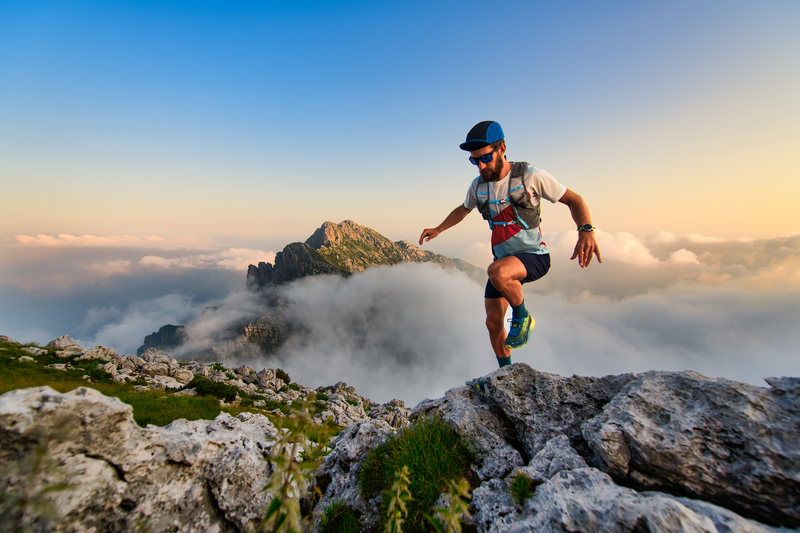
94% of researchers rate our articles as excellent or good
Learn more about the work of our research integrity team to safeguard the quality of each article we publish.
Find out more
MINI REVIEW article
Front. Nat. Prod. , 29 February 2024
Sec. Structural and Stereochemical Analysis
Volume 3 - 2024 | https://doi.org/10.3389/fntpr.2024.1359151
This article is part of the Research Topic NMR insights into Natural Product Chemistry View all 6 articles
The field of metabolomics has witnessed remarkable growth in the context of natural products studies, with Mass Spectrometry (MS) being the predominant analytical tool for data acquisition. However, MS has inherent limitations when it comes to the structural elucidation of key metabolites, which can hinder comprehensive compound identification. This review paper discusses the integration of Nuclear Magnetic Resonance (NMR) spectroscopy as a complementary technique to address these limitations. We explore the concept of Quality Control (QC) samples, emphasizing their potential use for in-depth compound annotation and identification. Additionally, we discuss NMR’s advantages, limitations, and strategies to enhance sensitivity. We present examples where MS alone falls short in delivering accurate compound identification and introduce various tools for NMR compound identification in complex mixtures and the integration of MS and NMR data. Finally, we delve into the concept of DBsimilarity to broaden the chemical space understanding, aiding in compound annotation and the creation of compound lists for specific sample analyses.
Metabolomics has witnessed a remarkable expansion within the realm of natural product studies, propelled by the growing interest in deciphering the intricate metabolic profiles of diverse biological systems (Sumner, Lei et al., 2015). In this analytical landscape, Mass Spectrometry (MS) stands out as the predominant choice for data acquisition, owing to its high-throughput capabilities and sensitivity (Demarque, Dusi et al., 2020). However, as metabolomics endeavors extend towards the elucidation of molecular structures crucial for understanding biological pathways, the inherent limitations of MS in providing detailed structural information become apparent (Marshall, 2017; Letertre, Dervilly et al., 2021).
In the realm of natural products research, metabolomics approaches have left an indelible mark. The analysis of complex mixtures serves as a crucial initial step in delineating the chemical profile of a particular organism. It is through this analytical lens that researchers embark on the discernment of intricate metabolic landscapes, laying the foundation for informed decisions on sample prioritization (Simmler, Napolitano et al., 2014). The inherent complexity of natural product mixtures requires sophisticated analytical tools to unravel the intricate web of chemical entities present. Here, the integration of MS has emerged as a cornerstone, facilitating high-throughput screening of diverse metabolites within complex matrices (Kuhn, Colreavy-Donnelly et al., 2019). However, the challenge persists in the elucidation of detailed molecular structures annotated with MS data only, a critical facet for understanding the biological significance of these natural products.
The challenge lies in the necessity for comprehensive structure elucidation of key metabolites, a task for which MS alone may fall short (Gathungu, Kautz et al., 2020). To address this limitation and enhance the robustness of studies, the integration of Nuclear Magnetic Resonance (NMR) spectroscopy emerges as a pivotal complement to MS. Unlike MS, NMR excels in offering direct insights into molecular structures, providing crucial information for the unambiguous identification of metabolites. Additionally, NMR provides inherent quantitative capabilities as a primary method of measurement (Bharti and Roy, 2012).
While NMR proves to be indispensable for structural elucidation, it is essential to acknowledge its own set of limitations. Sensitivity and database comprehensiveness stand out as notable challenges in NMR-based metabolomics and natural products. NMR does not match the sensitivity of MS, particularly in the detection of low-abundance metabolites. And, to supplement this comparison, it is noteworthy that, in these contexts, the majority of MS analyses entail a chromatographic separation, typically in liquid or gas phase chromatography (LC-MS and GC-MS), introducing a level of selectivity rarely employed in NMR analysis. Moreover, the breadth of NMR databases for metabolite identification does not match the comprehensive resources available in the MS domain. It is important to acknowledge that neither MS nor NMR-derived databases can claim completeness, and it seems unlikely that they will ever achieve the level of comprehensiveness desired. This inherent limitation has spurred efforts from various research groups to address the shortfall by developing innovative tools aimed at virtually augmenting and compensating for the deficiencies in these databases (Go, 2010; Bingol, Bruschweiler-Li et al., 2015; Vinaixa, Schymanski et al., 2016; Wang, Carver et al., 2016; Wishart, Guo et al., 2021).
MS is instrumental in detecting metabolites under various conditions, offering invaluable insights into the dynamic changes within organisms with unbeatable sensitivity and selectivity which makes it most attractive for complex mixtures analysis. However, its utility encounters significant constraints when it comes to unraveling the intricate structures of these metabolites.
The unattended reliance on library matching tools in the annotation of complex mixtures introduces a potential pitfall, as users may inadvertently misidentify compounds (Figure 1). This review refrains from assigning liability to any other paper but acknowledges instances where identifications based solely on MS data have been reported. To navigate these challenges, the Metabolomics Standardization Initiative suggests the use of four different levels of identification to provide readers a framework to assess the reliability of metabolite identifications (Fiehn, Kristal et al., 2006; Sansone, Fan et al., 2007; Sumner, Amberg et al., 2007; Fernie, Aharoni et al., 2011; Alseekh, Aharoni et al., 2021).
FIGURE 1. Example of isomers detected from a dataset (data not published) showing the MS fragmentogram of the precursor ion at m/z 449.1090 with the same diagnostic fragments at m/z 151.0027 and 287.0573. These are doubtless four different compounds that show the same MS fragmentogram and this is why it is impossible to assert the identity of each compound beyond reasonable doubt. Library matching provided the same annotation for all these four features.
The complexity amplifies when dealing with isomeric compounds and structural diversity, confounding MS-based analyses and impeding the differentiation of compounds with similar molecular weights. Furthermore, even in non-isomeric compounds, the presence of isobaric fragments can lead to erroneous annotations through library matching where the script might generate high similarity scores.
False identifications of a compound constitute one challenge, while another issue involves the failure to identify a compound present in the database, akin to a false negative result. This circumstance may arise from issues with the fragmentogram derived from MS, potentially attributable to experimental detection parameters within the MS methodology: mass range, cone and skimmer voltage (Murata, Takao et al., 1994), choice for the ionization mode, etc.
A significant limitation arises from the pure inability of MS to furnish definitive information for the analyst to identify the linkage of substituents to a core structure (Figure 1). This inherent shortfall highlights the need for complementary techniques, such as Nuclear Magnetic Resonance (NMR), to provide the requisite structural details for a comprehensive understanding of metabolite profiles. NMR stands as an indispensable and highly informative tool in the realm of organic chemistry. Its unparalleled capability to unravel molecular structures with atomic-level precision makes it a cornerstone in the analytical arsenal of organic chemists as it provides a wealth of detailed information about the chemical environment, connectivity, and configuration of atoms within a molecule. One of the key strengths of NMR lies in its non-destructive nature, allowing for the analysis of compounds in their native state without altering their integrity. Even though its sensitivity is lower than MS’ sensitivity in several orders of magnitude, advancements in technology, such as high-field magnets, cryoprobes, and sophisticated pulse sequences, NMR continues to evolve, pushing the boundaries of what can be achieved in metabolite identification.
In the pursuit of elevating the reliability and precision of metabolomics studies, the incorporation of quality control (QC) samples emerges as a strategic imperative (Dudzik, Barbas-Bernardos et al., 2018). QC samples play a crucial role in metabolomics studies, ensuring the reliability and consistency of data across complex experiments. Typically, QC samples are prepared as pooled samples (QC pooled) and reference standards. The pooled sample contains a representative mix of all the metabolites present in the study and is analysed periodically throughout the experiment. A reference standards sample contains commercially available reference standards of known metabolites. Among these, the QC pooled sample assumes a pivotal role in untargeted metabolomics due to its comprehensive representation of the study’s sample set.
A distinctive advantage in leveraging QC pooled samples for compound identification lies in their amenability to concentration, enabling a nuanced and thorough annotation strategy (Torres, Scalco et al., 2023). This sample can undergo fractionation, potentially simplifying the complexity of the mixture under examination. Furthermore, the prospect of purifying compounds and discerning biomarkers discovered in the metabolomics study adds an additional layer of refinement to the analytical approach.
In natural products studies, a prevalent practice involves dedicated efforts to fractionate compounds, aiming for enhanced purity conducive to comprehensive structure elucidation. Intriguingly, this rigorous approach is not as conspicuous in the metabolomics literature. Several factors contribute to this disparity, with a prominent one being the often limited quantity of samples available per study. This limitation may dissuade researchers from extensive fractionation efforts, emphasizing the need for efficient strategies that balance the quest for structural elucidation with the practical considerations of sample availability.
NMR spectroscopy stands as an invaluable complement in the analytical toolkit, unrivaled in its ability to furnish structural information with atomic-level precision (Figure 2). Despite its unmatched strengths, NMR grapples with limitations, primarily in sensitivity and database comprehensiveness. The challenge of low sensitivity is exacerbated when analyzing complex samples without prior fractionation, potentially leading to the oversight of diluted compounds.
FIGURE 2. A schematic representation illustrates the limitations of MS in offering the complete structure, emphasizing the complementary role of NMR. Here, nJXX denotes coupling constants through n bonds, encompassing both heteronuclear (CH) and homonuclear (HH) interactions.
In response to these challenges, innovative developments have emerged to enhance NMR sensitivity. Cryoprobes, which involve cooling both the probe and its electronics to near liquid helium temperatures (∼20K), present a remarkable up to 4x gain in sensitivity by reducing electronic thermal noise. Additionally, microprobes, exemplified by 1.7 mm probes, offer around a 2.4x sensitivity gain compared to their equivalent 3 mm counterparts. Pushing the boundaries further, the use of higher field magnets exceeding 18.8T (800 MHz for 1H) contributes to heightened sensitivity in NMR analyses. Non-Uniformly Sampling (NUS) schemes for NMR detection is an approach that allows the collection of NMR data in a fraction of time, presenting a paradigm shift in the efficiency of NMR detection (Aoto, Fenwick et al., 2014; Delaglio, Walker et al., 2017). NUS schemes depart from the conventional uniform sampling of data points in the time domain, instead selectively acquiring a subset of the data according to specific sampling schedules. This strategic selection optimizes the use of experimental time, offering accelerated data acquisition without compromising the fidelity of the spectral information. Another noteworthy advancement in optimizing NMR efficiency is the introduction of new pulse sequences, such as the NOAH supersequences (Hansen, Kupce et al., 2021). These sequences represent a pioneering class of NMR experiments designed to expedite data acquisition by collecting a batch of bidimensional NMR data also in a fraction of the time. Particularly, NOAH supersequences streamline the process by acquiring a sequence of experiments under a single relaxation delay, a critical component of a pulse sequence. NOAH supersequences can also be acquired using NUS detection scheme, further optimizing machine time efficiency. Several combinations of experimental modules can be generated and downloaded from the online tool NMR-GENESIS via https://nmr-genesis.co.uk (Yong, Kupce, et al., 2022).
Resuming to the potential of the QC pooled samples for deep compound annotation, a highly recommended strategy involves submitting these samples to NOAH supersequences NMR analysis using a combination of Heteronuclear Multiple Bond Correlation (HMBC), Heteronuclear Single Quantum Coherence (HSQC), and hybrid HSQC-Total Correlation Spectroscopy (HSQC-TOCSY). This concerted approach, implemented with high resolution in the f1 (13C axis) axis, comprising at least 1k points, along with an ample number of scans for robust signal averaging, facilitates the generation of well-defined peak shapes even for diluted compound (Nagana Gowda and Raftery, 2023). The emphasis on high resolution in the f1 axis is pivotal, especially for complex mixtures studies, as it ensures the meticulous delineation of intricate molecular structures, even for small and potentially diluted peaks.
Therefore, two primary tools freely available for the compound annotation of complex mixtures using bidimensional NMR data are COLMAR and NMRfilter. The first, Complex Mixture Analysis (COLMAR) by NMR (Wang, Timári et al., 2020), spearheaded by prof. Rafael Brüschweiler at The Ohio State University (United States of America), stands as a prominent method for compound identification in mixtures using NMR data. Accessible through a user-friendly web server, COLMAR is extensively utilized within the NMR metabolomics community. Notably, it combines metabolite data from major databases, including BMRB and HMDB, offering one of the most comprehensive tools for matching experimental NMR data of complex mixtures. While primarily impactful for primary metabolites, COLMAR does face limitations in studies with secondary metabolites. It relies on high-quality spectra acquired under standard conditions, often using D2O-phosphate buffer at pH 7.4 as the solvent. Recent enhancements include a library of less hydrophilic compounds analyzed in CDCl3. Despite its reliance on accurate chemical shifts, the chemical shift of 1H-NMR is highly dependent on solvation, solvent pH, and temperature. This poses challenges when analyzing compounds not soluble in D2O or CDCl3, such as those using CD3OD or DMSO.d6. A notable feature is the COLMARm method, where users can submit experimental HSQC, TOCSY, and HSQC-TOCSY data. This enables high-confidence searches and identifications, employing computational matching and subsequent validation using TOCSY data. COLMAR’s significance is underscored by over 70 citations and more than 2000 registered views, making it the tool of choice for water-soluble samples in primary metabolite studies.
NMRfilter, a collaborative effort led by prof. Ricardo M. Borges at the Federal University of Rio de Janeiro (Brazil) and prof. Stefan Kuhn at the University of Tartu (Estonia), originally aimed for combining MS and NMR data interpretation (Kuhn, Colreavy-Donnelly et al., 2019; Kuhn, Colreavy-Donnelly et al., 2020). From a list of candidate compounds (generated from the MS dereplication routine or using data from literature (Borges, Ferreira et al., 2023b), NMRfilter simulates and compares chemical shift data with users’ experimental NMR data. The algorithm then searches for scalar coupling networks in the HMBC and HSQC-TOCSY data to confirm shared chemical structures. The effectiveness of NMRfilter relies on accurate NMR data simulations, which is achieved through nmrshiftdb2 (Kuhn, Schlörer et al., 2012; Kuhn and Schlörer, 2015; Kuhn and Johnson, 2019). Users are encouraged to submit NMR data to nmrshiftdb2 for a more comprehensive and accurate database. The method provides visual validation, allowing users to evaluate matching rates and peak profiles for confident identifications. Similar to COLMARm, NMRfilter utilizes spin systems (HMBC and HSQC-TOCSY) for detecting spin fragments, enhancing the accuracy of compound identification.
The integration of MS and NMR data represents a robust strategy to surmount the inherent limitations of each technique. By capitalizing on the unique advantages offered by both methods, researchers can achieve a high level of confidence in compound identification.
One approach involves the meticulous analysis of QC pooled samples through both MS and NMR through a detailed dereplication scheme. This entails annotating detected compounds comprehensively, mainly using the available library for matching, combining the results to cross-validate compounds annotated through the MS with those exhibiting diagnostic peaks in NMR spectra. Alternatively, a more comprehensive strategy involves acquiring MS and NMR data for every sample in the study, assuming an adequate number of replicates was prepared. Biological variance, manifested by variable peak intensities in both MS and NMR across replicates, can be explored, leveraging the high correlation expected for peaks from the same compound. The introduction of DAFdiscovery emerges as a valuable tool in this context, facilitating the amalgamation of peak tables from MS and NMR for direct covariance and correlation calculations (Borges, das Neves Costa et al., 2023a). DAFdiscovery enables the identification of peak pairs strongly correlated through a Statistical Total Correlation Spectroscopy (STOCSY) (De Souza Wuillda et al., 2024) calculation, suggesting shared chemical structures due to their dependence on concentration. In instances where fractionation is applied to the QC pooled sample (or the raw extract sample), creating variance in compound concentrations based on chromatographic behavior, DAFdiscovery can further enhance confidence by connecting NMR peaks to MS features in the fractions.
As a final option, though no less significant, users can invest in the retention time parameter from the LC-MS (or GC-MS) of the designated biomarker to scale up the analytical technique and purify the compounds of interest. Subsequently, the focus shifts to a de novo structure elucidation, requiring the acquisition of a comprehensive set of bidimensional NMR data for unequivocal determination. Within the NOAH supersequences class, there are opportunities to synergistically combine HMBC, HSQC, COSY, NOESY/ROESY techniques. This integrated approach enables the acquisition of comprehensive NMR spectral data in significantly less time compared to employing individual pulse sequences (Kupče and Claridge, 2017).
Secondary metabolites are diverse chemical compounds produced by living organisms. They are not directly involved in primary growth and reproduction, but they represent a vast and fascinating area within the realm of natural products (Rose, Gottfries et al., 2009). This chemical space, which includes a remarkable variety of structures and properties, mirrors the complex interplay between evolutionary pressures and the limitless ingenuity of biological systems. These compounds are typically structurally complex, and their diversity is indicative of the broad range of biological roles they fulfill. Scientists are greatly interested in this chemical space, as these compounds often exhibit strong biological activities and can serve as a starting point for the development of new drugs. The term “chemical space” refers to the conceptual space encompassing a group of chemical compounds, defined by their molecular structures and the potential range of properties these structures exhibit. The exploration of such chemical spaces allows scientists to identify compounds with desirable properties or to understand the biochemical mechanisms underlying various physiological states and diseases. However, the exploration of this space is challenging due to the complexity and diversity of these compounds, as well as the difficulty in isolating and characterizing them. Despite these challenges, the study of the chemical space of secondary metabolites in natural products continues to provide valuable insights into biology and medicine.
The term “chemical space” describes a multi-dimensional domain where chemical compounds are positioned based on their structural characteristics, properties, or other molecular descriptors. The visualization of this space involves creating a graphical representation of these compounds, which allows researchers to identify relationships and patterns among them (Bade, Chan et al., 2010). This visualization is particularly useful when dealing with natural products, which are typically structurally complex and diverse. It enables researchers to locate known compounds and focus on discovering new compounds with potential properties. For example, it assists in identifying clusters or regions that are associated with specific bioactivities and in recognizing trends and similarities among natural products with similar biological properties. Although a rich dataset of chemical information for a list of compounds can be represented as a multidimensional space, humans have limitations in visualizing such complexity. Therefore, techniques for visualizing chemical space are necessary. Principal Component Analysis (PCA) is a statistical technique that reduces the dimensionality of chemical data while preserving its variability. It is commonly used to generate 2D or 3D plots that represent chemical space (Ringner, 2008; El-Elimat, Raja et al., 2020). Another technique, t-Distributed Stochastic Neighbor Embedding (t-SNE), is particularly effective for visualizing high-dimensional data in a lower-dimensional space, revealing both local and global relationships among compounds (Vivek, Mithun, et al., 2023). Self-Organizing Maps (SOM), a type of artificial neural network, can be used for clustering and visualizing chemical space, aiding in the identification of groups of structurally similar compounds (Bonachera, Marcou, et al., 2012). Furthermore, the concept of networking has been incorporated into the chemical space application DBsimilarity (Borges, de Assis Ferreira et al., 2023b). This tool calculates molecular similarities among all compounds in a given list of structures, creating a network of nodes and edges. In this network, nodes represent individual compounds, and the edges that connect them represent the similarity values between the respective pairs. The script also generates files with attributes for each compound, enriching the similarity network of valuable information. Attributes such as chemical classification, determined by an external tool, and various molecular descriptors can be integrated into the network visualization. DBsimilarity also allows users to include an additional list of compounds known to have specific properties during calculations, aiding in the identification of compounds closely related to the active ones and suggesting potential new active compounds.
Given the recognized taxonomic significance of secondary metabolites, visualizing the chemical spaces of a specific organism can aid in compound identification, as it allows for the formulation of hypotheses regarding potential compounds. While this concept has not been systematically explored yet, it forms the basis for some modern concepts in the field. This approach could potentially revolutionize the way we identify and study compounds, opening up new avenues for research and discovery. It is an exciting prospect for the future of natural product chemistry and drug discovery (Kim, Kim et al., 2020).
This paper highlights the significance of NMR in MS-based metabolomics and natural products studies. By addressing the limitations of MS and harnessing the strengths of NMR, researchers can achieve more comprehensive and accurate compound identification, ultimately advancing our understanding of the chemical diversity within natural sources. The QC pooled sample is highlighted as dual purpose. It not only ensures the reproducibility and reliability of the analytical measurements but also presents a unique opportunity for in-depth compound annotation and identification. The integration of NOAH supersequences and NUS detection into the analysis of QC pooled samples holds the promise of substantially enhancing the depth and accuracy of compound annotation. By leveraging the synergistic power of these advanced NMR techniques, researchers can unlock a wealth of information embedded in complex metabolomic profiles, providing a more comprehensive understanding of the chemical intricacies within biological samples. COLMAR and NMRfilter exemplify powerful tools for NMR-based compound identification in complex mixtures, each offering unique approaches to address specific challenges in the metabolomics field.
RB: Conceptualization, Writing–original draft, Writing–review and editing. AT: Conceptualization, Writing–original draft, Writing–review and editing.
The author(s) declare that financial support was received for the research, authorship, and/or publication of this article. Fundação Carlos Chagas Filho de Amparo à Pesquisa do Estado do Rio de Janeiro (FAPERJ): Grant 210.489/2019 APQ-1. Coordenação de Aperfeiçoamento de Pessoal de Nível Superior–Brasil (CAPES): Finance Code 001. Conselho Nacional de Desenvolvimento Científico e Tecnológico (CNPq): 304501/2021-2.
The authors would like to acknowledge the contributions of the scientific community in advancing the field of metabolomics, as well as the developers of the various tools and software discussed in this paper. This work was supported by FAPERJ and CNPq.
The authors declare that the research was conducted in the absence of any commercial or financial relationships that could be construed as a potential conflict of interest.
All claims expressed in this article are solely those of the authors and do not necessarily represent those of their affiliated organizations, or those of the publisher, the editors and the reviewers. Any product that may be evaluated in this article, or claim that may be made by its manufacturer, is not guaranteed or endorsed by the publisher.
Alseekh, S., Aharoni, A., Brotman, Y., Contrepois, K., D’auria, J., Ewald, J., et al. (2021). Mass spectrometry-based metabolomics: a guide for annotation, quantification and best reporting practices. Nat. Methods 18, 747–756. doi:10.1038/s41592-021-01197-1
Aoto, P. C., Fenwick, R. B., Kroon, G. J. A., and Wright, P. E. (2014). Accurate scoring of non-uniform sampling schemes for quantitative NMR. J. Magnetic Reson. 246, 31–35. doi:10.1016/j.jmr.2014.06.020
Bade, R., Chan, H. F., and Reynisson, J. (2010). Characteristics of known drug space. Natural products, their derivatives and synthetic drugs. Eur. J. Med. Chem. 45, 5646–5652. doi:10.1016/j.ejmech.2010.09.018
Bharti, S. K., and Roy, R. (2012). Quantitative 1H NMR spectroscopy. TrAC Trends Anal. Chem. 35, 5–26. doi:10.1016/j.trac.2012.02.007
Bingol, K., Bruschweiler-Li, L., Yu, C., Somogyi, A., Zhang, F., and Brüschweiler, R. (2015). Metabolomics beyond spectroscopic databases: a combined MS/NMR strategy for the rapid identification of new metabolites in complex mixtures. Anal. Chem. 87, 3864–3870. doi:10.1021/ac504633z
Bonachera, F., Marcou, G., Kireeva, N., Varnek, A., and Horvath, D. (2012). Using self-organizing maps to accelerate similarity search. Bioorg. Med. Chem. 20, 5396–5409. doi:10.1016/j.bmc.2012.04.024
Borges, R. M., Das Neves Costa, F., Chagas, F. O., Teixeira, A. M., Yoon, J., Weiss, M. B., et al. (2023). Data Fusion-based Discovery (DAFdiscovery) pipeline to aid compound annotation and bioactive compound discovery across diverse spectral data. Phytochem. Anal. 34, 48–55. doi:10.1002/pca.3178
Borges, R. M., De Assis Ferreira, G., Campos, M. M., Teixeira, A. M., Das Neves Costa, F., and Chagas, F. O. (2023). Data Base similarity (DBsimilarity) of natural products to aid compound identification on MS and NMR pipelines, similarity networking, and more. Phytochem. Anal. 35, 93–101. doi:10.1002/pca.3277
Delaglio, F., Walker, G. S., Farley, K. A., Sharma, R., Hoch, J. C., Arbogast, L. W., et al. (2017). Non-uniform sampling for all: more NMR spectral quality, less measurement time. Am. Pharm. Rev. 20, 339681.
Demarque, D. P., Dusi, R. G., De Sousa, F. D. M., Grossi, S. M., Silverio, M. R. S., Lopes, N. P., et al. (2020). Mass spectrometry-based metabolomics approach in the isolation of bioactive natural products. Sci. Rep. 10, 1051. doi:10.1038/s41598-020-58046-y
De Souza Wuillda, A. C. J., Das Neves Costa, F., Garrett, R., Dos Santos De Carvalho, M., and Borges, R. M. (2024). High-speed countercurrent chromatography with offline detection by electrospray mass spectrometry and nuclear magnetic resonance detection as a tool to resolve complex mixtures: a practical approach using Coffea arabica leaf extract. Phytochem. Anal. 35, 40–52. doi:10.1002/pca.3271
Dudzik, D., Barbas-Bernardos, C., García, A., and Barbas, C. (2018). Quality assurance procedures for mass spectrometry untargeted metabolomics. a review. J. Pharm. Biomed. Analysis 147, 149–173. doi:10.1016/j.jpba.2017.07.044
El-Elimat, T., Raja, H. A., Figueroa, M., Al Sharie, A. H., Bunch, R. L., and Oberlies, N. H. (2020). Freshwater fungi as a source of chemical diversity: a review. J. Nat. Prod. 84 (3), 898–916. doi:10.1021/acs.jnatprod.0c01340
Fernie, A. R., Aharoni, A., Willmitzer, L., Stitt, M., Tohge, T., Kopka, J., et al. (2011). Recommendations for reporting metabolite data. Plant Cell 23, 2477–2482. doi:10.1105/tpc.111.086272
Fiehn, O., Kristal, B. S., Ommen, B. V., Sumner, L. W., Sansone, S. A., Taylor, C., et al. (2006). Establishing reporting standards for metabolomic and metabonomic studies: a call for participation. OMICS A J. Integr. Biol. 10, 158–163. doi:10.1089/omi.2006.10.158
Gathungu, R. M., Kautz, R., Kristal, B. S., Bird, S. S., and Vouros, P. (2020). The integration of LC-MS and NMR for the analysis of low molecular weight trace analytes in complex matrices. Mass Spectrom. Rev. 39, 35–54. doi:10.1002/mas.21575
Go, E. P. (2010). Database resources in metabolomics: an overview. J. Neuroimmune Pharmacol. 5, 18–30. doi:10.1007/s11481-009-9157-3
Hansen, A. L., Kupce, E. R., Li, D. W., Bruschweiler-Li, L., Wang, C., and Bruschweiler, R. (2021). 2D NMR-based metabolomics with HSQC/TOCSY NOAH supersequences. Anal. Chem. 93, 6112–6119. doi:10.1021/acs.analchem.0c05205
Kim, H. W., Kim, S. S., Kang, K. B., Ryu, B., Park, E., Huh, J., et al. (2020). Combined MS/MS-NMR annotation guided discovery of Iris lactea var. chinensis seed as a source of viral neuraminidase inhibitory polyphenols. Molecules 25, 3383. doi:10.3390/molecules25153383
Kuhn, S., Colreavy-Donnelly, S., De Andrade Silva Quaresma, L. E., De Andrade Silva Quaresma, E., and Borges, R. M. (2020). Applying NMR compound identification using NMRfilter to match predicted to experimental data. Metabolomics 16, 123. doi:10.1007/s11306-020-01748-1
Kuhn, S., Colreavy-Donnelly, S., Santana De Souza, J., and Borges, R. M. (2019). An integrated approach for mixture analysis using MS and NMR techniques. Faraday Discuss. 218, 339–353. doi:10.1039/c8fd00227d
Kuhn, S., and Johnson, S. R. (2019). Stereo-aware extension of HOSE codes. ACS Omega 4, 7323–7329. doi:10.1021/acsomega.9b00488
Kuhn, S., and Schlörer, N. E. (2015). Facilitating quality control for spectra assignments of small organic molecules: nmrshiftdb2 – a free in-house NMR database with integrated LIMS for academic service laboratories. Magnetic Reson. Chem. 53, 582–589. doi:10.1002/mrc.4263
Kuhn, S., Schlörer, N. E., Kolshorn, H., and Stoll, R. (2012). From chemical shift data through prediction to assignment and NMR LIMS - multiple functionalities of nmrshiftdb2. J. Cheminformatics 4, 52. doi:10.1186/1758-2946-4-s1-p52
Kupče, Ē., and Claridge, T. D. W. (2017). NOAH: NMR supersequences for small molecule analysis and structure elucidation. Angew. Chem. Int. Ed. Engl. 56, 11779–11783. doi:10.1002/anie.201705506
Letertre, M. P. M., Dervilly, G., and Giraudeau, P. (2021). Combined nuclear magnetic resonance spectroscopy and mass spectrometry approaches for metabolomics. Anal. Chem. 93, 500–518. doi:10.1021/acs.analchem.0c04371
Marshall, D. D., and Powers, R. (2017). Beyond the paradigm Combining mass spectrometry and nuclear magnetic resonance for metabolomics. Prog. Nucl. Magnetic Reson. Spectrosc. 100, 1–16. doi:10.1016/j.pnmrs.2017.01.001
Murata, H., Takao, T., Shimonishi, Y., and Matsuo, T. (1994). Optimization of skimmer voltages of an electrospray ion source coupled with a magnetic sector instrument. Rapid Commun. Mass Spectrom. 8, 205–210. doi:10.1002/rcm.1290080216
Nagana Gowda, G. A., and Raftery, D. (2023). NMR metabolomics methods for investigating disease. Anal. Chem. 95, 83–99. doi:10.1021/acs.analchem.2c04606
Ringnér, M. (2008). What is principal component analysis? Nat. Biotechnol. 26 (3), 303–304. doi:10.1038/nbt0308-303
Rose, J., Gottfries, J., Muresan, S., Anders Backlund, A., and Oprea, T. I. (2009). Novel chemical space exploration via natural products. J. Med. Chem. 52, 1953–1962. doi:10.1021/jm801514w
Sansone, S.-A., Fan, T., Goodacre, R., Griffin, J. L., Hardy, N. W., Kaddurah-Daouk, R., et al. (2007). The metabolomics standards initiative. Nat. Biotechnol. 25, 846–848. doi:10.1038/nbt0807-846b
Simmler, C., Napolitano, J. G., Mcalpine, J. B., Chen, S. N., and Pauli, G. F. (2014). Universal quantitative NMR analysis of complex natural samples. Curr. Opin. Biotechnol. 25, 51–59. doi:10.1016/j.copbio.2013.08.004
Sumner, L. W., Amberg, A., Barrett, D., Beale, M. H., Beger, R., Daykin, C. A., et al. (2007). Proposed minimum reporting standards for chemical analysis. Metabolomics 3, 211–221. doi:10.1007/s11306-007-0082-2
Sumner, L. W., Lei, Z., Nikolau, B. J., and Saito, K. (2015). Modern plant metabolomics: advanced natural product gene discoveries, improved technologies, and future prospects. Nat. Prod. Rep. 32, 212–229. doi:10.1039/c4np00072b
Torres, C. L., Scalco, F. B., De Oliveira, M. L. C., Peake, R. W. A., and Garrett, R. (2023). Untargeted LC-HRMS metabolomics reveals candidate biomarkers for mucopolysaccharidoses. Clin. Chim. Acta 541, 117250. doi:10.1016/j.cca.2023.117250
Vinaixa, M., Schymanski, E. L., Neumann, S., Navarro, M., Salek, R. M., and Yanes, O. (2016). Mass spectral databases for LC/MS- and GC/MS-based metabolomics: state of the field and future prospects. TrAC Trends Anal. Chem. 78, 23–35. doi:10.1016/j.trac.2015.09.005
Vivek, P., Mohanan, M., Shajil, U. K., Sandesh, E.Pa., and Jaleel, U. C. A. (2023). Graph Attention Network based mapping of knowledge relations between chemical spaces of Nuclear factor kappa B and Centella asiatica. Comput. Biol. Chem. 107, 107955. doi:10.1016/j.compbiolchem.2023.107955
Wang, C., Timári, I., Zhang, B., Li, D.-W., Leggett, A., Amer, A. O., et al. (2020). COLMAR lipids web server and ultrahigh-resolution methods for two-dimensional nuclear magnetic resonance- and mass spectrometry-based lipidomics. J. Proteome Res. 19, 1674–1683. doi:10.1021/acs.jproteome.9b00845
Wang, M., Carver, J. J., Phelan, V. V., Sanchez, L. M., Garg, N., Peng, Y., et al. (2016). Sharing and community curation of mass spectrometry data with global natural products social molecular networking. Nat. Biotechnol. 34, 828–837. doi:10.1038/nbt.3597
Wishart, D. S., Guo, A., Oler, E., Wang, F., Anjum, A., Peters, H., et al. (2021). HMDB 5.0: the human metabolome database for 2022. Nucleic Acids Res. 50, D622–D631. doi:10.1093/nar/gkab1062
Keywords: NMR, MS-metabolomics, compound identification, dereplication, natural products
Citation: Borges RM and Teixeira AM (2024) On the part that NMR should play in mass spectrometry metabolomics in natural products studies. Front. Nat. Produc. 3:1359151. doi: 10.3389/fntpr.2024.1359151
Received: 20 December 2023; Accepted: 20 February 2024;
Published: 29 February 2024.
Edited by:
Alan Diego Conceição Santos, Federal University of Amazonas, BrazilReviewed by:
Livia Dutra, Federal University of São Francisco Valley, BrazilCopyright © 2024 Borges and Teixeira. This is an open-access article distributed under the terms of the Creative Commons Attribution License (CC BY). The use, distribution or reproduction in other forums is permitted, provided the original author(s) and the copyright owner(s) are credited and that the original publication in this journal is cited, in accordance with accepted academic practice. No use, distribution or reproduction is permitted which does not comply with these terms.
*Correspondence: Ricardo M. Borges, cmljYXJkb19tYm9yZ2VzQHVmcmouYnI=
Disclaimer: All claims expressed in this article are solely those of the authors and do not necessarily represent those of their affiliated organizations, or those of the publisher, the editors and the reviewers. Any product that may be evaluated in this article or claim that may be made by its manufacturer is not guaranteed or endorsed by the publisher.
Research integrity at Frontiers
Learn more about the work of our research integrity team to safeguard the quality of each article we publish.