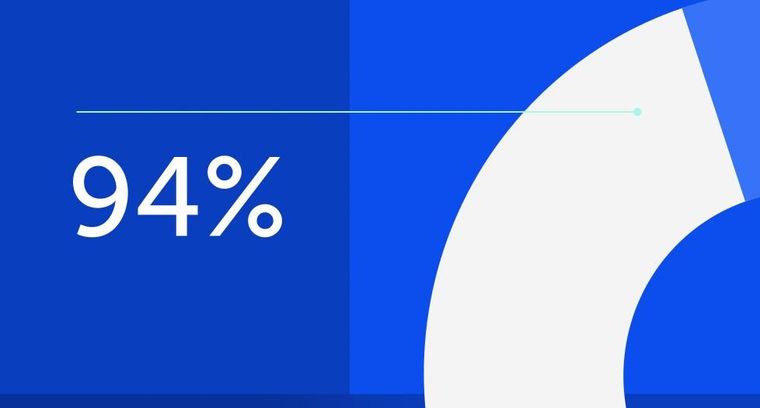
94% of researchers rate our articles as excellent or good
Learn more about the work of our research integrity team to safeguard the quality of each article we publish.
Find out more
PERSPECTIVE article
Front. Nanotechnol., 10 April 2025
Sec. Nanodevices
Volume 7 - 2025 | https://doi.org/10.3389/fnano.2025.1583483
This article is part of the Research TopicThought Leaders in Nanotechnology ResearchView all 4 articles
The demand for aggressive scaling in integrated circuits technology has been a primary driving force behind the rapid advancement of nanotechnology, leading to groundbreaking innovations in nanoscience, engineering, and technology. Initially, the unique phenomena observed at nanoscale enable innovative applications in nanodevices. Now, as our understanding has greatly developed, nanodevices are increasingly being leveraged to provide solutions for a growing range of applications. In this perspective, several key areas are featured that are proposed to benefit significantly from advancements in nanodevices.
Over the past few decades, continuous miniaturization has not only pushed the boundaries of traditional semiconductor devices but has also unlocked novel physical phenomena that emerge at the nanoscale (Veendrick, 2024). The unprecedented properties exhibited at nanoscale materials—such as ballistic transport and tailored optical behavior—fueled their integration into next-generation electronic and optoelectronic devices. And, as our understanding of nanomaterials and their interactions has deepened, their applications have expanded far beyond conventional electronic scaling. Today, nanodevices are playing an increasingly crucial role for transformative applications across multiple disciplines. As research continues to unlock new capabilities, many areas are expected to benefit significantly from the ongoing advancements in nanodevices. Here, we just mentioned few of them, as shown in Figure 1. From next-generation transistors and neuromorphic computing to neural interface and quantum computing, the potential for nanotechnology to drive innovation across multiple sectors remains vast and largely untapped.
Aggressive device scaling of silicon technologies has dominantly driven the semiconductor industry for decades, which brings great benefits to digital circuits in terms of speed, power consumption and density. As traditional silicon-based electronics approach their physical limits, nanodevices offer a pathway to continue Moore’s Law through innovations in materials and device architectures. Low-dimensional materials (2D materials, carbon nanotube, etc.) with high mobility, ultrathin bodies and good immunity to short-channel effects are extensively studied to explore their potential to provide solutions toward the ultimate scaling (Das et al., 2021; Franklin et al., 2022). Among diverse transistor structures have been investigated, nanosheet field-effect transistors (NS-FETs) (Loubet et al., 2017; Hook, 2018), are expected to be alternative to FinFET technology, especially for advanced semiconductor technologies beyond the 3 nm node (Alabdullah et al., 2024), which can provide superior electrostatic control over the channel with higher current drivability compared to other structures, and are compatibility with the conventional FinFET fabrication processes. These advancements are pushing the boundaries of performance, enabling faster, smaller, and more energy-efficient devices. In addition, monolithic 3D integration technology provides another solution to extend conventional transistor scaling, by integrating multiple transistor layers via vertical interconnects above a single substrate to enables unprecedented integration density and high bandwidth communication (Dhananjay et al., 2021; Kim et al., 2024; Shulaker et al., 2015). Innovations provided by nanomaterials, new device structure and integration architecture are critical to support continuous advancements toward the ultimate scaling.
Neuromorphic computing represents a paradigm shift in the way we design and implement computing systems. By mimicking the structure and function of the human brain, neuromorphic systems offer unparalleled efficiency and adaptability for tasks such as pattern recognition, real-time learning, and decision-making. New nanodevices that provide new physics to emulate synapse- and neuron-like functionalities, such as plasticity, spiking behavior and dispersion of conduction velocities (Kandel et al., 2020; Johansson and Flanagan, 2009), are the hardware foundations to realize useful features of the brain, like in-memory computing, spike-based information processing, signal processing resilient to noise, asynchronous communication, and analog computing (Upadhyay et al., 2019). Emerging nanodevices, memristor (Aguirre et al., 2024), phase change memory (Ambrogio et al., 2018), flash memory (Guo et al., 2017; Huang et al., 2023), ferroelectric transistors (Jerry et al., 2017; Kim et al., 2023), spintronics devices (Grollier et al., 2020), to name just a few, have demonstrated their tremendous role in this field. By further addressing device non-ideal challenges such as uniformity and yield, and adopting a properly designed architectures, the integrated systems can perform complex computations with minimal power consumption, which not only redefines artificial intelligence but also paves the way for applications in robotics, autonomous systems, and adaptive technologies (Zhang et al., 2020).
Neural interfaces are bridging the gap between biology and electronics, offering new possibilities for understanding and interacting with the human brain (Valeriani et al., 2022). Here, at the interface, both neural recording and brain stimulation play crucial roles in addressing a wide range of enhancement and therapeutic challenges (Shabani et al., 2023), including improving decision-making, improving learning efficiency, treating neurological disorders, intervening mental health, etc. For deep brain detection and regulation, integrating miniaturized nanodevices to create high-throughput implantable neural interfaces may enable high resolution neural recording and stimulation (Liu et al., 2020; Ranke et al., 2024). For example, by using a custom 130-nm CMOS fabrication processes, 960 recording sites were integrated on a single, 10-mm long, non-tapered shank with 70 × 20-μm cross-section (Jun et al., 2017). Also, higher integration density makes it possible to provide more functions in a limited space, like bidirectional communication and multimodal modulation and detection of neuronal activity, which are still very challenge but critical for understanding and interacting with the brain. Additionally, flexible neural interfaces are expected to overcome the limitations of traditional neurotechnology to minimizes damage to delicate brain tissue by conforming to the brain’s natural movement and shape, allowing for more stable and long-term neural signal recording and stimulation compared to rigid implants (Zhu et al., 2024).
At the nanoscale, quantum phenomena dominate the behavior of materials and devices, providing new opportunities for innovation. Understanding and exploring quantum conductance, electrochemical dynamics, and ionic transport in nanoscale systems are critical for developing next-generation devices such as memristors (Kumar et al., 2022) and quantum computers (de Leon et al., 2021). These advancements not only expand our fundamental knowledge of quantum mechanics but also enable their practical applications in secure communication, high-performance computing, and energy-efficient electronics. In addition, cryogenic nanoelectronics are noteworthy for overcoming low-temperature circuit design challenges for quantum computing (Olšteins et al., 2023; Shiomi and Uchida, 2024).
Innovation enabled by nanodevices will play a major role in multiple fields, to name just a few, that are driving a technological revolution beyond traditional boundaries. From brain-inspired computing to quantum devices, nanotechnology is providing solutions to global challenges in healthcare, energy, and sustainability. By addressing critical challenges related to scalability, fabrication, and integration, their convergence will bring new possibilities for innovation, creating a future where smart, adaptive, and sustainable technologies are seamlessly integrated into our daily lives. By investing in these areas, we are not only advancing science and engineering, but also shaping a better, more connected world.
The original contributions presented in the study are included in the article/supplementary material, further inquiries can be directed to the corresponding author.
YH: Writing – original draft, Writing – review and editing.
The author(s) declare that no financial support was received for the research and/or publication of this article.
The author declares that the research was conducted in the absence of any commercial or financial relationships that could be construed as a potential conflict of interest.
The author(s) declared that they were an editorial board member of Frontiers, at the time of submission. This had no impact on the peer review process and the final decision.
The author(s) declare that no Generative AI was used in the creation of this manuscript.
All claims expressed in this article are solely those of the authors and do not necessarily represent those of their affiliated organizations, or those of the publisher, the editors and the reviewers. Any product that may be evaluated in this article, or claim that may be made by its manufacturer, is not guaranteed or endorsed by the publisher.
Aguirre, F., Sebastian, A., Gallo, M. L., Song, W., Wang, T., Yang, J. J., et al. (2024). Hardware implementation of memristor-based artificial neural networks. Nat. Commun. 15, 1974. doi:10.1038/s41467-024-45670-9
Alabdullah, M. G. K., Elmessary, M. A., Nagy, D., Seoane, N., García-Loureiro, A. J., and Kalna, K. (2024). Scaling challenges of nanosheet field-effect transistors into sub-2 nm nodes. IEEE J. Electron Devices Soc. 12, 479–485. doi:10.1109/jeds.2024.3416200
Ambrogio, S., Narayanan, P., Tsai, H., Shelby, R. M., Boybat, I., di Nolfo, C., et al. (2018). Equivalent-accuracy accelerated neural-network training using analogue memory. Nature 558, 60–67. doi:10.1038/s41586-018-0180-5
Das, S., Sebastian, A., Pop, E., McClellan, C. J., Franklin, A. D., Grasser, T., et al. (2021). Transistors based on two-dimensional materials for future integrated circuits. Nat. Electron. 4, 786–799. doi:10.1038/s41928-021-00670-1
de Leon, N. P., Itoh, K. M., Kim, D., Mehta, K. K., Northup, T. E., Paik, H., et al. (2021). Materials challenges and opportunities for quantum computing hardware. Science 372, eabb2823. doi:10.1126/science.abb2823
Dhananjay, K., Shukla, P., Pavlidis, V. F., Coskun, A., and Salman, E. (2021). Monolithic 3D integrated circuits: recent trends and future prospects. IEEE Trans. Circuits Syst. II Express Briefs 68, 837–843. doi:10.1109/tcsii.2021.3051250
Franklin, A. D., Hersam, M. C., and Wong, H.-S. P. (2022). Carbon nanotube transistors: making electronics from molecules. Science 378 (6621), 726–732. doi:10.1126/science.abp8278
Grollier, J., Querlioz, D., Camsari, K. Y., Everschor-Sitte, K., Fukami, S., and Stiles, M. D. (2020). Neuromorphic spintronics. Nat. Electron. 3, 360–370. doi:10.1038/s41928-019-0360-9
Guo, X., Bayat, F. M., Bavandpour, M., Klachko, M., Mahmoodi, M. R., and Prezioso, M. (2017). Fast, energy-efficient, robust, and reproducible mixed-signal neuromorphic classifier based on embedded NOR flash memory technology. IEEE Int. Electron Devices Meet. (IEDM) 6 (5.1-6.5.4). doi:10.1109/IEDM.2017.8268341
Hook, T. B. (2018). Power and technology scaling into the 5 nm node with stacked nanosheets. Joule 2, 1–4. doi:10.1016/j.joule.2017.10.014
Huang, X., Liu, C., Tang, Z., Zeng, S., Wang, S., and Zhou, P. (2023). An ultrafast bipolar flash memory for self-activated in-memory computing. Nat. Nanotechnol. 18, 486–492. doi:10.1038/s41565-023-01339-w
Jerry, M., Chen, P.-Y., Zhang, J., Sharma, P., Ni, K., and Yu, S. (2017). Ferroelectric FET analog synapse for acceleration of deep neural network training. IEEE Int. Electron Devices Meet. (IEDM) 6 (2.1-6.2.4). doi:10.1109/IEDM.2017.8268338
Johansson, R. S., and Flanagan, J. R. (2009). Coding and use of tactile signals from the fingertips in object manipulation tasks. Nat. Rev. Neurosci. 10, 345–359. doi:10.1038/nrn2621
Jun, J. J., Steinmetz, N. A., Siegle, J. H., Denman, D. J., Bauza, M., Barbarits, B., et al. (2017). Fully integrated silicon probes for high-density recording of neural activity. Nature 551, 232–236. doi:10.1038/nature24636
Kandel, E. R., Schwartz, J. H., Jessell, T. M., Siegelbaum, S. A., and Hudspeth, A. J. (2020). Principles of neural science. McGraw-Hill.
Kim, I.-J., Kim, M.-K., and Lee, J.-S. (2023). Highly-scaled and fully-integrated 3-dimensional ferroelectric transistor array for hardware implementation of neural networks. Nat. Commun. 14, 504. doi:10.1038/s41467-023-36270-0
Kim, K. S., Seo, S., Kwon, J., Lee, D., Kim, C., Ryu, J.-E., et al. (2024). Growth-based monolithic 3D integration of single-crystal 2D semiconductors. Nature 636, 615–621. doi:10.1038/s41586-024-08236-9
Kumar, S., Wang, X., Strachan, J. P., Yang, Y., and Lu, W. D. (2022). Dynamical memristors for higher-complexity neuromorphic computing. Nat. Rev. Mater. 7, 575–591. doi:10.1038/s41578-022-00434-z
Liu, S., Zhao, Y., Hao, W., Zhang, X.-D., and Ming, D. (2020). Micro- and nanotechnology for neural electrode-tissue interfaces. Biosens. Bioelectron. 170, 112645. doi:10.1016/j.bios.2020.112645
Loubet, N., Hook, T., Montanini, P., Yeung, C., Kanakasabapathy, S., Guillom, M., et al. (2017). “Stacked nanosheet gate-all-around transistor to enable scaling beyond FinFET,” in 2017 symposium on VLSI technology (Kyoto, Japan), T230–T231.
Olšteins, D., Nagda, G., Carrad, D. J., Beznasyuk, D. V., Petersen, C. E. N., Martí-Sánchez, S., et al. (2023). Cryogenic multiplexing using selective area grown nanowires. Nat. Commun. 14, 7738. doi:10.1038/s41467-023-43551-1
Ranke, D., Lee, I., Gershanok, S. A., Jo, S., Trotto, E., Wang, Y., et al. (2024). Multifunctional nanomaterials for advancing neural interfaces: recording, stimulation, and beyond. Accounts Chem. Res. 57, 1803–1814. doi:10.1021/acs.accounts.4c00138
Shabani, L., Abbasi, M., Azarnew, Z., Amani, A. M., and Vaez, A. (2023). Neuro-nanotechnology: diagnostic and therapeutic nano-based strategies in applied neuroscience. Biomed. Eng. OnLine 22, 1. doi:10.1186/s12938-022-01062-y
Shiomi, J., and Uchida, K. (2024). Scientific challenges of cryo-electronics thermal management. Nat. Rev. Electr. Eng. 1, 762–763. doi:10.1038/s44287-024-00110-0
Shulaker, M. M., Wu, T. F., Sabry, M. M., Wei, H., Wong, H.-S. P., and Mitra, S. (2015). “Monolithic 3D integration: a path from concept to reality,” in 2015 design, automation and test in europe conference and exhibition (DATE) (Grenoble, France), 1197–1202.
Upadhyay, N. K., Jiang, H., Wang, Z., Asapu, S., Xia, Q., and Yang, J. J. (2019). Emerging memory devices for neuromorphic computing. Adv. Mater. Technol. 4, 1800589. doi:10.1002/admt.201800589
Valeriani, D., Santoro, F., and Ienca, M. (2022). The present and future of neural interfaces. Front. Neurorobotics 16, 953968. doi:10.3389/fnbot.2022.953968
Zhang, W., Gao, B., Tang, J., Yao, P., Yu, S., Chang, M.-F., et al. (2020). Neuro-inspired computing chips. Nat. Electron. 3, 371–382. doi:10.1038/s41928-020-0435-7
Keywords: nanodevice, application, next-generation electronics, innovations, nanoelectronics
Citation: Hu Y (2025) Application-driven innovations in nanodevices for next-generation transistors, neuromorphic computing, neural interface and quantum computing. Front. Nanotechnol. 7:1583483. doi: 10.3389/fnano.2025.1583483
Received: 25 February 2025; Accepted: 31 March 2025;
Published: 10 April 2025.
Edited by:
Bingqing Wei, University of Delaware, United StatesReviewed by:
Rafal Sliz, University of Oulu, FinlandCopyright © 2025 Hu. This is an open-access article distributed under the terms of the Creative Commons Attribution License (CC BY). The use, distribution or reproduction in other forums is permitted, provided the original author(s) and the copyright owner(s) are credited and that the original publication in this journal is cited, in accordance with accepted academic practice. No use, distribution or reproduction is permitted which does not comply with these terms.
*Correspondence: Youfan Hu, eW91ZmFuaHVAcGt1LmVkdS5jbg==
Disclaimer: All claims expressed in this article are solely those of the authors and do not necessarily represent those of their affiliated organizations, or those of the publisher, the editors and the reviewers. Any product that may be evaluated in this article or claim that may be made by its manufacturer is not guaranteed or endorsed by the publisher.
Research integrity at Frontiers
Learn more about the work of our research integrity team to safeguard the quality of each article we publish.