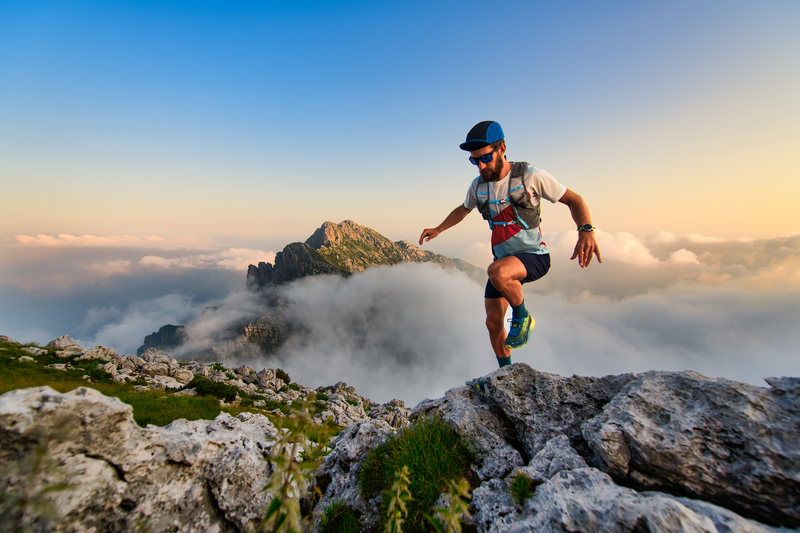
94% of researchers rate our articles as excellent or good
Learn more about the work of our research integrity team to safeguard the quality of each article we publish.
Find out more
PERSPECTIVE article
Front. Nanotechnol.
Sec. Nanomaterials
Volume 7 - 2025 | doi: 10.3389/fnano.2025.1559053
The final, formatted version of the article will be published soon.
You have multiple emails registered with Frontiers:
Please enter your email address:
If you already have an account, please login
You don't have a Frontiers account ? You can register here
Applications of nanotechnology have rapidly expanded across various fields, including materials, energy, medicine, and the environment. The toxicity of nanomaterials (NMs) and nanotechnology to living organisms, as well as potential negative environmental impacts, poses significant challenges. Laboratory approaches for assessing the negative impacts of NMs are costly, time-consuming, and frequently fall behind the development of novel materials. Therefore, intelligent systems of informatics to forecast their toxicity potentials are a possible alternative option. Research on nanotoxicology has generated extensive and diverse datasets. However, data alone does not equate to information. Since little is known about how to extract meaningful information from large streams of data, a broad discussion of potential concerns still exists. In this regard, we present a perspective on how the “big data” can change the paradigm toward data-centric computational materials research to decipher the toxicity mechanisms of NMs for their efficient risk assessment management to provide safe-by-design standards for the sustainable advancement of nanotechnology. We will also discuss the challenges of the current data-driven research into the nano realm.
Keywords: nanomaterials, Computational toxicity, artificial intelligence, machine learning, algorithm
Received: 11 Jan 2025; Accepted: 19 Feb 2025.
Copyright: © 2025 Saha. This is an open-access article distributed under the terms of the Creative Commons Attribution License (CC BY). The use, distribution or reproduction in other forums is permitted, provided the original author(s) or licensor are credited and that the original publication in this journal is cited, in accordance with accepted academic practice. No use, distribution or reproduction is permitted which does not comply with these terms.
* Correspondence:
Sarmistha Saha, GLA University, Mathura, India
Disclaimer: All claims expressed in this article are solely those of the authors and do not necessarily represent those of their affiliated organizations, or those of the publisher, the editors and the reviewers. Any product that may be evaluated in this article or claim that may be made by its manufacturer is not guaranteed or endorsed by the publisher.
Research integrity at Frontiers
Learn more about the work of our research integrity team to safeguard the quality of each article we publish.