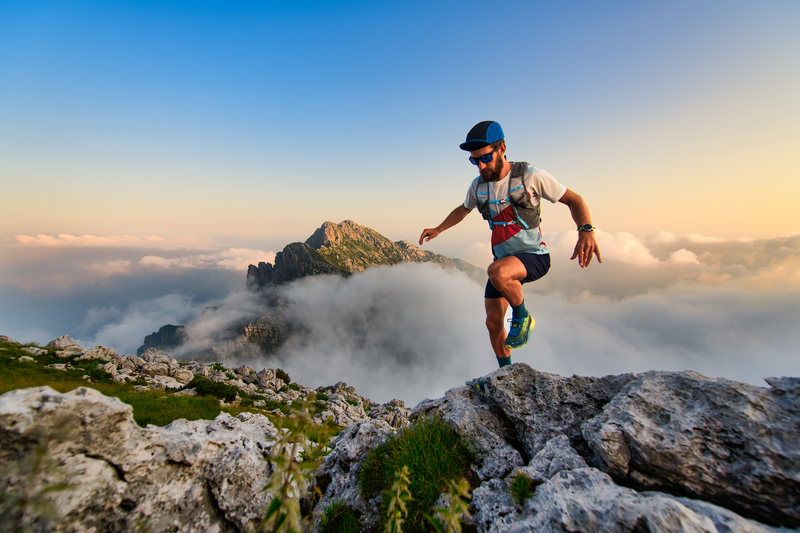
95% of researchers rate our articles as excellent or good
Learn more about the work of our research integrity team to safeguard the quality of each article we publish.
Find out more
ORIGINAL RESEARCH article
Front. Nanotechnol. , 07 January 2025
Sec. Nanoelectronics
Volume 6 - 2024 | https://doi.org/10.3389/fnano.2024.1505751
This article is part of the Research Topic Two-Dimensional Materials for Nanoelectronics View all articles
Surface plasmon resonance (SPR) biosensors are powerful tools for highly sensitive and specific detection of biomolecules. This study introduces a MoS₂-based SPR biosensor optimized for SARS-CoV-2 detection. The sensor integrates a multilayer configuration, including a BK7 prism, Ag film (45 nm), S₃N₄ layer (13 nm), MoS₂ monolayer (0.65 nm), and functionalized ssDNA layer (5 nm). Systematic optimization of each layer improved plasmonic coupling, propagation, and specificity, achieving a balance between sensitivity, resolution, and efficiency. The optimized biosensor was evaluated across virus concentrations ranging from 0.01 to 150 mM. The proposed biosensor demonstrated excellent performance at moderate to high concentrations, with sensitivity up to 261.33°/RIU, a quality factor of 36.16 RIU−1, and a limit of detection of 1.91 × 10−5. An optimal figure of merit of 405.50 RIU−1 was achieved at 10 mM, highlighting the sensor’s diagnostic potential. However, challenges remain at very low concentrations (0.01–0.1 mM), where angular shifts, sensitivity, and signal-to-noise ratio were negligible.
The COVID-19 (coronavirus disease 2019) pandemic, caused by the SARS-CoV-2 virus (Yang et al., 2024), has underlined the global need for advanced diagnostic tools that can address the growing challenges associated with rapid detection and monitoring (Liu et al., 2024). SARS-CoV-2, a single-stranded RNA (ribonucleic acid) virus with a characteristic spike protein that binds to the ACE2 receptor in human cells (Erkihun et al., 2024), has demonstrated unprecedented transmissibility and adaptability. Early and accurate detection of this virus is essential to prevent its spread and monitor emerging variants (Zhu et al., 2024), which may impact public health interventions and the efficacy of vaccines and treatments. While molecular techniques such as reverse transcription polymerase chain reaction (RT-PCR) remain the benchmark for sensitivity and specificity (Sajal et al., 2024), their dependence on centralized laboratories, skilled personnel, and specialized equipment makes them less suitable for widespread, decentralized testing. These limitations have motivated the exploration of alternative methods (Huggett et al., 2021), including biosensor-based platforms, to complement existing molecular diagnostics.
Biosensors offer a compelling solution to the limitations of conventional methods by enabling label-free, real-time, and highly sensitive detection of viral biomarkers such as RNA (Iravani, 2020), antigens (Cerutti et al., 2020), or antibodies (Drobysh et al., 2024). These devices integrate a biological recognition element, such as antibodies, aptamers, or nucleic acids, with a physical transducer to convert biological interactions into quantifiable signals (Justino et al., 2015). Surface plasmon resonance (SPR)-based biosensors (Tene et al., 2024a), in particular, have emerged as a promising technology for virus detection due to their high sensitivity to refractive index changes at the sensor surface (Patel and Parmar, 2021). SPR sensors exploit the resonant oscillation of free electrons at the interface between a metal and a dielectric medium (Tene et al., 2023a) under specific light conditions. These sensors not only allow the detection of viral components without the need for labeling but also provide real-time data, which is crucial in clinical and point-of-care settings.
It is important to remark that field-effect transistor (FET) modules have been widely explored for COVID-19 sensing due to their high sensitivity and rapid detection capabilities, leveraging the exceptional electronic properties of low-dimensional materials (Sengupta and Hussain, 2021), such as high carrier mobility and tunable bandgap. However, FET sensors often face challenges related to stability, signal drift, and the complexity of surface functionalization, which can limit their scalability for practical applications.
Recent advances in SPR biosensors have focused on integrating nanomaterials, particularly two-dimensional (2D) materials (Pisarra et al., 2022), to enhance sensitivity and specificity. 2D materials, such as graphene/graphene derivatives (Tene et al., 2023b) and transition metal dichalcogenides (TMDs) like molybdenum disulfide (MoS₂) (Ghodrati et al., 2024), exhibit unique physicochemical properties that make them attractive for biosensing applications. MoS₂, for instance, combines a high surface-to-volume ratio, strong light-matter interaction, and excellent electronic properties (Li and Zhu, 2015), which are critical for amplifying the sensor’s response to biomolecular interactions. Functionalized MoS₂ surfaces can immobilize biomolecules such as single-stranded DNA (ssDNA) or aptamers with high stability and specificity (Haque and Rouf, 2021), enabling the detection of SARS-CoV-2 RNA or spike proteins with interesting precision.
Despite the progress in integrating 2D materials into SPR biosensors, challenges remain in optimizing the multilayer configurations required for high-performance sensors. While prior studies have explored the use of graphene-based materials (Sindona et al., 2019) or hybrid structures (Cai et al., 2022) to enhance plasmonic properties, they often rely on fixed material dimensions and do not systematically investigate the impact of layer thickness on sensor performance. For example, a recent study proposed a graphene-coated SPR biosensor using PtSe₂ to enhance sensitivity (Akib et al., 2021), achieving promising results through numerical simulations. However, this approach did not include experimental approach or a detailed exploration of how variations in layer thickness influence key performance metrics such as sensitivity, full-width at half maximum (FWHM), and figure of merit (FoM).
In this context, our work aims to address these gaps by presenting a systematic optimization of a MoS₂-based SPR biosensor for SARS-CoV-2 detection at different concentrations. The sensor comprises a multilayer architecture including a silver (Ag) metallic layer, a silicon nitride (Si₃N₄) dielectric spacer, and a functionalized MoS₂ layer immobilized with thiol-tethered ssDNA. By varying the thickness of each layer, we comprehensively evaluate physical and performance metrics, including attenuation, FWHM, angle shift variation, sensitivity to refractive index changes, detection accuracy, quality factor (QF), FoM, limit of detection (LoD), and signal-to-noise ratio (SNR). This approach allows us to systematically understand the interplay between layer thickness, material properties, and sensor performance, providing critical insights for designing SPR biosensors with enhanced detection capabilities.
Hence, by tailoring each layer of the sensor to achieve optimal performance, this study meaningfully advances the capabilities of SPR biosensors for SARS-CoV-2 detection. Beyond the immediate application to COVID-19 diagnostics, the findings of this study provide a versatile framework for developing biosensors targeting other viral pathogens. This work demonstrates the potential of SPR technology for addressing current diagnostic challenges and paves the way for scalable, rapid, and accurate detection platforms that can play a key role in future pandemic preparedness.
The proposed biosensor, illustrated in Figure 1, leverages a multilayered architecture integrated into a SPR configuration based on the Kretschmann prism coupling method (Zheng et al., 2024). At the sensor’s core lies the BK-7 prism, which serves as the substrate and optical foundation. The prism plays a critical role in coupling incident light into the system, enabling the excitation of surface plasmons at the metal-dielectric interface. By directing polarized light at a specific angle (θ), the prism facilitates the resonance condition required for SPR, where a sharp dip in reflected light intensity occurs.
Figure 1. Illustration of the proposed biosensor for virus detection by using the surface plasmon resonance (SPR) approach.
Above the prism is the Ag layer, which forms the metal-dielectric interface crucial for surface plasmon generation. Silver is chosen due to its exceptional plasmonic properties (Naik et al., 2013), including low propagation losses and strong plasmon resonance. This layer amplifies the sensor’s sensitivity to subtle refractive index changes induced by biomolecular interactions. To enhance the propagation of surface plasmons and improve the adhesion between the Ag layer and subsequent functional layers, a Si₃N₄ layer is incorporated as a dielectric spacer (Mudgal et al., 2020). The Si₃N₄ layer is not only a structural component but also a functional one, as its thickness directly influences key optical parameters, such as the FWHM of the resonance curve and the angle shift variation (Tene et al., 2024b). By systematically varying the thickness of the Si₃N₄ layer, the SPR angle is fine-tuned to maximize sensitivity and SNR.
Above the dielectric spacer lies the MoS₂ layer, a 2D material that meaningfully enhances the sensor’s functionality. MoS₂ amplifies the local refractive index changes upon biomolecular binding. Its high refractive index further enhances the SPR signal, while its surface chemistry allows for robust functionalization. In this design, the MoS₂ layer is functionalized with thiol groups, enabling the covalent attachment of ssDNA probes. These probes are designed to specifically hybridize with SARS-CoV-2 RNA, making the biosensor highly selective for viral detection.
The topmost layer, consisting of thiol-tethered ssDNA, serves as the biological recognition element of the sensor. The thiol groups provide a stable attachment to the MoS₂ layer, while the ssDNA sequences offer high specificity for complementary SARS-CoV-2 RNA targets. When the viral RNA binds to the ssDNA, it induces a localized change in the refractive index at the sensor surface. This change is translated into an SPR angle shift (Figure 1), which is detected by the optical system. The specificity of the thiol-tethered ssDNA ensures that the biosensor is not only sensitive but also selective, minimizing false-positive signals from non-target molecules.
A numerical analysis is employed to calculate the reflectance curve using the transfer matrix method (TMM) and Fresnel equations, as described in (Tene et al., 2024c; Varasteanu, 2020). This approach allows for the systematic modeling of light propagation through a multilayer system by considering the optical properties and thickness of each layer. The thickness of each layer is defined along the perpendicular axis (z-axis), which is the direction normal to the plane of the layers. The optical behavior at the interfaces between adjacent layers is governed by boundary conditions that ensure the continuity of the electric and magnetic field components of the electromagnetic wave.
The boundary conditions are applied at the interfaces to connect the electromagnetic fields across each layer. At the first layer, located at
The Z-coordinate represents the position along the thickness of the multilayer structure, with
Then, the transfer matrix describes the relationship between the tangential components of the electric and magnetic fields as:
where,
And
here,
And
where,
by using Equation 6 the SPR curve as a function of the angle of incidence is computed. To analyze the performance of the biosensor is necessary to consider the following metrics. The sensitivity of the biosensors (
For biosensor optimization, we focus on the sensitivity enhancement (
The sensitivity to the refractive index change can be expressed as:
The parameter
Quality factor (QF) can be expressed in terms of
In addition, to compute the FoM, LoD, and SNR, the related equations can be expressed as (Uddin et al., 2021; Hu et al., 2021):
where,
Supplementary Table S1 illustrates the systematic progression of the systems considered in this work to optimize the SPR biosensor for SARS-CoV-2 detection. It provides a overview of the incremental changes made to the sensor’s architecture, ranging from the baseline configuration (Sys₀) to the fully functional systems incorporating advanced materials and specific biorecognition elements (Sys₉). The baseline system, Sys₀, consists of the simplest architecture: a prism, a silver layer, and water as the sensing medium. This configuration serves as a starting point for computing sensitivity enhancements across more complex systems. In Sys₁, the sensing medium is changed to phosphate-buffered saline (PBS), a biologically relevant environment, to bring the system closer to realistic conditions without altering the structural layers.
From Sys₂ onward, additional layers are incrementally introduced to evaluate their contribution to the biosensor’s functionality. Each subsequent configuration builds upon the previous one by incorporating either Si₃N₄, MoS₂, or thiol-tethered ssDNA. For instance, Sys₂ introduces Si₃N₄ to improve plasmonic performance, while Sys₃ functionalizes this layer with ssDNA to add selectivity for detecting viral RNA. Similarly, Sys₄ and Sys₅ explore the standalone and functionalized use of MoS₂, respectively. More advanced configurations, such as Sys₆, Sys₇, Sys₈, and Sys₉, involve combinations of Si₃N₄ and MoS₂ in various arrangements, with or without functionalization.
On the other hand, Supplementary Table S2 provides the initial parameters used for the SPR biosensor configurations investigated in this study. These parameters show the refractive index and thickness of each material layer, along with references to the experimental or theoretical studies from which these values were derived (Su et al., 2013; Luke et al., 2015; Kumar et al., 2022; Akib et al., 2024). The BK-7 prism, which forms the substrate of the SPR biosensor, has a refractive index of 1.5151. However, its thickness is not reported because, in the numerical modeling approach used here, the prism is treated as an infinite medium for light propagation. This assumption simplifies the simulation process while maintaining the accuracy of the results, as the thickness of the prism does not directly influence the excitation of surface plasmons at the metal-dielectric interface. Similarly, the thickness values for the sensing media, i.e., water and PBS, are not included. In these cases, the sensing medium is considered a semi-infinite layer in the numerical calculations, as its role is to provide the refractive index environment above the biosensor’s active layers. This treatment aligns with the typical approach in SPR modeling (Srivastava et al., 2020).
The Ag layer, with a refractive index of 0.056253 + 4.2760i, has a well-defined thickness of 55 nm. This thickness is optimized to achieve strong surface plasmon resonance and is consistent with values reported in the literature for SPR biosensors. The Si₃N₄ layer, with a refractive index of 2.0394 and a thickness of 5 nm, serves as a dielectric spacer. Its parameters are carefully chosen to enhance the propagation of surface plasmons and improve the adhesion of subsequent layers. The MoS₂ layer has a refractive index of 5.0805 + 1.1723i and a thickness of 0.65 nm, corresponding to a monolayer. These values are derived from theoretical studies that accurately model the optical properties of MoS₂ for plasmonic applications. Lastly, the thiol-tethered ssDNA layer has a refractive index of 1.462 and a thickness of 3.2 nm, which corresponds to a typical monolayer of DNA immobilized on a substrate. As stated, this layer provides the biosensor with its biochemical specificity for detecting SARS-CoV-2 RNA.
The results presented in Figure 2 and Supplementary Table S3 provide a detailed evaluation of the physical and performance metrics of the SPR biosensor configurations, systematically comparing their performance across different architectures. The SPR reflectance curves (Figure 2A) highlight the changes in resonance behavior caused by the incremental addition of functional layers, while the numerical metrics of attenuation, FWHM, and sensitivity enhancement (Supplementary Table S3) quantify the effects of these modifications. This comprehensive analysis is key to identifying the optimal configuration that balances plasmonic interaction, resonance resolution, and sensitivity.
Figure 2. (A) SPR reflectance curves plotted as a function of the incidence angle for the configurations investigated in this study: Sys0 in water and Sys1–Sys9 in a PBS solution. (B) Percentage attenuation of reflectance at the resonance dip for each configuration. (C) Full Width at Half Maximum (FWHM) of the resonance curve. (D) Percentage enhancement in sensitivity across all configurations, taking as the baseline, Sys0.
The SPR curves (Figures 2A, B) reveal how each system responds to changes in the angle of incidence. Sys₀, the baseline configuration in water, demonstrates a sharp and narrow resonance with minimal attenuation (0.023%), reflecting its simple and loss-minimized design. However, as additional layers are incorporated in Sys₁ through Sys₉, the resonance depth increases, accompanied by broader curves and higher attenuation values. This trend is particularly pronounced in Sys₄ to Sys₈, where the integration of MoS2 and ssDNA meaningfully enhances plasmonic interaction. While increased attenuation (peaking at 20.55% in Sys₈) indicates stronger interaction with the functional layers, it must be balanced to prevent excessive broadening of the resonance curve, which could compromise detection resolution.
The broadening of the resonance is quantitatively captured by the FWHM values (Figure 2C), which increase as the system evolves from Sys₀ to Sys₉. The baseline system exhibits the narrowest FWHM (0.88°), indicative of high-resolution performance but limited interaction with the sensing environment. As more layers are added, the FWHM values rise, reaching 2.97° in Sys₈, the widest among the configurations. While broader FWHM can reduce the precision of resonance peak detection, in this context, it reflects the enhanced plasmonic response due to the optimized multilayer structure. This trade-off is critical, as the broadening is accompanied by significant sensitivity gains, underlining the benefits of the multilayer architecture.
The sensitivity enhancement, shown in Figure 2D, provides a direct measure of the biosensor’s ability to detect refractive index changes. Relative to the baseline Sys₀, sensitivity enhancement increases consistently across the configurations, with the highest value observed in Sys₈ (7.50%). This result highlights the effectiveness of the combination of silicon nitride, molybdenum disulfide, and thiol-tethered DNA in optimizing the sensor’s optical and biochemical interactions. The role of each layer in enhancing the refractive index contrast and enabling specific biomolecular binding is evident in the important performance gains of Sys₈ compared to earlier systems. While Sys₉ achieves a similar sensitivity (7.29%), its marginally reduced enhancement compared to Sys₈ highlights the importance of layer arrangement and thickness optimization.
The results in Figure 3 and Supplementary Table S4 describe the impact of varying Ag thickness on the performance of the Sys₈ configuration. The SPR reflectance curves (Figures 3A, B) reveal a clear relationship between Ag thickness and resonance behavior. For thinner layers, such as 40 nm, the resonance dip is shallow, with an attenuation of 3.40%. This low attenuation indicates weaker plasmonic interaction and less effective light coupling. As the Ag thickness increases, the resonance dip becomes deeper, accompanied by a rise in attenuation, which reaches 53.13% for 65 nm. However, thicker layers also introduce substantial optical losses, which reduce the efficiency of the system. At 45 nm, attenuation is minimized at 0.17%, providing an effective balance between plasmonic coupling and energy conservation.
Figure 3. (A) SPR reflectance curves plotted as a function of the silver thickness: Agbase in water (with the original parameters from Supplementary Table S1) and Ag40–Ag65 in a PBS solution. (B) Percentage attenuation of reflectance at the resonance dip for each silver thickness. (C) Full Width at Half Maximum (FWHM) of the resonance curve. (D) Percentage enhancement in sensitivity across all thickness values, taking as the baseline, Agbase.
The FWHM values (Figure 3C) provide insight into the sharpness of the resonance peaks. At 40 nm, the FWHM is the widest, measuring 4.56°, indicating poor resolution and diffuse resonance behavior. Increasing the thickness to 45 nm narrows the FWHM to 3.79°, a significant improvement in resolution compared to thinner layers. Beyond 45 nm, the narrowing trend becomes less pronounced, stabilizing around 2.81° at 65 nm. The narrowing of FWHM with increased thickness reflects better optical resolution, though the limited improvements at higher thicknesses do not justify the trade-offs in energy loss and attenuation.
Sensitivity enhancement (Figure 3D) further emphasizes the performance improvements achieved with increasing Ag thickness. At 40 nm, sensitivity enhancement is measured at 0.58%, reflecting the reduced interaction between the plasmonic layer and the sensing medium. This value increases steadily with thickness, peaking at 0.86% for 65 nm. However, the incremental gains between 45 nm (0.67%) and 65 nm do not compensate for the higher attenuation and reduced resolution benefits. At 45 nm, sensitivity enhancement is sufficient for effective detection, while the low attenuation and improved resolution offer a more balanced performance. Hence, the selection of 45 nm as the optimal Ag thickness is supported by its ability to balance all critical performance metrics. The FWHM at 45 nm reflects improved resolution without the unnecessary broadening seen at lower thicknesses. Furthermore, its sensitivity enhancement, while not the highest, is competitive and provides reliable detection without the drawbacks associated with thicker layers.
The results in Figure 4 and Supplementary Table S5 examine the impact of varying the thickness of S₃N₄ on the performance of the Sys₈ configuration. The SPR reflectance curves (Figures 4A,B) demonstrate the effect of S₃N₄ thickness on the resonance behavior. At a thickness of 5 nm, the resonance dip is sharp but shallow, corresponding to an attenuation of 0.17%. This low attenuation indicates limited interaction between the plasmonic layer and the dielectric interface, reducing the system’s ability to effectively respond to refractive index changes. As the thickness increases, the resonance dip becomes more pronounced, peaking at 89.80% for 20 nm. However, such high attenuation introduces excessive optical losses, which can degrade signal response. At 13 nm, attenuation is moderate at 6.99%, providing a balanced interaction that enhances plasmonic coupling without significant energy dissipation.
Figure 4. (A) SPR reflectance curves plotted as a function of the silicon nitride thickness: S3N4_base in water (with the optimized Ag thickness and original parameters from Supplementary Table S1) and S3N4_5nm–S3N4_20nm in a PBS solution. (B) Percentage attenuation of reflectance at the resonance dip for each silicon nitride thickness. (C) Full Width at Half Maximum (FWHM) of the resonance curve. (D) Percentage enhancement in sensitivity across all thickness values, taking as the baseline, S3N4_base.
The FWHM values (Figure 4C) offer further insight into the sharpness of the resonance peaks. At 5 nm, the FWHM is narrow, measuring 3.89°, reflecting high resolution but limited plasmonic response. As the thickness increases, the FWHM widens progressively, reaching 14.32° at 20 nm. This widening indicates reduced precision in detecting resonance shifts, which can impact the sensor’s ability to resolve small refractive index changes. A thickness of 13 nm achieves a FWHM of 6.76°, representing an optimal trade-off between maintaining sufficient resolution and accommodating the broader resonance needed for enhanced sensitivity.
Sensitivity enhancement (Figure 4D) highlights the sensor’s performance in detecting refractive index changes. At 5 nm, the enhancement is minimal (0.80%), reflecting limited sensitivity due to the reduced interaction at the interface. Sensitivity increases steadily with thickness, peaking at 17.64% for 15 nm before slightly declining to 16.42% at 20 nm. While thicker layers improve sensitivity, the diminishing returns beyond 13 nm, coupled with the rapid increase in attenuation and FWHM, make thicker configurations less practical for more realistic applications. At 13 nm, the sensitivity enhancement reaches 12.80%, providing a significant improvement without excessive trade-offs in signal response or resolution. The choice of 13 nm reflects a strategic compromise that maximizes the effectiveness of the Sys₈ configuration, supporting its application in precise and reliable biosensing for SARS-CoV-2 detection and beyond.
The results presented in Figure 5 and Supplementary Table S6 assess the effect of varying the number of MoS₂ layers on the performance of the Sys₈ configuration. The SPR reflectance curves (Figures 5A, B) show that for a single layer, the resonance dip is sharp and well-defined, with attenuation at 6.99%. This moderate attenuation reflects efficient plasmonic interaction without introducing significant optical losses. As the number of layers increases, attenuation rises sharply, reaching 89.66% for six layers. This increase in attenuation indicates excessive energy losses that diminish the signal response, reducing its suitability for practical biosensing applications.
Figure 5. (A) SPR reflectance curves plotted as a function of the number of MoS2 layers: L1base in water (with the optimized Ag/S3N4 thickness and original parameters from Supplementary Table S1) and L1–L6 in a PBS solution. (B) Percentage attenuation of reflectance at the resonance dip for each MoS2 layer. (C) Full Width at Half Maximum (FWHM) of the resonance curve. (D) Percentage enhancement in sensitivity across all thickness values, taking as the baseline, L1base.
The FWHM values (Figure 5C) further highlight the effect of additional layers. For a single layer, the FWHM is narrow at 6.76°, providing precise resolution and clear detection of resonance shifts. However, as the number of layers increases, the FWHM broadens significantly, reaching 16.64° for six layers. This loss of resolution impacts the sensor’s ability to accurately detect small refractive index changes. The narrow resonance peak observed with a single layer supports higher detection precision, making it the most effective option for applications requiring accuracy.
Sensitivity enhancement (Figure 5D) reflects how the number of MoS₂ layers influences the sensor’s ability to detect refractive index changes. In this context, two layers achieve the highest sensitivity enhancement (4.71%), but this comes at the cost of higher attenuation (45.50%) and a wider FWHM (9.61°). Beyond two layers, sensitivity enhancement declines steadily, reaching only 0.44% for six layers. Although two layers show a slight improvement in sensitivity compared to a single layer, the significant trade-offs in attenuation and resolution reduce their overall viability. The single-layer configuration provides sufficient sensitivity (1.19%) while maintaining superior resolution and lower attenuation. Then, these findings demonstrate that a single MoS₂ layer provides the best balance of performance metrics, making it the most effective choice for the Sys₈ configuration.
The results in Figure 6 and Supplementary Table S7 evaluate the effect of varying ssDNA thickness on the performance of the Sys₈ configuration. The SPR reflectance curves (Figures 6A, B) demonstrate the changes in resonance behavior as the ssDNA thickness increases. For a baseline thickness of 3.2 nm, the resonance dip is sharp and well-defined, with an attenuation of 6.99%. This reflects moderate plasmonic interaction and low optical losses. As the thickness increases, the resonance dip deepens significantly, with attenuation peaking at 94.27% for 50 nm. Such high attenuation corresponds to excessive energy losses, reducing signal response and compromising biosensing performance. At 5 nm, attenuation is still low at 8.56%, providing efficient plasmonic coupling without substantial optical losses.
Figure 6. (A) SPR reflectance curves plotted as a function of the ssDNA thickness: ssDNA3.2nm_base in water (with the optimized Ag/S3N4/MoS2 thickness and original parameters from Supplementary Table S1) and ssDNA3.2nm–ssDNA50nm in a PBS solution. (B) Percentage attenuation of reflectance at the resonance dip for each ssDNA layer. (C) Full Width at Half Maximum (FWHM) of the resonance curve. (D) Percentage enhancement in sensitivity across all thickness values, taking as the baseline, ssDNA3.2nm_base.
The FWHM values (Figure 6C) reflect how the resonance sharpness evolves with increasing ssDNA thickness. At 3.2 nm, the FWHM is 6.76°, representing high resolution and precise detection of resonance shifts. However, as the thickness increases, the FWHM broadens, reaching 19.11° at 50 nm. This loss of resolution reduces the sensor’s ability to detect subtle refractive index changes. At 5 nm, the FWHM increases slightly to 6.92°, maintaining a strong balance between resolution and enhanced plasmonic interaction.
Sensitivity enhancement (Figure 6D) shows a steady increase with thicker ssDNA layers, peaking at 6.90% for 20 nm. At 5 nm, the sensitivity enhancement is 2.06%, a noticeable improvement over the baseline thickness of 3.2 nm. Beyond 5 nm, the higher sensitivity values are counterbalanced by the rapid rise in attenuation and broadening of the FWHM. This trade-off limits the practical applicability of thicker ssDNA layers despite their higher sensitivity. Indeed, at a thickness of 5 nm, the ssDNA layer provides a balance that minimizes energy losses, preserves adequate resolution, and ensures reliable sensitivity enhancement. This configuration avoids the excessive attenuation and reduced resolution associated with thicker layers while still offering improved biosensing capabilities.
To emphasize, Supplementary Table S8 presents the optimized parameters of Sys₈ following the detailed analysis of each layer’s thickness. These parameters, which include refractive index and thickness values, represent the final configuration of the SPR biosensor for effective detection of SARS-CoV-2 in PBS. The results also include the refractive index changes corresponding to different virus concentrations, based on experimental observations reported by Kumar et al. (2022), who observed a linear relationship between virus concentration and refractive index.
Hence, the optimized configuration of Sys₈ consists of a BK7 prism as the substrate, which has a refractive index of 1.5151. This serves as the base for the plasmonic structure. The Ag layer, with a thickness of 45 nm, was selected for its superior balance of attenuation, FWHM, and sensitivity enhancement. The S₃N₄ layer has an optimized thickness of 13 nm, contributing to enhanced plasmon propagation and improved resonance behavior. The MoS2 layer has a thickness of 0.65 nm, corresponding to a monolayer. Its refractive index is 2.7611 + 1.6987i, which ensures efficient light-matter interaction, further enhancing the system’s sensitivity. The ssDNA layer, which could be functionalized for specific binding to SARS-CoV-2 RNA, has a thickness of 5 nm and a refractive index of 1.462.
The PBS medium, with a refractive index of 1.334, serves as the surrounding environment for detecting the target analyte. We point out that the refractive index values of SARS-CoV-2 in PBS range from 1.340 for a virus concentration of 150 mM to 1.355 for 525 mM. However, the concentrations reported in the experimental study (Kumar et al., 2022), ranging from 150 to 525 mM, are not representative of realistic physiological conditions, where virus concentrations are typically much lower. To address this limitation and enable the realistic testing of the proposed Sys₈ biosensor, we extended the linear relationship to extrapolate the refractive index of SARS-CoV-2 in PBS for concentrations ranging from 0.01 mM to 150 mM (Supplementary Table S9). This extrapolation allows us to simulate and evaluate the biosensor’s performance under more plausible scenarios, providing a deeper understanding of its sensitivity and detection capability in practical applications.
We now move to the focus of the present work, say, the performance of the optimized Sys₈ biosensor was evaluated across SARS-CoV-2 concentrations ranging from 0.01 to 150 mM in PBS, as shown in Figure 7 and Supplementary Table S9. At baseline (UEJTQDAuMA== mM), the SPR reflectance curve shows a shallow resonance dip, reflecting the absence of analyte interaction (Figure 7A). As virus concentration increases, the resonance dip deepens progressively, with noticeable angular shifts at higher concentrations (50–150 mM). While the shifts at lower concentrations (0.01–0.1 mM) are minimal, the biosensor begins to demonstrate a measurable response starting at 1.0 mM, where sensitivity enhancement reaches 0.01% (discussed below). This indicates the biosensor’s capability to detect changes in refractive index at detectable levels while still being limited in resolving extremely dilute samples.
Figure 7. (A) SPR reflectance curves plotted as a function of the virus concentration: UEJTQDAuMA== mM in water (with the optimized Ag/S3N4/MoS2/ssDNA thickness) and UEJTQDAuMDEmI3gwMjAxMzs=; PBS@150 mM in a PBS solution. (B) Percentage attenuation of reflectance at the resonance dip for each virus concentration. (C) Full Width at Half Maximum (FWHM) of the resonance curve. (D) Percentage enhancement in sensitivity across all thickness values, taking as the baseline, UEJTQDAuMA== mM.
The attenuation values (Figure 7B) highlight the sensor’s performance in preserving signal response. At concentrations below 10 mM, attenuation remains relatively low, ranging between 8.56% and 8.82%, which supports minimal optical losses and efficient plasmonic coupling. However, for higher concentrations such as 50 mM and 150 mM, attenuation increases substantially, reaching 14.06% at 150 mM. This rise in attenuation reflects stronger plasmonic interaction with the analyte but introduces optical losses that may impact precision at elevated analyte concentrations.
The FWHM (Figure 7C) remains consistent across lower concentrations, ranging between 6.97° and 6.99° up to 10 mM. This stability highlights the sensor’s ability to maintain resolution for detecting subtle refractive index changes. At higher concentrations, such as 150 mM, the FWHM broadens slightly to 7.23°, indicating a gradual decline in resolution. While the broadening is not drastic, it points to the inherent trade-off between sensitivity and resolution as analyte concentrations increase.
The sensitivity enhancement (Figure 7D) further reinforces the biosensor’s behavior at varying concentrations. At the lowest concentrations (0.01 and 0.1 mM), sensitivity enhancement remains negligible. The biosensor begins to exhibit measurable sensitivity at 1.0 mM, with an enhancement of 0.01%. As the virus concentration increases, the sensitivity improves significantly, reaching 1.92% at 150 mM. This trend highlights the biosensor’s effectiveness in detecting moderate to high concentrations of the virus, although the minimal enhancement at very low concentrations indicates limitations in resolving trace amounts of the target analyte.
The Sys₈ biosensor offers several strengths, including low attenuation and consistent FWHM at lower concentrations, which ensure efficient plasmonic coupling and high resolution. The measurable sensitivity response beginning at 1.0 mM and its significant improvement at higher concentrations confirm its utility for detecting moderate to elevated virus levels. However, the limited sensitivity enhancement at concentrations below 1.0 mM and the increased attenuation at higher concentrations highlight areas for potential refinement.
Here, the performance evaluation of the optimized Sys₈ biosensor focuses on angular shift (Δθ), sensitivity to refractive index changes (S), detection accuracy (DA), and quality factor (QF). These metrics, presented in Figure 8 and Supplementary Table S10, provide a detailed understanding of how the biosensor responds to varying concentrations of SARS-CoV-2, ranging from 0.01 to 150 mM. The angular shift, shown in Figure 8A, measures the resonance angle change caused by variations in the refractive index due to the presence of the virus. At very low concentrations (0.01 and 0.1 mM), the Δθ is negligible, indicating limited interaction between the sensor and the analyte at these levels. However, starting at 1.0 mM, the biosensor begins to exhibit a measurable angular shift, with Δθ reaching 0.01°. As the concentration increases to 150 mM, the angular shift rises significantly to 1.57°. This increasing trend demonstrates the biosensor’s capability to effectively detect higher concentrations, although its limited response at lower concentrations highlights the challenge of detecting trace amounts of the virus.
Figure 8. Performance metrics of the optimized biosensor evaluated across varying virus concentrations: (A) Angular shift, (B) Sensitivity to refractive index variations, (C) Detection Accuracy (DA), and (D) Quality Factor (QF).
The sensitivity, quantified in Figure 8B, reflects the angular shift per refractive index unit (
The detection accuracy, as shown in Figure 8C, is a measure of the precision of the biosensor in detecting angular shifts. For virus concentrations below 1.0 mM, the DA remains negligible due to the absence of measurable angular shifts. At 1.0 mM, the DA improves to 0.001, increasing progressively to 0.217 at 150 mM. This trend confirms the biosensor’s reliability in detecting moderate to high analyte levels while indicating the need for enhancements to improve accuracy at very low concentrations.
The quality factor, displayed in Figure 8D, evaluates the efficiency of the biosensor by combining sensitivity and resolution. For concentrations below 1.0 mM, the QF is negligible, consistent with the zero angular shifts and sensitivity observed at these levels. At 1.0 mM, the QF reaches 28.68 RIU−1, and it increases steadily with higher concentrations, peaking at 36.16 RIU−1 for 150 mM. The consistent improvement in QF with rising concentrations demonstrates the biosensor’s robustness for detecting substantial analyte levels, where the combination of high sensitivity and reasonable resolution enhances its total effectiveness.
The results collectively show that the Sys₈ biosensor performs well for moderate to elevated virus concentrations, demonstrating measurable angular shifts, high sensitivity, improved detection accuracy, and strong quality factors. However, its limited response at very low concentrations (0.01–0.1 mM) highlights a challenge in detecting trace amounts of the analyte, which is critical for early diagnostic applications. Addressing this limitation would significantly enhance the sensor’s applicability for realistic scenarios where low analyte concentrations are common.
The performance of the optimized Sys₈ biosensor is further analyzed through the metrics of Figure of Merit (FoM), Limit of Detection (LoD), and Signal-to-Noise Ratio (SNR), as presented in Figure 9 and Supplementary Table S11. The FoM, shown in Figure 9A, captures the efficiency of the biosensor by combining sensitivity and resolution into a single metric. For the lowest concentrations (0.01 and 0.1 mM), the FoM remains at 0.0 RIU−1, reflecting the negligible angular shifts and sensitivity observed at these levels. The FoM rises significantly at 1.0 mM, reaching 333.97 RIU−1, marking a clear improvement in the biosensor’s ability to detect measurable refractive index changes. The performance peaks at 10 mM, where the FoM attains its maximum value of 405.50 RIU−1, indicating the optimal balance between sensitivity and resolution. However, at higher concentrations, such as 150 mM, the FoM decreases to 257.23 RIU−1, suggesting that optical losses and broader resonance peaks at elevated analyte levels begin to affect the sensor’s efficiency.
Figure 9. Performance metrics of the optimized biosensor evaluated across varying virus concentrations: (A) Figure of Merit (FoM), (B) Limit of Detection (LoD), and (C) Signal-to-Noise Ratio (SNR).
The LoD, reported in Figure 9B, highlights the biosensor’s ability to detect minimal analyte concentrations. At 1.0 mM, the LoD is 2.50 × 10−5, demonstrating the biosensor’s capability to detect low concentrations with reasonable precision. As the virus concentration increases, the LoD improves steadily, reaching 1.91 × 10−5 at 150 mM. This consistent trend reflects the sensor’s capacity to detect higher analyte levels with increasing accuracy. However, for concentrations below 1.0 mM, the LoD remains unresponsive, which accentuates a challenge in achieving reliable detection at trace analyte levels critical for early-stage diagnostics.
The SNR, presented in Figure 9C, evaluates the biosensor’s signal reliability relative to background noise. At very low concentrations (0.01 and 0.1 mM), the SNR is negligible (0.0) due to zero angular shifts and weak sensitivity responses. The SNR begins to rise at 1.0 mM, reaching 0.001, and increases progressively with higher concentrations. At 50 mM, the SNR improves to 0.072, and it peaks at 0.217 for 150 mM. This progression demonstrates the biosensor’s ability to produce a more distinguishable and reliable signal as analyte levels increase, but it also highlights its limited performance at very low concentrations where the signal remains indistinguishable from noise.
These results collectively indicate that the Sys₈ biosensor performs remarkably well at moderate to high SARS-CoV-2 concentrations. The high FoM at 10 mM highlights the biosensor’s efficiency in balancing sensitivity and resolution, while the steady improvements in LoD and SNR with increasing concentrations validate its reliability for detecting clinically relevant analyte levels.
In summary, we developed and optimized a MoS₂-based SPR biosensor for SARS-CoV-2 detection, employing a multilayer structure comprising a BK7 prism, Ag film, S₃N₄, MoS₂ monolayer, and a functionalized ssDNA layer. The numerical analysis was performed using the transfer matrix method (TMM), enabling a systematic optimization of the sensor configuration by adjusting layer thicknesses and material properties to maximize sensitivity, resolution, and efficiency.
The optimized sensor (Sys₈) features an Ag layer (45 nm), S₃N₄ layer (13 nm), MoS₂ monolayer (0.65 nm), and ssDNA layer (5 nm). The performance was evaluated across SARS-CoV-2 concentrations ranging from 0.01 to 150 mM. Key metrics such as angular shift, sensitivity, detection accuracy, quality factor, figure of merit, limit of detection, and signal-to-noise ratio were analyzed. The sensor achieved a sensitivity of 261.33°/RIU, a quality factor of 36.16 RIU−1, and a low limit of detection of 1.91 × 10−5. An optimal figure of merit of 405.50 RIU−1 was observed at 10 mM, highlighting the strong diagnostic potential for moderate analyte levels. Hence, this study demonstrates the effectiveness of the MoS₂-based SPR biosensor as a reliable platform for SARS-CoV-2 detection, leveraging advanced material properties and numerical modeling. While the sensor exhibited strong performance at moderate to high concentrations, further optimization is needed to enhance its sensitivity for trace analyte detection. These findings provide a foundation for future advancements in SPR biosensors for infectious disease diagnostics.
The original contributions presented in the study are included in the article/Supplementary Material, further inquiries can be directed to the corresponding author.
TT: Conceptualization, Data curation, Formal Analysis, Funding acquisition, Investigation, Methodology, Project administration, Resources, Software, Supervision, Validation, Visualization, Writing–original draft, Writing–review and editing. GT-U: Conceptualization, Data curation, Formal Analysis, Funding acquisition, Investigation, Methodology, Project administration, Resources, Software, Supervision, Validation, Visualization, Writing–original draft, Writing–review and editing. KG: Conceptualization, Data curation, Formal Analysis, Funding acquisition, Investigation, Methodology, Project administration, Resources, Software, Supervision, Validation, Visualization, Writing–original draft, Writing–review and editing. MM: Conceptualization, Data curation, Formal Analysis, Funding acquisition, Investigation, Methodology, Project administration, Resources, Software, Supervision, Validation, Visualization, Writing–original draft, Writing–review and editing. CG: Conceptualization, Data curation, Formal Analysis, Funding acquisition, Investigation, Methodology, Project administration, Resources, Software, Supervision, Validation, Visualization, Writing–original draft, Writing–review and editing.
The author(s) declare that financial support was received for the research, authorship, and/or publication of this article. This work was funded and supported by Universidad Técnica Particular de Loja under grant No: POA_VIN-56. This work was partially supported by LNF-INFN: Progetto HPSWFOOD Regione Lazio-CUP I35F20000400005.
GT-U, wishes to thank Escuela Superior Politécnica de Chimborazo for their hospitality during the completion of this work.
The authors declare that the research was conducted in the absence of any commercial or financial relationships that could be construed as a potential conflict of interest.
The author(s) declare that no Generative AI was used in the creation of this manuscript.
All claims expressed in this article are solely those of the authors and do not necessarily represent those of their affiliated organizations, or those of the publisher, the editors and the reviewers. Any product that may be evaluated in this article, or claim that may be made by its manufacturer, is not guaranteed or endorsed by the publisher.
The Supplementary Material for this article can be found online at: https://www.frontiersin.org/articles/10.3389/fnano.2024.1505751/full#supplementary-material
Akib, T. B. A., Mou, S. F., Rahman, M. M., Rana, M. M., Islam, M. R., Mehedi, I. M., et al. (2021). Design and numerical analysis of a graphene-coated SPR biosensor for rapid detection of the novel coronavirus. Sensors (Basel). 21, 3491. doi:10.3390/s21103491
Akib, T. B. A., Rana, M. M., and Mehedi, I. M. (2024). Multi-layer SPR biosensor for in-situ amplified monitoring of the SARS-CoV-2 omicron (B.1.1.529) variant. Biosens. Bioelectron. X 16, 100434. doi:10.1016/j.biosx.2023.100434
Cai, H., Wang, M., Wu, Z., Liu, J., and Wang, X. (2022). Performance enhancement of SPR biosensor using graphene–MoS2, hybrid structure. Nanomater. (Basel). 12, 2219. doi:10.3390/nano12132219
Cerutti, F., Burdino, E., Milia, M. G., Allice, T., Gregori, G., Bruzzone, B., et al. (2020). Urgent need of rapid tests for SARS-CoV-2 antigen detection: evaluation of the SD-biosensor antigen test for SARS-CoV-2. J. Clin. Virol. 132, 104654. doi:10.1016/j.jcv.2020.104654
Drobysh, M., Liustrovaite, V., Kanetski, Y., BrasIunas, B., Zvirbliene, A., Rimkute, A., et al. (2024). Electrochemical biosensing based comparative study of monoclonal antibodies against SARS-CoV-2 nucleocapsid protein. Sci. Total Environ. 908, 168154. doi:10.1016/j.scitotenv.2023.168154
Erkihun, M., Ayele, B., Asmare, Z., and Endalamaw, K. (2024). Current updates on variants of SARS-CoV-2: systematic review. Health Sci. Rep. 7, e70166. doi:10.1002/hsr2.70166
Ghodrati, M., Mir, A., and Farmani, A. (2024). Sensitivity enhancement of the surface plasmon resonance biosensor based on hybrid structure using MXene and MoS2 for refractive index sensing: an angular interrogation approach. Sens. Imaging 25, 39. doi:10.1007/s11220-024-00491-4
Haque, T., and Rouf, H. K. (2021). DNA hybridization detection using graphene-MoSe2–Ag heterostructure-based surface plasmon resonance biosensor. Appl. Phys. A 127 (10), 759. doi:10.1007/s00339-021-04888-w
Hu, J., Huang, Y., Chen, Y., Hu, Z., Wu, J., and Wang, J. (2021). High-sensitivity multi-channel refractive-index sensor based on a graphene-based hybrid tamm plasmonic structure. Opt. Mat. Express 11 (11), 3833–3843. doi:10.1364/OME.440987
Huggett, J. F., Moran-Gilad, J., and Lee, J. E. (2021). COVID-19 new diagnostics development: novel detection methods for SARS-CoV-2 infection and considerations for their translation to routine use. Curr. Opin. Pulm. Med. 27, 155–162. doi:10.1097/MCP.0000000000000768
Iravani, S. (2020). Nano- and biosensors for the detection of SARS-CoV-2: challenges and opportunities. Mat. Adv. 1, 3092–3103. doi:10.1039/D0MA00702A
Justino, C. I. L., Freitas, A. C., Pereira, R., Duarte, A. C., and Rocha-Santos, T. A. P. 2015, Recent developments in recognition elements for chemical sensors and biosensors. TrAC, Trends Anal. Chem, 68, 2–17. doi:10.1016/j.trac.2015.03.006
Kumar, A., Kumar, A., and Srivastava, S. K. (2022). Silicon nitride-BP-based surface plasmon resonance highly sensitive biosensor for virus SARS-CoV-2 detection. Plasmonics 17 (3), 1065–1077. doi:10.1007/s11468-021-01589-1
Li, X., and Zhu, H. (2015), Two-dimensional MoS2: properties, preparation, and applications. J. Mater, 1, 33–44. doi:10.1016/j.jmat.2015.03.003
Liu, Y., Li, Y., Hang, Y., Wang, L., Wang, J., Bao, N., et al. (2024). Rapid assays of SARS-CoV-2 virus and noble biosensors by nanomaterials. Nano Converg. 11, 2. doi:10.1186/s40580-023-00408-z
Luke, K., Okawachi, Y., Lamont, M. R. E., Gaeta, A. L., and Lipson, M. (2015). Broadband mid-infrared frequency comb generation in a Si_3N_4 microresonator. Opt. Lett. 40 (21), 4823–4826. doi:10.1364/OL.40.004823
Mudgal, N., Saharia, A., Choure, K. K., Agarwal, A., and Singh, G. (2020). Sensitivity enhancement with anti-reflection coating of silicon nitride (Si3N4) layer in silver-based Surface Plasmon Resonance (SPR) sensor for sensing of DNA hybridization. Appl. Phys. A 126 (12), 946. doi:10.1007/s00339-020-04126-9
Naik, G. V., Shalaev, V. M., and Boltasseva, A. (2013). Alternative plasmonic materials: beyond gold and silver. Adv. Mat. 25 (24), 3264–3294. doi:10.1002/adma.201205076
Patel, S. K., and Parmar, J. (2021), Highly sensitive and tunable refractive index biosensor based on phase change material. Physica B: Condensed Matter, 622, 413357. doi:10.1016/j.physb.2021.413357
Pisarra, M., Vacacela Gomez, C., and Sindona, A. (2022). Massive and massless plasmons in germanene nanosheets. Sci. Rep. 12, 18624. doi:10.1038/s41598-022-23058-3
Sajal, S. S. A., Islam, D. Z., Khandker, S. S., Solórzano-Ortiz, E., Fardoun, M., Ahmed, M. F., et al. (2024), Strategies to overcome erroneous outcomes in reverse transcription-polymerase chain reaction (RT-PCR) testing: insights from the COVID-19 pandemic. Cureus, 16, e72954. doi:10.7759/cureus.72954
Sengupta, J., and Hussain, C. M. (2021). Graphene-based field-effect transistor biosensors for the rapid detection and analysis of viruses: a perspective in view of COVID-19. Carbon Trends 2, 100011. doi:10.1016/j.cartre.2020.100011
Sindona, A., Pisarra, M., Bellucci, S., Tene, T., Guevara, M., and Vacacela Gomez, C. (2019). Plasmon oscillations in two-dimensional arrays of ultranarrow graphene nanoribbons. Phys. Rev. B 100 (23), 235422. doi:10.1103/PhysRevB.100.235422
Srivastava, A., Verma, A., Das, R., and Prajapati, Y. K. (2020). A theoretical approach to improve the performance of SPR biosensor using MXene and black phosphorus. Opt. (Stuttg). 203, 163430. doi:10.1016/j.ijleo.2019.163430
Su, L.-C., Tian, Y.-C., Chang, Y.-F., Chou, C., and Lai, C.-S. (2013). Rapid detection of urinary polyomavirus BK by heterodyne-based surface plasmon resonance biosensor. J. Biomed. Opt. 19 (1), 011013. doi:10.1117/1.JBO.19.1.011013
Tene, T., Arias Arias, F., Paredes-Páliz, K. I., Haro-Barroso, C., and Vacacela Gomez, C. (2024b). An optimized graphene-based surface plasmon resonance biosensor for detecting SARS-CoV-2. Appl. Sci. (Basel). 14, 10724. doi:10.3390/app142210724
Tene, T., Bellucci, S., Arias Arias, F., Carrera Almendariz, L. S., Flores Huilcapi, A. G., and Vacacela Gomez, C. (2024a). Role of graphene in surface plasmon resonance-based biosensors. Sensors (Basel). 24, 4670. doi:10.3390/s24144670
Tene, T., Guevara, M., Benalcázar Palacios, F., Morocho Barrionuevo, T. P., Vacacela Gomez, C., and Bellucci, S. (2023b). Optical properties of graphene oxide. Front. Chem. 11, 1214072. doi:10.3389/fchem.2023.1214072
Tene, T., Guevara, M., Moreano, G., Calderón, E., Bonilla García, N., Vaccalea Gomez, C., et al. (2023a). Modeling plasmonics and electronics in semiconducting graphene nanostrips. Emerg. Sci. J. 7 (5), 1459–1477. doi:10.28991/ESJ-2023-07-05-01
Tene, T., Svozilík, J., Colcha, D., Cevallos, Y., Vinueza-Naranjo, P. G., Vacacela Gomez, C., et al. (2024c). The tunable parameters of graphene-based biosensors. Sensors (Basel). 24, 5049. doi:10.3390/s24155049
Uddin, S. M. A., Chowdhury, S. S., and Kabir, E. (2021). Numerical analysis of a highly sensitive surface plasmon resonance sensor for SARS-CoV-2 detection. Plasmonics 16 (6), 2025–2037. doi:10.1007/s11468-021-01455-0
Varasteanu, P. (2020). Transition metal dichalcogenides/gold-based surface plasmon resonance sensors: exploring the geometrical and material parameters. Plasmonics 15 (1), 243–253. doi:10.1007/s11468-019-01033-5
Yang, D., Zhou, M., Zhang, Y., Geng, M., and Cheng, X. (2024), The coronavirus is a formidable foe: children’s multimodal articulations of SARS-CoV-2 virus and COVID-19 pandemic experiences in China. Psychology. Health, 1, 33. doi:10.1080/08870446.2024.2414797
Zheng, H., Xu, Y., Pan, J., Sun, M., and Wang, Q. (2024). Angle sensor for minimally invasive surgical robot based on surface plasmon resonance with Kretschmann prisms. Plasmonics (5), 1–10. doi:10.1007/s11468-024-02471-6
Keywords: surface plasmon resonance, MoS2, silicon nitrite, SARS-CoV-2, biosensor
Citation: Tene T, Tubon-Usca G, Gallegos KT, Mendoza Salazar MJ and Vacacela Gomez C (2025) MoS2-based biosensor for SARS-CoV-2 detection: a numerical approach. Front. Nanotechnol. 6:1505751. doi: 10.3389/fnano.2024.1505751
Received: 03 October 2024; Accepted: 13 December 2024;
Published: 07 January 2025.
Edited by:
Sujit Das, Indian Institute of Science (IISc), IndiaReviewed by:
Pravin Kavle, University of California, Berkeley, United StatesCopyright © 2025 Tene, Tubon-Usca, Gallegos, Mendoza Salazar and Vacacela Gomez. This is an open-access article distributed under the terms of the Creative Commons Attribution License (CC BY). The use, distribution or reproduction in other forums is permitted, provided the original author(s) and the copyright owner(s) are credited and that the original publication in this journal is cited, in accordance with accepted academic practice. No use, distribution or reproduction is permitted which does not comply with these terms.
*Correspondence: Cristian Vacacela Gomez, dmFjYWNlbGFAbG5mLmluZm4uaXQ=
Disclaimer: All claims expressed in this article are solely those of the authors and do not necessarily represent those of their affiliated organizations, or those of the publisher, the editors and the reviewers. Any product that may be evaluated in this article or claim that may be made by its manufacturer is not guaranteed or endorsed by the publisher.
Research integrity at Frontiers
Learn more about the work of our research integrity team to safeguard the quality of each article we publish.