- 1School of Engineering and Technology, Department of Computer Science and Engineering, Adamas University, Kolkata, West Bengal, India
- 2School of Technology, Woxsen University, Hyderabad, India
- 3Department of Computer Science and Engineering, BVRIT HYDERABAD College of Engineering for Women, Hyderabad, India
- 4NanoBioTech Laboratory, Department of Environmental Engineering, Florida Polytechnic University, Lakeland, FL, United States
The 6th-generation (6G) sensing technology is transforming the ways we perceive and interact with the world in real scenarios. It combines advanced materials, sophisticated algorithms, and connectivity to create intelligent, context-aware systems that can interpret and respond to environmental stimuli with unprecedented accuracy and speed. The key advancements include 1) ultra-sensitive sensors capable of detecting physical, chemical, and biological changes at low concentrations, 2) the integration of artificial intelligence (AI) and machine learning (ML) for enhanced data processing, and 3) the deployment of IoT networks with 5th-generation (5G) for seamless data transmission and real-time analysis. These cutting-edge technologies create immersive environments where devices capture data and anticipate user needs and environmental conditions. The 6G sensing technology has potential applications across sectors like point-of-care (PoC), healthcare, urban planning, and environmental monitoring. The transition from sensing to sense-making represents a paradigm shift, fostering a more intuitive, responsive, and interconnected world. The article provides a comprehensive overview of the current state and prospects of 6G sensing technology, highlighting its transformative potential and the challenges in realizing its full capabilities.
1 Introduction
Advanced sensing technology has revolutionized data-driven decision-making across various industries, including healthcare, environmental monitoring, manufacturing, and agriculture. Although the use of one-size-fits-all sensing schemes has been shown to dramatically enhance our ability to capture real-time data regarding multifaceted systems and occurrences, the nuanced and context-bound nature of the information we typically seek is often neglected. As the complexity, scope, and unique circumstances encompassing these situations expand, the need for more targeted and application-specific sensing becomes increasingly clear. The sensing-to-sense approach comprises a novel paradigm of developing actionable insights and decision-making support from the data of sensors.
Sensing technology has developed across generations, with improvements in usefulness, sensitivity, and accuracy brought about by each new version. First-generation sensors were mostly analog and had simple functions. Since the early 1990s, when 2nd-generation (2G) mobile radio network technologies were introduced, the worldwide communication network has advanced significantly. Unquestionably, the second-generation network has been acknowledged globally as the beginning of a new age in digital communications. In the early 2000s, 3rd-generation (3G) systems were introduced with innovations to address the connectivity problems that arise when multiple users attempt to access the network at the same time and to provide a better experience. Numerous commentators proposed that 3G had technological and regulatory challenges, which caused many operators to phase it out of their networks. On the other hand, the broad, international media acclaim for 4th-generation (4G), showed that it was, thus far, the most successful generation since 2G. Based on multiple-input, multiple-output (MIMO), and orthogonal frequency division multiplexing (OFDM) systems, the fourth-generation network can theoretically reach speeds of up to 1 Gb/s. Until recently, this was thought to be adequate for nearly all current network services and applications. The newly launched 5G system is frequently portrayed as an integrated system that bridges the gap between 4G and the needs of the current network, including extremely low connection latency and ultra-high transmission rates. Nevertheless, a fresh line of inquiry has just now begun, exploring beyond 5G and searching for alternatives (Salameh and Mohamed, 2022). With the integration of sophisticated features including enhancing sensor capabilities through machine learning algorithms for predictive and adaptive sensing and utilizing 5G and beyond for quicker and more dependable data transmission, 6G sensing technology marks a substantial advancement (Alsharif et al., 2020).
The potential of 6G sensing technology to propel technological progress, enhance decision-making, and tackle pressing global issues makes its study extremely important. The 6G sensors have the potential to transform several sectors, improve economic efficiency, and have a substantial influence on social wellbeing by going beyond simple data collecting and instead obtaining actionable insights. To create a smarter, more connected world, this study offers fundamental knowledge that can encourage more research, development, and use of cutting-edge sensing technology. This approach, in contrast to traditional sensing, converts raw data into actionable knowledge, helping stakeholders to make decisions faster and more informedly with precision and self-assurance. Figure 1. Depicts the smart sensing to sense from the 5th Generation to the 6th Generation. It is achieved through the leveraging of advanced sensing technologies and informatics tools of high sophistication to realize the full potential inherent in sensor data in various applications and industries (Banitaba et al., 2023). This approach goes beyond conventional disciplinary lines and promotes interaction between experts in such fields as engineering, computer science, healthcare, and environmental science.
The experimental setups for 6G sensing technologies use AI, IoT, ML, and multi-sensor integration to explore their potential uses and capacities in various industries. These setups evaluate sensor performance, improve data processing skills, and address specific issues in smart cities, environmental monitoring, healthcare, and automotive. By utilizing this data, wireless networks can enhance adaptability in dynamic contexts, increase resilience to channel-dependent effects like obstruction, and enable more sensing services (González-Prelcic et al., 2024). The integration of devices like smartphones, UAVs, and sensors into IoT networks involves managing resources, processing data, and maintaining security. Studies aim to improve accuracy and reliability by integrating multiple sensors (Mahmood et al., 2022). THz bands in wireless networks enable nano-scale and macro-scale applications. AI/ML aids in interpreting collected data for effective service provision, and ML operations solve physical layer problems in THz communication networks (Boulogeorgos et al., 2021). ML algorithms can enhance energy efficiency in network system design by optimizing resource management, user association, and routing paths for wireless rechargeable devices (Mao et al., 2022). Interdisciplinary synergy enriches our understanding of complicated systems, and it speeds up the translation of research discoveries into practical and scalable solutions. The 5th and 6th generations of biosensing technology are an emerging field that integrates efficient micro/nano-enabled sensing platforms using biomaterials with smart technologies like AI, IoT, and ML to create POC devices that study health management strategies (Chugh et al., 2023; Huseynov et al., 2024; Rosic, 2024; Khalilov et al., 2023).
The study looked at different parts of the health tech market, like the move from 5G to 6G (Guo et al., 2021), the Metaverse (Wang et al., 2022; Yang et al., 2022; Zawish et al., 2022; Chengoden et al., 2023), mental healthcare technology (Shin et al., 2022; Mangalik et al., 2024), and next-generation IoT applications like Internet-of-medical-things (IoMT) (Mathkor et al., 2024; Rahman et al., 2024) and Internet-of-Healthcare-Things (IoHT). The IoHT’s quick development transforms the current healthcare system. Projections suggest substantial growth across these sectors, with the transition to 6G technology driving significant market expansion. For example, the value of the 5G to 6G switch in the healthcare industry is predicted to reach USD 59.90 billion by 2031, while the Metaverse in the Healthcare market could soar to USD 71.65 billion by 2030. Additionally, the mental healthcare technology market anticipates reaching USD 10.93 billion by 2031, and the Next-Generation IoT (Mazumdar et al., 2023) sector is forecasted to achieve USD 1.61 billion in revenue by 2031. Key players such as Cisco Systems, Inc., Intel Corporation, and Oracle Corporation dominate the competitive landscape, providing stakeholders with insights into market positioning and growth potential (InsightAce Analytic, 2022).
The leading semiconductor industry Samsung’s advancements in radio technology underscore a significant shift towards spectrum expansion into upper mid-band and Terahertz (THz) bands (Wang et al., 2022; Chen et al., 2023a), alongside innovative antenna designs and the infusion of Artificial Intelligence (AI) into wireless protocol architecture. These advancements not only make it possible for 5G to continue developing and for 6G to finally appear but also highlight the indispensable role of deep learning (DL) in shaping the future of wireless communications (Luo et al., 2024). By leveraging DL across various layers of the air interface, Samsung aims to unlock unprecedented improvements in 6G technologies (Chowdhury et al., 2020). Nevertheless, even if AI and machine learning (ML) have the potential to improve wireless networks, challenges persist in their seamless integration. Samsung’s focus on utilizing DL to control millimeter-wave (mmWave) and THz communications marks a paradigm shift, offering promising avenues for overcoming the complexities associated with high-frequency technologies. Additionally, discussions surrounding DL-based channel state information feedback underscore the potential benefits and hurdles in implementing such techniques in next-generation wireless systems, reflecting Samsung’s commitment to driving innovation amidst a rapidly evolving technological landscape (Samsung Electronics, 2022).
Considering the above-discussed viewpoint, the change from general sensing to the sensing-to-sense method signifies a fundamental change in how we approach data-driven decision-making. A thorough and impartial assessment of the state-of-the-art 6th-generation sensing technology was assured by this methodical methodology, which laid a strong basis for comprehending the technology’s present condition and promise for the future. To get pertinent material, the literature search procedure for 6th-generation sensing technology entails careful database selection, tactical keyword usage, and filter application. We created a thorough literature evaluation that enriches current understanding and directs future directions in this quickly growing subject by concentrating on recent findings from 2020 to 2023. We are expecting a push the boundaries of efficiency, accuracy, and creativity by maximizing sensing technology and informatics to derive useful insights from sensor data. To elaborate on such an approach, this strategic perspective aims to cover the gaps in the proposed field and explore the aspects of sensing technology for personalized health management. This comprehensive article will pave the way for a time when data-driven decision-making permeates every facet of human endeavor and becomes the norm.
1.1 Fifth -generation sensing technology
The 5th-generation sensing technology revolutionizes data collection and utilization by introducing compact, low-power, and enhanced sensors. Driven by advancements in materials science, microfabrication, and wireless communication, these sensors offer unprecedented miniaturization and efficiency. This resulted in the development of bio-based smart wearable devices, portable diagnostic tools, and IoT sensors for environmental monitoring, paving the way for new possibilities in sensor deployment (Banitaba et al., 2023; Devi et al., 2023; Smith et al., 2023). From smart rings, and smartwatches that monitor heart rate and activity levels to handheld devices that perform rapid medical diagnostics, 5th -generation sensors are ubiquitous in our daily lives, seamlessly integrating into our routines while providing valuable insights into our health and surroundings (Georgiou et al., 2021).
Another outstanding characteristic of 5th-generation sensors is that they not only gather data but also process and transmit it ideal for continuous feedback, allowing for rapid interventions and decision-making (K. M et al., 2024). Whether it's measuring toxins in the air, checking crucial signs in a home-based patient, or monitoring environmental parameters in an agricultural setting, 5th-generation sensors, and the IoMT provide flexibility and responsiveness nothing like ever before, enabling a variety of applications across industry sectors (Tang et al., 2022; Umer et al., 2023; Rahman et al., 2024). IoMT allows remote health medicine sensors and is essential for radio medical devices to improve accuracy, consistency, availability, and commonality of the usefulness of the connected equipment. This method conceptualized an architecture for the retrieval approach of information for the sensors to gather information from medical records for biomedical analysis and diagnosis of various illnesses in order to quickly conduct hospital treatment, but also to provide customized healthcare and less expensive medical therapy (Mazumdar et al., 2023; Farid and Khondakar, 2024; Mathkor et al., 2024). The IoT is a rapidly expanding network of linked biomedical sensors that records every data transmission and sharing between other industries. The inspired 5G paradigm for healthcare application-oriented secure wireless medical sensor networks allows for optimum the numerous forms of sensors used in-line communication with a plethora of sensors (Al-Turjman and Alturjman, 2018). Smart sensors and IIoT are increasing the importance of Industry 4.0 which is rapid movement of the current business climate, altering work for factories, homes, and other works (Gupta, 2021).
Furthermore, the 5th-generation sensors are portable and versatile; hence, they are best suited for personalized sensing purposes. During these times when personalized healthcare is gaining momentum and personalized interventions have become the norm, the sensors are critical for individual-based data collection and analysis (Javaid et al., 2023). By creating individual-based data on physiological metrics, behavioral measures, and exposure data, the 5th-generation sensors help power an individual to get involved and drive his or her health journey easier (Oz et al., 2023; Kumar et al., 2024; Kaushik, 2024). Chronic diseases can be managed more prudently, diseases can easily be detected when still in their early stages, and lifestyle can be optimized through these sensors. In general, latency reduction in Beyond 5G (B5G) prevents the healthcare connection from significantly reducing the gap between rural and urban healthcare. It also boosts the outcome, quality, and access to rural healthcare in these areas (Kumar et al., 2024). ML is expected to be a key technology supporting the development of B5G mobile networks, in view of the tremendous amount of data traffic that has been generated for the deployment of the Internet of Everything (IoE) (Xu et al., 2023). Three communications types—digital twins, ubiquitous global coverage, and hyper-realistic communication—underpin the B5G revolution (Beyond, 2022). Fundamentally, intelligent systems based on digital twins have the power to fundamentally change human perception and interaction. An extensive variety of uses, including improved identity recognition, robotic control, personalized healthcare monitoring, rehabilitation, and encrypted interactions in Virtual Reality (VR) and Augmented Reality (AR) space, are just a few examples (Lu and Zheng, 2020; Zhang et al., 2022).
The design and application of intelligent biosensors have fundamentally changed with the advent of 5G sensing technology, which offers previously unheard-of levels of efficiency, adaptability, and miniaturization on a single chip (Chaudhary et al., 2023). With their continued development and widespread use, these sensors will surely be crucial in determining the direction of environmental monitoring, healthcare, and other fields going forward, ushering in a period of empowered, individualized decision-making based on data (Yin et al., 2024).
1.2 Sixth -generation sensing technology
The progression from 5th-generation to 6th-generation sensing technology represents a significant evolution in sensor capabilities, driven by the integration of advanced computational approaches such as AI, ML, and Big Data. Big data analytics and machine learning can manage diversified data, such as patient info, genetics, and Electronic Health Records (EHRs), making the doctors offer correct disease diagnoses. The use of IoT can provide healthcare implementation services like linking small healthcare items to the internet that generate big data analyzed using deep learning (Bhatkande et al., 2023). Using 6G-based big data analysis technology, which ensures low energy consumption and high access success rates, large-scale IoT devices can have greater access success rates (H. F. Ahmad et al., 2023a; Hadi et al., 2020; Lv et al., 2021; Lima et al., 2021). One THz operational frequency, 1 Tbps data throughput, 300 μm wavelength, and 1,000 km/h mobility range are the main prerequisites for 6G communication technology. Terahertz (THz) technology offers the required increased bandwidth and has the potential to greatly increase network capacity, thus, its incorporation into 6G healthcare systems signals the start of a new chapter in the history of medicine (Jiang et al., 2024). It also comes with a host of game-changing benefits (Sarkar et al., 2022). Furthermore, the IoT will give way to the Internet of Everything (IoE) as the main network (Bariah et al., 2020; Ji et al., 2021; Nayak and Patgiri, 2021; Mohjazi et al., 2023; Chataut et al., 2024a). IoE will require the ability to interface with millions of smart devices, gather tactile sensitivities, and translate them into digital data to meet 6G requirements. User-centric communications and excellent quality of service are defined by the Quality of Experiences (QoE). QoE will be enabled by the haptic Internet, AR, VR, and holographic communications, all of which need very fast data rates and low latency (Nayak and Patgiri, 2021). More processing power will be needed for big data to collect and analyze the enormous volumes of little data produced by healthcare devices. The term “Intelligent Internet of Medical Things,” or “IIoMT,” describes a class of intelligent AI-driven devices that leverage communication technology to get over limitations in terms of cost, time, and location in making decisions on their own. Blockchain is a disruptive technology that occurs that gives new ways to manage privacy and security issues in 6G-IoT networks (Liu et al., 2024).
6G sensing uses AI algorithms for sensors to handle and understand a lot of data automatically (Giordani et al., 2020). As sensors achieve a higher level of autonomy and intelligence, they become more context-aware and responsive to real-time environments, user preferences, and operational conditions (Ahad et al., 2024). Communication networks will function more accurately and efficiently when AI algorithms are used. The use of AI in communication can help in real-time communication, which is essential for current healthcare systems’ diagnosis and decision-making. AI has made it possible for healthcare to carry out real-time operations like remote surgery (Chugh et al., 2024; Kumar et al., 2024). Eventually, these technologies will mature and proliferate as they continue to advance. They hold tremendous potential for transforming industries, improving safety and efficiency, and helping us monitor, understand, and respond to the world around us (Shen et al., 2023).
The integration of 6G sensing technology in biomedical applications offers significant benefits in medical diagnostics, treatment, and patient monitoring. These sensors can identify disease-related biomarkers in bodily fluids, enabling early diagnosis of conditions like cancer or infectious diseases. Wearable sensors can continuously monitor vital signs, enabling real-time health tracking and timely intervention. Machine learning algorithms can process vast amounts of data collected by 6G sensors to predict disease progression and patient outcomes, leading to personalized treatment plans. AI-powered 6G systems can assist in diagnosing medical conditions by comparing patient data with extensive medical databases, providing accurate and rapid diagnoses. Patients can be monitored remotely, facilitating continuous care for chronic conditions and reducing hospital visits. Real-time data can alert medical personnel to sudden changes in a patient’s condition, enabling swift intervention. Smart implants equipped with 6G sensors can adapt their function based on real-time feedback, improving patient comfort and implant performance. 6G-enabled devices can track the spread of infectious diseases in real time, enabling public health officials to respond promptly to outbreaks (Chowdhury et al., 2020; Srinivasu et al., 2022; Chataut et al., 2024).
With the help of technologies like digital twins, blockchain, VR and AR, mixed and extended reality, high-speed internet, and AI, which are all enhanced by essentially infinite amounts of data, people can interact with their avatars in the newly emerging metaverse, which unites the virtual and physical worlds (Wang et al., 2022; Mazumdar et al., 2024). A metaverse of “medical technology and AI” (MeTAI) can support the development, testing, assessment, control, translation, and enhancement of AI-based medicine, including imaging-guided diagnosis and therapy (Yang et al., 2022; Chengoden et al., 2023).
1.3 Personalized sensing; sensing-to-sense
Personalized sensing is a technology that customizes sensing systems to individual needs and preferences. It involves customizing sensor hardware and developing AI-driven analytics algorithms to extract actionable insights from personalized data. Providing real-time decision-making support and actionable information enhances the effectiveness of point-of-care testing (POCT), facilitates targeted interventions, and improves the user experience (Saroğlu et al., 2024; Abubeker et al., 2024; A. K; Kaushik et al., 2020; Jain et al., 2021; Futane et al., 2023; Khondakar et al., 2023). Even, wearables have recently demonstrated positive advancements in drug delivery, further increasing their usefulness for personalized healthcare (Khondakar et al., 2019; Iqbal et al., 2021). The 5th and 6th generations of biosensing technology are an emerging field that integrates efficient micro/nano-enabled sensing platforms with smart technologies like AI, IoT, and ML to create POC devices that study health management strategies (Chugh et al., 2023). As we cast our gaze into the future, personalized sensing emerges as a linchpin in the realms of healthcare, wellness, and beyond. The trajectory of this evolution hinges upon the symbiotic integration of advanced sensor technology with AI-driven analytics, fostering the development of highly tailored and adaptive sensing systems meticulously crafted to cater to individual idiosyncrasies with unparalleled precision (Manickam et al., 2022). Utilizing bioimpedance’s deep tissue sensing capability, smart rings provide exceptional chances for ongoing physiological monitoring (Sel et al., 2023). Biomolecular sensing technologies based on the body, such as consumable, wearable, and implanted sensors, provide extensive health-related monitoring (Flynn et al., 2023). Figure 2. Describes the Smart Sensing Innovation Towards 6G such as Smart Ring, Smart Bandage, Quantum Lab-on-a-Chip, Living Tattoo in Human Skin, AI-Copilot for Telemedicine, Wearable chemical sensors for biomarker discovery, Brain-AI Closed loop, Metaverse, Large Language Models, and POC diagnostics using predictive diagnostics.
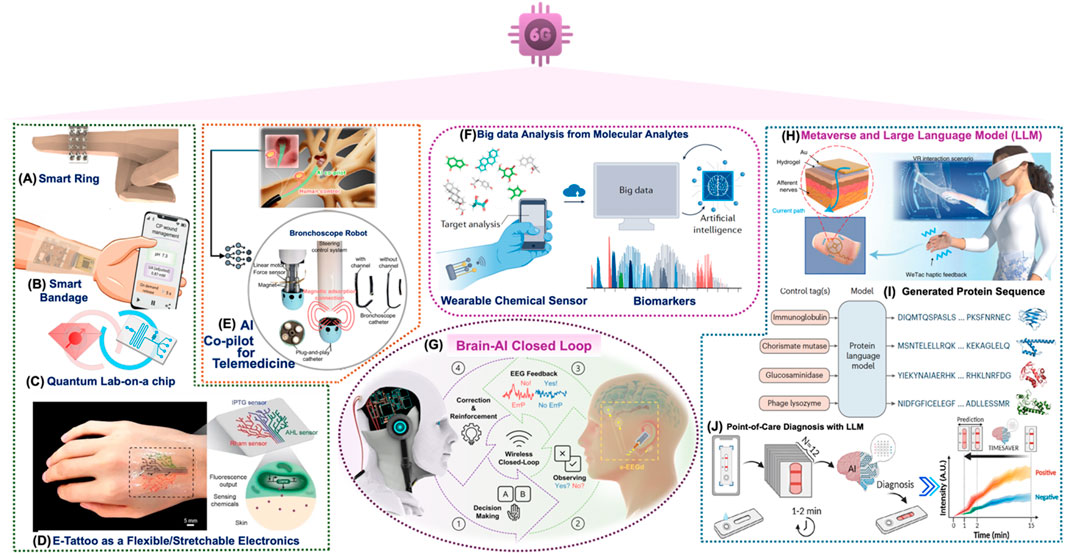
Figure 2. Smart Sensing Innovation Towards 6G, (A) Smart Ring [Adapted from (Sun et al., 2022)]; (B) Smart Bandage [Adapted from (Meng et al., 2024)]; (C) Quantum Lab-on-a-Chip [Adapted from (Allert et al., 2022)]; (D) Living Tattoo in Human Skin [Adapted from (Liu et al., 2018)]; (E) AI-Copilot for Telemedicine [Adapted from (Zhang et al., 2024)]; (F) Wearable chemical sensors for biomarker discovery [Adapted from (Sempionatto et al., 2022)]; (G) Brain-AI Closed loop [Adapted from (Shin et al., 2022)]; (H) Metaverse and LLM [Adapted from (Yao et al., 2022)]; (I) Generated using large language models, functional protein sequences in a variety of families [Adapted from (Madani et al., 2023)]; (J) Point-of-care (POC) diagnostics using predictive diagnostics [Adapted from (Lee et al., 2024)].
Utilizing the idea of quantum mechanics, which has significantly increased biosensor sensitivity, is the rapidly developing field of quantum biosensing technology. Microfluidic quantum sensing combined with 6G technology opens up a new and interesting avenue for lab-on-a-chip applications that allow for real-time data transmission and processing (Mazumdar et al., 2023; Das et al., 2024; Khondakar, 2024; Shrikrishna et al., 2024; Yasmin and Khondakar, 2024). A mix of new materials, advanced body sensing, and various data methods can give a full view of a person’s health and contact with the world (Ahmad et al., 2023b). A digital twin (DT) is an electronic representation of a person’s physiological condition made from real-time data gathered by sensors and health-related devices. It may be used to forecast, optimize, and mimic an individual’s health outcomes (Venkatesh et al., 2022; Tang et al., 2024). With holographic sensors based on hydrogel, PoC diagnosis can be made inexpensively, without labels, and qualitatively analyzed using spectroscopy (Davies et al., 2023). Similarly, in 3D printing hydrogel inks, live organisms like bacteria and cells are combined with synthetic materials to make objects that react to stimuli and interact with each other. These sensitive materials may be used in robotics, environmental sensing, and biomedicine (Liu et al., 2018; Argade and Hirak, 2023).
Furthermore, IoHT for patients is made up of several wearables that are sold in the market, such as fitness bands, smartwatches, and other wirelessly enabled devices (like blood pressure monitors, heart rate monitors, glucose meters, etc.). These intelligent gadgets are employed for personalized sensing (Ketu and Mishra, 2021). It is now possible to develop multimodal AI solutions that consider the complexity of human health and sickness since biomedical data from large biobanks, electronic health records, medical imaging, wearable and ambient biosensors, and less expensive genome and microbiome sequencing are readily available (Acosta et al., 2022). Through the continuous and non-invasive collection of rich physiological and activity-related personal data, wearable technologies have created enormous prospects for monitoring and enhancing the wellbeing of individuals at all stages of aging (Chen et al., 2023b). Novice physicians may perform lung exams on typical adult patients safely and proficiently beyond the fifth generation utilizing a co-pilot AI bronchoscope robot (Zhang et al., 2024). Human-machine interfaces, or smart, flexible electronic wearables with information-exchanging capabilities, have become essential conduits for the connection, exchange of ideas, and human-machine interaction. Smart wearable electronics are becoming a reality with the development of multimodal electronic textiles, which integrate multipurpose sensors into everyday apparel (Wei et al., 2023).
Propelled by ML models trained on extensive datasets of inorganic nanoparticles (NP), these algorithms hold the promise of unraveling individual variability and forecasting health outcomes with unprecedented precision (Mendes et al., 2024). Beyond the confines of healthcare, the vast potential of personalized sensing extends its reach into diverse domains, encompassing personalized nutrition, fitness tracking, telehealth, environmental monitoring, and personalized marketing (Vaghasiya et al., 2023; Khondakar et al., 2024). Customizing sensing systems to individual behaviors can enhance product relevance and specificity, boosting customer satisfaction and loyalty. The sensing-to-sense paradigm emphasizes context awareness, adaptability, and user engagement in shaping future sensing technologies. As advancements occur, sensing technologies will integrate into daily life, providing personalized, timely, and actionable feedback. The evolution of user interfaces will foster trust and widespread adoption, catalyzing a paradigm shift in how we perceive, interact with, and harness the world’s potential. This will lead to a more intuitive and user-friendly experience (Guk et al., 2019; Janjua et al., 2020).
Huge excitement has been created around the application of generative large language models (LLMs) with the rapid advancement of AI in healthcare when models like ChatGPT, Bard, and ProGen (protein) shocked the world by conversing and responding to questions and producing textual material like emails, essays, and even computer programs. While there is excitement about LLMs’ potential to help with various clinical care, improve patient care, hospital operations, adiagnostic accuracy, and make patient reports (like progress reports) easier to document, there is also concern about the risks associated with bias and stereotypes, hallucinations and fabrications, and patient privacy and ethics (Clusmann et al., 2023; Madani et al., 2023; Mazumder et al., 2023; Mazumdar et al., 2023; Meskó and Topol, 2023; Peng et al., 2023; Wornow et al., 2023; Zhou et al., 2023; Mazumdar et al., 2024). Graphs, medical text, genomics, imaging, electronic health records, laboratory results, and other combinations of medical modalities may all be interpreted flexibly by generalist medical artificial intelligence (GMAI). It is created by self-supervision using enormous, varied datasets (Moor et al., 2023). An RNA-focused pre-trained model called Multi-purpose RNA Language Modeling (RNAErnie) is designed to identify and make use of RNA sequence features (N. Wang et al., 2024).
1.4 Challenges and aspects
Advanced sensing technologies offer significant benefits, but they also face challenges such as interoperability, which hinders seamless integration and collaboration between sensors and data platforms. Siloed operations limit data flow, hindering cross-domain collaboration and innovation. A network operating in the THz band with much wider spectrum resources, intelligent communication environments that enable a wireless propagation environment with active signal transmission and reception, pervasive artificial intelligence, large-scale network automation, an all-spectrum reconfigurable front-end for dynamic spectrum access, ambient backscatter communications for energy savings, the Internet of Space Things made possible by CubeSats and UAVs, and cell-free massive MIMO communication networks are some of the major technological advancements needed to meet 6G’s connectivity goals (Akyildiz et al., 2020). To unlock the full potential of sensing technologies, efforts must be made to foster interoperability. There are also significant concerns regarding data privacy and security, particularly in sensitive fields like healthcare and personal sensing Long-term effects and device safety can only be evaluated with the help of electronic health records. The key to avoiding and mitigating disasters is proactive navigation (Callahan et al., 2019). Data protection is critical due to the proliferation of sensitive personal information because sensitive personal information is increasingly accessible, breached, and misused Data privacy and confidence regarding the security of point-of-care IoT devices are essential to improving user awareness and ensuring robust safeguards, including encryption, access controls, and anonymization techniques emphasized the importance of enhanced awareness and robust safeguards (Nath et al., 2023; Ahmad et al., 2024). The ethical implications of advanced sensing technologies loom large on the horizon. As AI algorithms become more and more sophisticated, fairness, equity, and justice become more difficult to maintain (Uwaoma, 2023; Ahmed et al., 2024).
As a result, the threat of privacy violations underscores the need for robust ethical frameworks and guidelines to govern the deployment of sensing technologies on an equitable and responsible basis. Furthermore, for the use of sensing technologies as instruments of progress, empowerment, and social good, transparency, accountability, and ethical considerations are essential. Sustainable management and use of sensing systems entail social elements such as user acceptability or trust (Chaudhary et al., 2022). Users need to see the sensing technologies they use as trustworthy, reliable, and useful to function properly. Hence, user involvement, education, and promotion of a climate conducive to trust and faith in sensing technologies are essential. In addition, designing, developing, and assessing sensing systems with the participation of end users ensures their alignment with their needs, desires, and expectations, making them widely implemented and leading to long-term benefits for society. Addressing these and other obstacles to advanced sensing technologies necessitates a comprehensive approach across multiple dimensions. Our capacity to navigate through the maze of sensors and seize their transformational ability is predicated on our ability to take pre-emptive action in these areas, such as interoperability, privacy, ethical considerations, and societal repercussions.
2 Conclusion and viewpoint
This article carefully and critically explored the approach of next-generation sensing systems focused on sensors-to-sensing methodologies. This is expected to have great potential to overcome the current healthcare issues and monitoring of environmental problems. By leveraging the capabilities of 5th and 6th-generation sensing technologies, along with personalized sensing and AI-driven analytics, we can develop more efficient, context-aware, and user-centric sensing systems (Waseem and Hassan, 2021). To fully utilize these technologies and guarantee their appropriate deployment for the good of society, it is necessary to address the related issues and concerns. The Futuristic Smart 6G Sensing Technologies is illustrated in Figure 3. To overcome these obstacles and realize the revolutionary promise of advanced sensing technologies, collaboration between disciplines—including engineering, computer science, healthcare, and social sciences—will be essential, i.e., 6G and beyond which will meet the needs of a fully linked world. It is anticipated that transformative solutions will propel the push to accommodate an ever-increasing number of intelligent devices and services (Akyildiz et al., 2020). Promising early-stage technologies that are predicted to have a significant influence on wireless communications beyond 6G include quantum communications, the Internet of NanoThings, and the Internet of BioNanoThings.
Data availability statement
The original contributions presented in the study are included in the article/Supplementary Material, further inquiries can be directed to the corresponding authors.
Author contributions
HM: Writing–original draft. KK: Writing–review and editing. SD: Writing–original draft. AK: Writing–review and editing.
Funding
The author(s) declare that no financial support was received for the research, authorship, and/or publication of this article.
Acknowledgments
The authors want to acknowledge their respective institutions for their support.
Conflict of interest
The authors declare that the research was conducted in the absence of any commercial or financial relationships that could be construed as a potential conflict of interest.
The author(s) declared that they were an editorial board member of Frontiers, at the time of submission. This had no impact on the peer review process and the final decision.
Publisher’s note
All claims expressed in this article are solely those of the authors and do not necessarily represent those of their affiliated organizations, or those of the publisher, the editors and the reviewers. Any product that may be evaluated in this article, or claim that may be made by its manufacturer, is not guaranteed or endorsed by the publisher.
References
Abubeker, K. M., Raja, K., Gogula, S., Baskar, S., Muthu, S., Chellamuthu, G., et al. (2024). Internet of things enabled open source assisted real-time blood glucose monitoring framework. Sci. Rep. 14 (1), 6151. doi:10.1038/s41598-024-56677-z
Acosta, J. N., Falcone, G. J., Rajpurkar, P., and Topol, E. J. (2022). Multimodal biomedical AI. Nat. Med. 28 (9), 1773–1784. doi:10.1038/s41591-022-01981-2
Ahad, A., Zheng, J., Tahir, M., Shayea, I., Sheikh, M. A., and Rasheed, F. (2024). 6G and intelligent healthcare: taxonomy, technologies, open issues and future research directions. Internet Things 25 (April), 101068. doi:10.1016/j.iot.2024.101068
Ahmad, H. F., Rafique, W., Ur Rasool, R., Alhumam, A., Anwar, Z., and Qadir, J. (2023a). Leveraging 6G, extended reality, and IoT big data analytics for healthcare: a review. Comput. Sci. Rev. 48 (May), 100558. doi:10.1016/j.cosrev.2023.100558
Ahmad, I., Ahmad, I., and Harjula, E. (2024). “Adaptive security in 6G for sustainable healthcare,” in Digital health and wireless solutions. Editors M. Särestöniemi, P. Keikhosrokiani, D. Singh, E. Harjula, A. Tiulpin, M. Janssonet al. (Cham: Springer Nature Switzerland), 38–47. doi:10.1007/978-3-031-59080-1_3
Ahmad, R., Hämäläinen, M., Wazirali, R., and Abu-Ain, T. (2023b). Digital-care in next generation networks: requirements and future directions. Comput. Netw. 224 (April), 109599. doi:10.1016/j.comnet.2023.109599
Ahmed, S., Sakib Alam, Md., Afrin, S., Rafa, S., Taher, S., Kabir, M., et al. (2024). Toward a secure 5G-enabled internet of things: a survey on requirements, privacy, security, challenges, and opportunities. IEEE Access 12, 13125–13145. PP (January). doi:10.1109/ACCESS.2024.3352508
Akyildiz, I. F., Kak, A., and Nie, S. (2020). 6G and beyond: the future of wireless communications systems. IEEE Access 8, 133995–134030. doi:10.1109/ACCESS.2020.3010896
Allert, R. D., Bruckmaier, F., Neuling, N. R., Freire-Moschovitis, F. A., Liu, K. S., Schrepel, C., et al. (2022). Microfluidic quantum sensing platform for lab-on-a-chip applications. Lab a Chip 22 (24), 4831–4840. doi:10.1039/D2LC00874B
Alsharif, M. H., Hilary Kelechi, A., Albreem, M. A., Chaudhry, S. A., Sultan Zia, M., and Kim, S. (2020). Sixth generation (6G) wireless networks: vision, research activities, challenges and potential solutions. Symmetry 12 (4), 676. doi:10.3390/sym12040676
Al-Turjman, F., and Alturjman, S. (2018). Context-sensitive access in industrial internet of things (IIoT) healthcare applications. IEEE Trans. Industrial Inf. 14 (6), 2736–2744. doi:10.1109/TII.2018.2808190
Argade, N. U., and Hirak, M. (2023). “3D printing: a game changer for Indian msme sector in industry 4.0,” in New horizons for industry 4.0 in modern business. Editors A. Nayyar, M. Naved, and R. Rameshwar (Cham: Springer International Publishing), 295–329. doi:10.1007/978-3-031-20443-2_14
Banitaba, S. N., Khademolqorani, S., Jadhav, V. V., Chamanehpour, E., Mishra, Y. K., Mostafavi, E., et al. (2023). Recent progress of bio-based smart wearable sensors for healthcare applications. Mater. Today Electron. 5 (September), 100055. doi:10.1016/j.mtelec.2023.100055
Bariah, L., Mohjazi, L., Muhaidat, S., Sofotasios, P. C., Kurt, G. K., Yanikomeroglu, H., et al. (2020). A prospective look: key enabling technologies, applications and open research topics in 6G networks. IEEE Access 8, 174792–174820. doi:10.1109/ACCESS.2020.3019590
Beyond (2022). Beyond 5G: the New Era of connectivity. Available at: https://www.nec.com/en/global/solutions/5g/blog/beyond-5g-the-new-era-of-connectivity.html (Accessed May 15, 2024).
Bhatkande, S., Mazumdar, H., and Gohel, H. (2023). “AI and IOT for biomedical smart applications,” in Analytical techniques for biomedical Nanotechnology (USA: IOP Publishing). doi:10.1088/978-0-7503-3379-5ch20
Boulogeorgos, A.-A. A., Yaqub, E., di Renzo, M., Alexiou, A., Desai, R., and Klinkenberg, R. (2021). Machine learning: a catalyst for THz wireless networks. Front. Commun. Netw. 2 (September). doi:10.3389/frcmn.2021.704546
Callahan, A., Fries, J. A., Ré, C., Huddleston, J. I., Giori, N. J., Delp, S., et al. (2019). Medical device surveillance with electronic health records. NPJ Digit. Med. 2, 94. doi:10.1038/s41746-019-0168-z
Chataut, R., Nankya, M., and Akl, R. (2024). 6G networks and the AI revolution—exploring technologies, applications, and emerging challenges. Sensors 24 (6), 1888. doi:10.3390/s24061888
Chataut, R., Nankya, M., and Akl, R. (2024a). 6G networks and the AI revolution-exploring technologies, applications, and emerging challenges. Sensors Basel, Switz. 24 (6), 1888. doi:10.3390/s24061888
Chaudhary, V., Kaushik, A., Furukawa, H., and Khosla, A. (2022). Review—towards 5th generation AI and IoT driven sustainable intelligent sensors based on 2D MXenes and borophene. ECS Sensors Plus 1 (1), 013601. doi:10.1149/2754-2726/ac5ac6
Chaudhary, V., Khanna, V., Awan, H. T. A., Singh, K., Khalid, M., Mishra, Y. K., et al. (2023). Towards hospital-on-chip supported by 2D MXenes-based 5th generation intelligent biosensors. Biosens. Bioelectron. 220 (January), 114847. doi:10.1016/j.bios.2022.114847
Chen, B., Yang, S., Chen, J., Wu, J., Chen, Ke, Li, W., et al. (2023a). Directional Terahertz holography with thermally active janus metasurface. Light Sci. Appl. 12 (1), 136. doi:10.1038/s41377-023-01177-4
Chen, C., Ding, S., and Wang, J. (2023b). Digital health for aging populations. Nat. Med. 29 (7), 1623–1630. doi:10.1038/s41591-023-02391-8
Chengoden, R., Victor, N., Huynh-The, T., Yenduri, G., Jhaveri, R. H., Alazab, M., et al. (2023). Metaverse for healthcare: a survey on potential applications, challenges and future directions. IEEE Access 11, 12765–12795. doi:10.1109/ACCESS.2023.3241628
Chowdhury, M. Z., Shahjalal, Md., Ahmed, S., and Jang, Y. M. (2020). 6G wireless communication systems: applications, requirements, technologies, challenges, and research directions. IEEE Open J. Commun. Soc. 1, 957–975. doi:10.1109/OJCOMS.2020.3010270
Chugh, V., Basu, A., Kaushik, A., and Basu, A. K. (2023). Progression in quantum sensing/bio-sensing technologies for healthcare. ECS Sensors Plus 2 (1), 015001. doi:10.1149/2754-2726/acc190
Chugh, V., Basu, A., Kaushik, A., Manshu, S. B., and Basu, A. K. (2024). Employing nano-enabled artificial intelligence (AI)-Based smart technologies for prediction, screening, and detection of cancer. Nanoscale 16 (11), 5458–5486. doi:10.1039/D3NR05648A
Clusmann, J., Kolbinger, F. R., Muti, H. S., Carrero, Z. I., Eckardt, J.-N., Laleh, N. G., et al. (2023). The future landscape of Large Language Models in medicine. Commun. Med. 3 (1), 141–148. doi:10.1038/s43856-023-00370-1
Das, S., Mazumdar, H., Reza Khondakar, K., Kaushik, A. K., and Mishra, Y. K. (2024). Review—quantum biosensors: principles and applications in medical diagnostics. ECS Sensors Plus 3, 025001. doi:10.1149/2754-2726/ad47e2
Davies, S., Hu, Y., Blyth, J., Jiang, N., and Yetisen, A. K. (2023). Reusable dual-photopolymerized holographic glucose sensors. Adv. Funct. Mater. 33 (18), 2214197. doi:10.1002/adfm.202214197
Devi, D. H., Duraisamy, K., Armghan, A., Alsharari, M., Aliqab, K., Sorathiya, V., et al. (2023). 5G technology in healthcare and wearable devices: a review. Sensors 23 (5), 2519. doi:10.3390/s23052519
Farid, I., and Khondakar, K. R. (2024). “Chapter 6 - smart sensors for infectious disease diagnosis,” in Next-generation smart biosensing. Editors K. R. Khondakar, and A. Kumar Kaushik (China: Academic Press), 149–187. doi:10.1016/B978-0-323-98805-6.00002-6
Flynn, C. D., Chang, D., Mahmud, A., Yousefi, H., Das, J., Riordan, K. T., et al. (2023). Biomolecular sensors for advanced physiological monitoring. Nat. Rev. Bioeng. 1 (8), 560–575. doi:10.1038/s44222-023-00067-z
Futane, A., Narayanamurthy, V., Jadhav, P., and Srinivasan, A. (2023). Aptamer-based rapid diagnosis for point-of-care application. Microfluid. Nanofluidics 27 (2), 15. doi:10.1007/s10404-022-02622-3
Georgiou, K. E., Georgiou, E., and Satava, R. M. (2021). 5G use in healthcare: the future is present. JSLS J. Soc. Laparosc. Robotic Surg. 25 (4), e2021. doi:10.4293/JSLS.2021.00064
Giordani, M., Polese, M., Mezzavilla, M., Rangan, S., and Zorzi, M. (2020). Toward 6G networks: use cases and technologies. IEEE Commun. Mag. 58 (3), 55–61. doi:10.1109/MCOM.001.1900411
González-Prelcic, N., Keskin, M. F., Kaltiokallio, O., Valkama, M., Dardari, D., Shen, X., et al. (2024). The integrated sensing and communication revolution for 6G: vision, techniques, and applications. Proc. IEEE, 1–0. doi:10.1109/JPROC.2024.3397609
Guk, K., Han, G., Lim, J., Jeong, K., Kang, T., Lim, E.-K., et al. (2019). Evolution of wearable devices with real-time disease monitoring for personalized healthcare. Nanomater. Basel, Switz. 9 (6), 813. doi:10.3390/nano9060813
Guo, F., Richard Yu, F., Zhang, H., Li, Xi, Ji, H., and Leung, V. C. M. (2021). Enabling massive IoT toward 6G: a comprehensive survey. IEEE Internet Things J. 8 (15), 11891–11915. doi:10.1109/JIOT.2021.3063686
Gupta, V. P. (2021). “Smart sensors and industrial IoT (IIoT): a driver of the growth of industry 4.0,” in Smart sensors for industrial internet of things: challenges, solutions and applications. Editors D. Gupta, V. H. C. de Albuquerque, A. Khanna, and P. L. Mehta (Cham: Springer International Publishing), 37–49. doi:10.1007/978-3-030-52624-5_3
Hadi, M. S., Lawey, A. Q., El-Gorashi, T. E. H., and Elmirghani, J. M. H. (2020). Patient-centric HetNets powered by machine learning and big data analytics for 6G networks. IEEE Access 8, 85639–85655. doi:10.1109/ACCESS.2020.2992555
Huseynov, E. (2024). Novel nanomaterials for hepatobiliary diseases treatment and future perspectives. Adv. Biol. Earth Sci.
InsightAce Analytic (2022). InsightAce analytic | market research reports and business consulting Firm. Available at: https://www.insightaceanalytic.com/(Accessed May 14, 2024).
Iqbal, S. M. A., Mahgoub, I., Du, E., Leavitt, M. A., and Asghar, W. (2021). Advances in healthcare wearable devices. Npj Flex. Electron. 5 (1), 9–14. doi:10.1038/s41528-021-00107-x
Jain, S., Nehra, M., Kumar, R., Dilbaghi, N., Hu, T. Y., Kumar, S., et al. (2021). Internet of medical things (IoMT)-Integrated biosensors for point-of-care testing of infectious diseases. Biosens. Bioelectron. 179 (May), 113074. doi:10.1016/j.bios.2021.113074
Janjua, M. B., Duranay, A. E., and Arslan, H. (2020). Role of wireless communication in healthcare system to cater disaster situations under 6G vision. Front. Commun. Netw. 1 (December). doi:10.3389/frcmn.2020.610879
Javaid, M., Haleem, A., Singh, R. P., and Suman, R. (2023). 5G technology for healthcare: features, serviceable pillars, and applications. Intell. Pharm. 1 (1), 2–10. doi:10.1016/j.ipha.2023.04.001
Ji, B., Wang, Y., Song, K., Li, C., Wen, H., Menon, V. G., et al. (2021). A survey of computational intelligence for 6G: key technologies, applications and trends. IEEE Trans. Industrial Inf. 17 (10), 7145–7154. doi:10.1109/TII.2021.3052531
Jiang, W., Zhou, Q., He, J., Habibi, M. A., Melnyk, S., El-Absi, M., et al. (2024). Terahertz communications and sensing for 6G and beyond: a comprehensive review. IEEE Commun. Surv. Tutorials, 1. doi:10.1109/COMST.2024.3385908
Kaushik, A. (2024). Smart electrochemical sensing of amyloid-beta to manage total alzheimer’s diseases. Neural Regen. Res. 19 (6), 1185–1186. doi:10.4103/1673-5374.385871
Kaushik, A. K., Singh Dhau, J., Gohel, H., Mishra, Y. K., Kateb, B., Kim, N.-Y., et al. (2020). Electrochemical SARS-CoV-2 sensing at point-of-care and artificial intelligence for intelligent COVID-19 management. ACS Appl. Bio Mater. 3 (11), 7306–7325. doi:10.1021/acsabm.0c01004
Ketu, S., and Mishra, P. K. (2021). Internet of healthcare things: a contemporary survey. J. Netw. Comput. Appl. 192 (October), 103179. doi:10.1016/j.jnca.2021.103179
Khalilov, R. (2024). Future prospects of biomaterials in nanomedicine. Adv. Biol. Earth Sci. 9, 5–10. doi:10.62476/abes.9s5
Khondakar, K. R. (2024). “Chapter 1 - perspective of quantum technology: towards a smarter world,” in Next-generation smart biosensing. Editors K. R. Khondakar,, and A. Kumar Kaushik (China: Academic Press), 1–16. doi:10.1016/B978-0-323-98805-6.00010-5
Khondakar, K. R., Dey, S., Wuethrich, A., Sina, A. A. I., and Trau, M. (2019). Toward personalized cancer treatment: from diagnostics to therapy monitoring in miniaturized electrohydrodynamic systems. Accounts Chem. Res. 52 (8), 2113–2123. doi:10.1021/acs.accounts.9b00192
Khondakar, R., Anwar, M. S., Mazumdar, H., and Kaushik, A. (2023). Perspective of point-of-care sensing systems in cancer management. Mater. Adv. 4 (21), 4991–5002. doi:10.1039/D3MA00525A
Khondakar, R., Tripathi, D., Mazumdar, H., Ahuja, K., and Kaushik, A. (2024). Tailored MXenes and graphene as efficient telemedicine platforms for personalized health wellness. Mater. Adv. 5, 4091–4111. doi:10.1039/D4MA00234B
Kumar, A., Gaur, N., and Aziz, N. (2024). Improving the latency for 5G/B5G based smart healthcare connectivity in rural area. Sci. Rep. 14 (1), 6976. doi:10.1038/s41598-024-57641-7
Kumar, A., Kaur, R., Gaur, N., and Aziz, N. (2024). Exploring and analyzing the role of hybrid spectrum sensing methods in 6G-based smart health care applications. F1000Research 13 (February), 110. doi:10.12688/f1000research.144624.1
Lee, S., Park, J. S., Woo, H., Yoo, Y. K., Lee, D., Chung, S., et al. (2024). Rapid deep learning-assisted predictive diagnostics for point-of-care testing. Nat. Commun. 15 (1), 1695. doi:10.1038/s41467-024-46069-2
Lima, De, Belot, D., Berkvens, R., Bourdoux, A., Dardari, D., Guillaud, M., et al. (2021). Convergent communication, sensing and localization in 6G systems: an overview of technologies, opportunities and challenges. IEEE Access 9, 26902–26925. doi:10.1109/ACCESS.2021.3053486
Liu, X., Yuk, H., Lin, S., Parada, G. A., Tang, T.-C., Tham, E., et al. (2018). 3D printing of living responsive materials and devices. Adv. Mater. 30 (4), 1704821. doi:10.1002/adma.201704821
Liu, Y., Wang, X., Zheng, G., Wan, X., and Ning, Z. (2024). An AoI-aware data transmission algorithm in blockchain-based intelligent healthcare systems. IEEE Trans. Consumer Electron. 70 (1), 1180–1190. doi:10.1109/TCE.2024.3365198
Lu, Y., and Zheng, X. (2020). 6G: a survey on technologies, scenarios, challenges, and the related issues. J. Industrial Inf. Integration 19 (September), 100158. doi:10.1016/j.jii.2020.100158
Luo, X., Chen, H.-H., and Guo, Q. (2024). LEO/VLEO satellite communications in 6G and beyond networks – technologies, applications and challenges. IEEE Netw., 1. doi:10.1109/MNET.2024.3353806
Lv, Z., Lou, R., Li, J., Singh, A. K., and Song, H. (2021). Big data analytics for 6G-enabled massive internet of things. IEEE Internet Things J. 8 (7), 5350–5359. doi:10.1109/JIOT.2021.3056128
Madani, A., Krause, B., Greene, E. R., Subramanian, S., Mohr, B. P., Holton, J. M., et al. (2023). Large Language models generate functional protein sequences across diverse families. Nat. Biotechnol. 41 (8), 1099–1106. doi:10.1038/s41587-022-01618-2
Mahmood, M. R., Abdul Matin, M., Sarigiannidis, P., and Goudos, S. K. (2022). A comprehensive review on artificial intelligence/machine learning algorithms for empowering the future IoT toward 6G Era. IEEE Access 10, 87535–87562. doi:10.1109/ACCESS.2022.3199689
Mangalik, S., Eichstaedt, J. C., Giorgi, S., Mun, J., Ahmed, F., Gill, G., et al. (2024). Robust Language-based mental health assessments in time and space through social media. Npj Digit. Med. 7 (1), 109–112. doi:10.1038/s41746-024-01100-0
Manickam, P., Mariappan, S. A., Monica Murugesan, S., Hansda, S., Kaushik, A., Shinde, R., et al. (2022). Artificial intelligence (AI) and internet of medical things (IoMT) assisted biomedical systems for intelligent healthcare. Biosensors 12 (8), 562. doi:10.3390/bios12080562
Mao, B., Tang, F., Kawamoto, Y., and Kato, N. (2022). AI models for green communications towards 6G. IEEE Commun. Surv. Tutorials 24 (1), 210–247. doi:10.1109/COMST.2021.3130901
Mathkor, D. M., Mathkor, N., Bassfar, Z., Bantun, F., Slama, P., Ahmad, F., et al. (2024). Multirole of the internet of medical things (IoMT) in biomedical systems for managing smart healthcare systems: an overview of current and future innovative trends. J. Infect. Public Health 17 (4), 559–572. doi:10.1016/j.jiph.2024.01.013
Mazumdar, H. 2023. Enhancing machine learning algorithms using GPT enhancing machine learning algorithms using GPT embeddings for binary classification embeddings for binary classification. doi:10.36227/techrxiv.22331053.v1
Mazumdar, H., Chakraborty, C., Bojja Venkatakrishnan, S., Kaushik, A., and Gohel, H. A. (2023). Quantum-inspired heuristic algorithm for secure healthcare prediction using blockchain technology. IEEE J. Biomed. Health Inf. 28, 3371–3378. doi:10.1109/JBHI.2023.3304326
Mazumdar, H., Chakraborty, C., Sathvik, MSVPJ, Jayakumar, P., and Kaushik, A. (2023). Optimizing Pix2Pix gan with attention mechanisms for AI-driven polyp segmentation in IoMT-enabled smart healthcare. IEEE J. Biomed. Health Inf., 1–8. doi:10.1109/JBHI.2023.3328962
Mazumdar, H., Chakraborty, C., Sathvik, MSVPJ, Mukhopadhyay, S., and Panigrahi, P. K. (2023). GPTFX: a novel GPT-3 based framework for mental health detection and explanations. IEEE J. Biomed. Health Inf., 1–8. doi:10.1109/JBHI.2023.3328350
Mazumdar, H., Das, S., Msvpj, S., and Reza Khondakar, K. 2024. “CliniXPromt: enhancing the comprehensibility of electronic health records using GPT-3 and chain of though.” doi:10.22541/au.171429080.04246842/v1
Mazumdar, H., Kaushik, A., and Gohel, H. A. (2023). To mitigate primary user emulation attack trajectory using cognitive single carrier frequency division multiple access approaches: towards next generation green IoT. Eng. Rep. 5 (12), e12672. doi:10.1002/eng2.12672
Mazumdar, H., Sathvik, MSVPJ, Chakraborty, C., Unhelkar, B., and Mahmoudi, S. (2024). Real-time mental health monitoring for metaverse consumers to ameliorate the negative impacts of escapism and post trauma stress disorder. IEEE Trans. Consumer Electron. 70 (1), 2129–2136. doi:10.1109/TCE.2024.3364169
Mendes, B. B., Zhang, Z., Conniot, J., Sousa, D. P., Ravasco, J. M. J. M., Onweller, L. A., et al. (2024). A large-scale machine learning analysis of inorganic nanoparticles in preclinical cancer research. Nat. Nanotechnol. 19, 867–878. doi:10.1038/s41565-024-01673-7
Meng, L., Liu, S., Ata Borsa, B., Eriksson, M., and Mak, W. C. (2024). A conducting polymer-based array with multiplex sensing and drug delivery capabilities for smart bandages. Commun. Mater. 5 (1), 28–10. doi:10.1038/s43246-024-00469-5
Meskó, B., and Topol, E. J. (2023). The imperative for regulatory oversight of Large Language Models (or generative AI) in healthcare. Npj Digit. Med. 6 (1), 120–126. doi:10.1038/s41746-023-00873-0
Mohjazi, L., Selim, B., Tatipamula, M., and Imran, M. 2023. The journey towards 6G: a digital and societal revolution in the making. doi:10.36227/techrxiv.24456772.v1
Moor, M., Banerjee, O., Abad, Z. S. H., Krumholz, H. M., Leskovec, J., Topol, E. J., et al. (2023). Foundation models for generalist medical artificial intelligence. Nature 616 (7956), 259–265. doi:10.1038/s41586-023-05881-4
Nath, S. S., Sadagopan, S., Vijendra Babu, D., Dinesh Kumar, R., Jonnala, P., and Murthy, M. Y. B. (2023). Block chain-based security and privacy framework for point of care health care IoT devices. Soft Comput. doi:10.1007/s00500-023-07932-4
Nayak, S., and Patgiri, R. (2021). “6G communication technology: a vision on intelligent healthcare,” in Health informatics: a computational perspective in healthcare. Editors R. Patgiri, A. Biswas, and R. Pinki (Singapore: Springer), 1–18. doi:10.1007/978-981-15-9735-0_1
Oz, T., Kumar Kaushik, A., and Kujawska, M. (2023). Advances in graphene-based nanoplatforms and their application in Parkinson’s disease. Mater. Adv. 4 (24), 6464–6477. doi:10.1039/D3MA00623A
Peng, C., Yang, Xi, Chen, A., Smith, K. E., PourNejatian, N., Costa, A. B., et al. (2023). A study of generative Large Language model for medical research and healthcare. Npj Digit. Med. 6 (1), 210–10. doi:10.1038/s41746-023-00958-w
Rahman, A., Wadud, Md A. H., Islam, Md J., Kundu, D., Amir-Ul-Haque Bhuiyan, T. M., Muhammad, G., et al. (2024). Internet of medical things and blockchain-enabled patient-centric agent through SDN for remote patient monitoring in 5G network. Sci. Rep. 14 (1), 5297. doi:10.1038/s41598-024-55662-w
Rosic, G. (2024). Cancer signaling, cell/gene therapy, diagnosis and role of nanobiomaterials. Adv. Biol. Earth Sci. 9 (Special Issue), 11–34. doi:10.62476/abes9s11
Salameh, A. I., and Mohamed, El T. (2022). From 5G to 6G—challenges, technologies, and applications. Future Internet 14 (4), 117. doi:10.3390/fi14040117
Samsung Electronics (2022). Samsung electronics Unfolds the next generation communications technology at the First Samsung 6G Forum. Available at: https://news.samsung.com/global/samsung-electronics-unfolds-the-next-generation-communications-technology-at-the-first-samsung-6g-forum (Accessed May 14, 2024).
Sarkar, P., Saha, A., Banerjee, A., Banerjee, A., Seteikin, A. Y., and Samusev, I. G. (2022). Review on the evolution of 6G and Terahertz communication for highspeed information processing. Bull. Russ. Acad. Sci. Phys. 86 (1), S166–S170. doi:10.3103/S1062873822700617
Saroğlu, H. E., Shayea, I., Saoud, B., Hadri Azmi, M., El-Saleh, A. A., Ali Saad, S., et al. (2024). Machine learning, IoT and 5G technologies for breast cancer studies: a review. Alexandria Eng. J. 89 (February), 210–223. doi:10.1016/j.aej.2024.01.043
Sel, K., Osman, D., Huerta, N., Edgar, A., Pettigrew, R. I., and Jafari, R. (2023). Continuous cuffless blood pressure monitoring with a wearable ring bioimpedance device. NPJ Digit. Med. 6 (1), 59. doi:10.1038/s41746-023-00796-w
Sempionatto, J. R., Lasalde-Ramírez, J. A., Mahato, K., Wang, J., and Gao, W. (2022). Wearable chemical sensors for biomarker discovery in the omics Era. Nat. Rev. Chem. 6 (12), 899–915. doi:10.1038/s41570-022-00439-w
Shen, X., Gao, J., Li, M., Zhou, C., Hu, S., He, M., et al. (2023). Toward immersive communications in 6G. Front. Comput. Sci. 4 (January). doi:10.3389/fcomp.2022.1068478
Shin, J. H., Kwon, J., Kim, J.Uk, Ryu, H., Ok, J., Kwon, S. J., et al. (2022). Wearable EEG electronics for a brain–AI closed-loop system to enhance autonomous machine decision-making. Npj Flex. Electron. 6 (1), 32–12. doi:10.1038/s41528-022-00164-w
Shrikrishna, S., Sharma, R., Sahoo, J., Kaushik, A., and Gandhi, S. (2024). Navigating the landscape of optical biosensors. Chem. Eng. J. 490 (June), 151661. doi:10.1016/j.cej.2024.151661
Smith, A. A., Li, R., and Tse, Z. T.Ho (2023). Reshaping healthcare with wearable biosensors. Sci. Rep. 13 (1), 4998. doi:10.1038/s41598-022-26951-z
Srinivasu, P. N., Ijaz, M. F., Shafi, J., Woźniak, M., and Sujatha, R. (2022). 6G driven fast computational networking framework for healthcare applications. IEEE Access 10, 94235–94248. doi:10.1109/ACCESS.2022.3203061
Sun, Z., Zhu, M., Shan, X., and Lee, C. (2022). Augmented tactile-perception and haptic-feedback rings as human-machine interfaces aiming for immersive interactions. Nat. Commun. 13 (1), 5224. doi:10.1038/s41467-022-32745-8
Tang, C., Yi, W., Occhipinti, E., Dai, Y., Gao, S., and Occhipinti, L. G. (2024). A roadmap for the development of human body digital twins. Nat. Rev. Electr. Eng. 1 (3), 199–207. doi:10.1038/s44287-024-00025-w
Tang, X., Zhao, L., Chong, J., You, Z., Zhu, L., Ren, H., et al. (2022). 5G-Based smart healthcare system designing and field trial in hospitals. IET Commun. 16 (1), 1–13. doi:10.1049/cmu2.12300
Umer, M., Aljrees, T., Karamti, H., Ishaq, A., Alsubai, S., Omar, M., et al. (2023). Heart failure patients monitoring using IoT-based remote monitoring system. Sci. Rep. 13 (1), 19213. doi:10.1038/s41598-023-46322-6
Uwaoma, C. (2023). Securing healthcare systems in the Era of 6G networks: a perspective on the enabling technologies. Int. J. Appl. Inf. Syst. 12 (42), 36–42. doi:10.5120/ijais2023451954
Vaghasiya, J. V., Mayorga-Martinez, C. C., and Pumera, M. (2023). Wearable sensors for telehealth based on emerging materials and nanoarchitectonics. Npj Flex. Electron. 7 (1), 26–14. doi:10.1038/s41528-023-00261-4
Venkatesh, K. P., Raza, M. M., and Kvedar, J. C. (2022). Health digital twins as tools for precision medicine: considerations for computation, implementation, and regulation. Npj Digit. Med. 5 (1), 150–152. doi:10.1038/s41746-022-00694-7
Wang, Ge, Badal, A., Jia, X., Maltz, J. S., Mueller, K., Myers, K. J., et al. (2022). Development of metaverse for intelligent healthcare. Nat. Mach. Intell. 4 (11), 922–929. doi:10.1038/s42256-022-00549-6
Wang, G., Gu, H., Li, X., Yu, Z., Li, O., Liu, Q., et al. (2022). Terahertz sensing and communication towards future intelligence connected networks. Available at: https://www.semanticscholar.org/paper/Terahertz-Sensing-and-Communication-Towards-Future-Wang-Gu/baf637825e04f792f91dc66a869299ac592aef00.
Wang, N., Bian, J., Li, Y., Li, X., Mumtaz, S., Kong, L., et al. (2024). Multi-purpose RNA Language modelling with motif-aware pretraining and type-guided fine-tuning. Nat. Mach. Intell. 6 (May), 548–557. doi:10.1038/s42256-024-00836-4
Waseem, M., and Hassan, S. (2021). TaNTIN: terrestrial and non-terrestrial integrated networks-A collaborative technologies perspective for beyond 5G and 6G. Internet Technol. Lett. 7. doi:10.1002/itl2.274
Wei, X., Liang, X., Meng, C., Cao, S., Shi, Q., and Wu, J. (2023). Multimodal electronic textiles for intelligent human-machine interfaces. Soft Sci. 3 (2), 17. doi:10.20517/ss.2023.09
Wornow, M., Xu, Y., Thapa, R., Patel, B., Steinberg, E., Scott, F., et al. (2023). The shaky foundations of Large Language Models and foundation models for electronic health records. Npj Digit. Med. 6 (1), 135–210. doi:10.1038/s41746-023-00879-8
Xu, W., Yang, Z., Ng, D. W. K., Levorato, M., Eldar, Y. C., and Debbah, M. (2023). Edge learning for B5G networks with distributed signal processing: semantic communication, edge computing, and wireless sensing. IEEE J. Sel. Top. Signal Process. 17 (1), 9–39. doi:10.1109/JSTSP.2023.3239189
Yang, D., Zhou, J., Chen, R., Song, Y., Song, Z., Zhang, X., et al. (2022). Expert consensus on the metaverse in medicine. Clin. eHealth 5 (December), 1–9. doi:10.1016/j.ceh.2022.02.001
Yao, K., Zhou, J., Huang, Q., Wu, M., Yiu, C.Ki, Li, J., et al. (2022). Encoding of tactile information in hand via skin-integrated wireless haptic interface. Nat. Mach. Intell. 4 (10), 893–903. doi:10.1038/s42256-022-00543-y
Yasmin, I. A., and Khondakar, K. R. (2024). “Chapter 10 - nanomicrofluidics-based sensors for drug and therapy management,” in Next-generation smart biosensing. Editors K. R. Khondakar, and A. Kumar Kaushik (USA: Academic Press), 261–286. doi:10.1016/B978-0-323-98805-6.00012-9
Yin, Z., Yang, Y., Hu, C., Li, J., Qin, B., and Yang, X. (2024). Wearable respiratory sensors for health monitoring. NPG Asia Mater. 16 (1), 8–29. doi:10.1038/s41427-023-00513-9
Zawish, M., Dharejo, F., Khowaja, S., Dev, K., Davy, S., Qureshi, N. M. F., et al. 2022. AI and 6G into the metaverse: fundamentals, challenges and future research trends. doi:10.48550/arXiv.2208.10921
Zhang, J., Liu, L., Xiang, P., Fang, Q., Nie, X., Ma, H., et al. (2024). AI Co-pilot bronchoscope robot. Nat. Commun. 15 (1), 241. doi:10.1038/s41467-023-44385-7
Zhang, Z., Wen, F., Sun, Z., Guo, X., He, T., and Lee, C. (2022). Artificial intelligence-enabled sensing technologies in the 5G/internet of things Era: from virtual reality/augmented reality to the digital twin. Adv. Intell. Syst. 4 (7), 2100228. doi:10.1002/aisy.202100228
Keywords: 5th-generation sensing, 6th-generation sensing, nanotechnology, smart sensing, personalized sensing, sensing-to-sense
Citation: Mazumdar H, Khondakar KR, Das S and Kaushik A (2024) Aspects of 6th generation sensing technology: from sensing to sense. Front. Nanotechnol. 6:1434014. doi: 10.3389/fnano.2024.1434014
Received: 22 May 2024; Accepted: 24 June 2024;
Published: 11 July 2024.
Edited by:
Themis Prodromakis, University of Edinburgh, United KingdomReviewed by:
Parviz Vahedi, Maragheh University of Medical Sciences, IranCopyright © 2024 Mazumdar, Khondakar, Das and Kaushik. This is an open-access article distributed under the terms of the Creative Commons Attribution License (CC BY). The use, distribution or reproduction in other forums is permitted, provided the original author(s) and the copyright owner(s) are credited and that the original publication in this journal is cited, in accordance with accepted academic practice. No use, distribution or reproduction is permitted which does not comply with these terms.
*Correspondence: Hirak Mazumdar, aGlyYWttLnRlY2guZWNlQGdtYWlsLmNvbQ==; Ajeet Kaushik, YWplZXQubnBsQGdtYWlsLmNvbQ==
†ORCID: Hirak Mazumdar, orcid.org/0000-0001-6469-1515