- 1Student Research Committee, School of Medicine, Shahroud University of Medical Sciences, Shahroud, Iran
- 2Department of Chemistry, University of Birjand, Birjand, Iran
- 3Department of Pharmaceutics and Pharmaceutical Nanotechnology, School of Pharmacy, Birjand University of Medical Sciences, Birjand, Iran
- 4Department of Basic Sciences, School of Medicine, Shahroud University of Medical Sciences, Shahroud, Iran
Nanomaterials, especially graphene derivatives, have become major tools in the biomedical area. Understanding the way that graphene interacts with component elements of biological systems, like biological membranes, is critical for the development of successful biomedical applications. The interaction mechanism of graphene sheets with a model cell membrane was investigated in this study using molecular dynamics (MD) simulations under three different conditions: pristine graphene (PG), carboxyl group-functionalized graphene (G-COOH), and amine group-functionalized graphene (G-NH2). The MD simulations demonstrated that functional groups on graphene surfaces improve their interaction with the head groups of the membrane. In 200 nanoseconds, PG reached equilibrium outside and near the phospholipid membrane. G-NH2 was positioned away from the model membrane’s surface, while G-COOH and G-NH2 also achieved equilibrium outside the membrane. It was shown by molecular dynamics simulations that after 200 ns, all three systems had attained their stable states. Crucially, it is discovered that the kind of functional groups greatly affected how the nanoparticles and membrane interacted. Each and every nanocarrier has a strong propensity to break through the membrane. The van der Waals interaction energies for the PG, G-NH2, and G-COOH systems were further shown by the obtained data to be roughly −400.66, −397.52, and −876.36 kJ/mol, respectively. These results support the notion that G-COOH interacts with the model cell bilayer more strongly than PG and G-NH2. This work highlights the influence of functional groups on the interaction of graphene sheets with biological membranes, offering important insights into the equilibrium behavior and entry mechanism of graphene sheets in a model cell membrane.
1 Introduction
With its hexagonal honeycomb lattice structure, graphene is a sheet of carbon atoms with exceptional mechanical and electrical properties (Geim and Novoselov, 2007). High density of delocalized electrons in the two-dimensional (2D) honeycomb lattice of pristine graphene (PG) yields an excellent surface area with remarkable mechanical, electrical, and photothermal capabilities (Novoselov et al., 2004). Because of these special properties, graphene holds a great deal of promise for a variety of in vivo and in vitro therapeutic and diagnostic biomedical applications, including as biosensing, tissue engineering, and drug transport (Novoselov et al., 2012; Geetha Bai et al., 2019; Lawal, 2019).
However, because of its hydrophobic nature, the PG’s biological and biomedical value is restricted. The most practical and effective method for increasing graphene’s water dispersibility is to chemically oxidize graphene sheets, producing graphene oxide (GO), a significant derivative of graphene. Because of their high concentration of chemical groups that contain oxygen, graphene oxide (GO) sheets often exhibit good hydrophilicity. As a result, there has been significant interest in GO sheets as potential biomedical applications (Reina et al., 2017). Because GO’s surface contains groups that contain oxygen, it is simple to add functional groups to it, including hyaluronic acid (Pramanik et al., 2019), polyethylene glycol (Kazempour et al., 2019), and chitosan (Chen et al., 2013).
Through a variety of chemical interactions, GO and its derivatives come into direct contact with various biosystem compartments in the case of in vivo applications, such as lipid bilayers (Zhang et al., 2017). Thus, it is critical to comprehend how functionalized GO interacts at the molecular level with biological components.
Molecular dynamics (MD) simulations are widely employed in the study of the interactions between biological macromolecules and nanomaterials. Numerous advantages come with this approach, such as its affordability and capacity to investigate interactions at the atomic level. Researchers can learn important things about complicated systems that would be costly or difficult to investigate experimentally by using computer simulations. MD simulations emphasize the value of computational methods in scientific study by providing a strong means of comprehending the basic laws that underpin the behavior of nanomaterials and their interactions with biological macromolecules. These simulations make it possible to see atoms, which makes it possible to observe in great detail how nanoparticles and biomolecules—like proteins, DNA, and cell membranes—interact (Chen et al., 2016; Willems et al., 2017).
Kong et al. investigated the impact of shape on graphene quantum dot (GQD) penetration into a POPC (1-palmitoyl-2-oleoyl-sn-glycero-3-phosphocholine) cell membrane using 100 ns MD simulation (Kong et al., 2021). They looked at how different shaped and sized GQDs moved within the simulated membrane. Each and every GQD pierced the membrane in the simulations. Prior to bending their structure and penetrating the POPC membrane, the GQDs were first absorbed parallel to the membrane.
The impact of functionalizing graphene with various chemical groups on the administration of the medication doxorubicin was examined by Mirhosseini et al. (Mirhosseini et al., 2017). The hydroxyl, carboxyl, methyl, and amine groups functionalized the graphene surface in this investigation. The findings of this study demonstrated that the doxorubicin molecule interacts more strongly with carboxyl-functionalized graphene, and that hydrogen atoms on the surface of functionalized graphene nanosheets with hydroxyl, carboxyl, and amine groups can form hydrogen bonds with the oxygen and nitrogen atoms present in the doxorubicin molecule. Ji et al. showed in a different study that graphene oxide (GO) and graphene-based nanomaterials promote therapeutic molecules’ penetration into cell membranes (Ji et al., 2021).
In our earlier work, we used molecular dynamics (MD) simulations to investigate the interactions between modified carbon nanotubes (CNTs)—aminated and carboxylated CNTs, in particular—and a cell membrane model. According to our research, every nanotube was able to pass through the membrane. It was found that the penetration process was highly impacted by the type of functional group that was affixed to the nanotube. In comparison to pristine and aminated CNTs, carboxylated CNTs demonstrated a stronger interaction with the lipid bilayer, as demonstrated by the increased interaction between functionalized CNTs and the head groups of the membrane caused by the presence of functional groups on the nanotubes’ surface (Kiani et al., 2023). Additionally, in order to better understand the toxicity mechanism and antibacterial and antibiofilm activities of these three types of graphene-based nanomaterials, we carried out an experimental comparison between Graphene Oxide (GO), Reduced Graphene Oxide (RGO), and Carboxylated Graphene Oxide (GO-COOH). Standardized strains of all three microbes were used. The most effective antibacterial characteristics of graphene were found in its carboxylated and more oxidized form, according to the results of our antimicrobial tests (Saedi et al., 2022; Shirshahi et al., 2023).
This work examines the POPC bilayer using a series of molecular dynamics (MD) simulations. The study specifically examined the molecular interactions between functionalized graphene (G-NH2 and G-COOH) and virgin graphene (PG) with POPC. We anticipate that the findings of this study will contribute to a better understanding of the interactions between graphene nanosheets and cell membranes, providing information for the development of novel nanocarriers that may find use in drug delivery and biomedicine.
2 Computational methods
This study’s graphene model was modified from one that was conducted earlier (Zaboli et al., 2023). The model comprises 276 carbon atoms in total, with hydrogen atoms incorporated to passivate the edge carbon atoms. Based on the Lerf-Klinowski model (Lerf et al., 1998), the G-COOH structures were formed by dotting the graphene nanosheet with 15 carboxyl, 30 hydroxyl, and 30 epoxy groups. Based on a model that was published in earlier research, the G-NH2 was built (Aguilar-Bolados et al., 2017; Rabchinskii et al., 2020; Zahid et al., 2021). To add functional groups to G-COOH and G-NH2, the Gauss View software was utilized.
After equilibrating for 20 ns, the standardized lipid membrane was acquired via CHARMM-GUI (Wu, 2014; Witzke et al., 2016). 200 1-palmitoyl-2-oleoyl-sn-glycero-3-phosphocholine (POPC, Table 1) are present in each leaflet of this membrane.
The graphene nanoparticles were deposited exactly above the cell membrane, with a gap of 2 nm between them and the centroid of the membrane. The simulation boxes were filled using the TIP3P water model (Jorgensen et al., 1983) Furthermore, a particular quantity of ions (Na+, Cl−) were supplied to the simulated boxes in order to neutralize the system and recreate a biologically appropriate environment (i.e., 0.15 mol L−1) (Wu, 2014). The structural features of the graphene- and POPC-based phospholipid nanosheets are shown in Figure 1. Table 2 contains comprehensive details on the MD simulation systems.
The charmm36 force field (Lee et al., 2016) served as the source of the force field parameters for each component. The following equations were used to define the words “angle bending” and “bond stretching”:
Stretching a bond:
Bending an angle:
The force constants related to bond and angle forces, respectively, are denoted by the variables kb and kθ in the provided equations. The bond length and bond angle are denoted by the variables b and θ, respectively, and the equilibrium values for these terms are denoted by b0 and θ0.
The following equation was used to numerically represent the torsional potential:
In this case, the dihedral angles and force constants are denoted by φ and kφ, respectively. The multiplicity, represented by n, and phase, represented by δ, dictate the potential’s minima and maxima’s locations.
The Lenard-Jones (LJ) potential was used to characterize the van der Waals (vdW) interactions:
In Eq. 4,
The steepest descent algorithm was used for the first minimization of the simulated systems. Two 200 picosecond (ps) equilibration procedures were conducted within the NVT (canonical ensemble) and NPT (isothermal-isobaric ensemble) ensembles to achieve consistent temperature and pressure settings. The Nose-Hoover thermostat (Abraham et al., 2015) was used in the NVT ensemble to maintain temperature stability, while the Parrinello-Rahman barostat (Kučerka et al., 2011) was used in the NPT ensemble to maintain a constant pressure of 1 atm (atm) and a temperature of 310 K (K).
The simulation systems underwent an initial minimization process utilizing the steepest descent algorithm. To establish consistent temperature and pressure conditions, two equilibration procedures were carried out within the NVT (canonical ensemble) and NPT (isothermal-isobaric ensemble) ensembles, each lasting for a duration of 200 picoseconds (ps). To ensure temperature stability, the Nose-Hoover thermostat (Jorgensen et al., 1983) was implemented in the NVT ensemble, while the Parrinello-Rahman barostat (Lee et al., 2016), was utilized in the NPT ensemble to maintain a constant temperature of 310 K (K) and a pressure of 1 atm (atm).
The GROMACS software package, version 2019.2, was utilized for all MD simulations (Abraham et al., 2015). Periodic boundary conditions were applied along all three spatial directions, and the simulations lasted 200 nanoseconds (ns). To ensure accuracy, the simulations used a very tiny integration time step of 1.5 femtoseconds (fs). The bond lengths were successfully constrained and kept at their equilibrium positions by using the LINCS method (Hess et al., 1997). Van der Waals interactions were evaluated at a cutoff distance of 1.4 nm (nm), and electrostatic forces were precisely calculated using the particle mesh Ewald (PME) method (Darden et al., 1993). The VMD program was then used to visualize and analyze the results of the simulation (Humphrey et al., 1996).
3 Results and discussion
For both the lipid membrane with and without graphene nanosheets, the area per lipid (APL), which denotes the precise area occupied by a lipid, is computed. The APL for the two lipid bilayers is found to be 67.73 Å2/lipid by dividing the simulation box area in the xy plane by the number of POPC lipids in each row. This value is in close agreement with experimental data obtained through X-ray scattering and suggests that the lipid force field CHARMM36 is well parameterized (Kučerka et al., 2011).
To fully understand the interactions of the PG, G-NH2, and G-COOH with the lipid bilayer membrane, three different simulation systems are created.
The diffusion mechanism of the PG, G-NH2, and G-COOH models into the lipid bilayer was investigated by a number of investigations. Taking pictures of the system, measuring the number of contacts, calculating the van der Waals (vdW) energy, and monitoring the center of mass (COM) distance in the z-direction (dz) between the nanomaterials and the membrane over the course of the simulation were all included in these analyses. Figure 2 through Figure 4 contain comprehensive data and graphic representations that demonstrate these analyses.
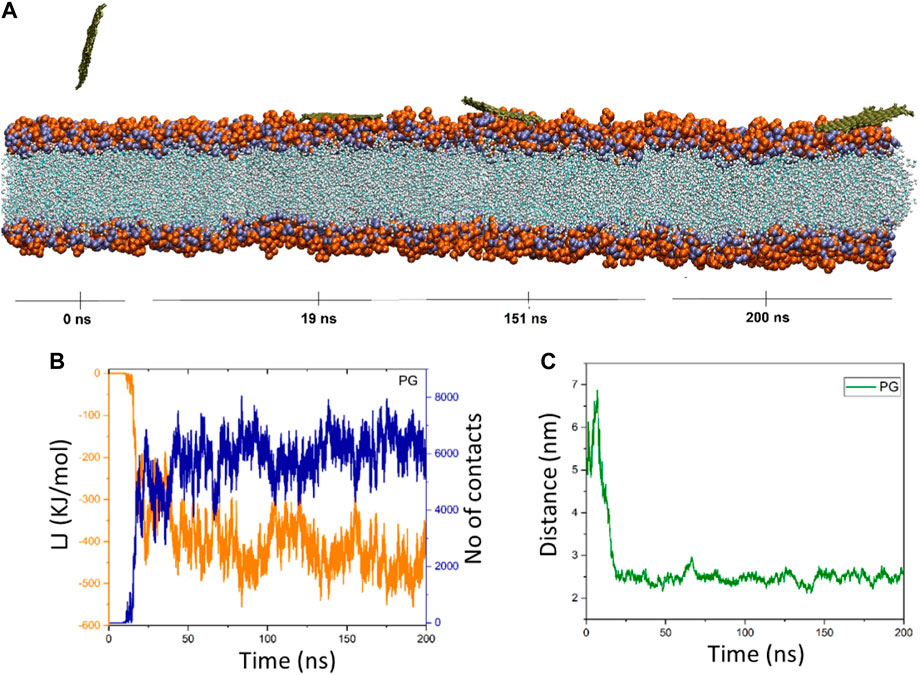
Figure 2. (A) Provided snapshots of the interaction between the PG nanosheet and the membrane at different times. (B) van der Waals energy between PG and the cellular membrane (orange color), as well as the number of contacts between the PG and the membrane (blue color). (C) Center of mass (COM) distance between the PG and the membrane as a function of simulation time.
3.1 PG’s interaction with POPC
Upon closer inspection, Figure 2B showed that in the time interval of 0–11 ns (≈0 kJ/mol), there is no discernible energy interaction between the membrane and the PG nanosheet. This discovery validates that the nanosheet is submerged in water over this duration, and the early consequences have been circumvented. The interactions between the POPC membrane and the PG gradually increase and fluctuate around a mean value as the simulation goes on. In addition, there are more and more interactions between the membrane and the PG. At t = 175 ns, the energy reaches its lowest state of −559.36 kJ/mol. The energy of the nanosheet then drops to −389.06 kJ/mol at the end of the simulation duration (t = 200 ns) with ongoing oscillations (Figure 2C). The pure graphene nanosheet has moved from the water towards the membrane, where PG fluctuates at the water-lipid interface and dz drops from 5.4 nm to 2.25 nm (at t = 42 ns), according to the center of mass distance graph. It is located 2.7 nm away from the membrane model at the conclusion of the simulation duration (t = 200 ns) (Figure 2C). The system snapshots that are provided at different times verify these observations.
3.1.1 G-NH2’s interaction with POPC
There is no contact between the membrane and G-NH2 at the start of the simulation (from 0 ns to 19 ns), just like in the graphene system, as shown in Figure 3. This demonstrates that the nanosheet was indeed present in the water at this period. The interactions between the POPC membrane and the G-NH2 nanosheet gradually increased and varied about the average value as the simulation went on. At t = 190 ns, the energy reaches its lowest state, which is −236.94 kJ/mol. After that, with continuous fluctuations, the energy of the aminated graphene nanosheet drops to −159.69 kJ/mol at the end of the simulation duration (t = 200 ns). The aminated graphene nanosheet containing amino groups travels from the water towards the membrane, where dz drops from 5.4 nm to 3.18 nm, based on the center of mass distance graph. The images that were supplied demonstrated how the G-NH2 entered the membrane in the same manner and maintained its vertical shape. This result showed that the knife impact of G-NH2 on the membrane has enhanced even though its interaction energy has decreased.
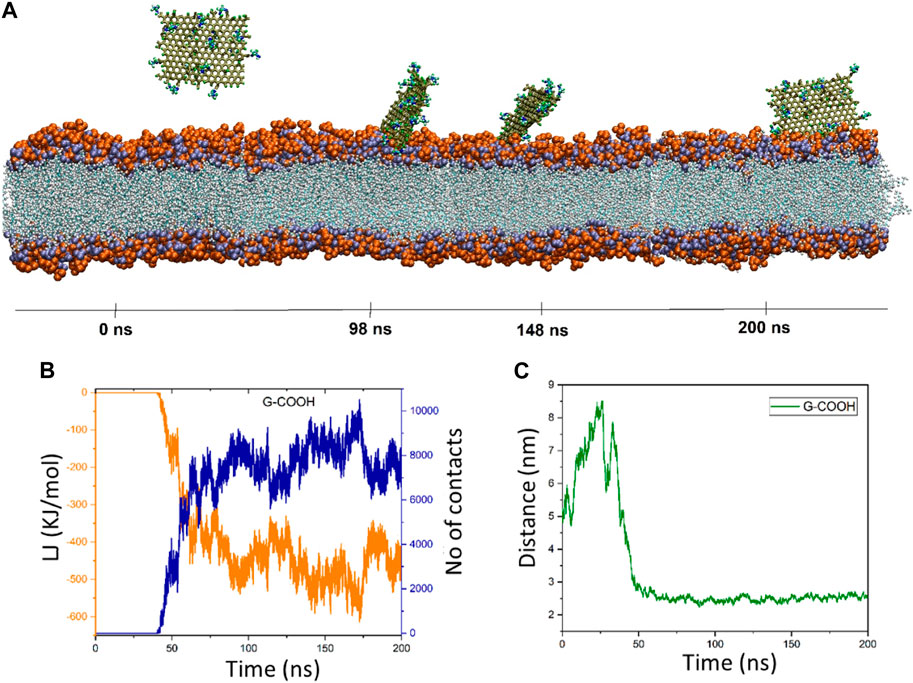
Figure 3. (A) Presented momentary views of the G-NH2 nanosheet-membrane interaction at various moments. The orange van der Waals energy and the blue hue represent the number of interactions between G-NH2 and the cellular membrane, respectively, in (B). (C) The G-NH2 and membrane center of mass (COM) distance as a function of simulation time.
3.2 G-COOH’s interaction with POPC
Graphene oxide’s behavior is fascinating. The distance figure demonstrates how the nanosheet separates from the membrane during a period of ∼25 ns, reaching a distance of ∼8.5 nm before dropping to less than 6 ns at ∼30 ns. The distance increases once again between 30 and 40 ns, reaching 7.9 nm. Following that, the graphene oxide begins to naturally migrate in the direction of the membrane, forming the first contacts between the nanosheet and the membrane. It should be mentioned that because the graphene oxide was outside of the cut-off radius, the energy diagram and the number of contacts exhibit these changes (Figure 4B). However, it is evident from this diagram that the interaction formed after 40 nanoseconds. The energy graph shows a dramatic drop in time from 40 to roughly 90 ns, resulting in an energy value of −500 kJ/mol. After roughly 66 nanoseconds, as seen in Figure 4A, graphene oxide is positioned parallel to the membrane. The figures show fluctuations around the average values starting at 90 ns, indicating that graphene oxide maintains its parallel state.
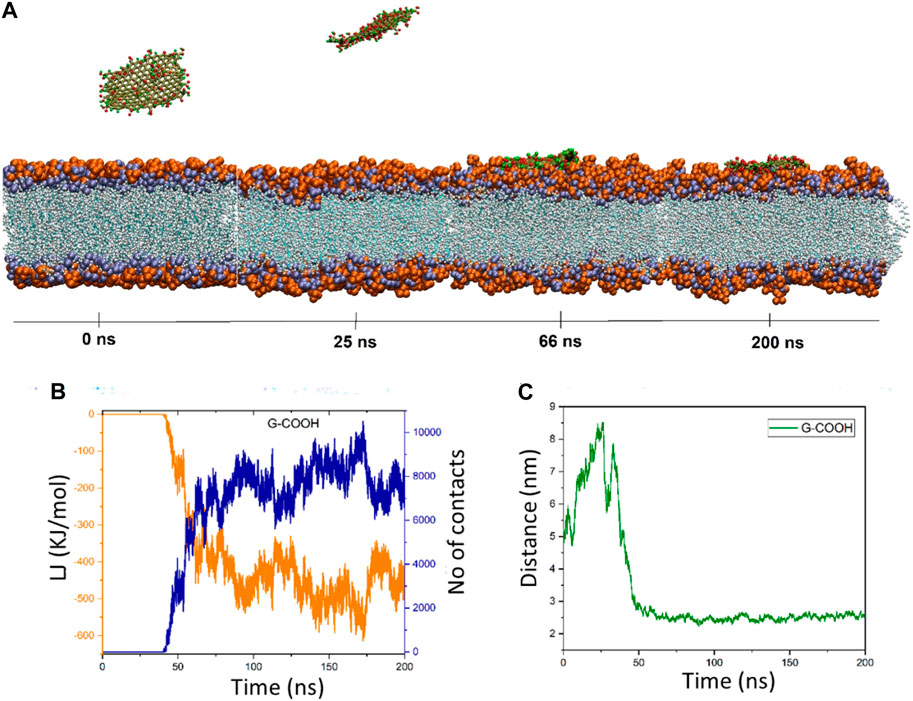
Figure 4. (A) Presented momentary views of the G-COOH nanosheet-membrane interaction at various moments. (B) The amount of van der Waals energy (orange color) and the number of contacts (blue color) between the cellular membrane and G-COOH. (C) The center of mass (COM) separation as a function of simulation time between the membrane and the G-COOH.
3.3 Comparison of simulation systems
At the start (0 ns) and finish (200 ns) of the simulation period, the computed van der Waals (vdW), electrostatic (Ele), and total (vdW + ele) energies between the various components of the simulation systems were examined (Table 3).
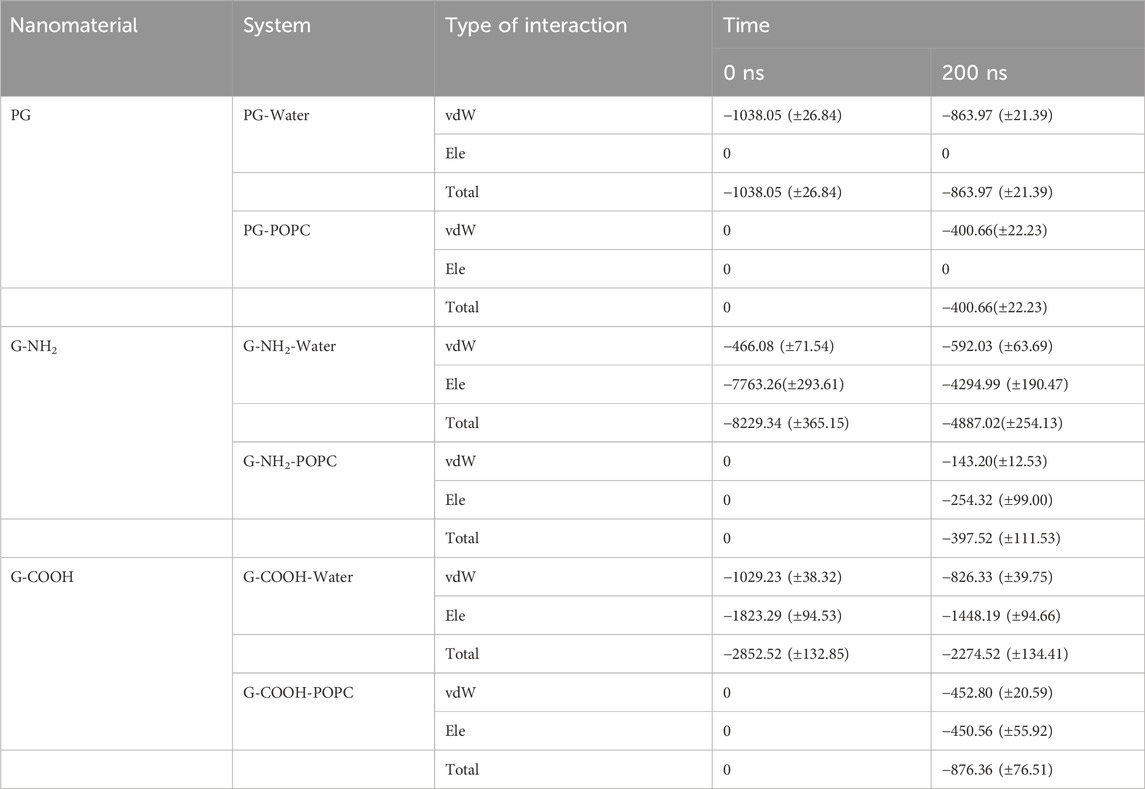
Table 3. Total energy terms (kJ/mol), van der Waals (vdW), and electrostatic (ele) terms between the various simulated system pairs at the start and finish of the MD simulation.
For PG, G-NH2, and G-COOH, the total interaction energy values with the model membrane were roughly −400.66 (±22.23), −397.52 (±111.53), and −876.36 (±76.51) kJ/mol, in that order. It is discovered that, in comparison to PG and G-NH2, the energy of G-COOH with POPC is roughly 119% and 120% greater, respectively. Compared to the other systems, this data suggests that the carboxylated graphene nanosheet interacts with the membrane more firmly (Figure 5C). Our findings suggest that the strong contact between the polar head groups of POPC molecules and the graphene-based nanomaterials can lead to the adsorption of functionalized graphene-based nanomaterials onto the membrane, where the Ele energy plays a significant role. As such, these interactions impact the stability of graphene structures on the POPC membrane that have carboxyl and amine groups (Figure 5). Moreover, the contribution of van der Waals and electrostatic energy to the interactions between the functionalized graphene nanosheets and water is less when they migrate from the aqueous phase towards the membrane. It is discovered that functionalized graphenes have a strong solvent interaction, primarily due to electrostatic attraction. Because G-NH2 interacts with water the strongest, it may be the reason for its vertical orientation (Figure 3). G-NH2 can interact with the membrane and has a strong interaction with water in this position.
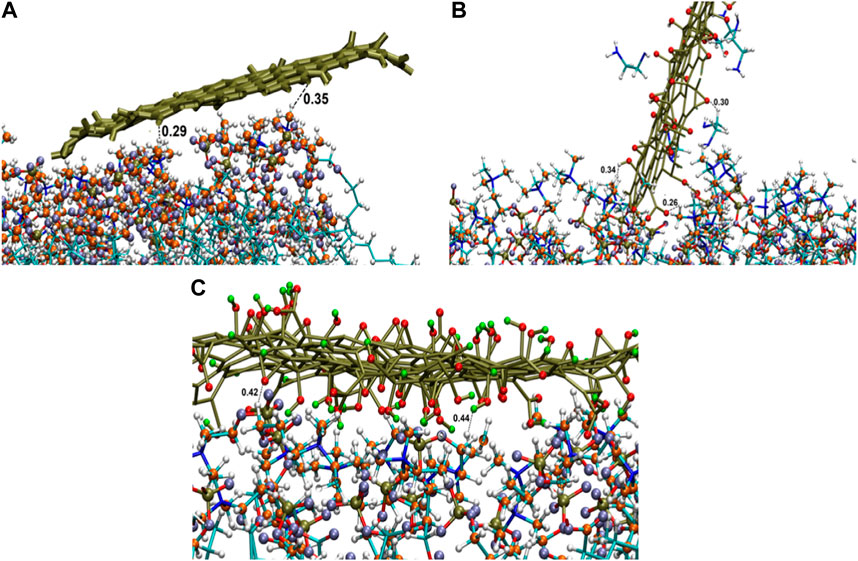
Figure 5. Interactions of graphene sheet with different functionalities with POPC head groups (A) PG, (B) G-NH2, and (C) G-COOH nanosheets at the end of the simulation.
Density profiles along the z-axis are shown in Figure 6 to help grasp the distribution of various components in the systems under study. Using the “gmx density” module, the bilayer lipid membrane was selected as the reference for the density computation. The density profile study showed that the POPC membrane correctly equilibrated during a 200 nanosecond period. Moreover, this research validates that the three graphenes approached the membrane without causing any damage to the hydration layer.
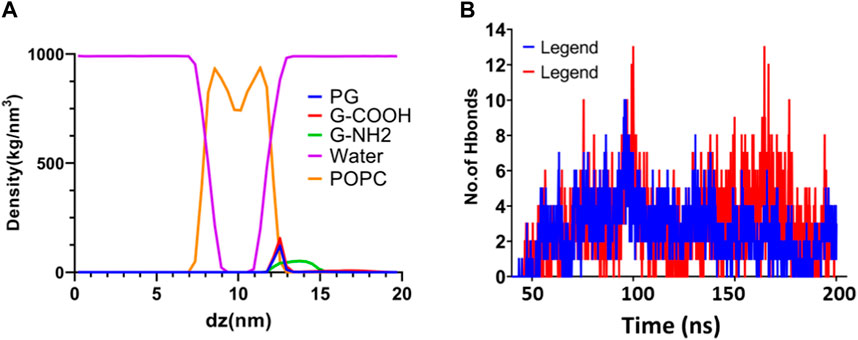
Figure 6. (A) Density profiles following a 200 ns simulation of water, graphene, functionalized graphenes, and POPC. (B) The quantity of H bonds that form between POPC and the G-NH2 and G-COOH nanosheets during the simulation.
The dispersion of functionalized graphene nanosheets inside the lipid bilayer of the model membrane is largely dependent on hydrogen bonding. It is a useful instrument for researching the natural factors that contribute to the penetration process. We used the “gmx hbond” tool with a 3.5-Å cut-off for the donor-acceptor distance to assess the number of hydrogen bonds between functionalized graphene nanosheets and the membrane. The results of this analysis are shown in Figure 6. Our goal was to identify hydrogen bonding by establishing criteria based on the acceptor-hydrogen-donor angle and the hydrogen-acceptor distance. Functional groups like NH and OH act as hydrogen donors, whereas oxygen and nitrogen, which are not linked to hydrogen, act as hydrogen acceptors. The high number of hydrogen bonds that the functionalized graphene nanosheets (amine and carboxyl) exhibit with the membrane highlights the importance of this interaction in the interface between these nanosheets and the lipid bilayer. Interestingly, carboxylated graphene shows a stronger inclination to pierce the lipid bilayer, probably because this system has more hydrogen bonds. All things considered, the results emphasize how crucial hydrogen bonding is to the way functionalized graphene nanosheets interact with the lipid bilayer.
The observed variations in interaction mechanisms between PG, G-COOH, and G-NH2 with model cell membranes bear significant implications for the field of drug delivery. Our study unveils that the incorporation of functional groups, such as carboxyl groups in the case of G-COOH, substantially amplifies the interaction between graphene sheets and the membrane’s head groups. This heightened interaction holds promise for designing more efficient drug delivery systems, wherein functionalized graphene acts as a carrier, facilitating targeted delivery to specific cells or tissues.
Moreover, understanding the equilibrium behavior and entry mechanism of graphene sheets into a model cell membrane is imperative when gauging the potential toxicity of these nanomaterials. In the context of G-COOH, the introduction of carboxyl groups enhances the hydrophilicity and biocompatibility of graphene derivatives, potentially reducing adverse reactions and minimizing inflammatory responses (Sasidharan et al., 2011). Additionally, the negative charge conferred by carboxyl groups may alter the interactions with cell membranes, influencing cellular uptake, membrane disruption, and overall cytotoxicity. Furthermore, the hydrogen bonds and electrostatic interactions formed by carboxyl groups may affect the binding and recognition of G-COOH by biological molecules, influencing its behavior within cellular environments. Therefore, properly engineered functional groups can reduce unwanted interactions and enhance the selectivity of the nanomaterial.
Our experimental tests assessing the antimicrobial properties of graphene with various functional groups demonstrated that the most potent antibacterial characteristics were observed in graphene when it was carboxylated and more oxidized (Saedi et al., 2022; Shirshahi et al., 2023). This experimental data validate the crucial role of functional groups in influencing the antibacterial effects of carboxylated and highly oxidized graphenes. A comparative analysis between the present study and our experimental data leads to the conclusion that the presence of oxygen-containing functional groups on graphene sheets fosters more stable interactions with bacterial cell walls. This heightened stability is directly correlated with a higher content of oxygen-containing functional groups. As a result, these interactions have the potential to disrupt bacterial cell walls through various mechanisms (Pulingam et al., 2021), including physical damage caused by their sharp edges and high surface area. This damage can result in the leakage of cellular contents, ultimately leading to cell death (Akhavan and Ghaderi, 2010). This insight into the impact of functionalization provides valuable information for tailoring graphene-based nanomaterials with enhanced antibacterial properties for potential biomedical applications.
4 Conclusion
We performed molecular dynamics (MD) simulations to gain a better understanding of the interactions between graphene nanosheets and lipid membranes, as well as to evaluate their potential in biomedical applications. In order to study the interplay between functionalized graphene nanosheets and lipid bilayer membranes, we created three simulation systems.
These systems consisted of PG, G-NH2, and G-COOH separated by a POPC membrane. We found that PG achieved equilibrium outside the phospholipid membrane after 200 nanoseconds, while G-COOH was near the membrane and G-NH2 was farther away, based on full-atom molecular dynamics simulations. By the end of the experiment, all three systems had eventually reached a low-energy condition. PG, G-COOH, and G-NH2 had different interaction energies with the model membrane of about −389, −450, and −159 kJ/mol, in that order. The energy difference between PG and G-COOH was 11% greater, and the energy difference between PG and G-NH2 was almost 24% higher. This suggests that G-COOH interacts with the membrane more strongly. The contact between the graphene nanosheet and the head group of the model membrane is improved when carboxyl functional groups are present on the graphene surface. Graphene-based nanomaterials were adsorbed onto the POPC cell membrane rather than penetrating it during the 200 ns simulations. This work shows that the interplay between nanomaterials and the cell membrane is largely determined by variations in surface chemistry and the nature of functional groups. It is anticipated that these discoveries will enhance comprehension of the principles governing the interaction between graphene nanosheets and the cellular membrane, hence facilitating the development of novel nanocarriers for diverse uses in drug delivery and biomedicine.
Data availability statement
The raw data supporting the conclusion of this article will be made available by the authors upon formal request.
Author contributions
MG: Writing–original draft. AZ: Writing–review and editing, Validation, Software. HH: Writing–review and editing, Validation, Supervision. VS: Writing–review and editing, Supervision, Investigation, Funding acquisition, Conceptualization.
Funding
The author(s) declare that financial support was received for the research, authorship, and/or publication of this article. The MSc thesis, supported by grant no. 140063 and ethics code IR. SHMU.REC.1400.209 from Shahroud University of Medical Sciences, provided the basis for this study.
Acknowledgments
We extend our gratitude to Mr. Khani for his invaluable technical assistance.
Conflict of interest
The authors declare that the research was conducted in the absence of any commercial or financial relationships that could be construed as a potential conflict of interest.
Publisher’s note
All claims expressed in this article are solely those of the authors and do not necessarily represent those of their affiliated organizations, or those of the publisher, the editors and the reviewers. Any product that may be evaluated in this article, or claim that may be made by its manufacturer, is not guaranteed or endorsed by the publisher.
References
Abraham, M. J., Murtola, T., Schulz, R., Páll, S., Smith, J. C., Hess, B., et al. (2015). GROMACS: high performance molecular simulations through multi-level parallelism from laptops to supercomputers. SoftwareX 1, 19–25. doi:10.1016/j.softx.2015.06.001
Aguilar-Bolados, H., Vargas-Astudillo, D., Yazdani-Pedram, M., Acosta-Villavicencio, G., Fuentealba, P., Contreras-Cid, A., et al. (2017). Facile and scalable one-step method for amination of graphene using leuckart reaction. Chem. Mater. 29 (16), 6698–6705. doi:10.1021/acs.chemmater.7b01438
Akhavan, O., and Ghaderi, E. (2010). Toxicity of graphene and graphene oxide nanowalls against bacteria. ACS Nano 4 (10), 5731–5736. doi:10.1021/nn101390x
Chen, J., Zhou, G., Chen, L., Wang, Y., Wang, X., and Zeng, S. (2016). Interaction of graphene and its oxide with lipid membrane: a molecular dynamics simulation study. J. Phys. Chem. C 120 (11), 6225–6231. doi:10.1021/acs.jpcc.5b10635
Chen, Y., Chen, L., Bai, H., and Li, L. (2013). Graphene oxide–chitosan composite hydrogels as broad-spectrum adsorbents for water purification. J. Mater. Chem. A 1 (6), 1992–2001. doi:10.1039/c2ta00406b
Darden, T., York, D., and Pedersen, L. (1993). Particle mesh Ewald: an N⋅ log (N) method for Ewald sums in large systems. J. Chem. Phys. 98 (12), 10089–10092. doi:10.1063/1.464397
Geetha Bai, R., Muthoosamy, K., Manickam, S., and Hilal-Alnaqbi, A. (2019). Graphene-based 3D scaffolds in tissue engineering: fabrication, applications, and future scope in liver tissue engineering. Int. J. Nanomedicine 14, 5753–5783. doi:10.2147/ijn.s192779
Geim, A. K., and Novoselov, K. S. (2007). The rise of graphene. Nat. Mater. 6 (3), 183–191. doi:10.1038/nmat1849
Hess, B., Bekker, H., Berendsen, H. J. C., and Fraaije, J. G. E. M. (1997). LINCS: a linear constraint solver for molecular simulations. J. Comput. Chem. 18 (12), 1463–1472. doi:10.1002/(sici)1096-987x(199709)18:12<1463::aid-jcc4>3.3.co;2-l
Humphrey, W., Dalke, A., and Schulten, K. (1996). VMD: visual molecular dynamics. J. Mol. Graph. 14 (1), 33–38. doi:10.1016/0263-7855(96)00018-5
Ji, Y., Zhu, R., Shen, Y., Tan, Q., and Chen, J. (2021). Comparison of loading and unloading of different small drugs on graphene and its oxide. J. Mol. Liq. 341, 117454. doi:10.1016/j.molliq.2021.117454
Jorgensen, W. L., Chandrasekhar, J., Madura, J. D., Impey, R. W., and Klein, M. L. (1983). Comparison of simple potential functions for simulating liquid water. J. Chem. Phys. 79 (2), 926–935. doi:10.1063/1.445869
Kazempour, M., Namazi, H., Akbarzadeh, A., and Kabiri, R. (2019). Synthesis and characterization of PEG-functionalized graphene oxide as an effective pH-sensitive drug carrier. Artif. cells, nanomedicine, Biotechnol. 47 (1), 90–94. doi:10.1080/21691401.2018.1543196
Kiani, M. F., Zaboli, A., Shirshahi, V., and Hashemzadeh, H. (2023). The state of art in the prediction of mechanism and modeling of the processes of surface functionalized carbon nanotubes into the membrane cell. Appl. Surf. Sci. 623, 157039. doi:10.1016/j.apsusc.2023.157039
Kong, Z., Zhang, P., Chen, J., Zhou, H., Ma, X., Wang, H., et al. (2021). Effect of shape on the entering of graphene quantum dots into a membrane: a molecular dynamics simulation. ACS Omega 6 (16), 10936–10943. doi:10.1021/acsomega.1c00689
Kučerka, N., Nieh, M.-P., and Katsaras, J. (2011). Fluid phase lipid areas and bilayer thicknesses of commonly used phosphatidylcholines as a function of temperature. Biochimica Biophysica Acta (BBA)-Biomembranes 1808 (11), 2761–2771. doi:10.1016/j.bbamem.2011.07.022
Lawal, A. T. (2019). Graphene-based nano composites and their applications. A review. Biosens. Bioelectron. 141, 111384. doi:10.1016/j.bios.2019.111384
Lee, J., Cheng, X., Jo, S., MacKerell, A. D., Klauda, J. B., and Im, W. (2016). CHARMM-GUI input generator for NAMD, GROMACS, AMBER, OpenMM, and CHARMM/OpenMM simulations using the CHARMM36 additive force field. Biophysical J. 110 (3), 641a. doi:10.1016/j.bpj.2015.11.3431
Lerf, A., He, H., Forster, M., and Klinowski, J. (1998). Structure of graphite oxide revisited. J. Phys. Chem. B 102 (23), 4477–4482. doi:10.1021/jp9731821
Mirhosseini, M. M., Rahmati, M., Zargarian, S. S., and Khordad, R. (2017). Molecular dynamics simulation of functionalized graphene surface for high efficient loading of doxorubicin. J. Mol. Struct. 1141, 441–450. doi:10.1016/j.molstruc.2017.04.007
Novoselov, K. S., Fal′ko, V. I., Colombo, L., Gellert, P. R., Schwab, M. G., and Kim, K. (2012). A roadmap for graphene. Nature 490 (7419), 192–200. doi:10.1038/nature11458
Novoselov, K. S., Geim, A. K., Morozov, S. V., Jiang, D., Zhang, Y., Dubonos, S. V., et al. (2004). Electric field effect in atomically thin carbon films. science 306 (5696), 666–669. doi:10.1126/science.1102896
Pramanik, N., Ranganathan, S., Rao, S., Suneet, K., Jain, S., Rangarajan, A., et al. (2019). A composite of hyaluronic acid-modified graphene oxide and iron oxide nanoparticles for targeted drug delivery and magnetothermal therapy. ACS omega 4 (5), 9284–9293. doi:10.1021/acsomega.9b00870
Pulingam, T., Thong, K. L., Appaturi, J. N., Lai, C. W., and Leo, B. F. (2021). Mechanistic actions and contributing factors affecting the antibacterial property and cytotoxicity of graphene oxide. Chemosphere 281, 130739. doi:10.1016/j.chemosphere.2021.130739
Rabchinskii, M. K., Ryzhkov, S. A., Kirilenko, D. A., Ulin, N. V., Baidakova, M. V., Shnitov, V. V., et al. (2020). From graphene oxide towards aminated graphene: facile synthesis, its structure and electronic properties. Sci. Rep. 10 (1), 6902. doi:10.1038/s41598-020-63935-3
Reina, G., González-Domínguez, J. M., Criado, A., Vázquez, E., Bianco, A., and Prato, M. (2017). Promises, facts and challenges for graphene in biomedical applications. Chem. Soc. Rev. 46 (15), 4400–4416. doi:10.1039/c7cs00363c
Saedi, M., Shirshahi, V., Mirzaii, M., and Nikbakht, M. (2022). Preparation of graphene oxide nanoparticles and their derivatives: evaluation of their antimicrobial and anti-proliferative activity against 3T3 cell line. J. Dispersion Sci. Technol. 45, 381–389. doi:10.1080/01932691.2022.2151458
Sasidharan, A., Panchakarla, L. S., Chandran, P., Menon, D., Nair, S., Rao, C. N. R., et al. (2011). Differential nano-bio interactions and toxicity effects of pristine versus functionalized graphene. Nanoscale 3 (6), 2461–2464. doi:10.1039/c1nr10172b
Shirshahi, V., Saedi, M., Nikbakht, M., and Mirzaii, M. (2023). Unveiling the antimicrobial potential of oxidized graphene derivatives: promising materials for advanced wound dressings and antibacterial surfaces. J. Drug Deliv. Sci. Technol. 88, 104949. doi:10.1016/j.jddst.2023.104949
Willems, N., Urtizberea, A., Verre, A. F., Iliut, M., Lelimousin, M., Hirtz, M., et al. (2017). Biomimetic phospholipid membrane organization on graphene and graphene oxide surfaces: a molecular dynamics simulation study. ACS Nano 11 (2), 1613–1625. doi:10.1021/acsnano.6b07352
Witzke, S., Petersen, M., Carpenter, T. S., and Khalid, S. (2016). Molecular dynamics simulations reveal the conformational flexibility of lipid ii and its loose association with the defensin plectasin in the staphylococcus aureus membrane. Biochemistry 55 (23), 3303–3314. doi:10.1021/acs.biochem.5b01315
Wu, E. L. (2014). CHARMM-GUI membrane builder toward realistic biological membrane simulations. Wiley Online Library.
Zaboli, A., Raissi, H., Hashemzadeh, H., and Farzad, F. (2023). Graphene oxide hosting a pH-sensitive prodrug: an in silico investigation of graphene oxide-based nanovehicle toward cancer therapy. ACS Appl. Bio Mater. 6, 2826–2836. doi:10.1021/acsabm.3c00276
Zahid, M., Akram, S., Rashid, A., Rehan, Z. A., Javed, T., Shabbir, R., et al. (2021). Investigating the antibacterial activity of polymeric membranes fabricated with aminated graphene oxide. Membranes 11 (7), 510. doi:10.3390/membranes11070510
Keywords: graphene derivatives, molecular dynamics simulation, biological membranes, functional groups, nanovehicle
Citation: Gholami M, Zaboli A, Hashemzadeh H and Shirshahi V (2024) Interaction of functionalized graphene with cellular membranes: an in silico investigation of graphene-based nanovehicle toward biomedical applications. Front. Nanotechnol. 6:1347284. doi: 10.3389/fnano.2024.1347284
Received: 30 November 2023; Accepted: 01 April 2024;
Published: 19 April 2024.
Edited by:
Tomaz Urbic, University of Ljubljana, SloveniaReviewed by:
Arunkumar Rengaraj, Wake Forest University, United StatesAna Figueiras, University of Coimbra, Portugal
Copyright © 2024 Gholami, Zaboli, Hashemzadeh and Shirshahi. This is an open-access article distributed under the terms of the Creative Commons Attribution License (CC BY). The use, distribution or reproduction in other forums is permitted, provided the original author(s) and the copyright owner(s) are credited and that the original publication in this journal is cited, in accordance with accepted academic practice. No use, distribution or reproduction is permitted which does not comply with these terms.
*Correspondence: Hassan Hashemzadeh, aGFzaGVtemFkZV9oQGJ1bXMuYWMuaXI=; Vahid Shirshahi, c2hpcnNoYWhpQHNobXUuYWMuaXI=