- 1Centre for Microsystems Technology, Department of Electronics and Information Systems, Ghent University–IMEC, Gent, Belgium
- 2Centre for Electronics Frontiers, School of Engineering, University of Edinburgh, Edinburgh, United Kingdom
Nanoscale technologies have brought significant advancements to modern diagnostics, enabling unprecedented bio-chemical sensitivities that are key to disease monitoring. At the same time, miniaturized biosensors and their integration across large areas enabled tessellating these into high-density biosensing panels, a key capability for the development of high throughput monitoring: multiple patients as well as multiple analytes per patient. This review provides a critical overview of various nanoscale biosensing technologies and their ability to unlock high testing throughput without compromising detection resilience. We report on the challenges and opportunities each technology presents along this direction and present a detailed analysis on the prospects of both commercially available and emerging biosensing technologies.
Introduction
Among the plethora of micro/nanotechnology applications, those in the medical field have drawn considerable attention. The micro and nanoscale structures offer a sensing platform relatively comparable in size to the species of interest such as antigen and antibody molecules (approximate average size of 10 nm), viruses (size ranging from 20 nm to 250–400 nm), bacteria (approximate size of 1 μm), cells (diameter ranging from 1 μm to hundreds of μm) and other biological entities. The advancement of nanotechnologies has aided the miniaturization of electronic components from the micro to the nano-scale, where at least one dimension of the structure is equal or below 100 nm while nanomaterials, chemical substances or materials consisting of very small particles of different shapes and sizes no larger than hundred nanometers (Figures 1A–L), exhibit novel characteristics such as increased strength, chemical reactivity or conductivity compared to the same material without nanoscale features particularly benefiting the medical practice in both diagnostics and therapeutics (Alvarez et al., 2017). New opportunities in the development of highly-sensitive biosensors have been demonstrated as appropriate solutions for enabling precise sensing of bio/chemical events that occur at tissue, cell, and even at molecular level (Hannah et al., 2020).
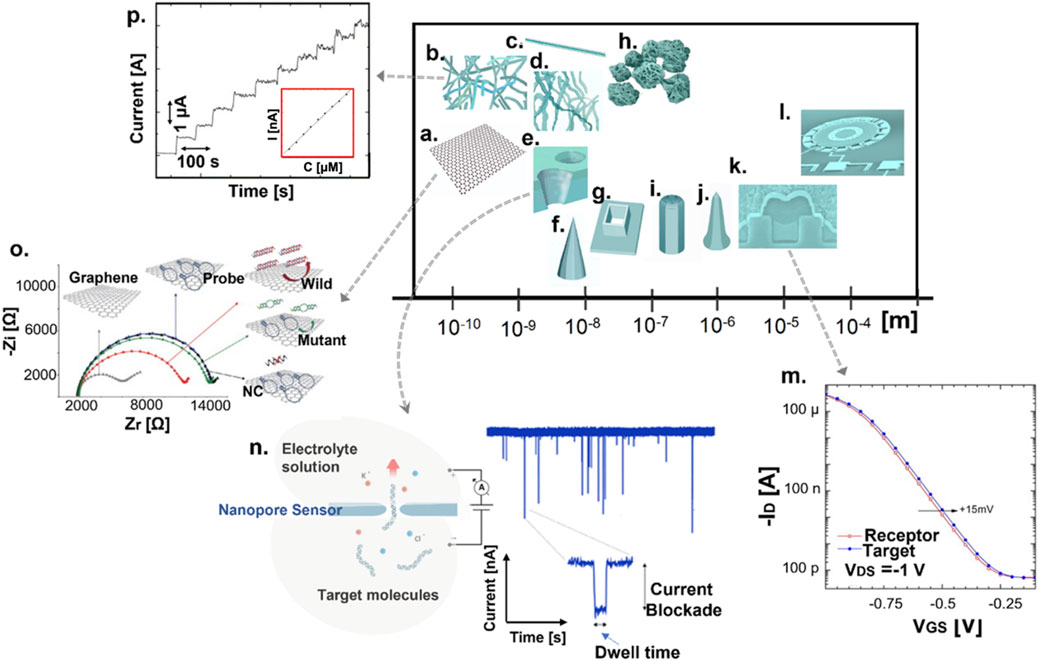
FIGURE 1. Scale-oriented illustration of the landscape of nanomaterials and micro/nanostructures involved in biosensing technologies (A–L) and characteristic biosensing data plots demonstrating indicative sensing principles and transduction mechanisms (M–P). (A) Graphene. (B) Nanotubes. (C) Nanowires. (D) Nanoribbons. (E) Nanopores. (F) Nanoneedles. (G) Nano-wells. (H) Nano/microscale spheres. (I) Nano/micropillars. (J) Nano-pencil structures. (K) MOSFET. (L) Microelectromechanical (MEM) systems. (M) Transfer characteristics of a receptor-target molecule interaction on gold extended-gate MOSFET, a p-type transistor with gate length 0.7 μm and width 3,860 μm. (N) A nanopore separating two aqueous compartments filled with electrolyte solution while small molecules are electrokinetically pulled through the pore by an applied potential, temporarily inducing a partial current blockade which is detected by an amplifier. The amplitude of the current blockade, proportional to the volume of the molecule in the nanopore, and the dwell time, are the signatures of a single-molecule translocation event. (O) Schematic illustration and Nyquist plots of an impedimetric graphene-based biosensing platform. (P) Amperometric curve for the oxidation of a coenzyme at CNT-composite electrode and the corresponding calibration plot (inset figure). Source: (M) Reproduced from Wang et al. (2010) with CCC permission from Elsevier (N) Reproduced under CC-BY-4.0 from Fragasso et al. (2020) (O) Reproduced under CC-BY-4.0 from Bonanni and Pumera (2011) (P) Reproduced from Chakraborty and Retna Raj (2007) with CCC permission from Elsevier.
Screen-Printed Electrodes (SPEs) (Mincu et al., 2020) usually comprise a 3-electrode configuration (working, counter, and reference) printed on substrates such as plastic or ceramic utilizing carbon, silver, gold and platinum and are often combined with a broad range of functionalization strategies introducing for example graphene, and quantum dots, implemented as enhancement to existing electrodes for boosting the sensing performance comparing to the bulk electrode as well as specific-to-the-target receptors (e.g., enzymes). These sensors are suitable for the rapid in situ detection, however, considering that commercially available SPEs cannot be mechanically or electrochemically polished between measurements to refresh the electrode surface for stability over consecutive scans for sensing reproducibility and prevention of the electrodes’ passivation, SPEs are more suitable for single-use experiments and applications where disposability is preferred (Kerr et al., 2021). Paper-based sensors have a natural ability to wick fluids, enabling the fabrication of pump-free microfluidic analytic devices. In paper-based sensors (Martinez et al., 2007) the sensing electrodes are patterned onto the different areas of paper substrates using a variety of different methods such as screen printing, inkjet printing, photolithography and wax-printing. Those patterned areas are thereafter modified to be selective to the target biomarker which reaches the sensing core through capillary and lateral flow properties. These are very low cost, simple and disposable sensing platforms that are versatile and easy to customize, however, limitations in terms of reproducibility and long-term stability need to still to be addressed. In MEMS (Algamili et al., 2021) (Figure 1L), as well as in nano-electromechanical-system (NEMS), the sensitive transduction mechanisms enable novel functionalities in devices with low power consumption. MEMS can be batch-fabricated onto semiconductors with active components [mechanical, (bio) sensors, actuators, and electronic elements] readily integrated resulting in miniaturized, microns-sized, features. Several variations of the metal–oxide–semiconductor field-effect transistor (MOSFET) (Figure 1K) have been developed for sensing (Bergveld, 1985): notable, among these are, the ion-sensitive field-effect transistors (ISFETs) (Wadhera et al., 2019) where the metal gate is replaced by an ion-sensitive membrane, electrolyte and reference electrode and the biologically sensitive field-effect transistor BioFET (Schöning and Poghossian, 2002). BioFETs are essentially ISFETs which are sensitive towards a selection of biomolecules due to the introduction of bio-recognition elements that are specific to the target molecules and are chemically or electrostatically bound to the transducer surface.
One important issue in most FET-based sensing applications is the requirement of a stable reference electrode e.g., an Ag/AgCl element, that provides a constant and stable potential during operation. Other applications replace the reference electrode with inert metals like gold or platinum or use gold as the sensing gate conjugated with bio-functionalization treatments. Measuring in dry conditions has achieved successful detection without using a reference electrode. In this framework, the extended-gate FET (EGFET) by extending off-chip the sensing pad, ultimately achieves separation of the wet and dry environments. Apart from these factors, ionic strength, pH level, temperature and samples of complex mixtures can create potential variabilities and instabilities in the detection processes (Kaisti, 2017). As the downscaling and miniaturization of the sensing elements progressed, nanoscale structures (Figures 1A–J) have revolutionized the biosensing status quo and are expected to render even more advancements in diagnostics (Justino et al., 2010) as transducing elements of high sensitivity and, consequently, low limit of detection (LoD).
The transducing mechanisms through which the chemical information is ultimately communicated and quantified, is a pivotal aspect in biosensors (Erickson et al., 2008) since their role is to convert the bio-recognition event into a measurable signal. Among the main transducing mechanisms optical biosensing methods are commonly used for the detection of biological markers. Surface Plasmon Resonance (SPR)-based biosensors use surface plasmon waves to detect changes when the target analyte interacts with a biorecognition element on the sensor and are one of the most favoured optical biosensing tools.
In colorimetric-based detection methods colour changes quantitated in the visible light spectrum indicate the different concentration levels through the differences in the optical density. The enzyme-linked immunosorbent assay (ELISA) colorimetric assay is of the most utilized tools in clinical laboratories and captures the bio-specific interactions occurring in antibody-antigen and enzyme-substrate pairs giving a quantification of the concentration of a target inside a sample through a visual signal. On the other hand, Fluorescent ELISA (FELISA) is a variation of the standard colorimetric ELISA that rather than a visible colour change, the enzymatic reaction creates a product that fluoresces when excited by light at a specific wavelength. Specifically, in fluorescence-based biosensing platforms an external light source is required to initiate the electronic transitions in an atom or molecule which then produces luminescence. However, in chemiluminescence-based platforms luminescence is produced as a side-product of a chemical reaction, when the atom or molecule relaxes from its excited state to its ground state. The electrochemical biosensors handle electrical signals/properties providing measurement and quantification of the concentration of an analyte inside a sample, covering a rather wide-range: from small (DNA and proteins) to large biological objects (cells and bacteria). Electrochemical biosensors can be discriminated based on the nature of the electrochemical changes detected during a biorecognition event (Figures 1M–P) and can be categorized as amperometric, potentiometric, impedance-based and conductometric. Amperometric sensors are based on the measurement of current as a function of time as a result of the oxidation and reduction of electroactive species in a biochemical reaction that mainly depends on the concentration of an analyte with a fixed potential. A potentiometric biosensor is essentially a device that combines a biorecognition element (i.e., an enzyme) with a transducer that senses the ions amount variation with the recorded analytical signal being logarithmically correlated with the analyte concentration (Nernst equation). In the Electrical Impedance Spectroscopy (EIS) the transduction mechanism is based on a conductivity detection that scans the detection volume with an electrical frequency sweep (usually in the range of 10 kHz and 10 MHz). Finally, conductometric-based devices consist of two electrodes, separated by a medium such as nanowire, and the transduction method is essentially based on the information linked to the ability of an electrolyte solution to conduct an electric current between electrodes. Meanwhile, magnetic-based bio-detection involves either a permanent magnet or external coil to magnetize and sense magnetic particles. The capturing probe is immobilized on a solid substrate (e.g., glass) and the sample is labelled with magnetic particles will mix with the capturing probe. The matched target will be captured, and unbound objects will then be rinsed off. A magnetic transducer, such as an LC-oscillator or Hall sensor will transduce the magnetism of the sample to electrical signals, which will be subsequently processed by the readout circuit. Finally, pressure-based sensors (Chang et al., 2020) are mainly based on macro-scale diaphragm configurations, the deformation of which indicates the applied pressure that is transduced into an electrical or other identifiable output signal. In a similar concept mechanical-based bio-detection exploits a cantilever and the mass introduced by the presence of the biomolecules attached to it, transducing the bending force on the cantilever to electrical signals i.e., a resistance, and ultimately resistance variations.
Moving towards highly dense and large assays
An essential part of evaluating the success of global health, from monitoring an individual’s health status till the transmission of an infectious disease in a large community, is the access to appropriate diagnostic tools. These should be portable, complete and autonomous, including the necessary readout circuitry for a direct and short result turnaround time (Samson et al., 2020) as well as offering a comfortable experience for patients, by relying on small specimens. In this light, nanostructure-based immunosensors offer miniaturization possibilities and potential for integration of the technology in a small device together with the incorporation of processing and signal conditioning circuitry. The application of nanotechnologies also bares benefits for applications in point-of-care (PoC) diagnosis (Vashist, 2017), on the patient’s bedside, and Lab-on-a-chip (LOC) solutions (Dekker et al., 2018). It allows integration of one, or multiple, lab functions on a single integrated chip for providing easy-to-use, health-self-monitoring systems (Wu et al., 2018) and self-diagnostics at home. Τhis may apply in the case of an infectious disease (e.g., COVID-19 (Bhalla et al., 2020; Samson et al., 2020)), where any infected members of a community can be quickly identified (Bhalla et al., 2020; Samson et al., 2020) through the use of self-diagnostics. Similarly, the collection of various signatures reflecting a disease state and progression is essential to maximize the amount of information that can be obtained for the health condition of an individual patient. Multiplexed systems allow detection of multiple biomarkers that favors a quicker, more accurate, and early detection of the disease. Thus, new sensor platforms should have inherently high degrees of multiplexing capabilities. In both cases of large-scale sensing platform for high sample throughput and multiplexing schemes the presence of multiple identical device arrays is a fundamental prerequisite and can be achieved by the incorporation of multiple transducers attached to the individual sensing area (Zupančič et al., 2021). In addition, wearable biosensors (Kim et al., 2019a) can provide long-term, continuous and real-time monitoring of the physiological status of an individual via dynamic, non-invasive measurements of biochemical markers in biofluids, such as sweat, tears, saliva and interstitial fluid.
Moving towards highly dense and large multiplex screening assays comes with a range of challenges. First one needs to consider the device and array-level challenges due to constraints related with device scalability, inter-sensor differences and increased costs in fabrication and operation. Then, system-level aspects for the development of optimized large-scale testing tools such as integration interoperability with complementary electronic and micro-fluidic components need particular attention. Paper-based sensors have demonstrated some multiplexing capabilities through some sophisticated manufacturing schemes (e.g., 3D paper-based platform simultaneously running assays in different layers), minimising the need for external pumps thanks to the direct flow ability, alike conventional microfluidics. These sensors however, exhibit a few limitations (Gutiérrez-Capitán et al., 2020) in terms of reproducibility and long-term stability as well as limited potential for scalability and integration in larger complete schemes involving readout electronics. Conventional SPEs sensors can contribute moderate multi panel detection systems via utilizing multiple working electrodes and a signal output channel (Zhao et al., 2018), however, this technology is still limited due to the need for relatively bulky instrumentation. Integrated, direct connection of the sensor with readout electronics that perform both signal processing and accurate analysis of the measured quantity is of paramount importance since it ultimately defines the response time of the sensor. On the other hand, (bio) MEM/NEM systems can perform the operation of sensing, controlling, and actuating at microscale, either individually or in bulk, also providing high sensing performance expressed through ultralow limits of sensing (e.g., ultralow mass sensitivity for resonant MEMS) and a high degree of multiplexing (bio) NEMS. Nevertheless, issues related to the robustness of the provided detection answer still remains as an obstacle for reaching the end users in the form of diagnostic tools (Leïchlé et al., 2020). Advances in micro and nanotechnology ultimately provide biosensors that are small, ultrasensitive, compatible with standardized batch complementary metal oxide semiconductor (CMOS) technology, mature production processes allowing accurate and low-cost fabrication of large-area arrays, along with a straightforward integration with readout circuitry reducing the time required for the diagnosis and consequently the waiting time for the test results, offering significant benefits in clinical decision-making.
This paper reviews the current landscape of most celebrated nano-biosensing technologies, their underpinning transducing mechanisms, and use cases in applications. We focus our review on biosensor technologies with excellent scaling prospects, including: nanopores, nanowires, nanotubes, memristors and CMOS-based transducers, along with those supporting integration in large testing deployments such as PCB-based schemes, ultimately commenting on the critical metrics for delivering optimized large-area multi-panel testing platforms. Finally, the review concludes by discussing the technological potential and possibilities emerging from the use of dense multi-panel sensing arrays combined with embedded AI, providing an innovation roadmap for the medical diagnostics of the future.
Nanopore-technologies
Nature performs exceptionally efficient cell signaling and signal transduction via the diffusion of ions through highly selective transmembrane ion-channels (Hille, 1978). Those channels ultimately undertake the role of effective sensors opening and closing in response to electrical, mechanical, or chemical stimuli. Inspired by the ion-channel sensing, research efforts have been focused in emulating the structural properties of ion-channels related to the extracellular-intracellular potential, the ionic flows, and the molecular transport across cellular membranes, by creating molecular-scale holes also known as nanopores (Anselmetti, 2012). According to the utilized material and fabrication method, nanopores fall into two main categories: the organic (Crnković et al., 2021) and the solid-state (Dekker, 2007) nanopores; a third hybrid category (Hall et al., 2010) can be thought that entails the insertion of a biological nanopore into a solid-state one. Organic nanopores (Figures 2A, B) can be found as natural protein ion-channels (Varongchayakul et al., 2018), with a defined size ranging from 1 nm to 4 nm of diameter, in the cell lipid membrane (e.g., α-hemolysin, mycobacterium smegmatis porin A and Aerolysin) or they are fabricated by biological materials (Howorka, 2017) and synthetic organic compounds (Langecker et al., 2012; Diederichs et al., 2019) through solution based biological techniques. Biological materials such as lipid membranes, proteins, and functionalized enzymes usually necessitate storage in aqueous conditions, often present substrate to substrate transfer challenges while the fixed size range of the biological nanopores may limit their applications. On the other hand, solid-state nanopores (Figures 2C, D) are artificially formed pores in solid-state membranes (Tang et al., 2016) (i.e., implementing SiNx and SiO2, Si and multi-layer Si, MoS2 and polymers) via electron beam milling (Goto et al., 2016), focused ion beam and electron beam lithography (Storm et al., 2003) followed by etching, overall allowing a dimensional control with sub-nanometer accuracy and a tailored to the application geometry (i.e., size, shape, and thickness) of the resulted nanostructures. Ion-channel mimicking sensing was first proposed by Sugawara et al., (1987) and was deployed by Cornell et al., (1997) through the synthesis of lipid multilayer membranes on glassy carbon electrodes. Varying the nature and type of integrated receptors, enabled the detection of a wide range of analytes of interest (Anselmetti, 2012). The nanopore ultimately provides a single connection path between two separated compartments in an electrolyte, while small molecules are electrokinetically pulled through the pore by an applied potential, through an external bias voltage added on the electrolyte across the nanopore membrane through reference (Ag/AgCl) electrodes that in turn establish an ionic current through the nanopore. During their passage, these small molecules temporarily induce a partial current blockade with an amplitude proportional to the volume of the molecules. This amplitude and the so-called as dwell time, are the signatures of a single-molecule translocation event (Figure 1N).
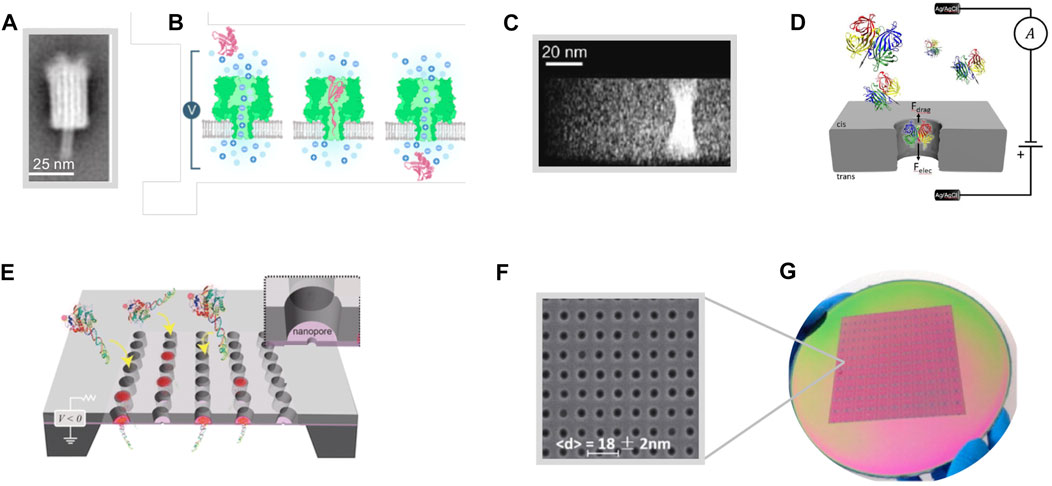
FIGURE 2. Nanopore-arrays technology (A) Averaged negative-stain TEM images obtained from purified DNA channel structures source (B) Illustration of the single-molecule organic nanopore sensing mechanism; Ions flow freely across the nanopore due to an applied electric field, physical blockage of a protein obstructs the ion flow, resulting in a drop in the pore’s current that is restored once the protein leaves the pore. (C) TEM characterization of a nanopore fabricated by electron beam drilling. (D) Illustration of the single-molecule solid-state nanopore sensing mechanism; A voltage bias is applied across the nanopore, ions move through the nanopore toward the oppositely charged electrode and the molecules of interest are subjected to net force contributions (hydrodynamic drag force, Fdrag, and an opposing electrophoretic force, Felec) and translocate through the pore. (E) Illustration of a nanopore-array at the bases of waveguides positioned on a 35 nm silicon nitride membrane. A voltage bias actively draws complexes of biotinylated DNA and fluorescently labelled streptavidin to the pore placing the fluorophore in the waveguide excitation volume. (F) Illustration of a 200-mm wafer patterned in a matrix of 11 × 11 three-metal 18-nm-diameter nanopores fabricated with standard semiconductor process. Source: (A) Reproduced from Langecker et al. (2012) with CCC permission from The American Association for the Advancement of Science (B) Reproduced from Varongchayakul et al. (2018) with CCC permission from ROYAL SOCIETY OF CHEMISTRY (C) Reproduced under CC-BY-4.0 from Kim et al. (2006) (D) Reproduced under CC-BY-4.0 from Carlsen and Cossa (2020) (E) Reproduced under CC-BY-4.0 from Larkin et al. (2014) (F,G) Reproduced from Feng et al. (2015) with CCC permission from Elsevier.
Nanopore sensors (Xue et al., 2020) and modified biological nanopores have been used in sequencing applications (Manrao et al., 2012) primarily aiming at DNA sequencing (Feng et al., 2015; Deamer et al., 2016) (Figure 2E), capturing dynamic processes such as DNA-protein interaction (Bell and Keyser, 2016) and nucleic acid analysis (Venkatesan and Bashir, 2011) as well as detection of peptides and proteins (Yusko et al., 2017), enabling ultra-low (attomole range) limit-of-detection (Wu et al., 2020) and single-molecule detection (Muthukumar et al., 2015) pushing the sensitivity to its limit. While the solution-based fabrication techniques often jeopardize the prospects of biological nanopores for large-scale integration, solid-state nanopores allow fast (Kim et al., 2006), large-scale (Deng et al., 2016) production (Figures 2F, G), excellent geometry control (e.g., via electron beam lithography and etching), a wide library of materials, compatibility with semiconductor processes and integration with readout electronics for high-throughput parallelized architecture (Magierowski et al., 2016). Although solid-state nanopores hold promises for many emerging applications with the capability to sense a variety of analytes, there are still limitations impeding their commercialization for real-life applications (Tang et al., 2016), such as poor device-to-device repeatability and stability (Chou et al., 2020). The same nanopore may give distinct background noise levels and translocation behaviors for the same analytes at different times of measurements, even under the same measurement conditions. This can be attributed to the non-repeatable physicochemical microenvironment in and around the pore, including surface charge density, wettability, chemical groups and adsorption of contaminants (Balme et al., 2016; Zhou et al., 2018) which are difficult to remain under strict control in every measurement. Even though a very carefully cleaning process has been done prior to each measurement, such as long-term oxygen plasma treatment and piranha soaking, high repeatability of the translocation outcome cannot be guaranteed (Beamish et al., 2013). Another crucial problem is instability during measurement. It is related to the sticky surface of solid-state materials (Balme et al., 2016) which renders adsorption of hydrated ions, analyte molecules, and other contaminates, often resulting to the clogging of the pore. Consequently, the translocation process may be easily influenced to deviate from the assumption of a stationary stochastic process. Furthermore, relatively high noise level of solid-state nanopores is a problem worthy of attention (Beamish et al., 2013). To achieve a higher time and spatial resolution, the ability to distinguish weak signals from background noise is essential.
Nanowire and nanotube technologies
Nanowire (Figures 3A, B) and nanotube (Figure 3C) technologies have attracted immense interest in building nanoscale electronic devices or as interconnections in extremely small circuits. Thanks to their high surface-to-volume ratio (e.g., typical nanowire length-to-width ratio is 1,000 or more) these nanostructures exhibit many exciting (electrical, magnetic, optical, and mechanical) properties that have been leveraged in applications ranging from energy to solar cells, logic gates, computation, and even as emerging transistor technologies for sustaining the scaling beyond convectional MOSFETs. Notably, the tunable electron transport properties of nano-wires/-tubes lead to a highly sensitive electrical response (Elfström et al., 2007) while their electrical properties are strongly influenced by even minor perturbations. These attributes, in addition to their dimension-related properties and their size that is comparable to that of many biological species of interest, is key in attaining ultra-high sensitivity biosensors (Cui et al., 2001).
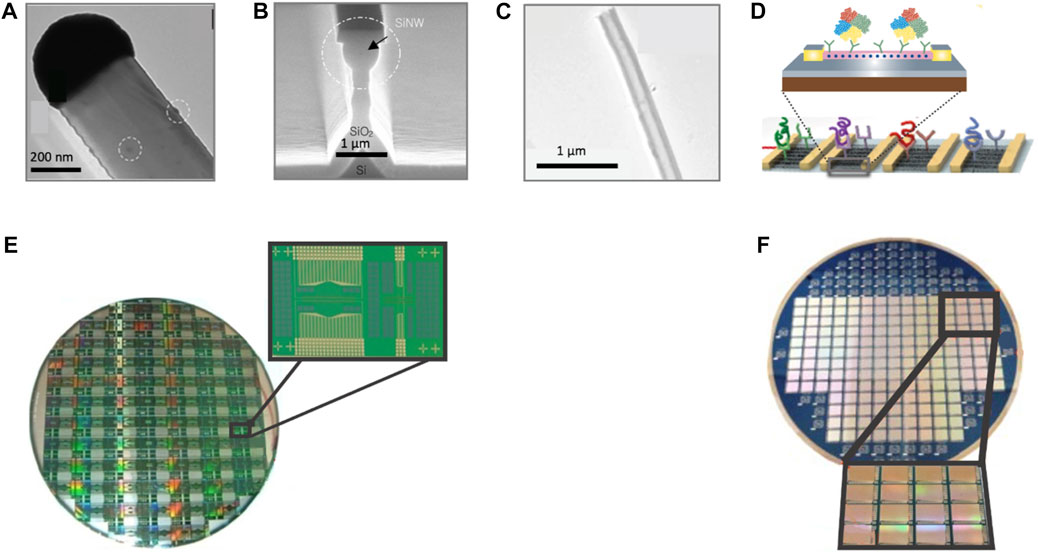
FIGURE 3. Nanowire and Nanotube-based schemes. (A) HRTEM image of an Al/Au-catalyzed silicon nanowire. (B) SEM image of Si nanowire obtained by a top-down-based fabrication process and thermal oxidation thinning. (C) Illustration an individual nanotube, grown from natural abundance CO2. (D) Schematic illustrations of a densely aligned CNT biosensor array and of a nanowire biosensor device demonstrating the biosensing principle. (E) Image of an 8-inch wafer overall comprising 72 biosensing chips; each individual biosensing chip comprises 40 similar nanowire clusters, each consisting of five individual nanowires of 50 nm width and 90 μm in length. (F) Illustration of an 100-mm- wide wafer on which the integrated circuits are fabricated and the 1.7 cm × 2.2 cm chip-arrays are distributed. Source: (A) Reproduced under CC-BY-4.0 from Behroudj et al. (2019) (B) Reproduced from Lee et al., (2007) with CCC permission from IOP Publishing (C) Reproduced under CC-BY-4.0 from Ren and Licht (2016) (D) Reproduced from Patolsky et al. (2006) with CCC permission from FUTURE MEDICINE LTD and under CC-BY-4.0 from Kim et al. (2020) (E) Reproduced from Zhang and Ning (2012) with CCC permission from Elsevier (F) Reproduced from Shulaker et al. (2017) with CCC permission from Springer Nature.
Single-layer (SWCNTs) (Figure 3C) or multilayer (MWCNTs) carbon nanotubes (CNTs), cylinders of one or more layers of graphene, respectively, are very often reported in literature for boosting the biosensors’ performance, offering dimensional and chemical compatibility with biomolecules, such as DNA and proteins or used as the channel of a field-effect transistor (CNFETs) with traditional lithographically defined source, drain and gate regions (Figure 3D). In these frameworks CNTs based biosensors are implemented for optical and electrochemical biosensing (Heller et al., 2008) e.g., in amperometric (Chakraborty and Retna Raj, 2007), potentiometric (Maehashi and Matsumoto, 2009), capacitance change (Snow et al., 2005), impedance-based (Lee et al., 2022) and conductance (Heller et al., 2008) measurements.
Nanowire technologies (Figure 3D, E) have been reported as promising building blocks for high sensitivity biosensors, enabling direct electrical detection of biomolecules (Patolsky et al., 2006) (Figure 3D) and various biological species, such as proteins (Cui et al., 2001; Zheng et al., 2005), viruses (Patolsky et al., 2004) and DNA (Hahm and Lieber, 2004). Such structures have mainly been implemented as the core (chanel) of FETs (Patolsky and Lieber, 2005). The interaction of the target molecule with the detector element on the surface can be directly translated into a readable electrical signal (e.g., variations in conductance), while sensing specificity is defined via the choice of the detector element (e.g., antibody species, DNA, aptamers, peptides, engineered proteins with elevated affinity toward target molecules). Bio-functionalization processes typically includes a surface activation step, followed by surface treatments providing chemical compatibility between the probe reagents and the surface, enabling the optimum receptor molecule coupling.
The fabrication of such nanostructures falls into two main approaches (Hobbs et al., 2012) the bottom-up and top-down. In the top-down methodology, nanostructures are etched out of a substrate whereas in a bottom-up approach, the structures are grown and subsequently assembled. Bottom-up methods provide a straightforward growth process of nanostructures with a wide range of characteristics and properties (e.g., nanowires or nanowire-heterostructures of different lengths and cross-sectional profiles) supporting a molecular-level control and the acquisition of very small geometries (Figure 3A). The synthesis of nanostructures can be performed via spontaneous growth driven by the reduction of Gibbs free energy or chemical potential that involves evaporation (dissolution) condensation or through vapor (or solid) growth and stress induced re-crystallization. Growth methods include Vapor Liquid Solid (VLS), Vapor Solid-Solid (VSS), Oxygen Assisted Growth (OAG) and laser assisted catalytic growth (LAC). Template based synthesis, that includes electrochemical deposition, electrophoretic deposition, colloid dispersion, Chemical Vapor Deposition (CVD), Atomic layer deposition (ALD), Molecular Beam Epitaxy (MBE), add more pathways towards nanostructure synthesis. On the other side, top-down nanopatterning techniques are typically carried out through lithographic processes defining the nanostructures that are then etched (Figure 3B). Many different approaches, including Electron-Beam Lithography (EBL), Focused Ion Beam (FIB) lithography, Nano Imprint Lithography (NIL) and Nano Function advanced optical lithography have been developed. Other conventional top-down nano patterning techniques, such as extreme-UV lithography (deep UV and immersion deep UV photolithography). Overall, this prototyping versatility allows the fabrication of a wide range of nanowires consisting either of inorganic (Meyyappan and Sunkara, 2018) or organic materials (Min et al., 2015), including different types of structures such as metallic (i.e., Ni, Pt, Au), semiconducting (i.e., Si, InP, Si, GaN), insulating (i.e SiO2,TiO2), multi-component (Wang et al., 2021) as well as molecular multi-segments (i.e., DNA, MoSI). Meanwhile nanotube structures can be grown on different type of substrates with CVD and the electrophoretic deposition highlighted as the most popular growth methodologies.
The development of highly dense and large-scale arrays for biosensing applications, requisites a controlled alignment and pattering, formation of a well-ordered assembly of individual nanowire and nanotube structures and uniform placement of multiple devices at precise locations over a large area are fundamental prerequisites. Top-down fabrication methods use standard semiconductor processing techniques allowing more precise designs and full control of the geometry and alignment of the nanostructures, offering the possibility to directly acquire pattern arrays consisting of homogeneous structures with no need of post-transfer, thus enabling the large-scale integration of a large number of nanostructures on a single chip. Electrohydrodynamic printing for the definition of nanowires, allows printing large-area organic semiconducting nanowire arrays directly on device substrates in a precisely, individually controlled manner, enabling sophisticated large-area nanowire lithography for nano-electronics (Min et al., 2013). On the other hand, bottom-up methods often present some restrictions related to the production of entangled meshes, lack of precise control, periodic ordering, and accurate placement. Most importantly, the fabricated nanostructures need post-transferring and alignment to a new substrate, or deposition from solution onto a device substrate. Efforts towards controlling the alignment and achieving a directed and precise assembly of single nanostructures include micro-machining processes (Lee et al., 2007), dispersion and sorting for the acquisition of a dimension-limited self-alignment of nanostructures with tunable density, as recently showcased for CNTs with density of 100–200 nanostructures/μm (Liu et al., 2020). Vijayaraghavan et al. (2007) demonstrated a nanotube dielectrophoresis method for achieving an integration density of several million devices/cm2. In this paradigm, the dielectrophoretic force fields change incisively as the nanostructures assemble into the contact areas, leading to a reproducible directed assembly which is self-limiting in forming single-tube devices. Rao et al. (2003) utilized organic molecular marks on a substrate to guide the self-assembly of individual single-walled carbon nanotubes while, nanostructures assembly into parallel arrays with control of the average separation has been demonstrated by Huang et al. (2001) combining fluidic alignment with surface-patterning techniques enabled periodicity control. Bishop et al. (2020) presented a deposition technique in which the substrate is submerged within a nanotube solution allowing CNFETs to be fabricated within industrial facilities. Finally, in their work Park et al. (2012) applied ion-exchange chemistry, leveraging the strong electrostatic interaction between a functional surface monolayer and a surfactant (i.e., wrapped carbon nanotubes in aqueous solution) to developed selectively placed arrays of individually positioned carbon nanotubes resulting in an outstanding high density (1 × 109/cm2) of carbon-nanotube transistors. A computing system prototype which integrates multiple new nanotechnologies i.e., RRAM arrays, silicon and CNFET computation units and memory access circuitry and more than one million CNFET-based gas sensors for inputs has been also reported (Shulaker et al., 2017), with all components fabricated on overlapping vertical layers (Figure 3F) to realize a 3D integrated circuit architecture.
CMOS-based technologies: ISFETs
CMOS technology leverages the maturity of microelectronics in manufacturing for realizing low-cost and low-power electronic elements along with the possibility of high-dense, large-scale integration of multiple transistors on tiny chips (<1 cm2). The ability of CMOS technologies to monolithically integrate transducers and signal processing units on the same chip, allows to combine the two key functions of a biosensing cell: transducing, by seamlessly handling electrical signals i.e., current, impedance and capacitance, thanks to the incorporated readout electronics, and ultra-fast signal processing (Mitra and Cumming, 2018). Combining considerations of process compatibility between CMOS functionality and biosensor needs, metal electrodes with affinity to biotargets are connected to circuitry through pre-designed surface contact pads. Recent CMOS biosensors unified different transducing mechanisms (Lei et al., 2016) (impedance, fluorescence, and nuclear spin) and readout electronics, demonstrated competitive sensitivity elements comparing to traditional electrochemical sensors and instrumentation for in vitro (Lei et al., 2016) diagnosis healthcare systems e.g., detection of DNA (down to 10 a.m.), protein (down to 10 fM), bacteria/cells (single cell) as well as for wearable systems, by efficiently moving transistors to flexible and stretchable designs (Dai et al., 2021).
Among the advancement of sensing schemes based on CMOS technology, field-effect transistor (FET) biosensors take full advantage of the native passivation and merits CMOS process i.e., miniaturisation, repeatability, minimal process variation, high yield, and low cost of large volumes. Within those FET biosensors, ion-sensitive FET (Bergveld, 1970; Bergveld, 2003) (ISFET) (Figure 4A) and BioFET (Schöning and Poghossian, 2002) were suggested as chemical sensors and in particular for measuring ion concentrations in a solution. Their operation principles are similar to MOSFET (Bergveld, 1985), where the current between source and drain terminals is proportional to the gate voltage that, for an ISFET, corresponds the charges on the surface of the FET (Figure 4B). The introduction of nanoparticles, on the gate and the use of aptamers as bio-recognition elements has been shown to improve sensitivity (Nakatsuka et al., 2018). The most highlighted implementations of ISFETs is their role in biosensing technologies (Moser et al., 2016) (Figure 4B) diagnostics (Sakata, 2019) and in DNA sequencing (Rothberg et al., 2011; Toumazou et al., 2013) (Figure 4C). Large-scale sensing architectures (Figures 4D, E) with ISFET biosensors have been developed for a plethora of applications (Moser et al., 2016) for enzyme sensing, measuring antigen-antibody bonding reactions, sensing of glucose as indication of diabetes, creatinine and urea as a determinant for renal function, potassium and sodium for neuronal monitoring and the detection of infectious diseases such as the SARS-Covid (Gibani et al., 2020).
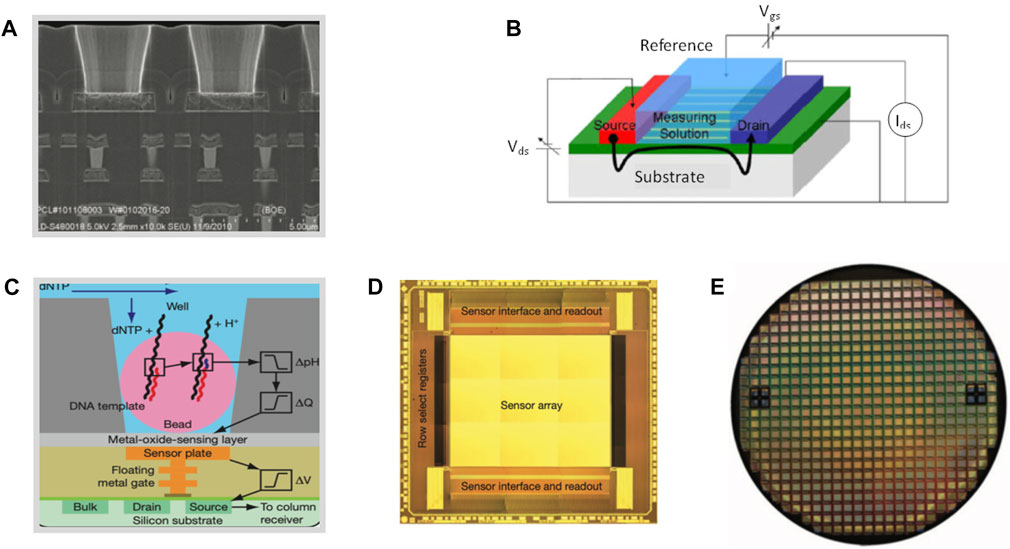
FIGURE 4. Behind CMOS-based Technology. (A) Electron micrograph showing the aligned wells over the ISFET metal sensor plate and the underlying electronic layers. (B) Schematic illustration demonstrating the ISFET working principle. (C) Schematic diagram of the underlying technology for semiconductor sequencing with DNA template. (D) Unpackaged die indicating functional regions and (E) CMOS sensor built on an 8-inch wafer comprising approximately 200 individual functional ion sensor dies (d). Source: Reproduced under CC-BY-4.0 from Rothberg et al. (2011).
Lab-on-PCB technologies
Printed Circuit Board (PCB) technologies hold great promise in providing scalable biosensing devices (Moschou and Tserepi, 2017; Zhao et al., 2020), as they integrate control electronics and sample handling modules (Sánchez et al., 2016) (Figure 5A) via low-cost techniques. Thanks to the standardized fabrication processes and manufacturing technology i.e., typically comprising photolithography and wet etching to form copper tracks on planar insulating layers followed by drilling of vias (holes) enabling the connection between the copper layers (Figure 5B), PCB systems show high cost-effective mass-manufacturing potential, while can be customized according to specific remands, thus, opening the way to the market and to reaching the end-users. Lab-on-PCB, a scheme integrating microchannels, sensing and electronic modules, and other components on a PCB, was first introduced (Merkel et al., 1999) as a platform comprising fluidic systems (Perdigones, 2021) arranged together with electronic circuitry for the monitoring of various metabolites by an integrated microdevice biosensor array and, for handling fluids (i.e., transport, mix, flow control, heating) while monitoring their properties i.e., temperature, viscosity, density, and pH). Such seamless, full integration of bio/chemical sensors (e.g., Figure 5C) led to portable and automate diagnostic devices for biomedical applications (Zhao et al., 2020) exhibiting rapid response time and requiring miniaturized sample volumes along with minimizing the effective area of the whole diagnostic system. According to the detection methods and the analysis principles, these biomedical applications can be discriminated into those dedicated to cell counting and analysis (Giménez-Gómez et al., 2020) i.e., red/white and tumor cells, and those targeting at biochemical analysis, namely, PCB-based electrochemical sensors (Shamkhalichenar et al., 2020) for the analysis of blood glucose, electrolytes, and other nutrients in the human body, for immunoassays leveraging the antigen–antibody specific reaction targeting sensing of protein biomarker (Sánchez et al., 2016), detection of nucleic acids (Kaprou et al., 2019) and viral diagnostics (Zhu et al., 2020). Cell analysis applications involve cell detection, counting and sorting according to their size and viability (Zhao et al., 2020). These applications are mainly based on the monitoring of the impedance change and comprise integrated resistance and capacitive sensors, microfluidic cytometers, single-cell imaging technology, dielectrophoresis (DEP)-based cell manipulation and Coulter’s counting schemes (Guo et al., 2014), that rely on the change in the electrical resistance of a conducting solution upon the introduction of an insulating biological particle through a micro/nano aperture that leads to a significant drop in the electrical current across the aperture (Tsai et al., 2017).
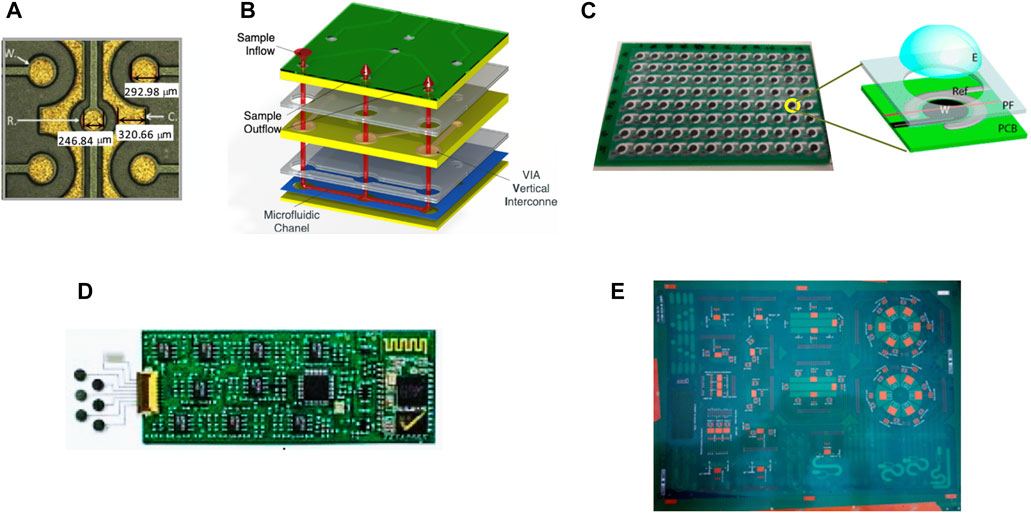
FIGURE 5. PCB technologies. (A) Magnified image electrodes developed using PCB technology showing the working (W), counter (C) and reference (R) electrodes with a diameter of 300, 250, and 250 μm, respectively. Overall, a single PCB chip (side length of 24.6 mm) comprises a 64-electrode array. (B) Manufacturing technology behind the Lab-on-PCB schemes. (C) Image of a 96-well PCB platform overlaid by the screen-printed carbon working electrode (W), the Ag/AgCl counter/reference electrode (Ref), by an adhesive plastic foil (PF) and finally by the electrolyte drop (E). (D) Demonstration of a wearable sensing platform for multiplexed perspiration analysis comprising a flexible sensing array integrated with the circuit components on PCB. (E) Lab-on-PCB up-scaling paradigm; a multi-panel platform including designs of multiple, on-PCB-devices and modules. Source: (A) Reproduced from Sánchez et al. (2016) with CCC permission from Elsevier (B) Reproduced from Vasilakis et al. (2016) with CCC permission from IEEE (C) Reproduced under CC-BY-4.0 from Abdellaoui et al. (2013) (D) Reproduced from Gao et al. (2016) with CCC permission from Springer Nature.
Electrochemical sensors comprise microchannel networks fabricated in SU-8, TMMF, 1002F, PDMS and polyimide and formed through standard lithography, in combination with layer-stacked sensing and reference electrodes, microelectrode arrays and flow driving methods, in addition to electronic instrumentation for collecting data. Post-processing the PCB electrodes by employing gold nanoparticles and graphene is another widely used strategy for increasing the sensing sensitivity. New advancements on the printed circuit industry have emerged with the fabrication of flexible printed circuits (FPC) and combinations of flexible and rigid (flex-rigid) printed circuits (Gao et al., 2016) (Figure 5D). In this framework, flexible PCB technologies have promoted the development of sensing platforms in deformable and stretchable formats (Gao et al., 2016) that facilitate their use as wearable biosensing. The standardized and mature PCB manufacturing process enables the realization of PCB-based detection devices on a large scale and provide more processes and prototypes adapted to the PCB that may include multiple designs and modules (Figure 5E). Multiplexed screening has been reported for genetic biosensors (Prindle et al., 2012) involving a genetic clock approach, with the synchronization of thousands of oscillating colony bio-pixels, and bio/chemical detectors (Hierlemann and Baltes, 2003) where hybrid approaches combining PCB with other technologies pave the way for advanced biosensing by combining the benefits that each technology brings. Integrating a CMOS-based ISFET sensor via wire bonding into a PCB microfluidic platform and PCB-based extended gate ISFETs ultimately combines the high sensitivity of the ISFET sensors for discriminating e.g., small pH changes, with the automated and rapid response output provided by the PCB circuitries (Tseng et al., 2015).
Memristor technologies
The memristor, was first theoretically introduced by L. Chua (Chua, 1971) as a non-linear electronic element whose current to voltage characteristics appear as frequency-dependent hysteresis loops. Thereupon, memristive technologies comprising a variety of different materials and architectures have been implemented in a plethora applications (Zidan et al., 2018), mainly focusing on memory, logic and computing systems and artificial neural networks (ANNs). Memristors can simultaneously carry out computational tasks and store information (Ielmini and Wong, 2018) at very low energy requirements (Jeong et al., 2016) and extremely low feature sizes (Pi et al., 2019) (Figure 6A) and react to the occurrence of an event, such as a voltage pulse stimulation of an amplitude that exceeds certain thresholds, as thresholded, weighted integrators (Gupta et al., 2016) and with a change in the state variable. The state variable of a memristor can also change as a result of a chemical input in the role of the excitation parameter. In these chemical-memristors, the chemical and biological species composed by charged residues, modify the net charge and effective local potential ultimately acting equivalently to an electrical stimulus or to a virtual all-around bio-gate effect (Carrara, 2020). Τhe integration of a multitude of such events gives rise to electrochemical bio-sensory systems (Figure 6B), for antigen-specific transduction, with the sensor’s specificity determined via bio-functionalization, for mimicking the natural neurotransmitter’s behavioral cycle (Keene et al., 2020) (Figure 6C) and for compression and sorting of electrical neuronal activity (Gupta et al., 2016) (Figure 6D). Meanwhile, the high promises in the overall speed/energy and throughput efficiency of larger arrays increasingly drove the attention towards levering memristors’ scalability properties, reaching crossbar arrays comprising 2 × 2 nm2 devices (Pi et al., 2019) in dense, large-scale schemes. Such systems coupled with bio-functionalization treatments providing sensing specificity to arrays or series of individual devices, will be able to support diagnostic tools for large specimen throughput and simultaneous monitoring of multiple analytes per patient. So far, only relatively small arrays have been demonstrated experimentally, mainly focusing on neural networks.
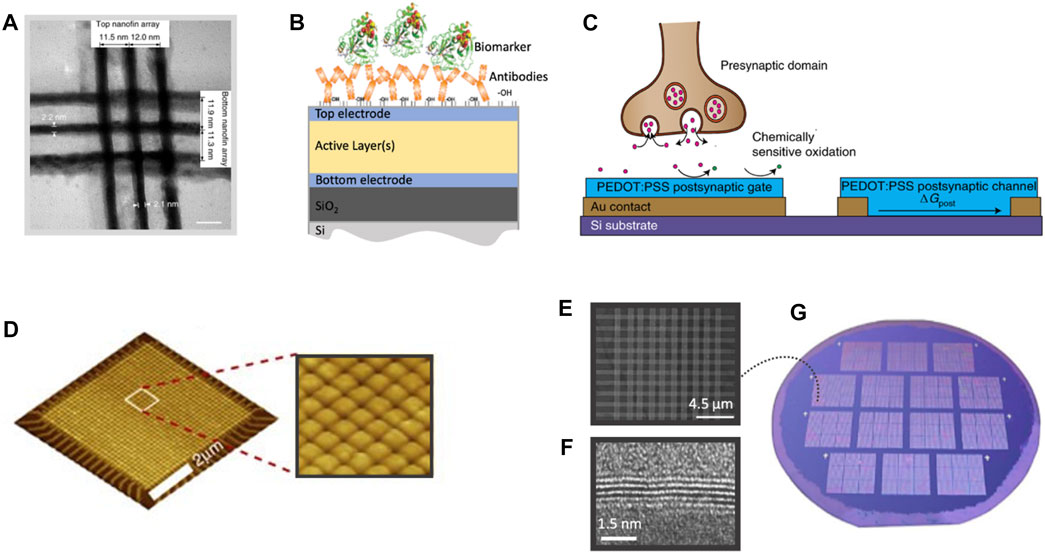
FIGURE 6. Memristor-arrays technologies. (A) TEM image depicting a 3 × 3 memristor crossbar array with 2 × 2 nm2 device area and with sub-12-nm pitch. (B) Schematic illustration of the surface bio-functionalization of a memristor device. (C) Illustration of a biosensing application showcasing a neurotransmitter-mediated neuromorphic device. The scheme function is based on the oxidation of dopamine at the postsynaptic gate electrode controlling the change in conductance of the post synaptic channel. (D) AFM image of a 32 × 32 solid-state TiOx-memristors crossbar-array. (E) SEM image of a 750-nm × 750-nm Ag/h-BN/Ag memristors crossbar-array and (F) cross-sectional TEM image showing the detailed layered structure. (G) The memristor arrays are distributed along a 2-inch wafer. Source: (A) Reproduced from Pi et al. (2019) with CCC permission from Springer Nature (B) Reproduced under CC-BY-4.0 from Tzouvadaki et al. (2020) (C) Reproduced from Keene et al. (2020) with CCC permission from Springer Nature (D) Reproduced under CC-BY-4.0 from Gupta et al. (2016) (E–G) Reproduced from Chen et al. (2020) with CCC permission from Springer Nature.
Challenges such as non-idealities in device properties, generated offsets and variable responses across the sensing cells are particularly highlighted in large array integration that get more pronounced when increasing the number of the panels involved. In the case of a biosensing platform, this means resourcing to external off-line software/hardware calibration approaches. Limitations in fabrication, automated device alignment and integration with peripheral circuitries also consist important obstacles towards this direction. Relevant mitigation strategies include improved pattering and fabrication methods (Xia and Yang, 2019), optimizing the ratio between mass production and high resolution, thickness uniformity, accurate control and tuning of the film composition, smart alignment strategies, or even minimization of the overlay alignment requirements, as for example by simultaneously pattering the complete memristor stuck (bottom electrode/switching layer/top electrode) via a one step nanoimprint lithography process (Xia et al., 2010). Advanced interconnection technologies also adopting organisation in multiple smaller arrays into different layers, either in lateral organization (2D) or vertically (3D) (Xia and Yang, 2019) have also been applied. The reconfigurability (Lee and Lu, 2018) attributes of memristive systems enable an in-situ, at-device-level calibration of entire biosensing arrays rendering a homogeneous sensing baseline across the individual biosensing cells. Besides, the fabrication of memristor arrays showcasing standalone device-to-device variability as low as 5.74% has been reported (Chen et al., 2020), while exhibiting arrays of high device yield reaching 98% (Figures 6D–G) and ultralow switching energy in the zeptojoule regime. Finally, the integration compatibility of memristors with CMOS (Xia, 2016) can be leveraged for integration of the memristive technology in more complex schemes.
Outline and future prospects
Lab-on-PCB systems can provide complete biosensing schemes that include well-integrated fluidics and electronic circuitry, whilst CMOS technologies leverage the maturity of microelectronics in manufacturing to monolithically integrate transducers and signal processing units on the same chip at low power and cost signal processing (Mitra and Cumming, 2018). Thanks to their standardized low-cost, mass-production fabrication processes, both technologies offer the capability of delivering high-density features, suitable for large-scale integration, rapid and quantitative multi-analyte sensing platforms, while they can be customized according to the specific needs of the end-user. CMOS technologies, and specifically those integrating ISFET and BioFETs, offer sensing performance and sensitivity; similarly, the sensitivity of Lab-on-PCB systems strongly depends and can be enhanced by the involvement of nanoscale components. Nanoscale sensors such as nanopores, nanowires and nanotubes show excellent potential for the detection of various analytes with ultra-low limit-of-detection (reaching even single-molecule sensing) while the biosensing specificity can be defined in an ad hoc manner via bio-functionalization with receptor molecules. These attributes combined with their scaling attributes, the compatibility with mature, batch production technologies and semiconductor processes and sound integration with readout electronics (Magierowski et al., 2016) and computing systems (Shulaker et al., 2017) render these nanoscale structures candidates for advance large-scale biosensing tools. Nevertheless, issues regarding robustness of the provided detection output, poor repeatability and stability (Chou et al., 2020), high noise levels (as well as different background noise levels), instability and translocation behaviors during sensing are prohibiting the way towards the end users and be readily used as diagnostic tools. Design and fabrication of low noise nanostructures is a prerequisite for high quality sensing. Memristors may benefit the biosensing field with their reconfigurability (Lee and Lu, 2018) addressing the longstanding bottleneck of generated offsets and variable responses across the sensing cells. Moreover, memristive technology simultaneously carries out computing and memory (Ielmini and Wong, 2018) at very low energy requirements (Jeong et al., 2016), enabling low-power consumption for the biosensing systems, that is one major issue especially present in the wearable sensing systems.
The ability of distinguishing weak signals from the background noise is essential for achieving a higher time and spatial resolution; embedding artificial intelligence (AI) algorithms can be a great benefit. AI and nanotechnology are instrumental merits in realizing precision diagnostics (Adir et al., 2020) addressing complex problems that require parallel treatment of many interacting parameters and multiple description levels and interactions (Cui et al., 2020). AI algorithms can deal with pattern association, recognition, classification, optimization, and prediction (Jin et al., 2020) tasks, addressing the challenges of big data; enabling fast real-time complex analysis and computations (Haick and Tang, 2021) and generalizing complex or unknown functions or data. For example bio-inspired AI paradigms based on evolutionary or genetic algorithms are implemented to provide solutions to optimization and search problems dealing with genomic diagnostics (Dias and Torkamani, 2019) and DNA sequencers (Huo et al., 2021). A label-free method for identification of respiratory viruses based on silicon nanopores and a machine-learning algorithm, trained on the changes in current as the viruses pass through the nanopores was reported build a working tool for accurate distinguishing and identifying multiple viral species (Arima et al., 2021). An artificial neural network (ANN) model-assisted nanowire FET sensing system is also implemented for specific volatile organic compounds detection (Wang et al., 2014). Machine learning advances the FET-based technology for chemical and biological sensing, enabling the data analysis as well as ameliorated sensor design, while an ANN was implemented to convert the measured resistance change into analyte concentration in a system comprising SWCNTs ultimately targeting at ammonia and CO2 detection (Kim et al., 2019b). A nanoarray involving a random network of SWCNT combined with AI pattern analysis was showcased (Nakhleh et al., 2017) for non-invasive diagnosis and classification of a number of diseases of exhaled breath. Memristors have also proven their potential in seamlessly supporting a bio-AI fusion scheme (Serb et al., 2020). Thanks to their function resembling synaptic operations and plasticity and their ability to perform simultaneously memory-related and computation tasks, this technology may lead to independent and complete sensing-AI-processing systems with minimized energy requirements for extraction of medical information patterns, ultimately shaping diagnostics models out of big biological data.
Author contributions
All authors listed have made a substantial, direct, and intellectual contribution to the work and approved it for publication.
Funding
This work was supported in part by UGent Special Research Fund (BOF) (BOF/STA/202209/021), in part by the Engineering and Physical Sciences Research Council (EPSRC) Programme under Functional Oxide Reconfigurable Technologies (FORTE) Grant EP/R024642/2, in part by a SYnaptically connected brain-silicon Neural Closed-loop Hybrid system (SYNCH) under Grant H2020-FET- PROACT-2018-01, and in part by the RAEng Chair in Emerging Technologies under Grant CiET1819/2/93.
Conflict of interest
The authors declare that the research was conducted in the absence of any commercial or financial relationships that could be construed as a potential conflict of interest.
Publisher’s note
All claims expressed in this article are solely those of the authors and do not necessarily represent those of their affiliated organizations, or those of the publisher, the editors and the reviewers. Any product that may be evaluated in this article, or claim that may be made by its manufacturer, is not guaranteed or endorsed by the publisher.
References
Abdellaoui, S., Noiriel, A., Henkens, R., Bonaventura, C., Blum, L. J., and Doumeche, B. (2013). A 96-well electrochemical method for the screening of enzymatic activities. Anal. Chem. 85, 3690–3697. doi:10.1021/ac303777r
Adir, O., Poley, M., Chen, G., Froim, S., Krinsky, N., Shklover, J., et al. (2020). Integrating artificial intelligence and nanotechnology for precision cancer medicine. Adv. Mater. 32, 1901989. doi:10.1002/adma.201901989
Algamili, A. S., Khir, M. H. M., Dennis, J. O., Ahmed, A. Y., Alabsi, S. S., Ba Hashwan, S. S., et al. (2021). A review of actuation and sensing mechanisms in MEMS-based sensor devices. Nanoscale Res. Lett. 16, 16. doi:10.1186/s11671-021-03481-7
Alvarez, M. M., Aizenberg, J., Analoui, M., Andrews, A. M., Bisker, G., Boyden, E. S., et al. (2017). Emerging trends in micro- and nanoscale technologies in medicine: From basic discoveries to translation. ACS Nano 11, 5195–5214. doi:10.1021/acsnano.7b01493
Anselmetti, D. (2012). Tiny holes with great promise. Nat. Nanotechnol. 7, 81–82. doi:10.1038/nnano.2012.11
Arima, A., Tsutsui, M., Washio, T., Baba, Y., and Kawai, T. (2021). Solid-state nanopore platform integrated with machine learning for digital diagnosis of virus infection. Anal. Chem. 93, 215–227. doi:10.1021/acs.analchem.0c04353
Balme, S., Coulon, P. E., Lepoitevin, M., Charlot, B., Yandrapalli, N., Favard, C., et al. (2016). Influence of adsorption on proteins and amyloid detection by silicon nitride nanopore. Langmuir 32, 8916–8925. doi:10.1021/acs.langmuir.6b02048
Beamish, E., Kwok, H., Tabard-Cossa, V., and Godin, M. (2013). Fine-tuning the size and minimizing the noise of solid-state nanopores. J. Vis. Exp. 2013, e51081. doi:10.3791/51081
Behroudj, A., Geiger, D., and Strehle, S. (2019). Epitaxial bottom-up growth of silicon nanowires on oxidized silicon by alloy-catalyzed gas-phase synthesis. Nano Lett. 19, 7895–7900. doi:10.1021/acs.nanolett.9b02950
Bell, N. A. W., and Keyser, U. F. (2016). Digitally encoded DNA nanostructures for multiplexed, single-molecule protein sensing with nanopores. Nat. Nanotechnol. 11, 645–651. doi:10.1038/nnano.2016.50
Bergveld, P. (1970). Development of an ion-sensitive solid-state device for neurophysiological measurements. IEEE Trans. Biomed. Eng. BME 17, 70–71. doi:10.1109/tbme.1970.4502688
Bergveld, P. (1985). The impact of MOSFET-based sensors. Sensors Actuators 8, 109–127. doi:10.1016/0250-6874(85)87009-8
Bergveld, P. (2003). Thirty years of ISFETOLOGY: What happened in the past 30 years and what may happen in the next 30 years. Sensors Actuators B Chem. 88, 1–20. doi:10.1016/s0925-4005(02)00301-5
Bhalla, N., Pan, Y., Yang, Z., and Payam, A. F. (2020). Opportunities and challenges for biosensors and nanoscale analytical tools for pandemics: COVID-19. ACS Nano 14, 7783–7807. doi:10.1021/acsnano.0c04421
Bishop, M. D., Hills, G., Srimani, T., Lau, C., Murphy, D., Fuller, S., et al. (2020). Fabrication of carbon nanotube field-effect transistors in commercial silicon manufacturing facilities. Nat. Electron. 3, 492–501. doi:10.1038/s41928-020-0419-7
Bonanni, A., and Pumera, M. (2011). Graphene platform for hairpin-DNA-based impedimetric genosensing. ACS Nano 5, 2356–2361. doi:10.1021/nn200091p
Carlsen, A., and Cossa, V. T. (2020). Mapping shifts in nanopore signal to changes in protein and protein-DNA conformation. bioRxiv. doi:10.1101/2020.04.01.020420
Carrara, S. (2020). The birth of a new field: Memristive sensors. A review. IEEE Sensors J. 21, 12370–12378. doi:10.1109/JSEN.2020.3043305
Chakraborty, S., and Retna Raj, C. (2007). Amperometric biosensing of glutamate using carbon nanotube based electrode. Electrochem. Commun. 9, 1323–1330. doi:10.1016/j.elecom.2007.01.039
Chang, Y., Zuo, J., Zhang, H., and Duan, X. (2020). State-of-the-art and recent developments in micro/nanoscale pressure sensors for smart wearable devices and health monitoring systems. Nanotechnol. Precis. Eng. 3, 43–52. doi:10.1016/j.npe.2019.12.006
Chen, S., Mahmoodi, M. R., Shi, Y., Mahata, C., Yuan, B., Liang, X., et al. (2020). Wafer-scale integration of two-dimensional materials in high-density memristive crossbar arrays for artificial neural networks. Nat. Electron. 3, 638–645. doi:10.1038/s41928-020-00473-w
Chou, Y. C., Masih Das, P., Monos, D. S., and Drndić, M. (2020). Lifetime and stability of silicon nitride nanopores and nanopore arrays for ionic measurements. ACS Nano 14, 6715–6728. doi:10.1021/acsnano.9b09964
Chua, L. (1971). Memristor-The missing circuit element. IEEE Trans. Circuit Theory 18, 507–519. doi:10.1109/tct.1971.1083337
Cornell, B. A., Braach-Maksvytis, V. L. B., King, L. G., Osman, P. D. J., Raguse, B., Wieczorek, L., et al. (1997). A biosensor that uses ion-channel switches. Nature 387, 580–583. doi:10.1038/42432
Crnković, A., Srnko, M., and Anderluh, G. (2021). Biological nanopores: Engineering on demand. Life (Basel) 11, 27. doi:10.3390/life11010027
Cui, F., Yue, Y., Zhang, Y., Zhang, Z., and Zhou, H. S. (2020). Advancing biosensors with machine learning. ACS Sens. 5, 3346–3364. doi:10.1021/acssensors.0c01424
Cui, Y., Wei, Q., Park, H., and Lieber, C. M. (2001). Nanowire nanosensors for highly sensitive and selective detection of biological and chemical species. Science 293, 1289–1292. doi:10.1126/science.1062711
Dai, Y., Hu, H., Wang, M., Xu, J., and Wang, S. (2021). Stretchable transistors and functional circuits for human-integrated electronics. Nat. Electron. 4, 17–29. doi:10.1038/s41928-020-00513-5
Deamer, D., Akeson, M., and Branton, D. (2016). Three decades of nanopore sequencing. Nat. Biotechnol. 34, 518–524. doi:10.1038/nbt.3423
Dekker, S., Isgor, P. K., Feijten, T., Segerink, L. I., and Odijk, M. (2018). From chip-in-a-lab to lab-on-a-chip: A portable coulter counter using a modular platform. Microsystems Nanoeng. 4, 34–38. doi:10.1038/s41378-018-0034-1
Deng, T., Wang, Y., Chen, Q., Chen, H., and Liu, Z. (2016). Massive fabrication of silicon nanopore arrays with tunable shapes. Appl. Surf. Sci. 390, 681–688. doi:10.1016/j.apsusc.2016.07.171
Dias, R., and Torkamani, A. (2019). Artificial intelligence in clinical and genomic diagnostics. Genome Med. 11, 70. doi:10.1186/s13073-019-0689-8
Diederichs, T., Pugh, G., Dorey, A., Xing, Y., Burns, J. R., Hung Nguyen, Q., et al. (2019). Synthetic protein-conductive membrane nanopores built with DNA. Nat. Commun. 10, 5018. doi:10.1038/s41467-019-12639-y
Elfström, N., Juhasz, R., Sychugov, I., Engfeldt, T., Karlstrom, A. E., and Linnros, J. (2007). Surface charge sensitivity of silicon nanowires: Size dependence. Nano Lett. 7, 2608–2612. doi:10.1021/nl0709017
Erickson, D., Mandal, S., Yang, A. H. J., and Cordovez, B. (2008). Nanobiosensors: Optofluidic, electrical and mechanical approaches to biomolecular detection at the nanoscale. Microfluid Nanofluid 4, 33–52. doi:10.1007/s10404-007-0198-8
Feng, Y., Zhang, Y., Ying, C., Wang, D., and Du, C. (2015). Nanopore-based fourth-generation DNA sequencing technology. Genomics, Proteomics Bioinforma. 13, 4–16. doi:10.1016/j.gpb.2015.01.009
Fragasso, A., Schmid, S., and Dekker, C. (2020). Comparing current noise in biological and solid-state nanopores. ACS Nano 14, 1338–1349. doi:10.1021/acsnano.9b09353
Gao, W., Emaminejad, S., Nyein, H. Y. Y., Challa, S., Chen, K., Peck, A., et al. (2016). Fully integrated wearable sensor arrays for multiplexed in situ perspiration analysis. Nature 529, 509–514. doi:10.1038/nature16521
Gibani, M. M., Toumazou, C., Sohbati, M., Sahoo, R., Karvela, M., Hon, T. K., et al. (2020). Assessing a novel, lab-free, point-of-care test for SARS-CoV-2 (CovidNudge): A diagnostic accuracy study. Lancet Microbe 1, e300–e307. doi:10.1016/s2666-5247(20)30121-x
Giménez-Gómez, P., Rodriguez-Rodriguez, R., Rios, J. M., Perez-Montero, M., Gonzalez, E., Gutierrez-Capitan, M., et al. (2020). A self-calibrating and multiplexed electrochemical lab-on-a-chip for cell culture analysis and high-resolution imaging. Lab a Chip 20, 823–833. doi:10.1039/c9lc01051c
Goto, Y., Yanagi, I., Matsui, K., Yokoi, T., and Takeda, K. (2016). Integrated solid-state nanopore platform for nanopore fabrication via dielectric breakdown, DNA-speed deceleration and noise reduction. Sci. Rep. 6, 31324. doi:10.1038/srep31324
Guo, J., Li, H., Chen, Y., and Kang, Y. (2014). A microfluidic impedance cytometer on printed circuit board for low cost diagnosis. IEEE Sensors J. 14, 2112–2117. doi:10.1109/jsen.2013.2295399
Gupta, I., Serb, A., Khiat, A., Zeitler, R., Vassanelli, S., and Prodromakis, T. (2016). Real-time encoding and compression of neuronal spikes by metal-oxide memristors. Nat. Commun. 7, 12805–12809. doi:10.1038/ncomms12805
Gutiérrez-Capitán, M., Baldi, A., and Fernández-Sánchez, C. (2020). Electrochemical paper-based biosensor devices for rapid detection of biomarkers. Sensors 20, 967. doi:10.3390/s20040967
Hahm, J., and Lieber, C. M. (2004). Direct ultrasensitive electrical detection of DNA and DNA sequence variations using nanowire nanosensors. Nano Lett. 4, 51–54. doi:10.1021/nl034853b
Haick, H., and Tang, N. (2021). Artificial intelligence in medical sensors for clinical decisions. ACS Nano 15, 3557–3567. doi:10.1021/acsnano.1c00085
Hall, A. R., Scott, A., Rotem, D., Mehta, K. K., Bayley, H., and Dekker, C. (2010). Hybrid pore formation by directed insertion of α-haemolysin into solid-state nanopores. Nat. Nanotechnol. 5, 874–877. doi:10.1038/nnano.2010.237
Hannah, S., Blair, E., and Corrigan, D. K. (2020). Developments in microscale and nanoscale sensors for biomedical sensing. Curr. Opin. Electrochem. 23, 7–15. doi:10.1016/j.coelec.2020.02.012
Heller, I., Janssens, A. M., Mannik, J., Minot, E. D., Lemay, S. G., and Dekker, C. (2008). Identifying the mechanism of biosensing with carbon nanotube transistors. Nano Lett. 8, 591–595. doi:10.1021/nl072996i
Hierlemann, A., and Baltes, H. (2003). CMOS-based chemical microsensors. Analyst 128, 15–28. doi:10.1039/b208563c
Hille, B. (1978). Ionic channels in excitable membranes. Current problems and biophysical approaches. Biophys. J. 22, 283–294. doi:10.1016/s0006-3495(78)85489-7
Hobbs, R. G., Petkov, N., and Holmes, J. D. (2012). Semiconductor nanowire fabrication by bottom-up and top-down paradigms. Chem. Mater. 11, 1975–1991. doi:10.1021/cm300570n
Howorka, S. (2017). Building membrane nanopores. Nat. Nanotechnol. 12, 619–630. doi:10.1038/nnano.2017.99
Huang, Y., Duan, X., Wei, Q., and Lieber, C. M. (2001). Directed assembly of one-dimensional nanostructures into functional networks. Science 291, 630–633. doi:10.1126/science.291.5504.630
Huo, W., Ling, W., Wang, Z., Li, Y., Zhou, M., Ren, M., et al. (2021). Miniaturized DNA sequencers for personal use: Unreachable dreams or achievable goals. Front. Nanotechnol. 3, 628861. doi:10.3389/fnano.2021.628861
Ielmini, D., and Wong, H. S. P. (2018). In-memory computing with resistive switching devices. Nat. Electron. 1, 333–343. doi:10.1038/s41928-018-0092-2
Jeong, D. S., Kim, K. M., Kim, S., Choi, B. J., and Hwang, C. S. (2016). Memristors for energy-efficient new computing paradigms. Adv. Electron. Mater. 2, 1600090. doi:10.1002/aelm.201600090
Jin, X., Liu, C., Xu, T., Su, L., and Zhang, X. (2020). Artificial intelligence biosensors: Challenges and prospects. Biosens. Bioelectron. 165, 112412. doi:10.1016/j.bios.2020.112412
Justino, C. I. L., Rocha-Santos, T. A., Duarte, A. C., and Rocha-Santos, T. A. (2010). Review of analytical figures of merit of sensors and biosensors in clinical applications. TrAC Trends Anal. Chem. 29, 1172–1183. doi:10.1016/j.trac.2010.07.008
Kaisti, M. (2017). Detection principles of biological and chemical FET sensors. Biosens. Bioelectron. 98, 437–448. doi:10.1016/j.bios.2017.07.010
Kaprou, G. D., Papadopoulos, V., Papageorgiou, D. P., Kefala, I., Papadakis, G., Gizeli, E., et al. (2019). Ultrafast, low-power, PCB manufacturable, continuous-flow microdevice for DNA amplification. Anal. Bioanal. Chem. 411, 5297–5307. doi:10.1007/s00216-019-01911-1
Keene, S. T., Lubrano, C., Kazemzadeh, S., Melianas, A., Tuchman, Y., Polino, G., et al. (2020). A biohybrid synapse with neurotransmitter-mediated plasticity. Nat. Mater. 19, 969–973. doi:10.1038/s41563-020-0703-y
Kerr, E., Alexander, R., Francis, P. S., Guijt, R. M., Barbante, G. J., and Doeven, E. H. (2021). A comparison of commercially available screen-printed electrodes for electrogenerated chemiluminescence applications. Front. Chem. 8, 628483. doi:10.3389/fchem.2020.628483
Kim, B., Norman, T. J., Jones, R. S., Moon, D. i., Han, J. w., and Meyyappan, M. (2019). Carboxylated single-walled carbon nanotube sensors with varying pH for the detection of Ammonia and carbon dioxide using an artificial neural network. ACS Appl. Nano Mat. 2, 6445–6451. doi:10.1021/acsanm.9b01401
Kim, J., Campbell, A. S., de Ávila, B. E. F., and Wang, Z. (2019). Wearable biosensors for healthcare monitoring. Nat. Biotechnol. 37, 389–406. doi:10.1038/s41587-019-0045-y
Kim, K., Kim, M. J., Kim, D. W., Kim, S. Y., Park, S., and Park, C. B. (2020). Clinically accurate diagnosis of Alzheimer’s disease via multiplexed sensing of core biomarkers in human plasma. Nat. Commun. 11, 119. doi:10.1038/s41467-019-13901-z
Kim, M. J., Wanunu, M., Bell, D. C., and Meller, A. (2006). Rapid fabrication of uniformly sized nanopores and nanopore arrays for parallel DNA analysis. Adv. Mater. 18, 3149–3153. doi:10.1002/adma.200601191
Langecker, M., Arnaut, V., Martin, T. G., List, J., Renner, S., Mayer, M., et al. (2012). Synthetic lipid membrane channels formed by designed DNA nanostructures. Science 338, 932–936. doi:10.1126/science.1225624
Larkin, J., Foquet, M., Turner, S. W., Korlach, J., and Wanunu, M. (2014). Reversible positioning of single molecules inside zero-mode waveguides. Nano Lett. 14, 6023–6029. doi:10.1021/nl503134x
Lee, B. E., Kang, T., Jenkins, D., Li, Y., Wall, M. M., and Jun, S. (2022). A single-walled carbon nanotubes-based electrochemical impedance immunosensor for on-site detection of Listeria monocytogenes. J. Food Sci. 87, 280–288. doi:10.1111/1750-3841.15996
Lee, J., and Lu, W. D. (2018). On-demand reconfiguration of nanomaterials: When electronics meets ionics. Adv. Mater. 30, 1702770. doi:10.1002/adma.201702770
Lee, K. N., Jung, S. W., Kim, W. H., Lee, M. H., Shin, K. S., and Seong, W. K. (2007). Well controlled assembly of silicon nanowires by nanowire transfer method. Nanotechnology 18, 445302. doi:10.1088/0957-4484/18/44/445302
Lei, K. M., Mak, P. I., Law, M. K., and Martins, P. R. (2016). CMOS biosensors for in vitro diagnosis – transducing mechanisms and applications. Lab a Chip 16, 3664–3681. doi:10.1039/c6lc01002d
Leïchlé, T., Nicu, L., and Alava, T. (2020). MEMS biosensors and COVID-19: Missed opportunity. ACS Sens. 5, 3297–3305. doi:10.1021/acssensors.0c01463
Liu, L., Han, J., Xu, L., Zhou, J., Zhao, C., Ding, S., et al. (2020). Aligned, high-density semiconducting carbon nanotube arrays for high-performance electronics. Science 368, 850–856. doi:10.1126/science.aba5980
Maehashi, K., and Matsumoto, K. (2009). Label-free electrical detection using carbon nanotube-based biosensors. Sensors 9, 5368–5378. doi:10.3390/s90705368
Magierowski, S., Huang, Y., Wang, C., and Ghafar-Zadeh, E. (2016). Nanopore-CMOS interfaces for DNA sequencing. Biosens. (Basel) 6, 42. doi:10.3390/bios6030042
Manrao, E. A., Derrington, I. M., Laszlo, A. H., Langford, K. W., Hopper, M. K., Gillgren, N., et al. (2012). Reading DNA at single-nucleotide resolution with a mutant MspA nanopore and phi29 DNA polymerase. Nat. Biotechnol. 30, 349–353. doi:10.1038/nbt.2171
Martinez, A. W., Phillips, S. T., Butte, M. J., and Whitesides, G. M. (2007). Patterned paper as a platform for inexpensive, low-volume, portable bioassays. Angew. Chem. Int. Ed. 46, 1318–1320. doi:10.1002/anie.200603817
Merkel, T., Graeber, M., and Pagel, L. (1999). A new technology for fluidic microsystems based on PCB technology. Sensors Actuators A Phys. 77, 98–105. doi:10.1016/s0924-4247(99)00062-x
Meyyappan, M., and Sunkara, M. K. (2018). Inorganic nanowires: Applications, properties, and characterization. USA: CRC Press.
Min, S. Y., Kim, T. S., Kim, B. J., Cho, H., Noh, Y. Y., Yang, H., et al. (2013). Large-scale organic nanowire lithography and electronics. Nat. Commun. 4, 1773. doi:10.1038/ncomms2785
Min, S. Y., Kim, T. S., Lee, Y., Cho, H., Xu, W., and Lee, T. W. (2015). Organic nanowire fabrication and device applications. Small 11, 45–62. doi:10.1002/smll.201401487
Mincu, N. B., Lazar, V., Stan, D., Mihailescu, C. M., Iosub, R., and Mateescu, A. L. (2020). Screen-printed electrodes (SPE) for in vitro diagnostic purpose. Diagnostics 10, 517. doi:10.3390/diagnostics10080517
Mitra, S., and Cumming, D. R. S. (2018). CMOS circuits for biological sensing and processing. USA: Springer International Publishing. doi:10.1007/978-3-319-67723-1
Moschou, D., and Tserepi, A. (2017). The lab-on-PCB approach: Tackling the μTAS commercial upscaling bottleneck. Lab. Chip 17, 1388–1405. doi:10.1039/c7lc00121e
Moser, N., Lande, T. S., Toumazou, C., and Georgiou, P. (2016). ISFETs in CMOS and emergent trends in instrumentation: A review. IEEE Sensors J. 16, 6496–6514. doi:10.1109/jsen.2016.2585920
Muthukumar, M., Plesa, C., and Dekker, C. (2015). Single-molecule sensing with nanopores. Phys. Today 68, 40–46. doi:10.1063/pt.3.2881
Nakatsuka, N., Yang, K. A., Abendroth, J. M., Cheung, K. M., Xu, X., Yang, H., et al. (2018). Aptamer–field-effect transistors overcome Debye length limitations for small-molecule sensing. Science 362, 319–324. doi:10.1126/science.aao6750
Nakhleh, M. K., Amal, H., Jeries, R., Broza, Y. Y., Aboud, M., Gharra, A., et al. (2017). Diagnosis and classification of 17 diseases from 1404 subjects via pattern analysis of exhaled molecules. ACS Nano 11, 112–125. doi:10.1021/acsnano.6b04930
Park, H., Afzali, A., Han, S. J., Tulevski, G. S., Franklin, A. D., Tersoff, J., et al. (2012). High-density integration of carbon nanotubes via chemical self-assembly. Nat. Nanotechnol. 7, 787–791. doi:10.1038/nnano.2012.189
Patolsky, F., and Lieber, C. M. (2005). Nanowire nanosensors. Mater. Today 8, 20–28. doi:10.1016/s1369-7021(05)00791-1
Patolsky, F., Zheng, G., Hayden, O., Lakadamyali, M., Zhuang, X., and Lieber, C. M. (2004). Electrical detection of single viruses. PNAS 101, 14017–14022. doi:10.1073/pnas.0406159101
Patolsky, F., Zheng, G., and Lieber, C. M. (2006). Nanowire sensors for medicine and the life sciences. Nanomedicine 1, 51–65. doi:10.2217/17435889.1.1.51
Perdigones, F. (2021). Lab-on-PCB and flow driving: A critical review. Micromachines 12, 175. doi:10.3390/mi12020175
Pi, S., Li, C., Jiang, H., Xia, W., Xin, H., Yang, J. J., et al. (2019). Memristor crossbar arrays with 6-nm half-pitch and 2-nm critical dimension. Nat. Nanotechnol. 14, 35–39. doi:10.1038/s41565-018-0302-0
Prindle, A., Samayoa, P., Razinkov, I., Danino, T., Tsimring, L. S., and Hasty, J. (2012). A sensing array of radically coupled genetic ‘biopixels. Nature 481, 39–44. doi:10.1038/nature10722
Rao, S. G., Huang, L., Setyawan, W., and Hong, S. (2003). Large-scale assembly of carbon nanotubes. Nature 425, 36–37. doi:10.1038/425036a
Ren, J., and Licht, S. (2016). Tracking airborne CO 2 mitigation and low cost transformation into valuable carbon nanotubes. Sci. Rep. 6, 27760. doi:10.1038/srep27760
Rothberg, J. M., Hinz, W., Rearick, T. M., Schultz, J., Mileski, W., Davey, M., et al. (2011). An integrated semiconductor device enabling non-optical genome sequencing. Nature 475, 348–352. doi:10.1038/nature10242
Sakata, T. (2019). Biologically coupled gate field-effect transistors meet in vitro diagnostics. ACS Omega 4, 11852–11862. doi:10.1021/acsomega.9b01629
Samson, R., Navale, G. R., and Dharne, M. S. (2020). Biosensors: Frontiers in rapid detection of COVID-19. 3 Biotech. 10, 385. doi:10.1007/s13205-020-02369-0
Sánchez, J. L. A., Henry, O., Joda, H., Solnestam, B., Kvastad, L., Johansson, E., et al. (2016). Multiplex PCB-based electrochemical detection of cancer biomarkers using MLPA-barcode approach. Biosens. Bioelectron. 82, 224–232. doi:10.1016/j.bios.2016.04.018
Schöning, M. J., and Poghossian, A. (2002). Recent advances in biologically sensitive field-effect transistors (BioFETs). Analyst 127, 1137–1151. doi:10.1039/b204444g
Serb, A., Corna, A., George, R., Khiat, A., Rocchi, F., Reato, M., et al. (2020). Memristive synapses connect brain and silicon spiking neurons. Sci. Rep. 10, 2590–2597. doi:10.1038/s41598-020-58831-9
Shamkhalichenar, H., Bueche, C. J., and Choi, J. W. (2020). Printed circuit board (PCB) technology for electrochemical sensors and sensing platforms. Biosensors 10, 159. doi:10.3390/bios10110159
Shulaker, M. M., Hills, G., Park, R. S., Howe, R. T., Saraswat, K., Wong, H. S. P., et al. (2017). Three-dimensional integration of nanotechnologies for computing and data storage on a single chip. Nature 547, 74–78. doi:10.1038/nature22994
Snow, E. S., Perkins, F. K., Houser, E. J., Badescu, S. C., and Reinecke, T. L. (2005). Chemical detection with a single-walled carbon nanotube capacitor. Science 307, 1942–1945. doi:10.1126/science.1109128
Storm, A. J., Chen, J. H., Ling, X. S., Zandbergen, H. W., and Dekker, C. (2003). Fabrication of solid-state nanopores with single-nanometre precision. Nat. Mater. 2, 537–540. doi:10.1038/nmat941
Sugawara, M., Kojima, K., Sazawa, H., and Umezawa, Y. (1987). Ion-channel sensors. Anal. Chem. 59, 2842–2846. doi:10.1021/ac00151a004
Tang, Z., Zhang, D., Cui, W., Zhang, H., Pang, W., and Duan, X. (2016). Fabrications, applications and challenges of solid-state nanopores: A mini review. Nanomater. Nanotechnol. 6, 35. doi:10.5772/64015
Toumazou, C., Shepherd, L. M., Reed, S. C., Chen, G. I., Patel, A., Garner, D. M., et al. (2013). Simultaneous DNA amplification and detection using a pH-sensing semiconductor system. Nat. Methods 10, 641–646. doi:10.1038/nmeth.2520
Tsai, D., Sawyer, D., Bradd, A., Yuste, R., and Shepard, K. L. (2017). A very large-scale microelectrode array for cellular-resolution electrophysiology. Nat. Commun. 8, 1802. doi:10.1038/s41467-017-02009-x
Tseng, H. Y., Lum, J., Malfesi, S., and Gray, B. L. (2015). Development of rapid screening for glucose-6-phosphate dehydrogenase deficiency prior to malaria treatment utilizing on-board pH-based electrochemical assay. Measurement 73, 158–161. doi:10.1016/j.measurement.2015.05.012
Tzouvadaki, I., Stathopoulos, S., Abbey, T., Michalas, L., and Prodromakis, T. (2020). Monitoring PSA levels as chemical state-variables in metal-oxide memristors. Sci. Rep. 10, 15281. doi:10.1038/s41598-020-71962-3
Varongchayakul, N., Song, J., Meller, A., and Grinstaff, M. W. (2018). Single-molecule protein sensing in a nanopore: A tutorial. Chem. Soc. Rev. 47, 8512–8524. doi:10.1039/c8cs00106e
Vashist, S. K. (2017). Point-of-Care diagnostics: Recent advances and trends. Biosens. (Basel) 7, 62. doi:10.3390/bios7040062
Vasilakis, N., Papadimitriou, K. I., Evans, D., Morgan, H., and Prodromakis, T. (2016). “The Lab-on-PCB framework for affordable, electronic-based point-of-care diagnostics: From design to manufacturing,” in 2016 IEEE Healthcare Innovation Point-Of-Care Technologies Conference (HI-POCT), Cancun, Mexico, 09-11 November 2016 (IEEE). doi:10.1109/HIC.2016.7797713
Venkatesan, B. M., and Bashir, R. (2011). Nanopore sensors for nucleic acid analysis. Nat. Nanotech 6, 615–624. doi:10.1038/nnano.2011.129
Vijayaraghavan, A., Blatt, S., Weissenberger, D., Oron-Carl, M., Hennrich, F., Gerthsen, D., et al. (2007). Ultra-large-scale directed assembly of single-walled carbon nanotube devices. Nano Lett. 7, 1556–1560. doi:10.1021/nl0703727
Wadhera, T., Kakkar, D., Wadhwa, G., and Raj, B. (2019). Recent advances and progress in development of the field effect transistor biosensor: A review. J. Elec Materi 48, 7635–7646. doi:10.1007/s11664-019-07705-6
Wang, B., Cancilla, J. C., Torrecilla, J. S., and Haick, H. (2014). Artificial sensing intelligence with silicon nanowires for ultraselective detection in the gas phase. Nano Lett. 14, 933–938. doi:10.1021/nl404335p
Wang, J., Li, Z., and Gu, Z. (2021). A comprehensive review of template-synthesized multi-component nanowires: From interfacial design to sensing and actuation applications. Sensors Actuators Rep. 3, 100029. doi:10.1016/j.snr.2021.100029
Wang, L., Estrela, P., Huq, E., Li, P., Thomas, S., Ferrigno, P. K., et al. (2010). Fabrication of BioFET linear array for detection of protein interactions. Microelectron. Eng. 87, 753–755. doi:10.1016/j.mee.2009.11.148
Wu, J., Dong, M., Rigatto, C., Liu, Y., and Lin, F. (2018). Lab-on-chip technology for chronic disease diagnosis. npj Digit. Med. 1, 7–11. doi:10.1038/s41746-017-0014-0
Wu, Y., Yao, Y., Cheong, S., Tilley, D., and Justin Gooding, J. (2020). Selectively detecting attomolar concentrations of proteins using gold lined nanopores in a nanopore blockade sensor. Chem. Sci. 11, 12570–12579. doi:10.1039/d0sc04552g
Xia, Q., Yang, J. J., Wu, W., Li, X., and Williams, R. S. (2010). Self-aligned memristor cross-point arrays fabricated with one nanoimprint lithography step. Nano Lett. 11, 2909–2914. doi:10.1021/nl1017157
Xia, Q. (2016). “Memristive nanodevices: CMOS compatibility and novel applications,” in 2016 18th Mediterranean Electrotechnical Conference (MELECON), Lemesos, Cyprus, 18-20 April 2016 (IEEE), 1–4. doi:10.1109/MELCON.2016.7495316
Xia, Q., and Yang, J. J. (2019). Memristive crossbar arrays for brain-inspired computing. Nat. Mater. 18, 309–323. doi:10.1038/s41563-019-0291-x
Xue, L., Yamazaki, H., Ren, R., Wanunu, M., Ivanov, A. P., and Edel, J. B. (2020). Solid-state nanopore sensors. Nat. Rev. Mater. 5, 931–951. doi:10.1038/s41578-020-0229-6
Yusko, E. C., Bruhn, B. R., Eggenberger, O. M., Houghtaling, J., Rollings, R. C., Walsh, N. C., et al. (2017). Real-time shape approximation and fingerprinting of single proteins using a nanopore. Nat. Nanotechnol. 12, 360–367. doi:10.1038/nnano.2016.267
Zhang, G. J., and Ning, Y. (2012). Silicon nanowire biosensor and its applications in disease diagnostics: A review. Anal. Chim. Acta 749, 1–15. doi:10.1016/j.aca.2012.08.035
Zhao, L., Han, H., and Ma, Z. (2018). Improved screen-printed carbon electrode for multiplexed label-free amperometric immuniosensor: Addressing its conductivity and reproducibility challenges. Biosens. Bioelectron. 101, 304–310. doi:10.1016/j.bios.2017.10.041
Zhao, W., Tian, S., Huang, L., Liu, K., and Dong, L. (2020). The review of Lab-on-PCB for biomedical application. ELECTROPHORESIS 41, 1433–1445. doi:10.1002/elps.201900444
Zheng, G., Patolsky, F., Cui, Y., Wang, W. U., and Lieber, C. M. (2005). Multiplexed electrical detection of cancer markers with nanowire sensor arrays. Nat. Biotechnol. 23, 1294–1301. doi:10.1038/nbt1138
Zhou, P., Hou, J., Yan, Y., and Wang, Z. (2018). The effect of surfactant adsorption on surface wettability and flow resistance in slit nanopore: A molecular dynamics study. J. Colloid Interface Sci. 513, 379–388. doi:10.1016/j.jcis.2017.11.045
Zhu, H., Fohlerová, Z., Pekárek, J., Basova, E., and Neužil, P. (2020). Recent advances in lab-on-a-chip technologies for viral diagnosis. Biosens. Bioelectron. 153, 112041. doi:10.1016/j.bios.2020.112041
Zidan, M. A., Strachan, J. P., and Lu, W. D. (2018). The future of electronics based on memristive systems. Nat. Electron. 1, 22–29. doi:10.1038/s41928-017-0006-8
Keywords: large-scale, biosensor, biosensor array fabrication, nanotechnology, nanodevice
Citation: Tzouvadaki I and Prodromakis T (2023) Large-scale nano-biosensing technologies. Front. Nanotechnol. 5:1127363. doi: 10.3389/fnano.2023.1127363
Received: 19 December 2022; Accepted: 30 January 2023;
Published: 15 February 2023.
Edited by:
Carlo Ricciardi, Polytechnic University of Turin, ItalyReviewed by:
Vishva Ray, University of Michigan, United StatesGianluca Milano, National Institute of Metrological Research (INRiM), Italy
Copyright © 2023 Tzouvadaki and Prodromakis. This is an open-access article distributed under the terms of the Creative Commons Attribution License (CC BY). The use, distribution or reproduction in other forums is permitted, provided the original author(s) and the copyright owner(s) are credited and that the original publication in this journal is cited, in accordance with accepted academic practice. No use, distribution or reproduction is permitted which does not comply with these terms.
*Correspondence: Themis Prodromakis, t.prodromakis@ed.ac.uk