- School of Electronic Science and Engineering, Nanjing University, Nanjing, China
The sensory nervous system serves as the window for human beings to perceive the outside world by converting external stimuli into distinctive spiking trains. The sensory neurons in this system can process multimodal sensory signals with extremely low power consumption. Therefore, new-concept devices inspired by the sensory neuron are promising candidates to address energy issues in nowadays’ robotics, prosthetics and even computing systems. Recent years have witnessed rapid development in transistor-based bionic perceptual devices, and it is urgent to summarize the research and development of these devices. In this review, the latest progress of transistor-based bionic perceptual devices for artificial sense is reviewed and summarized in five aspects, i.e., vision, touch, hearing, smell, and pain. Finally, the opportunities and challenges related to these areas are also discussed. It would have bright prospects in the fields of artificial intelligence, prosthetics, brain-computer interface, robotics, and medical testing.
Introduction
With the spread of the Internet of Things (IoT) and artificial intelligence (AI), dataset sizes of images, documents, speeches, and videos have exploded, becoming a heavy burden on transmission networks and processing units. Classical von Neumann-based computing systems depend on centralized and sequential operations with a clock cycle (Hu et al., 2018; Park et al., 2020), so the information processing of the von Neumann computer requires a large amount of data transmission between processors and memory units, which will inevitably limit the computational efficiency and the scalability of the architecture. In addition, von Neumann computers face a dramatic increase in energy consumption while increasing their computing speed (Dai et al., 2019). Biological nervous systems are based on distributed, parallel, and event-driven operations (Park et al., 2020), these characteristics allow us to perceive and react appropriately when confronting the events of the real world in a more robust, plastic, fault-tolerant, and energy-efficient manners than current digital systems (Merolla et al., 2014; Xia and Yang, 2019; Wan et al., 2020b). The human brain is a neuronal network connected by synapses (Yu et al., 2021). Synaptic responses to inputs can change; this adaptability is the basis of learning. Synapses also perform computing and memory concurrently; this ability has the advantages of faster data processing and lower power consumption than traditional von Neumann machines, which perform these functions in separate areas. The development of brain-like computers requires artificial synapses that have the adaptability of real synapses. The development of artificial synapses would also lead to artificial systems that perform sensory functions (perception, hearing, vision, and motion) and thereby open a new era of bioinspired electronics for next-generation prosthetics and neurorobotics.
The nervous system of vertebrates can be divided into the central nervous system (CNS, the brain and spinal cord) and the peripheral nervous system (PNS, the sensory and motor organs and nerves). The CNS and PNS work together to recognize external information, make appropriate decisions, and control the mechanics of the body (Lee and Lee, 2019). Humans can recognize information from external stimuli (e.g., light, pressure, sound, gas, and flavor) through a biological sensory system consisting of the sensory part of the PNS and the computational part of the CNS. The sensory part of the PNS (sensory organs and nerves) detects external stimuli (e.g., light, pressure, sound, gas, and flavor), and the computing part of the CNS recognizes stimuli information and makes appropriate decisions (Cai et al., 2021; Cho et al., 2021). In the biological sensory system, there are corresponding receptors that initiate a sensation in response to physical stimulus. Sensory receptors are the interface between the external environment and biological nervous system. Receptor categories in humans include chemoreceptors, photoreceptors, mechanoreceptors, and thermoreceptors. Chemoreceptors are mainly found in the nose and tongue, which receive chemical stimuli and provide olfactory or gustatory information. Photoreceptors lie inside the subretinal space of the retina and receive visual information through the corneal lens of the eye. Mechanoreceptors are randomly distributed in human skin and respond to mechanical stimuli, such as pressure, distortion, and vibration. Temperature receptors are distributed throughout the dermis and in certain organs, such as the liver and muscles, which detect cold and warmth, respectively. Nociceptors respond to damaging stimuli and are also known as pain receptors (Jung et al., 2019).
The circuitry of the human brain is composed of a trillion (1012) neurons and a quadrillion (1015) synapses, whose connectivity underlies all human perception, emotion, thought, and behavior (Ho et al., 2011; Xu et al., 2016; Yu et al., 2021). The power consumption of the brain is only on the order of fJ/spike (Sun et al., 2021) and the power consumption of parallel processing is 20 W (Zhu et al., 2022). Action potentials generated in a presynaptic neuron propagate through the axon and arrive at synapses, where they transfer important information to postsynaptic neuron. Synaptic weight is the strength of the link between presynaptic neurons and postsynaptic neurons. Synaptic plasticity is a biological process in which specific patterns of synaptic activity result in changes in synaptic weight, and it is regarded as the basic mechanism of learning and memory in the brain, allowing it to learn from experience (Han et al., 2019). A number of bionic perceptual devices that imitate the human brain have been developed, inspired by the human nervous system. Professor Chua conceptualized the memristor idea in 1971 (Chua, 1971). Until 2008, Hewlett-Packard (HP) linked its theoretical and physical models to develop a memristor with adjustable resistance and memory (Strukov et al., 2008). Synaptic devices of various materials, architectures, and mechanisms have since been proposed to simulate synaptic plasticity. Bionic perceptual devices can be classified into three types based on their operation: ion migration, phase transition, and electron migration (Zhu et al., 2020). Two-terminal memristors and three-terminal synaptic transistors are two types of synaptic devices that have received intensive attention. A two-terminal memristor has a simple structure with only three layers: two electrodes for sending and receiving electrical impulses, and a “storage” layer in between. It is not the same as static resistor, which can be utilized to store, process, and store data (Zidan et al., 2018). There have been proposed two-terminal memristor devices based on electrochemical metallization mechanism (ECM), valence change mechanism (VCM), phase-change mechanism (PCM), and ferroelectric mechanism (FeM). The memory layer of memristor with a high density is suited for high-density electronic devices. However, performing both signal transmission and self-learning activities at the same time is difficult for such devices (Nishitani et al., 2012). The three-terminal synaptic transistors could potentially address this disadvantage of the two-terminal memristor. Floating gate transistors, electrochemical transistors, ferroelectric field effect transistors, and photo-synaptic transistors have all been developed and successfully mimicked essential synaptic plasticity including inhibitory postsynaptic currents (IPSC), excitatory postsynaptic currents (EPSC), long-term plasticity (LTP), short-term plasticity (STP), paired-pulse facilitation (PPF), spike-timing-dependent plasticity (STDP), and spike-rate-dependent plasticity (SRDP). For example, the electric-double-layer (EDL) transistors can achieve high current output with low gate voltage. The larger the EDL capacitance, the lower the gate voltage, the higher the output current, so it has great promise for low-power electronic devices. Jiang et al. (2009) fabricated ultralow-voltage InGaZnO4 TFTs gated with mesoporous SiO2 dielectric grown by plasma-enhanced chemical vapor deposition PECVD at room temperature. Subsequently, Zhou et al. (2013) reported the fabrication of flexible indium-zinc-oxide (IZO) EDL transistors on PET plastic substrates by a simple self-assembly method. Such flexible EDL transistors exhibit an excitatory postsynaptic current (EPSC), Simultaneously, paired-pulse facilitation (PPF) and long-term memory are also successfully mimicked in flexible artificial synapses. Sensory neuron incorporates receptors for detecting the external stimulations, afferent nerve fiber for information transmitting, and synapses for communicating with other neurons. Synapse is also regarded as the information preprocessing element in a sensory neuron. In parallel, a bionic perceptual device might have sensors, ionic cable or signal convertor, an artificial synapse. The artificial synapse in a bionic perceptual device is also response for preprocessing of sensory information, e.g., signal refining, integration, or memory. So, artificial synapse is also an important component for a bionic perceptual device. Bionic perceptual device with sensing and processing capabilities like as light, sound, electricity, chemicals, and pressure, have been developed over the last 10 years (Park et al., 2020; Zeng et al., 2021). More recently, synaptic devices integrated with multiple sensory modalities (Wan et al., 2020a; Taunyazov et al., 2020) were also developed, which are able to fuse multiple sensory stimulations in the manner of sensory neurons (Zeng et al., 2021). This has paved the way for next-generation bionic perceptual computing devices to be developed (Zhu et al., 2022). In this review, recent progress in transistor-based bionic perceptual devices with respect to device structure, operating principle, and realization function will be discussed firstly. The advancement of research in visual perception, tactile perception, auditory perception, olfactory perception, and pain perception will also be reviewed (Figure 1). Finally, the opportunities and challenges of transistor-based bionic perceptual devices will also be provided. We positively believe that the development of transistor-based bionic perceptual devices will bring a bright future for the development of various intelligent applications in the era of big data.
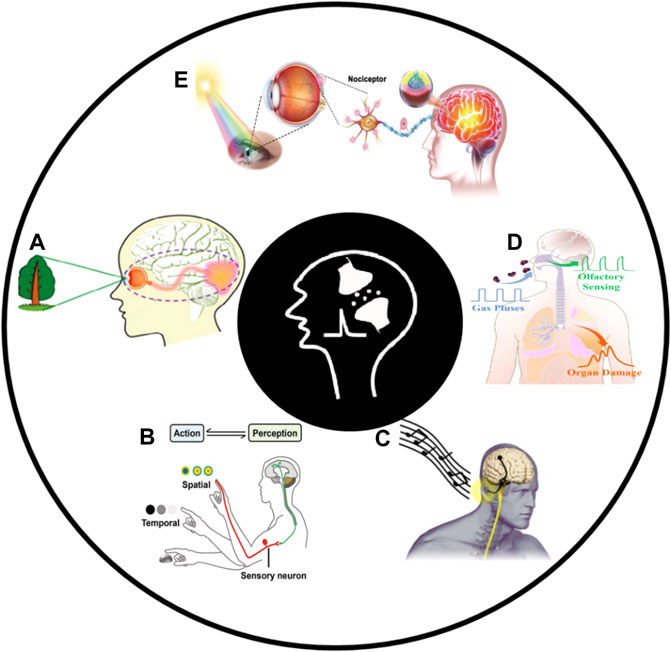
FIGURE 1. (A) Visual perception (adapted from Gao et al., 2019). (B) Tactile perception (adapted from Wan et al., 2018). (C) Auditory perception (adapted from Seo et al., 2019). (D) Olfactory perception (adapted from Song et al., 2019). (E) Nociceptor Perception (adapted from Feng et al., 2021).
Visual Perception
One of the most important organs of the human body is the eye, which can perform neural information processing and feature detection with direct optical inputs (Kumar et al., 2019b). It provides crucial visual information to distinguish the size, shape, color, volume, outline, material, and brightness of objects, distance, location sensation, smoothness, and roughness of the object’s surface, etc., (Quiroga et al., 2005; Sligte et al., 2010). Human visual memory is formed by receiving image information from the retina and allows individuals to remember the impressions of images our eyes observed (Gkoupidenis et al., 2016; Chen et al., 2018; Xie et al., 2018) (Figure 2A). Agnus et al. (2010) demonstrated that light-controlled single-walled carbon nanotubes (SWNTs) as programmable resistors can be used to store synaptic weights in adaptive nanoarchitectures, where synapses will be constructed at the nanoscale. Qu et al. (2020) demonstrated a monolithic integrated circuit with dual functions of sensing and storage with an active channel composed of carbon nanotubes. Li et al. (2016) demonstrated a synaptic transistor based on an indium gallium zinc oxide (IGZO) -aluminum oxide (Al2O3) thin-film structure. The device stimulated with UV light as the input, simulated synaptic plasticity. However they may require more complex structural systems. Lee et al. (2017) reported photonic bionic perceptual devices based on amorphous oxide semiconductors (AOSs) to mimic important synaptic functions. Lei et al. (2022) demonstrate a memristor based on 2D bismuth oxyiodide (BiOI) nanosheets. The memristor demonstrates not only electrical voltage-driven long-term potentiation, depression plasticity, and paired-pulse facilitation, but also light-induced short- and long-term plasticity. Moreover, the photonic synapse can be used to simulate the “learning experience” behaviors of human brain.
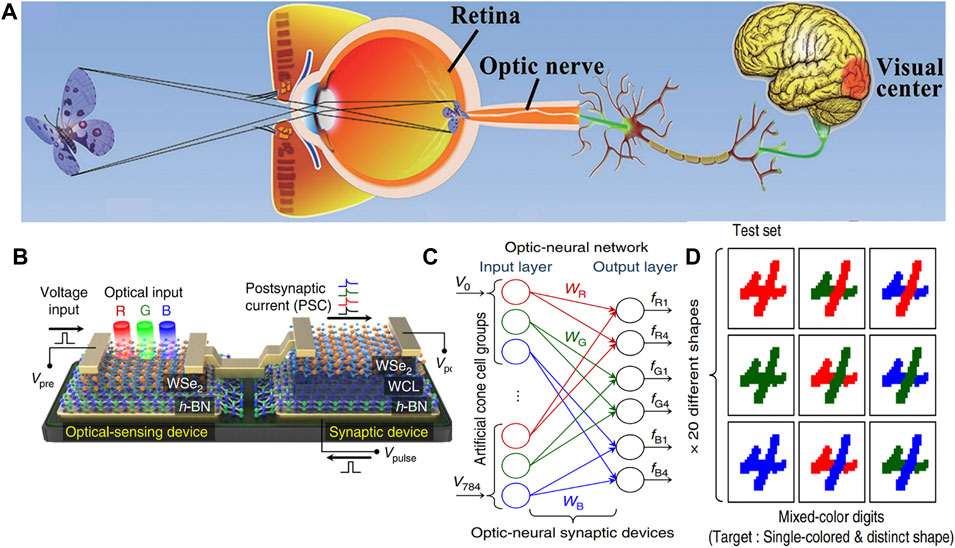
FIGURE 2. (A) Schematic diagrams of human visual system when a butterfly was observed by eyes (adapted from Chen et al., 2018). (B) The h-BN/WSe2 synaptic device integrated with h-BN/WSe2 photodetector. (C) Developed optic neural network for recognition of 28 × 28 RGB-colored images. (D) Examples of the training and the testing datasets consisting of single-colored and color-mixed numeric pattern images, respectively (adapted from Seo et al., 2018).
Seo et al. (2018) fabricated an optic-neural synaptic (ONS) device by integrating a synaptic device with an optical-sensing device on a van der Waals (vdW) heterostructure (h-BN/WSe2) (Figure 2B). The h-BN flake was then transferred onto the control electrode, which was patterned on the 90 nm-thick SiO2 oxide layer on heavily boron-doped Si substrate. The WSe2 flake was transferred to the h-BN/SiO2/Si substrate by the residue-free transfer method. The electrodes were patterned on the h-BN/WSe2 sample by an optical lithography process which were connected to the presynaptic terminal of the vdW synaptic device, followed by 10 nm thick Pt and 50 nm thick Au via an e-beam evaporator. This device mimics the colored and color-mixed pattern recognition capabilities of the human vision system. The synaptic connections of traditional neural networks do not have optical sensing capabilities to distinguish colors. Optical sensing in optic-neural network (ONN) is added to synaptic connections, so the device can distinguish colors like the human eye (Figure 2C) and recognize target digits from mixed color patterns (Figure 2D).
Chen et al. (2018) designed a bioinspired flexible visual memory system by the rational integration of UV image sensors and resistive switching memristors. 30nm gold patterned film was prepared on a substrate deposited with semiconductor micrometer-sized wires (SMWs) by thermal evaporation and photolithography as the bottom electrode and a 23 nm gold film was prepared by photolithography and atomic layer deposition. Al2O3 memory layer and 70 nm Ni top electrode were prepared by photolithography and thermal evaporation. Resistive switching of the memristor from the OFF state to the ON state when the photo response reaches a critical value. Visual memory can realize “write” and “erase” operations, so it has proved that bistable memory switching is fully reversible and repeatable. A 10 × 10 pixel flexible visual memory array was fabricated to record patterned light information. The memristor and image sensor were connected in series as a visual memory unit (Figure 3A). Butterfly-shaped pattern photo produced by UV irradiation through a diffraction optical element (DOE) image. Only the device exposed to the patterned light has a photo response (Figure 3B). After the light was removed, each pixel unit was recorded in the memory array. It was left at room temperature for a week, the memory array still had a butterfly pattern with little attenuation (Figure 3C).
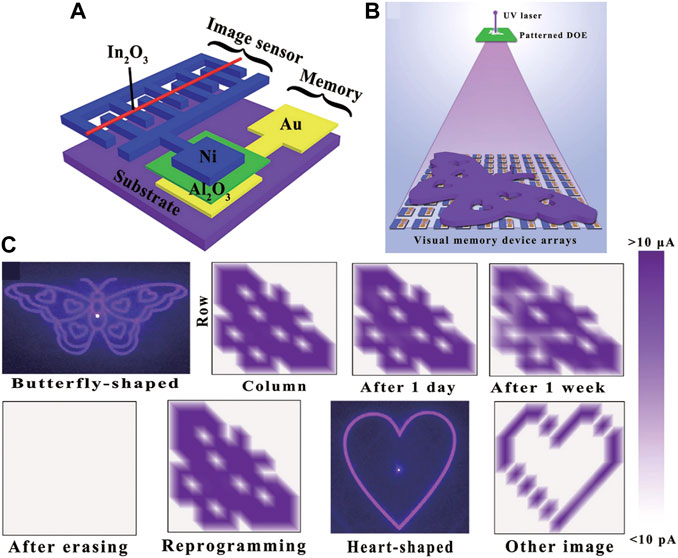
FIGURE 3. (A) Schematic diagram of the bio-inspired visual memory unit integrated by resistive switching memory device and image sensor. (B) Schematic diagram of the light distribution information produced by the pattern DOE. (C) Information Storage Behavior of Flexible Visual Memory Device Arrays (adapted from Chen et al., 2018).
Although researchers have successfully developed artificial visual perception devices, implementing an adaptive visual perception system that changes with ambient light is a great challenge for transistor-based bionic perceptual visual perception. Kwon et al. (2019) took advantage of a simply designed light-adjustable optoelectronic transistor-based bionic perceptual circuit array consisting of a photovoltaic divider and an ionotronic synaptic transistor to emulate the biological visual functionalities, demonstrating the environment-adaptable artificial visual perception system (Figure 4A). Various synaptic functions such as short-term plasticity, long-term plasticity, and neural facilitation were achieved. The ability of the artificial retina to automatically adjust light according to the external environment was simulated (Figure 4B).
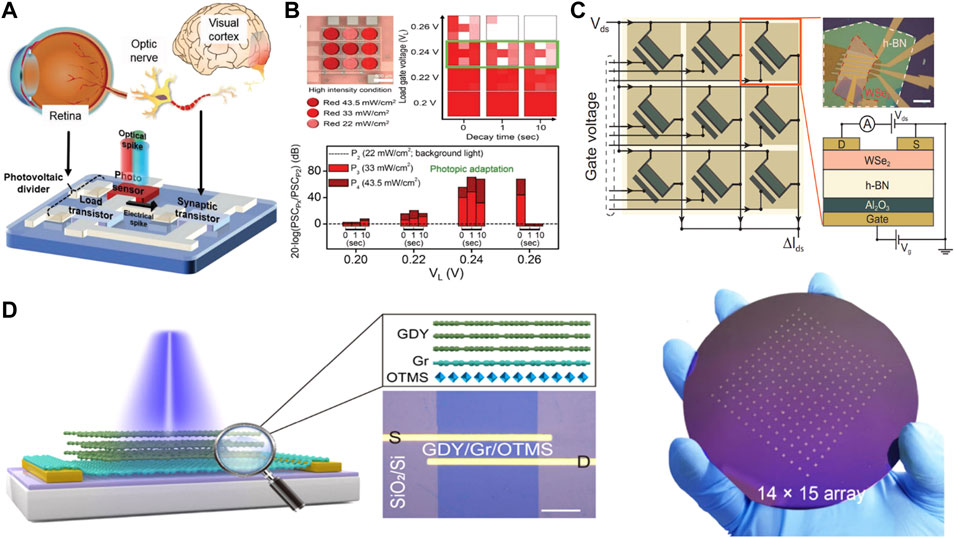
FIGURE 4. (A) Schematic diagram of a light-tunable optoelectronic transistor-based bionic perceptual circuit consisting of a photovoltaic voltage divider and an ionic synaptic transistor.(B) Low-intensity (10.5–33 mW cm−2) and red illuminance gradation, respectively. For the PSC decibel levels, P1–P4 represent red-light intensities of 10.5, 22, 33, and 43.5 mW cm−2, respectively (adapted from Kwon et al., 2019). (C) Schematic diagram of a 3 × 3 retinal morphometric sensor with WSe2/h-BN/Al2O3 vdW device (adapted from Wang S. et al., 2021).(D) Schematic of the GDY/Gr-based transistor and its optical microscopy image and photograph of a 14 × 15 GDY/Gr-based transistor array on a SiO2/Si wafer (adapted from Zhang et al., 2021).
Wang S. et al. (2021) fabricate the retinomorphic sensor based on WSe2/h-BN/Al2O3 vdW heterostructure to emulate the retinal function of simultaneously sensing and processing an image. By assembling nine vdW heterostructure devices into an array to process the visual information on the pixel level (Figure 4C). Photolithography was utilized to develop Ti/Au as the bottom electrode on the silicon wafer, and atomic layer deposition was used to grow Al2O3 on the bottom electrode. To manufacture vdW heterostructures, WSe2 and h-BN flakes were mechanically exfoliated and transported to the Al2O3 layer, and Pd/Au was deposited on the heterostructures as source and drain to fabricate van der Waals heterostructures based on WSe2/h-BN/Al2O3. They linked retinal morphometric sensors with large-scale memory crossbars to simulate picture recognition and object tracking. Zhang et al. (2021) constructed an optoelectronic synapse based on a large-scale wafer-scale (6 cm × 6 cm) GDY (Graphdiyne)/Gr (graphene) vertical heterostructure array device (Figure 4D). The synaptic functions such as EPSC, IPSC, SRDP, and PPF were realized, and the logic functions of “NAND” and “NOR” were demonstrated. Images were acquired, memorized and differentiated in real time using a 7 × 6 GDY/Gr panel array. When the letter “G" was input into the system, there was a significant difference in conductance between the array transistors with and without illumination, proving the real-time image acquisition and in-situ memory functions.
The future of transistor-based bionic perceptual visual perception technologies is bright. The majority of current researches are centered on mimicking a single human visual neuron. It is planned to develop a more complicated, high-integration, low-power, parallel-processing, high-efficiency visual system in the future. It has significant application potential in wearable gadgets, electronic eyes, robots, and assistive technologies for visually impaired people.
Tactile Perception
Skin, the largest sensory organ with a surface area of between 1.5 and 2.0 m2 in the human body, serves as a barrier between our internal and external environments, protects the former from diverse unfavorable factors of the latter, thus allowing us to maintain homeostasis (Romanovsky, 2014; Zimmerman et al., 2014; Aghaei et al., 2016; Soni and Dahiya, 2020). It houses a huge nerve network comprising a variety of sensory receptors that are responsible for detecting various internal and external disturbances, such as pressure, strain, vibration, temperature, pain, and chemical species, allowing humans to interact with the environment and perform daily tasks (Parvizi-Fard et al., 2021; Wang M. et al., 2021). The organ conveys sensory information to the brain through afferent neurons to form haptic memory, allowing humans to remember the impressions of the stimuli applied to the skin (Dahiya et al., 2010; Zhu et al., 2016). For the past few decades, tremendous efforts have been made in the development of tactile perception devices from innovation to structural design. Tee et al. (2015) proposed a tactile receptor system consisting of a voltage-controlled oscillator with skin mechanoreceptors, a sensitive resistive pressure sensor, and a channel rhodopsin engineered specifically to enable optical neuron stimulation above 100 Hz, which successfully mimicked the response of tactile receptors. It has great application potential in the fields of nerve repair, electronic skin, prosthesis, and so on. Sun et al. (2022) firstly proposed a full reflex arc with multiple sensory-modality coding that senses visual and tactile information. It processes the information using the photoelectric synapse unit, and then sends efferent orders to control the actions of artificial muscle.
Zang et al. (2017) demonstrated a dual organic transistor tactile element (DOT-TPE) that integrates synaptic OFETs and pressure sensors of OFETs (Figure 5A). The organic synaptic transistor was fabricated by growing Au on the substrate as the source-drain electrode, PDPP3T was spin-coated on the substrate as the active layer, chitosan was used as the dielectric material, and Al was deposited on the dielectric layer as the gate electrode. DOT-TPE can generate stress-triggered electrical signals and transduce and process the signals. The DOT-TPE prototype was constructed with floating gate OFET and OFET synapses as pressure sensing and processing elements, respectively (Figure 5B). Four frequency-increasing holding pressure bias cycles were applied to pixels 1 to 9 to simulate the tactile sensation of dynamic contact (Figure 5C). The first pressing action A1 corresponded to the EPSC gradually decreasing from pixel 1 to pixel 9, while the gain A4/A1 gradually increases, reflecting the dynamic filtering function of the system as shown in Figure 5D.
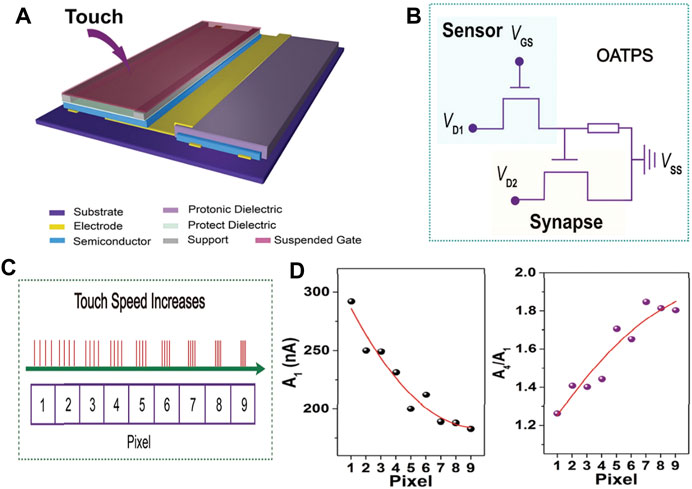
FIGURE5. (A) Schematic illustration of the DOT-TPS. (B) Equivalent electrical circuit of the DOT-TPS. (C) Four continuous and repeated touch cycles applied to the nine pixel. (D) The EPSC (A1) and the gain A4/A1 for each pixel (adapted from Zang et al., 2017).
The devices with tactile memory retain tactile information after the stimulus is removed, but they cannot distinguish tactile patterns, while the devices that have been proposed to distinguish tactile patterns lack the learning ability in recognition. Therefore, it is very important to have the human knowledge learning ability to recognize and distinguish patterns (Langley, 2011; Jordan and Mitchell, 2015). A tactile sensing device for imitating sensory neurons was demonstrated (Wan et al., 2018). The device was composed of a resistive pressure sensor, a soft ion cable, and a synaptic transistor, corresponding to the sensory receptors, axons, and synapses in the sensory neurons, respectively (Figure 6A). The resistance pressure sensor converted the pressure stimuli into an electrical signal, which was transmitted to the synapse via a soft ion cable. The device realized tactile pattern recognition. Two patterns in one row were used as the objects for recognition (Figure 6B), in which the convex pattern is defined as “1” and the flat pattern is defined as “0”. Each mode pair was marked with a binary coded code: “00”, “01”, “10”, “11”. In the experiment, the channel conductance of the transistor was used as the output. Only the mode “1” caused pressure changes. The “11” mode has two convex modes, so the maximum change in conductance is shown in Figure 6C. Figure 6D shows that the error rate decreased as the number of learnings increased, and the error rate of 6 learning was approximately 0.4%.
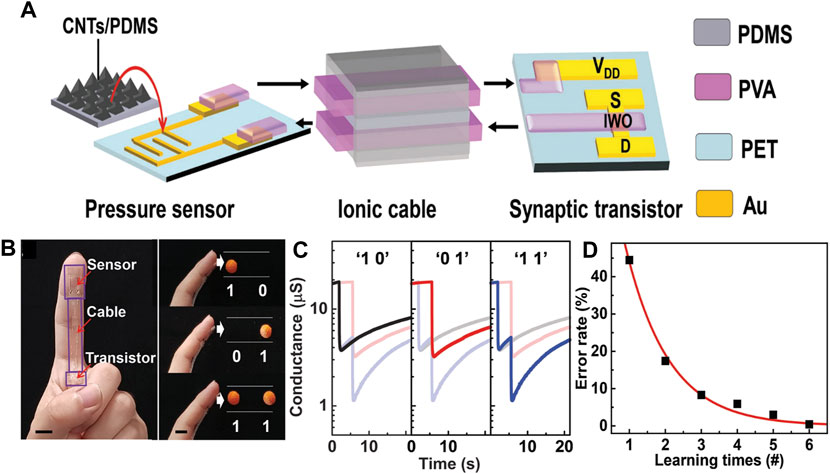
FIGURE 6. (A) Diagram illustrating the details of the NeuTap. (B) Schematic diagram of the digital image and pattern pair of NeuTap on the finger and the corresponding two-digit binary code label.(C) NeuTap’s response to three types of pattern pairs.(D) Recognition error rate plotted as a function of learning time (adapted from Wan et al., 2018).
Zhang et al. (2019) designed an artificial sensory neuron using nafion-based memristor as synapses and piezoresistive sensors as sensory receptors. The pyramid-structured sensor had good sensitivity (Figure 7A). Under a certain pressure, the contact between the top of the pyramid and the bottom electrode led to an increase in current. The jump in current required 22 ms (Figure 7B) for the pressure from 2 to 5 kPa, and the corresponding current increased from 2.9 to 4.9 mA (Figure 7C). Figure 7D shows that the device was connected to a finger joint to recognize the English letters: “L”, “A”, “B”, “S”, “F”, “N”. The overall recognition accuracy rate was 91.7%.
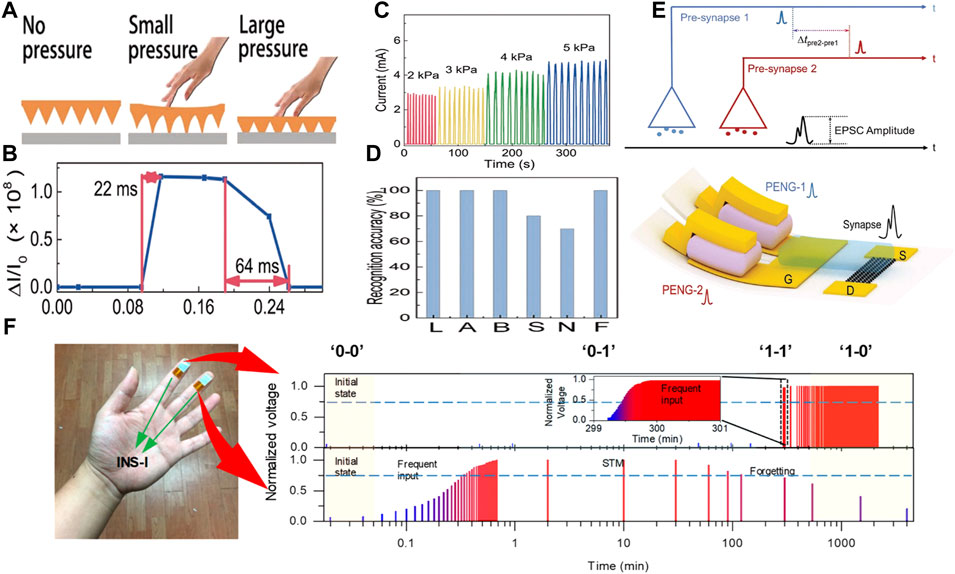
FIGURE 7. (A) Illustration of the sensor at zero, low, and high pressure. (B) Response and relaxation time of the sensor operating at 1v.(C) Current responses of pressure sensor under pressure pulses from 2 to 5 kPa. (D) Recognition accuracy of each character after 10 times training (adapted from Zhang et al., 2019). (E) Schematic diagram of the spatiotemporally correlated spike EPSC triggered by presynaptic 1 and presynaptic 2 and two (PENG-1 and PENG-2) inputs, one processing artificial neural network schematic (adapted from Chen et al., 2019). (F) The device is worn on the finger and records the relevant signal output (adapted from Wu et al., 2020).
Although various types of integrated artificial tactile perception systems have been realized, how to develop devices with a simple structure, low power consumption, and high performance is still a problem. Piezoelectric potentiometric sensing can replace traditional perception devices that require power by self-powering. Chen et al. (2019) proposed an integrated piezoelectric nanogenerator (PENG) for piezoelectric graphene artificial sensory synapses with iongel-gated transistors. Various synaptic behaviors, such as EPSC, IPSC, PPF, response to multiple strain spikes, and dynamic modulation behavior were simulated. Dynamic modulation based on bisensory presynaptic components was achieved (Figure 7E). This work may pave the way for self-powered artificial intelligence, bionic perceptual mechanosensory systems/interfaces, and neurorobotics. Wu et al. (2020) demonstrated a self-powered tactile sensor based on a well-designed single-electrode-based triboelectric nanogenerator (SE-TENG) (Figure 7F), which possesses learning ability and variable memory. This shows that the device can fully conform to the ability of the finger to act as a self-powered mechanical sensor with intelligence and touch, which is capable of stimulating a large amount of information related to history.
Wang D. et al. (2020) proposed a double-ended piezoresistive synaptic device with synapse and pressure-sensitive resistance based on ZnO/PVA heterostructure, which could simultaneously exhibit volatile resistance switching and pressure-adjustable synaptic behavior. The device had the functions of directly responding to pressure stimulation, temporary memory, and real-time processing of tactile information and sensory data. This improved the accuracy and efficiency of tactile information processing by increasing the pressure duration compared to traditional tactile sensors. It has great application prospects in electronic prosthetics, neurorobotics and bionic flexible electronics, etc., Chen et al. (2021) used triboelectric nanogenerator technology to realize an artificial slowly adapting type I tactile peripheral nervous system (SA-I TPNS), which improved the fidelity of tactile information. The resolvable dimension is 2 times smaller than the smallest inter-receiver distance. It breaks through the spatial resolution of tactile stimulus information, enabling human fingertips to identify fine textures. Parvizi-Fard et al. (2021) and his colleagues achieved the inclusion of SA-I/RA-I afferents and nociceptors, which enabled a high-resolution tactile sensor for the first time on an FPGA to implement the nociceptor concept. It opens a new window in the analysis of digital afferents and nociceptors from a neuroscience perspective, which holds promise for biorobotics and prosthetic applications.
Auditory Perception
The sound wave vibrates the eardrums with a specific frequency and amplitude. The mechanical vibrations are transmitted via ossicles to the cochlear hair cells and converted into electrical signals (Hudspeth, 2014). The amazingly complex structure of the cochlear system, can amplify the vibration signals up to several hundredfold, so that even the minutest sound is selectively recognized (Jung et al., 2019). The functional goal of the auditory system is to detect and extract wave information in the medium. Creatures can know what is happening in the environment through sound. Sound waves have spatiotemporal coding and additive properties, and the auditory system is more complicated to process than the visual system. The sound received by the ear has wave components of different frequencies. In order to isolate the different frequency components, the ear must have excellent sensitivity and frequency selectivity. In addition, sound changes over time, so it is also time-dependent. Therefore, while considering the different frequency characteristics of information carried by sound, the dependence of sound over time must also be considered (Ji et al., 2020). In addition to basic hearing, our auditory system also has higher-level perceptions such as tone recognition (Agus et al., 2012), pitch perception (Grahn, 2012), rhythm perception (Grahn, 2012) and sound localization (Grothe et al., 2010).
Most of the previous research has focused on the realization of basic hearing, so various materials and structures have been used to realize the cochlear implant. Lyon and Mead proposed an auditory sensor in 1988 that uses micro-power technology to simulate the human cochlea in CMOS VLSI. This is a low-power real-time model that uses a sub-threshold to simulate the cascade of the second-order filter part in the VLSI to achieve early auditory processing. Watts et al. (1992) further improved these devices in 1992 to solve the problems of dynamic range, stability, device mismatch, and compactness of the devices, so that their performance, robustness, and efficiency have been significantly improved. Sarpeshkar and Lyon (1998) described an electronic cochlea in 1998, which can process sound with an intensity of more than 6 orders of magnitude and dissipate 0.5 mW. These early studies laid the foundation for further research on the silicon cochlea. After the emergence of 3D printing, 3D printing technology, and artificial bionic auditory system were gradually used to construct artificial ears (Mannoor et al., 2013; Kong et al., 2016; Kuru et al., 2016; Liu et al., 2016). It accepts electromagnetic signals of a wide range of frequencies to enhance auditory perception, which expands opportunities for biomimetic tissues and organs. In order to absorb noise, the emergence of nanogenerators made of various materials and methods for energy harvesting is very important for the development of green technologies needed for portable wearable devices and energy harvesting (Wang et al., 2007a; Wang et al., 2007b). Triboelectric nanogenerators can be used for self-powered systems for recording and sound source localization. They have the characteristics of strong adaptability and low performance (Yang et al., 2014). Performance optimization of triboelectric nanogenerators to make them more suitable for humid and dusty environments (Gu et al., 2015). The design of flexible triboelectric nanogenerators lays the foundation for the further development of wearable electronic devices and cochlear implants (Fan et al., 2015). Triboelectric nanogenerators enable binary digital communication transmission (Yu et al., 2016). Ultrasoft, cuttable, and low-cost paper-based triboelectric nanogenerators can convert various mechanical energies in the environment into electricity. This is an advance in the development of green energy technology (Wu et al., 2018).
The human brain can quickly convert hearing sound information into electrical signals and can realize sound localization (Wang et al., 2018). Sound localization plays an important role in the biological nervous systems. Inspired by the human brain, a large number of researchers are working hard to develop morphological-based auditory perception devices. Sun et al. (2018) reported the artificial synaptic computation of STP-based monolayer MoS2 devices, achieving tunable synaptic plasticity through Joule heating of the device channels. Figures 8A,B show the current changes with and without heating, respectively, higher transmission post-excitation currents are shown in Figure 8B. The I-V curve hysteresis has a memory effect (Figure 8C). Two main sound localization mechanisms, interaural time difference (ITD) and interaural level difference (ILD), are shown in Figure 8D. This synaptic device encodes only transition segment information over a wide range of sound intensities and frequencies by suppressing ILD interference, That is, the interaural time difference is detected by suppressing sound intensity or frequency-dependent synaptic connections. Thus, sound localization is achieved (Figure 8E). In synaptic MoS2 devices, the resistive heating of MoS2 monolayer modulates the conductance of MoS2, and the characteristic time is controlled between seconds and minutes to realize the plastic function of synapses.
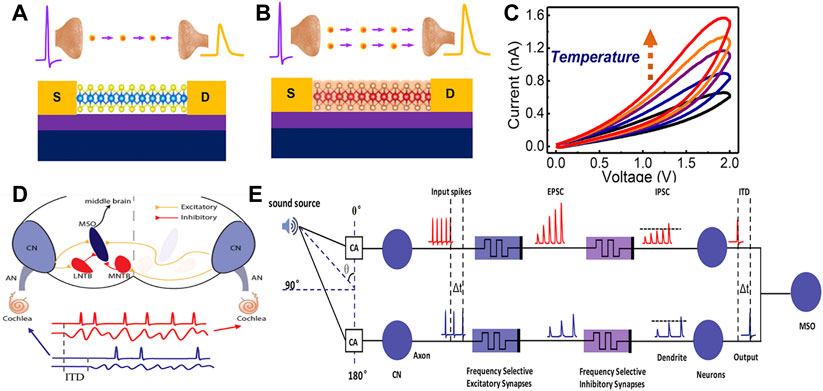
FIGURE 8. (A) Schematic diagram of two-terminal MoS2 device without Joule heating. (B) Schematic diagram of a Joule-heated MoS2 double-ended device. (C) Joule heating-driven conductance (G) Facilitation with multiple voltage sweeps. (D) Schematic picture for sound localization with both ITD and ILD. (E) Schematic picture of the working mechanism of synaptic computation for ITD-based sound localization (adapted from Sun et al., 2018).
Seo et al. (2019) used an ion-gel gated organic synaptic transistor (IGOST) with tunable synaptic properties based on a single semiconductor polymer to mimic the general synaptic behavior of the brain and peripheral nervous system. IGOST was prepared using polythiophene isoblue naphthalene (PTIIG-Np) film as the active layer and 1-ethyl-3-methylimidazolium bis (trifluoromethylsulfonyl) imide ([EMIM][TFSI]) is an ion-gel dielectric. Olythiophene isoindigonaphthalene thin films were spin-coated on Si/SiO2 (100 nm) substrates, and annealed at 80, 150, 200, 250, and 310°C for 10 min, respectively. Au source-drain electrodes were prepared by thermal evaporation, ion gel was drop-cast on the top of the channel region, and vacuum annealed at room temperature for 24 h. Artificial auditory synapses of triboelectric sensors and IGOST were demonstrated (Figure 9A). The triboelectric device was powered by a square acoustic wave at 5 Hz to IGOST, and the postsynaptic currents of IGOST were measured on membranes prepared at 80 and 310°C, respectively (Figure 9B). The membrane prepared at 80°C was suitable for simulating artificial sensory synapses.
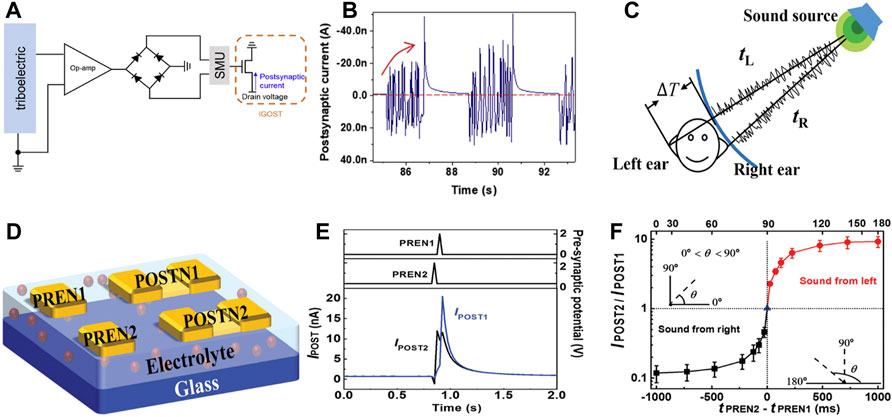
FIGURE 9. (A) Circuit diagram of the auditory nervous system. Triboelectric device detects sonic connection IGOST. An operational amplifier amplifies the voltage from the triboelectric device. The rectifier converts the bidirectional output signal to a unidirectional output. (B) Membranes prepared at 80°C measured postsynaptic currents of IGOST (adapted from Seo et al., 2019). (C) Schematic diagram of binaural effect localizing sound in the human brain. (D) Schematic diagram of the sound localization of the prepared artificial neural network. (E) Postsynaptic currents of POSTN1 and POSTN2 when sound comes in the correct direction. (F) Change of IPOST2/IPOST1 with time interval, sound azimuth (adapted from He et al., 2019).
Wang et al. (2018) proposed a transistor-based bionic perceptual method that uses resistive switch synapses to perform brain-like space-time calculations. In this work, the device was based on HfOx 1 transistor-1 resistance synapse designed to connect to a spike neural network. The positioning function of sound was simulated using this device. The network is similar to a biological neural network in that it can detect the precise time interval of the pulse time interval. He et al. (2019) proposed to build an artificial neural network that simulates the sound localization function of the human brain based on capacitively coupled multi-port oxide bionic perceptual transistors (Figure 9C). Sound localization is achieved by detecting the time difference between the two ears. There are two pairs of source/drain terminals as pren (PREN1 and PREN2) and postn (POSTN1 and POSTN2). PREN1 and PREN2 are left and right ear acoustic neurons, respectively, which are fully connected to POSTN1 and POSTN2 through the strong capacitive coupling effect of the proton-conducting electrolyte (Figure 9D). The connection strength between PREN1, PREN2, and the two POSTNs is in a diagonal relationship. It shows that the order of the two POSTN processing synaptic spikes is reversed. When the sound comes from different directions, the post-synaptic current amplitudes of POSTN1 and POSTN2 are different (Figures 9E,F) Therefore, sound localization can be achieved by detecting the time difference between the two ears.
Flexible biomimetic devices are extensively employed in current research for sound localization and acoustic information collecting processing. Furthermore, the achieved function is a single and preliminary function. This is a great far from the performance of an actual human neural system. To create transistor-based bionic perceptual auditory perception devices in the future, it will be important to develop new materials and device structure integration based on these foundations.
Olfactory Perception
The olfactory system plays an important role in reproductive and maternal function, neuroendocrine regulation, emotional responses, allograft aggression, predator and prey recognition, mate recognition, navigation, threat detection, and early disease diagnosis (Shipley and Ennis, 1996). The role of the olfactory system is to decode the complex eddies of molecules in the environment and shape them into pieces of relevant information that will allow the animal to make decisions and engage in adapted behaviors (Sandoz, 2011). The olfactory system of vertebrates and invertebrates consists of three main parts: the odor delivery system that transports odor molecules to sensory neurons; the large sensor array that converts chemical information into electrical signals; neural circuits that process sensory input to meet various analytical challenges (Raman et al., 2011). Since the first attempts to mimic the human nose with artificial devices, a variety of sensors have been developed. However, two issues remain to be addressed in order to create a device capable of mimicking the human nose: the complexity of olfactory coding and the extreme sensitivity of biological systems (Pelosi et al., 2018).
In 1961, artificial olfactory devices were used for the measurement and determination of odor (Moncrieff, 1961). A lot of work has been devoted to developing bionic perceptual devices with high sensitivity and high selectivity like biological systems. Three types of bionic perceptual olfactory systems, electronic nose, bioelectronic nose, and colorimetric nose, have been developed one after another (Giannoukos et al., 2016; Kida et al., 2018; Wang G. et al., 2020). The first bionic perceptual olfactory system was proposed in 1982, and the device could repeatedly distinguish between multiple odors (Persaud and Dodd, 1982). Since then, researchers have focused on system simplification and performance improvements. The electronic nose is not sensitive enough to distinguish an odor from the odor mixture. It cannot fundamentally imitate the biological smell because there are no olfactory receptors. The bioelectronic nose has olfactory receptor cells on its surface, which can well mimic the animal olfactory system (Zhang et al., 2018). Rakow and Suslick (2000) pioneered a metalloporphyrin-dye colorimetric nose in 2000, using intermolecular forces to achieve high-sensitivity discrimination (Li et al., 2017). Since the material is disposable, it will cause environmental pollution and waste of resources (Wang G. et al., 2020).
Guo et al. (2017) demonstrated the fabrication of silicon nanowire (SiNWs) arrays/TiO2/reduced graphene oxide (rGO) Schottky sensors in the dark. In the principal component analysis (PCA) plot, different explosive vapors can be distinguished and the approximate concentration of a certain explosive can be initially estimated (Figure 10A). Sensing array functionality can be implemented on a single sensor by simply changing the light intensity periodically (Figure 10B). The device has fairly high sensitivity, fast response, recovery characteristics, and good reproducibility when detecting gases. The SiNWs/ZnO/rGO Schottky sensor is very suitable for the detection of explosive vapors. Almost all electronic devices exposed to harmful gases are designed to simulate human olfactory receptors. Although traditional sensors can quickly respond and recover, they cannot fully remember the perception process like humans. Therefore, the application of bionics in analog devices is limited.
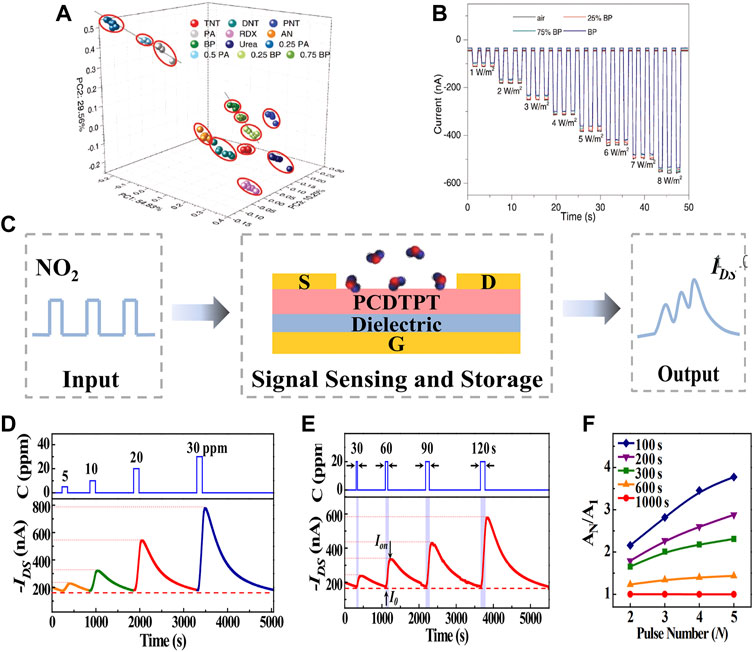
FIGURE 10. (A) Principal component analysis diagrams of 8 kinds of normal temperature saturated explosive vapors. (B) The time-dependent photo response of the Schottky sensor in different concentrations of BP gas was measured (adapted from Guo et al., 2017). (C) Schematic diagram of the operation model of the artificial organ injury memory system of a single PCDTPT OFET. (D) The effect of NO2 concentration from 5 to 30ppm on IDS. ∆ττ = 120s. (E) Influence of 20ppm NO2 pulse width from 30 to 120s on IDS. (F) Gain AN/A1 as a function of pulse number N at different pulse intervals (adapted from Song et al., 2019).
So Song et al. (2019) used an effective and simple method to construct a sensory memory system for detecting harmful gas leakage with only one organic transistor at room temperature. The device used poly [4-(4,4-dihexadecyl-4H-cyclopenta [1,2-b:5,4-b']dithiophen-2-yl)alt [1,2,5]thiadiazolo [3,4c]pyridine](PCDTPT) dissolved in chlorobenzene, and it was coated on a Si/SiO2 substrate. Au is grown by thermal evaporation as the source and drain electrodes to construct a bottom gate field effect transistor. The geometry of this simple device well simulates the inhalation, metabolism, and cumulative organ damage of noxious gases. NO2 was used as the input pulse, and the current was used as the output (Figure 10C). The longer the device was exposed to NO2 (Figure 10D), the higher the NO2 concentration (Figure 10E), the higher the exposure frequency (Figure 10F), the greater the current, the more obvious the pain, and the greater the harm. The flexible PCDTPT OFET biomimetic device was integrated. This device provides a great opportunity to simulate organ damage and wearable health equipment in the future.
Gao et al. (2021) developed a flexible artificial olfactory system integrating Sr-ZnO-based gas sensors, HfOx-based memristors, and electrochemical actuators (Figure 11A). HfOx-based memristor is not only a memory device but also a threshold controlling unit. The Sr-ZnO-based gas sensor serves as the basic unit of the gas-perception element, which is analogous to human odor receptors, generating a corresponding output voltage upon combining with gas molecules. The Sr-ZnO-based gas sensor has a single selectivity for NH3 and no response to other gases (Figure 11B). NH3 was transmitted to the memristor to switch the resistance state to store ammonia information (Figures 11C,D), which triggered the movement of the electrochemical actuator to block the airflow. A bionic nose was made as shown in Figure 11E to simulate the reaction of covering the nose after smelling ammonia gas. When the ammonia concentration is higher than the threshold, the memristor opens, triggering the actuator to bend inward and then close. This artificial olfactory system provides a strategy for future bionic electronics research.
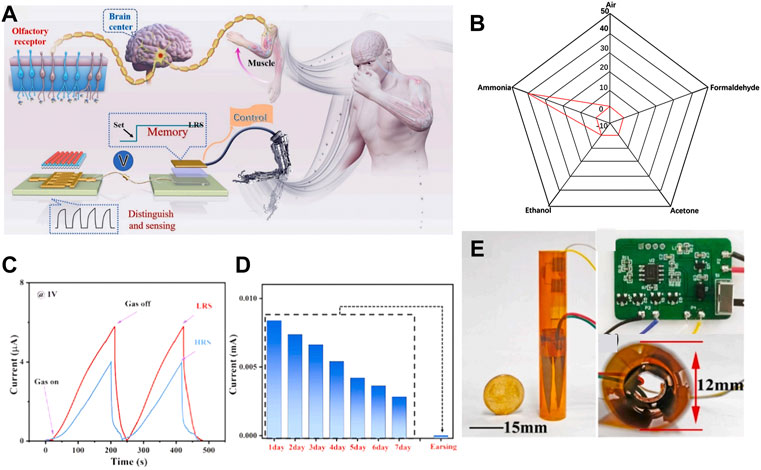
FIGURE 11. (A) Overall comparison of the biological and artificial olfactory system. (B)Selectivity of gas sensor to various testing gases at concentrations of 100 ppm. (C) Time-domain responses of the olfactory memory device to the HRS and LRS of 5000 ppm NH3. (D) Memory persistence of olfactory memory devices. (E) A photo of the bionic nose, a picture of its printed circuit board (PCB) and a picture of the main part and the diameter of the bionic nose (adapted from Gao et al., 2021).
According to current research, traditional bionic perceptual olfactory sensors struggle to distinguish and classify numerous gases (Karakaya et al., 2020). Spike coding and temporal information processing in a traditional artificial neural network makes it more like the human brain (Guo et al., 2019; Yao et al., 2020). But the system’s response time is short, the power consumption is large, and it is unstable (Zhang et al., 2020). Reservoir computing artificial perceptual neural networks with memory elements are booming and are considered attractive candidates for artificial synapses (Du et al., 2017). Wang T. et al. (2021) realized an artificial olfactory inference system consisting of a reserve computing (RC) system and a classifier, which consisted of a W/WO3/PEDOT: PSS/Pt volatile memory device. A classification of 10 different concentrations of ethanol, methane, ethylene, and carbon monoxide was achieved. The accuracy rate is as high as 95%.
Nociceptor Perception
Pain has been defined as a complex constellation of unpleasant sensory, emotional, and cognitive experiences caused by real or perceived tissue damage. It is manifested by certain autonomic, psychological, and behavioral reactions (Dubin and Patapoutian, 2010). Pain is caused only when the pressure and temperature are extreme enough to damage tissues, toxic molecules, and inflammatory mediators. It contrasts with the high sensitivity of vision, touch, hearing, smell, taste, and somatosensory organs to their uncomfortable stimuli. Therefore, the sensory neurons of the pain receptors protect the organism from danger by causing pain and driving avoidance (Price and Dubner, 1977). Pain receptors exist in different subgroups, which can respond to sound, light, heat, force, electricity, mechanical, chemical, or noxious stimuli (Pinho-Ribeiro et al., 2017).
Achieving pain-sensing-responsive behavior in electronic devices remains a central issue for researchers designing transistor-based bionic perceptual devices. Yoon et al. (2018) realized a nociceptor based on diffusion memristors with no adaptation, relaxation, and sensitization properties. The working principle of the device is shown in Figure 12A. The nerve endings receive noxious stimulation, and the pain receptors decide whether to send signals to the brain. The nociceptor is only excited when the values of electric pulses generated by noxious stimuli (e.g., heat and stabbing) exceed a certain threshold level. Once the nociceptor is triggered, pain information is received, integrated, and conveyed to the central nervous system, which exhibits long-lasting plastic changes. It can enable us to react appropriately and perceive precisely when facing unusual dangerous conditions in the real world. So, its essence is a threshold switching device. The electrical pulse applied to the device is higher than the threshold voltage of the memristor, the memristor enters a low resistance state, and the output terminal detects the current pulse, which is the process of pain. The electrical pulse is not strong enough, the memristor remains in a high resistance state, no current flows to the output, and external stimulation is harmless. A thermal nociceptor was constructed with this device (Figure 12B), and a large output signal was observed above 50°C (Figure 12C), which was triggered.
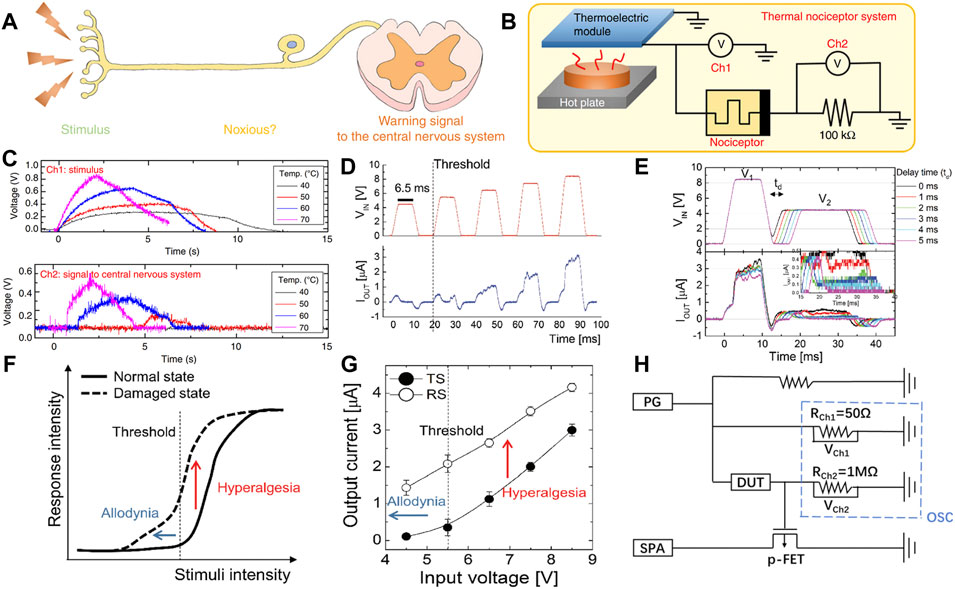
FIGURE 12. (A) Nerve endings receive noxious stimuli, and pain receptors decide whether to transmit the stimuli to the brain. (B) Schematic diagram of artificial thermal nociceptor circuit composed of thermoelectric module and diffusion memristor. (C) The voltage generated by the thermoelectric module (top) and the ON-switching and OFF-switching of the threshold switches monitored by the oscilloscope (bottom) (adapted from Yoon et al., 2018). (D) Ch1 (top panel) monitors the input voltage applied by the pulse generator, and Ch2 (bottom panel) monitors the output current. (E) The signal relaxation properties of the device are shown. The input voltage stimulus is shown above and the output current response is shown below. (F) Schematic comparison of nociceptor response signals in normal and impaired states. (G) Output currents as a function of the identical voltages at the threshold switching (TS) and resistance switching (RS) modes. (H) Simple circuit configuration to simulate reflection behavior (adapted from Kim et al., 2018).
A nociceptive device based on a Pt/HfO2/TiN (PHT) memristor structure was fabricated by Kim et al. (2018). Due to the electron capture/release of traps in the HfO2 layer, the energy level depth of the traps is about 0.7 eV. Four nociceptive behaviors of threshold (Figure 12D), relaxation (Figure 12E), ectopic pain (Figure 12F), and hyperalgesia (Figure Figure12G) are shown. A simple circuit was fabricated to simulate the reflection effect (Figure 12H), its working period time is short and its good relaxation time is conducive to bionic devices. Kumar et al. (2019a) demonstrated a highly transparent UV-triggered nociceptor based on ZnO/ATO/FTO heterostructure peroxidation. The use of threshold illumination to determine the output of the response enables nociceptive behavior under light stimulation. Five features of pain sensation could be achieved under the modulation of intensity, duration, and repetition rate of light input. It has been shown that the device is similar to human eye pain receptors (Figure 13A), replacing complex circuits with simple structures to achieve high integration.
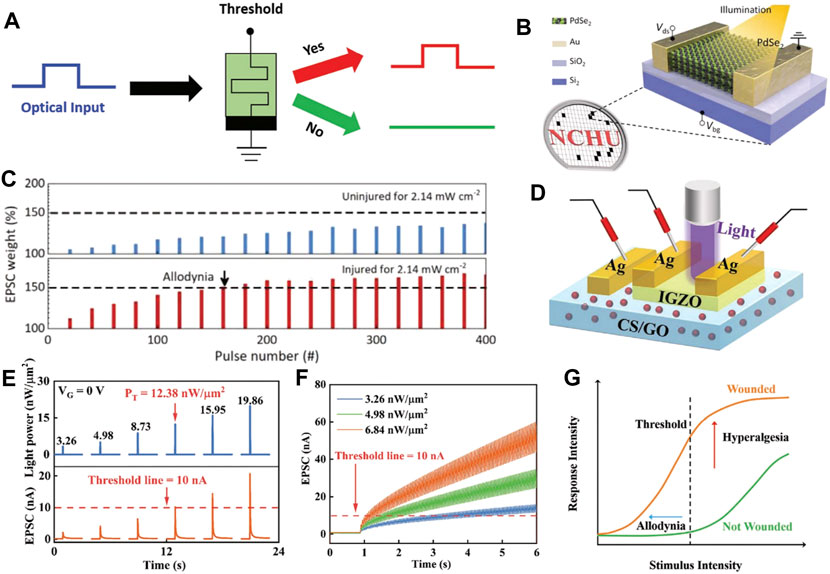
FIGURE 13. (A) Threshold-intensity-dependent photo response of the photodetector (adapted from Kumar et al., 2019a). (B) 3D schematic diagram of a transistor under laser irradiation. (C) To simulate the allodynia characteristic of sensitization function, EPSC weights for a series of innocuous stimuli were obtained in both uninjured and injured states (adapted from Li et al., 2021). (D) The structure illustration of the transistor. (E) The intensity-dependent threshold characteristic (F) Threshold characteristic and no-adaptation characteristic. (G) Schematic diagram of allodynia and hyperalgesia (adapted from Ke et al., 2021)
Researchers have made multiple attempts to increase the adaptability of pain receptors based on most mechanoreceptor prototypes, such as the usage of PdSe2 bipolar transistors with light-induced plasticity, in response to varied real-world dangers. Li et al. (2021) developed an artificial mode-regulable nociceptor (MR-nociceptor) based on the reversible photo-induced doping effect of bipolar palladium diselenide (PdSe2) Figure 13B is a schematic diagram of its transistor structure. The nociceptors represent the detected external environmental signals as light stimulus input, and the PCS weights of PdSe2 channels serve as system action potentials. The threshold warning level of injured nociceptors was lower than that of uninjured nociceptors (Figure 13C). The light-induced doping level determined by the frequency and intensity of light stimulation determines the adaptation rate and sensitization properties of nociceptors, which is beneficial to the ability to perceive and adapt to complex work environments.
Ke et al. (2021) fabricated a-IGZO-based optoelectronic bionic perceptual transistors on chitosan/graphene oxide (CS/GO) nanocomposite electrolyte membranes. The CS/GO solution was coated on the glass substrate to form a dielectric film, the a-IGZO channel was prepared on the composite film exfoliated from the glass substrate by magnetron sputtering, and the gate-source-drain electrodes were prepared by thermally evaporating Ag (Figure 13D). The functions of threshold, relaxation, no-adaptation, allodynia, and hyperalgesia of PCN were simulated. A single pulse below the threshold voltage fails to activate the transistor, and multiple such pulses in succession will push the EPSC beyond the threshold line (Figure 13E). As the optical power increased, the time to reach the threshold line became shorter and shorter (Figure 13F). The relaxation properties of PCN were simulated. After the noxious stimuli were removed, it slowly returned to a relaxed state. Before the previous stimuli completely disappeared, even a slight stimulation would produce more pain (Figure 13G). In addition to the above, allodynia and hyperalgesia were also simulated.
Nociceptors have achieved the basic properties of threshold, relaxation, ectopic pain, and hyperalgesia (Feng et al., 2020; Deng et al., 2021; Ke et al., 2021; Wei et al., 2021). Most studies have focused on mimicking the behavior of pain receptors using electrical and optical stimulation. Stable and high-performance nociceptors that are flexible and integrated with vision (Feng et al., 2021; Wei et al., 2021), touch, hearing, smell (Gao et al., 2021) and other functions are also being widely developed. This provides a basis for the research and development of smart wearable transistor-based bionic perceptual devices in the future.
Summary and Perspectives
In this review, a critical review has been presented on the current state-of-art in transistor-based bionic perceptual devices. Several aspects have been covered: vision, touch, hearing, smell, and pain. The device structures, characterization parameters, working mechanism, and the performance requirements of transistor-based bionic perceptual devices and systems are presented. Great progress and knowledge generation have been made in each of these domains, such as wearable electronics, artificial nervous systems, bionics, prosthetics, and robotics. Although transistor-based bionic perceptual systems based on synaptic devices have been implicated in a broad range of applications, the techniques are still at an early stage and far from practical applications. Transistor-based bionic Perceptual systems still have a long way to go before practical applications are possible. Several key problems and challenges related to transistor-based bionic perceptual systems remain to be addressed and are discussed below.
1) Perceptional synaptic devices can only simulate a small part of biological synaptic behaviors, and the functions that have been simulated remain at a simple level, such as only realizing one or two functions such as sensing light, pressure, sound location, and distinguishing certain gases. 2) Most previous studies have focused on basic synaptic functions and ignored performance indicators such as device size, read/write speed, power consumption, dynamic range, state hold time, switching noise, and endurance. 3) The human brain is comprehensive and powerful. It can take in information from the outside world and process multiple spatiotemporal information at the same time. It can learn from it, think about it, and react quickly. However, the limited understanding of brain functions and mechanisms by devices currently implemented has limited the development of “brain-like computing”. 4) The detection of subtle sensory information is still challenged by issues with regard to resolution, sensitivity, and selectivity.
In contrast to the two terminal neuromorphic devices, signal transmission and self-learning can be performed simultaneously in three-/multiterminal transistor-based artificial synapses. More importantly, the channel conductance of the neuromorphic transistor could be tuned by multiple gates through the electrolyte-based dielectric based on the interfacial EDL capacitive coupling or electrochemical processes. This provide a unique advantage for integrating spatiotemporal signals, which is very required for mimicking the spatiotemporal integration of sensory information. In the future, researchers will focus on mimicking brain function using neuromorphic transistors. To reproduce a bionic perceptual system, a diversity of stimuli-responsive materials/structures are needed for the detection of external information (touch, sight, sound, smell, taste, and PH), inner information (pain, hunger, and other homeostatic conditions), and action/reaction information (body position, movement, and acceleration) of an individual. In addition, synaptic devices should be designed and integrated to process sensory information with such nature-evolved merits as massive parallelism, ultralow energy consumption, and high connectivity. By combining with fields such as physics, chemistry, biology, materials engineering, computer science, and medicine, the field of bionic perceptual devices will achieve access to a wide range of applications. We believe it would be a great feat in the history of human development to realize a device as intelligent as human beings.
Author Contributions
QW and YH conceptualized the concept and wrote the manuscript. YZ, LZ, and XL participated in writing the manuscript. All authors contributed to the article and approved the submitted version.
Conflict of Interest
The authors declare that the research was conducted in the absence of any commercial or financial relationships that could be construed as a potential conflict of interest.
Publisher’s Note
All claims expressed in this article are solely those of the authors and do not necessarily represent those of their affiliated organizations, or those of the publisher, the editors and the reviewers. Any product that may be evaluated in this article, or claim that may be made by its manufacturer, is not guaranteed or endorsed by the publisher.
References
Aghaei, M., Aghaei, S., and Nilforoushzadeh, M. (2016). The Role of Peroxisome Proliferator-Activated Receptor-Coactivator-1 Gene in Skin Aging. J. Res. Med. Sci. 21, 36. doi:10.4103/1735-1995.183999
Agnus, G., Zhao, W., Derycke, V., Filoramo, A., Lhuillier, Y., Lenfant, S., et al. (2010). Two-Terminal Carbon Nanotube Programmable Devices for Adaptive Architectures. Adv. Mat. 22 (6), 702–706. doi:10.1002/adma.200902170
Agus, T. R., Suied, C., Thorpe, S. J., and Pressnitzer, D. (2012). Fast Recognition of Musical Sounds Based on Timbre. J. Acoust. Soc. Am. 131 (5), 4124–4133. doi:10.1121/1.3701865
Cai, J. C., Ren, Z. Y., Zhu, L. Q., Dai, Y. J., Zeng, Y. H., Guo, W., et al. (2021). Highly Sensitive Flexible Tactile Perceptual Interactive Platform with Functions of Braille Code Recognition. J. Phys. D. Appl. Phys. 54 (37), 375102. doi:10.1088/1361-6463/ac055b
Chen, L., Wen, C., Zhang, S.-L., Wang, Z. L., and Zhang, Z.-B. (2021). Artificial Tactile Peripheral Nervous System Supported by Self-Powered Transducers. Nano Energy 82, 105680. doi:10.1016/j.nanoen.2020.105680
Chen, S., Lou, Z., Chen, D., and Shen, G. (2018). An Artificial Flexible Visual Memory System Based on an UV-Motivated Memristor. Adv. Mat. 30 (7), 1705400. doi:10.1002/adma.201705400
Chen, Y., Gao, G., Zhao, J., Zhang, H., Yu, J., Yang, X., et al. (2019). Piezotronic Graphene Artificial Sensory Synapse. Adv. Funct. Mat. 29 (41), 1900959. doi:10.1002/adfm.201900959
Cho, S. W., Kwon, S. M., Kim, Y.-H., and Park, S. K. (2021). Recent Progress in Transistor-Based Optoelectronic Synapses: From Neuromorphic Computing to Artificial Sensory System. Adv. Intell. Syst. 3 (6), 2000162. doi:10.1002/aisy.202000162
Chua, L. (1971). Memristor-The Missing Circuit Element. IEEE Trans. Circuit Theory 18 (5), 507–519. doi:10.1109/tct.1971.1083337
Dahiya, R. S., Metta, G., Valle, M., and Sandini, G. (2010). Tactile Sensing-From Humans to Humanoids. IEEE Trans. Robot. 26 (1), 1–20. doi:10.1109/tro.2009.2033627
Dai, S., Zhao, Y., Wang, Y., Zhang, J., Fang, L., Jin, S., et al. (2019). Recent Advances in Transistor-Based Artificial Synapses. Adv. Funct. Mat. 29 (42), 1903700. doi:10.1002/adfm.201903700
Deng, X., Wang, S. Q., Liu, Y. X., Zhong, N., He, Y. H., Peng, H., et al. (2021). A Flexible Mott Synaptic Transistor for Nociceptor Simulation and Neuromorphic Computing. Adv. Funct. Mat. 31 (23), 2101099. doi:10.1002/adfm.202101099
Du, C., Cai, F., Zidan, M. A., Ma, W., Lee, S. H., and Lu, W. D. (2017). Reservoir Computing Using Dynamic Memristors for Temporal Information Processing. Nat. Commun. 8, 2204. doi:10.1038/s41467-017-02337-y
Dubin, A. E., and Patapoutian, A. (2010). Nociceptors: the Sensors of the Pain Pathway. J. Clin. Invest. 120 (11), 3760–3772. doi:10.1172/jci42843
Fan, X., Chen, J., Yang, J., Bai, P., Li, Z., and Wang, Z. L. (2015). Ultrathin, Rollable, Paper-Based Triboelectric Nanogenerator for Acoustic Energy Harvesting and Self-Powered Sound Recording. Acs Nano 9 (4), 4236–4243. doi:10.1021/acsnano.5b00618
Feng, G., Jiang, J., Li, Y., Xie, D., Tian, B., and Wan, Q. (2021). Flexible Vertical Photogating Transistor Network with an Ultrashort Channel for In-Sensor Visual Nociceptor. Adv. Funct. Mat. 31 (36), 2104327. doi:10.1002/adfm.202104327
Feng, G., Jiang, J., Zhao, Y., Wang, S., Liu, B., Yin, K., et al. (2020). A Sub-10 Nm Vertical Organic/Inorganic Hybrid Transistor for Pain-Perceptual and Sensitization-Regulated Nociceptor Emulation. Adv. Mat. 32 (6), 1906171. doi:10.1002/adma.201906171
Gao, S., Liu, G., Yang, H. L., Hu, C., Chen, Q. L., Gong, G. D., et al. (2019). An Oxide Schottky Junction Artificial Optoelectronic Synapse. ACS Nano 13 (2), 2634–2642. doi:10.1021/acsnano.9b00340
Gao, Z., Chen, S., Li, R., Lou, Z., Han, W., Jiang, K., et al. (2021). An Artificial Olfactory System with Sensing, Memory and Self-Protection Capabilities. Nano Energy 86, 106078. doi:10.1016/j.nanoen.2021.106078
Giannoukos, S., Brkić, B., Taylor, S., Marshall, A., and Verbeck, G. F. (2016). Chemical Sniffing Instrumentation for Security Applications. Chem. Rev. 116 (14), 8146–8172. doi:10.1021/acs.chemrev.6b00065
Gkoupidenis, P., Koutsouras, D. A., Lonjaret, T., Fairfield, J. A., and Malliaras, G. G. (2016). Orientation Selectivity in a Multi-Gated Organic Electrochemical Transistor. Sci. Rep. 6, 27007. doi:10.1038/srep27007
Grahn, J. A. (2012). Neural Mechanisms of Rhythm Perception: Current Findings and Future Perspectives. Top. Cogn. Sci. 4 (4), 585–606. doi:10.1111/j.1756-8765.2012.01213.x
Grothe, B., Pecka, M., and McAlpine, D. (2010). Mechanisms of Sound Localization in Mammals. Physiol. Rev. 90 (3), 983–1012. doi:10.1152/physrev.00026.2009
Gu, L., Cui, N., Liu, J., Zheng, Y., Bai, S., and Qin, Y. (2015). Packaged Triboelectric Nanogenerator with High Endurability for Severe Environments. Nanoscale 7 (43), 18049–18053. doi:10.1039/c5nr05514h
Guo, L., Yang, Z., and Dou, X. (2017). Artificial Olfactory System for Trace Identification of Explosive Vapors Realized by Optoelectronic Schottky Sensing. Adv. Mat. 29 (5), 1604528. doi:10.1002/adma.201604528
Guo, Y., Wu, H., Gao, B., and Qian, H. (2019). Unsupervised Learning on Resistive Memory Array Based Spiking Neural Networks. Front. Neurosci. 13, 812. doi:10.3389/fnins.2019.00812
Han, H., Yu, H., Wei, H., Gong, J., and Xu, W. (2019). Recent Progress in Three-Terminal Artificial Synapses: From Device to System. Small 15 (32), 1900695. doi:10.1002/smll.201900695
He, Y., Nie, S., Liu, R., Jiang, S., Shi, Y., and Wan, Q. (2019). Spatiotemporal Information Processing Emulated by Multiterminal Neuro-Transistor Networks. Adv. Mat. 31 (21), 1900903. doi:10.1002/adma.201900903
Ho, V. M., Lee, J.-A., and Martin, K. C. (2011). The Cell Biology of Synaptic Plasticity. Science 334 (6056), 623–628. doi:10.1126/science.1209236
Hu, M., Graves, C. E., Li, C., Li, Y., Ge, N., Montgomery, E., et al. (2018). Memristor-Based Analog Computation and Neural Network Classification with a Dot Product Engine. Adv. Mat. 30 (9), 1705914. doi:10.1002/adma.201705914
Hudspeth, A. J. (2014). Integrating the Active Process of Hair Cells with Cochlear Function. Nat. Rev. Neurosci. 15 (9), 600–614. doi:10.1038/nrn3786
Ji, X., Zhao, X., Tan, M. C., and Zhao, R. (2020). Artificial Perception Built on Memristive System: Visual, Auditory, and Tactile Sensations. Adv. Intell. Syst. 2 (3), 1900118. doi:10.1002/aisy.201900118
Jiang, J., Wan, Q., Sun, J., and Lu, A. (2009). Ultralow-voltage Transparent Electric-Double-Layer Thin-Film Transistors Processed at Room-Temperature. Appl. Phys. Lett. 95 (15), 152114. doi:10.1063/1.3251782
Jordan, M. I., and Mitchell, T. M. (2015). Machine Learning: Trends, Perspectives, and Prospects. Science 349 (6245), 255–260. doi:10.1126/science.aaa8415
Jung, Y. H., Park, B., Kim, J. U., and Kim, T. i. (2019). Bioinspired Electronics for Artificial Sensory Systems. Adv. Mat. 31 (34), 1803637. doi:10.1002/adma.201803637
Karakaya, D., Ulucan, O., and Turkan, M. (2020). Electronic Nose and its Applications: A Survey. Int. J. Autom. Comput. 17 (2), 179–209. doi:10.1007/s11633-019-1212-9
Ke, S., He, Y., Zhu, L., Jiang, Z., Mao, H., Zhu, Y., et al. (2021). Indium-Gallium-Zinc-Oxide Based Photoelectric Neuromorphic Transistors for Modulable Photoexcited Corneal Nociceptor Emulation. Adv. Electron. Mat. 7 (11), 2100487. doi:10.1002/aelm.202100487
Kida, H., Fukutani, Y., Mainland, J. D., de March, C. A., Vihani, A., Li, Y. R., et al. (2018). Vapor Detection and Discrimination with a Panel of Odorant Receptors. Nat. Commun. 9, 4556. doi:10.1038/s41467-018-06806-w
Kim, Y., Kwon, Y. J., Kwon, D. E., Yoon, K. J., Yoon, J. H., Yoo, S., et al. (2018). Nociceptive Memristor. Adv. Mat. 30 (8), 1704320. doi:10.1002/adma.201704320
Kong, Y. L., Gupta, M. K., Johnson, B. N., and McAlpine, M. C. (2016). 3D Printed Bionic Nanodevices. Nano Today 11 (3), 330–350. doi:10.1016/j.nantod.2016.04.007
Kumar, M., Kim, H. S., and Kim, J. (2019a). A Highly Transparent Artificial Photonic Nociceptor. Adv. Mat. 31 (19), 1900021. doi:10.1002/adma.201900021
Kumar, M., Som, T., and Kim, J. (2019b). A Transparent Photonic Artificial Visual Cortex. Adv. Mat. 31 (39), 1903095. doi:10.1002/adma.201903095
Kuru, I., Maier, H., Müller, M., Lenarz, T., and Lueth, T. C. (2016). A 3D-Printed Functioning Anatomical Human Middle Ear Model. Hear. Res. 340, 204–213. doi:10.1016/j.heares.2015.12.025
Kwon, S. M., Cho, S. W., Kim, M., Heo, J. S., Kim, Y. H., and Park, S. K. (2019). Environment-Adaptable Artificial Visual Perception Behaviors Using a Light-Adjustable Optoelectronic Neuromorphic Device Array. Adv. Mat. 31 (52), 1906433. doi:10.1002/adma.201906433
Langley, P. (2011). The Changing Science of Machine Learning. Mach. Learn. 82 (3), 275–279. doi:10.1007/s10994-011-5242-y
Lee, M., Lee, W., Choi, S., Jo, J.-W., Kim, J., Park, S. K., et al. (2017). Brain-Inspired Photonic Neuromorphic Devices Using Photodynamic Amorphous Oxide Semiconductors and Their Persistent Photoconductivity. Adv. Mat. 29 (28), 1700951. doi:10.1002/adma.201700951
Lee, Y., and Lee, T.-W. (2019). Organic Synapses for Neuromorphic Electronics: From Brain-Inspired Computing to Sensorimotor Nervetronics. Acc. Chem. Res. 52 (4), 964–974. doi:10.1021/acs.accounts.8b00553
Lei, P., Duan, H., Qin, L., Wei, X., Tao, R., Wang, Z., et al. (2022). High-Performance Memristor Based on 2D Layered BiOI Nanosheet for Low-Power Artificial Optoelectronic Synapses. Adv. Funct. Mater. 32, 2201276. doi:10.1002/adfm.202201276
Li, H. K., Chen, T. P., Liu, P., Hu, S. G., Liu, Y., Zhang, Q., et al. (2016). A Light-Stimulated Synaptic Transistor with Synaptic Plasticity and Memory Functions Based on InGaZnOx-Al2O3 Thin Film Structure. J. Appl. Phys. 119 (24), 244505. doi:10.1063/1.4955042
Li, M., Yang, F. S., Hsu, H. C., Chen, W. H., Kuo, C. N., Chen, J. Y., et al. (2021). Defect Engineering in Ambipolar Layered Materials for Mode-Regulable Nociceptor. Adv. Funct. Mat. 31 (5), 2007587. doi:10.1002/adfm.202007587
Li, Z., Fang, M., LaGasse, M. K., Askim, J. R., and Suslick, K. S. (2017). Colorimetric Recognition of Aldehydes and Ketones. Angew. Chem. Int. Ed. 56 (33), 9860–9863. doi:10.1002/anie.201705264
Liu, X.-P., Koehler, K. R., Mikosz, A. M., Hashino, E., and Holt, J. R. (2016). Functional Development of Mechanosensitive Hair Cells in Stem Cell-Derived Organoids Parallels Native Vestibular Hair Cells. Nat. Commun. 7, 11508. doi:10.1038/ncomms11508
Mannoor, M. S., Jiang, Z., James, T., Kong, Y. L., Malatesta, K. A., Soboyejo, W. O., et al. (2013). 3D Printed Bionic Ears. Nano Lett. 13 (6), 2634–2639. doi:10.1021/nl4007744
Merolla, P. A., Arthur, J. V., Alvarez-Icaza, R., Cassidy, A. S., Sawada, J., Akopyan, F., et al. (2014). A Million Spiking-Neuron Integrated Circuit with a Scalable Communication Network and Interface. Science 345 (6197), 668–673. doi:10.1126/science.1254642
Moncrieff, R. W. (1961). An Instrument for Measuring and Classifying Odors. J. Appl. Physiology 16 (4), 742–749. doi:10.1152/jappl.1961.16.4.742
Nishitani, Y., Kaneko, Y., Ueda, M., Morie, T., and Fujii, E. (2012). Three-terminal Ferroelectric Synapse Device with Concurrent Learning Function for Artificial Neural Networks. J. Appl. Phys. 111 (12), 124108. doi:10.1063/1.4729915
Park, H. L., Lee, Y., Kim, N., Seo, D. G., Go, G. T., and Lee, T. W. (2020). Flexible Neuromorphic Electronics for Computing, Soft Robotics, and Neuroprosthetics. Adv. Mat. 32 (15), 1903558. doi:10.1002/adma.201903558
Parvizi-Fard, A., Salimi-Nezhad, N., Amiri, M., Falotico, E., and Laschi, C. (2021). Sharpness Recognition Based on Synergy between Bio-Inspired Nociceptors and Tactile Mechanoreceptors. Sci. Rep. 11 (1), 2109. doi:10.1038/s41598-021-81199-3
Pelosi, P., Zhu, J., and Knoll, W. (2018). From Gas Sensors to Biomimetic Artificial Noses. Chemosensors 6 (3), 32. doi:10.3390/chemosensors6030032
Persaud, K., and Dodd, G. (1982). Analysis of Discrimination Mechanisms in the Mammalian Olfactory System Using a Model Nose. Nature 299 (5881), 352–355. doi:10.1038/299352a0
Pinho-Ribeiro, F. A., Verri, W. A., and Chiu, I. M. (2017). Nociceptor Sensory Neuron-Immune Interactions in Pain and Inflammation. Trends Immunol. 38 (1), 5–19. doi:10.1016/j.it.2016.10.001
Price, D. D., and Dubner, R. (1977). Mechanisms of First and Second Pain in the Peripheral and Central Nervous Systems. J. Investigative Dermatology 69 (1), 167–171. doi:10.1111/1523-1747.ep12497942
Qu, T. Y., Sun, Y., Chen, M. L., Liu, Z. B., Zhu, Q. B., Wang, B. W., et al. (2020). A Flexible Carbon Nanotube Sen-Memory Device. Adv. Mat. 32 (9), 1907288. doi:10.1002/adma.201907288
Quiroga, R. Q., Reddy, L., Kreiman, G., Koch, C., and Fried, I. (2005). Invariant Visual Representation by Single Neurons in the Human Brain. Nature 435 (7045), 1102–1107. doi:10.1038/nature03687
Rakow, N. A., and Suslick, K. S. (2000). A Colorimetric Sensor Array for Odour Visualization. Nature 406 (6797), 710–713. doi:10.1038/35021028
Raman, B., Stopfer, M., and Semancik, S. (2011). Mimicking Biological Design and Computing Principles in Artificial Olfaction. ACS Chem. Neurosci. 2 (9), 487–499. doi:10.1021/cn200027r
Romanovsky, A. A. (2014). Skin Temperature: its Role in Thermoregulation. Acta Physiol. 210 (3), 498–507. doi:10.1111/apha.12231
Sandoz, J. C. (2011). Behavioral and Neurophysiological Study of Olfactory Perception and Learning in Honeybees. Front. Syst. Neurosci. 5, 98. doi:10.3389/fnsys.2011.00098
Sarpeshkar, R., Lyon, R. F., and Mead, C. (1998). A Low-Power Wide-Dynamic-Range Analog VLSI Cochlea. Anal. Integr. Circuits Signal Process. 16 (3), 245–274. doi:10.1023/a:1008218308069
Seo, D.-G., Lee, Y., Go, G.-T., Pei, M., Jung, S., Jeong, Y. H., et al. (2019). Versatile Neuromorphic Electronics by Modulating Synaptic Decay of Single Organic Synaptic Transistor: From Artificial Neural Networks to Neuro-Prosthetics. Nano Energy 65, 104035. doi:10.1016/j.nanoen.2019.104035
Seo, S., Jo, S.-H., Kim, S., Shim, J., Oh, S., Kim, J.-H., et al. (2018). Artificial Optic-Neural Synapse for Colored and Color-Mixed Pattern Recognition. Nat. Commun. 9, 5106. doi:10.1038/s41467-018-07572-5
Shipley, M. T., and Ennis, M. (1996). Functional Organization of Olfactory System. J. Neurobiol. 30 (1), 123–176. doi:10.1002/(sici)1097-4695(199605)30:1<123::aid-neu11>3.0.co;2-n
Sligte, I. G., Vandenbroucke, A. R. E., Scholte, H. S., and Lamme, V. A. F. (2010). Detailed Sensory Memory, Sloppy Working Memory. Front. Psychol. 1, 175. doi:10.3389/fpsyg.2010.00175
Song, Z., Tong, Y., Zhao, X., Ren, H., Tang, Q., and Liu, Y. (2019). A Flexible Conformable Artificial Organ-Damage Memory System towards Hazardous Gas Leakage Based on a Single Organic Transistor. Mat. Horiz. 6 (4), 717–726. doi:10.1039/c8mh01577e
Soni, M., and Dahiya, R. (2020). Soft eSkin: Distributed Touch Sensing with Harmonized Energy and Computing. Phil. Trans. R. Soc. A 378 (2164), 20190156. doi:10.1098/rsta.2019.0156
Strukov, D. B., Snider, G. S., Stewart, D. R., and Williams, R. S. (2008). The Missing Memristor Found. Nature 453 (7191), 80–83. doi:10.1038/nature06932
Sun, F., Lu, Q., Feng, S., and Zhang, T. (2021). Flexible Artificial Sensory Systems Based on Neuromorphic Devices. Acs Nano 15 (3), 3875–3899. doi:10.1021/acsnano.0c10049
Sun, L., Du, Y., Yu, H., Wei, H., Xu, W., and Xu, W. (2022). An Artificial Reflex Arc that Perceives Afferent Visual and Tactile Information and Controls Efferent Muscular Actions. Research 2022, 1–11. doi:10.34133/2022/9851843
Sun, L., Zhang, Y., Hwang, G., Jiang, J., Kim, D., Eshete, Y. A., et al. (2018). Synaptic Computation Enabled by Joule Heating of Single-Layered Semiconductors for Sound Localization. Nano Lett. 18 (5), 3229–3234. doi:10.1021/acs.nanolett.8b00994
Taunyazov, T., Sng, W., Lim, B., Hian See, H., Kuan, J., Fatir Ansari, A., et al. (2020). “Event-Driven Visual-Tactile Sensing and Learning for Robots,” in Proceedings of Robotics: Science and Systems (RSS). Corvalis, OR; MIT Press. doi:10.15607/RSS.2020.XVI.020
Tee, B. C.-K., Chortos, A., Berndt, A., Nguyen, A. K., Tom, A., McGuire, A., et al. (2015). A Skin-Inspired Organic Digital Mechanoreceptor. Science 350 (6258), 313–316. doi:10.1126/science.aaa9306
Wan, C., Cai, P., Guo, X., Wang, M., Matsuhisa, N., Yang, L., et al. (2020a). An Artificial Sensory Neuron with Visual-Haptic Fusion. Nat. Commun. 11 (1), 4602. doi:10.1038/s41467-020-18375-y
Wan, C., Cai, P., Wang, M., Qian, Y., Huang, W., and Chen, X. (2020b). Artificial Sensory Memory. Adv. Mat. 32 (15), 1902434. doi:10.1002/adma.201902434
Wan, C., Chen, G., Fu, Y., Wang, M., Matsuhisa, N., Pan, S., et al. (2018). An Artificial Sensory Neuron with Tactile Perceptual Learning. Adv. Mat. 30 (30), 1801291. doi:10.1002/adma.201801291
Wang, D., Wang, L., Ran, W., Zhao, S., Yin, R., Yan, Y., et al. (2020). Threshold Switching Synaptic Device with Tactile Memory Function. Nano Energy 76, 105109. doi:10.1016/j.nanoen.2020.105109
Wang, G., Li, Y., Cai, Z., and Dou, X. (2020). A Colorimetric Artificial Olfactory System for Airborne Improvised Explosive Identification. Adv. Mat. 32 (14), 1907043. doi:10.1002/adma.201907043
Wang, M., Luo, Y., Wang, T., Wan, C., Pan, L., Pan, S., et al. (2021). Artificial Skin Perception. Adv. Mat. 33 (19), 2003014. doi:10.1002/adma.202003014
Wang, S., Wang, C.-Y., Wang, P., Wang, C., Li, Z.-A., Pan, C., et al. (2021). Networking Retinomorphic Sensor with Memristive Crossbar for Brain-Inspired Visual Perception. Natl. Sci. Rev. 8 (2), nwaa172. doi:10.1093/nsr/nwaa172
Wang, T., Huang, H. M., Wang, X. X., and Guo, X. (2021). An Artificial Olfactory Inference System Based on Memristive Devices. InfoMat 3 (7), 804–813. doi:10.1002/inf2.12196
Wang, W., Pedretti, G., Milo, V., Carboni, R., Calderoni, A., Ramaswamy, N., et al. (2018). Learning of Spatiotemporal Patterns in a Spiking Neural Network with Resistive Switching Synapses. Sci. Adv. 4 (9), eaat4752. doi:10.1126/sciadv.aat4752
Wang, X., Liu, J., Song, J., and Wang, Z. L. (2007a). Integrated Nanogenerators in Biofluid. Nano Lett. 7 (8), 2475–2479. doi:10.1021/nl0712567
Wang, X., Song, J., Liu, J., and Wang, Z. L. (2007b). Direct-current Nanogenerator Driven by Ultrasonic Waves. Science 316 (5821), 102–105. doi:10.1126/science.1139366
Watts, L., Kerns, D. A., Lyon, R. F., and Mead, C. A. (1992). Improved Implementation of the Silicon Cochlea. IEEE J. Solid-State Circuits 27 (5), 692–700. doi:10.1109/4.133156
Wei, H., Ni, Y., Sun, L., Yu, H., Gong, J., Du, Y., et al. (2021). Flexible Electro-Optical Neuromorphic Transistors with Tunable Synaptic Plasticity and Nociceptive Behavior. Nano Energy 81, 105648. doi:10.1016/j.nanoen.2020.105648
Wu, C., Kim, T. W., Park, J. H., Koo, B., Sung, S., Shao, J., et al. (2020). Self-Powered Tactile Sensor with Learning and Memory. Acs Nano 14 (2), 1390–1398. doi:10.1021/acsnano.9b07165
Wu, C., Kima, T. W., Sung, S., Park, J. H., and Li, F. (2018). Ultrasoft and Cuttable Paper-Based Triboelectric Nanogenerators for Mechanical Energy Harvesting. Nano Energy 44, 279–287. doi:10.1016/j.nanoen.2017.11.080
Xia, Q., and Yang, J. J. (2019). Memristive Crossbar Arrays for Brain-Inspired Computing. Nat. Mat. 18 (4), 309–323. doi:10.1038/s41563-019-0291-x
Xie, D., Jiang, J., Hu, W., He, Y., Yang, J., He, J., et al. (2018). Coplanar Multigate MoS2 Electric-Double-Layer Transistors for Neuromorphic Visual Recognition. ACS Appl. Mat. Interfaces 10 (31), 25943–25948. doi:10.1021/acsami.8b07234
Xu, W., Cho, H., Kim, Y.-H., Kim, Y.-T., Wolf, C., Park, C.-G., et al. (2016). Organometal Halide Perovskite Artificial Synapses. Adv. Mat. 28 (28), 5916–5922. doi:10.1002/adma.201506363
Yang, J., Chen, J., Liu, Y., Yang, W., Su, Y., and Wang, Z. L. (2014). Triboelectrification-Based Organic Film Nanogenerator for Acoustic Energy Harvesting and Self-Powered Active Acoustic Sensing. ACS Nano 8 (3), 2649–2657. doi:10.1021/nn4063616
Yao, P., Wu, H., Gao, B., Tang, J., Zhang, Q., Zhang, W., et al. (2020). Fully Hardware-Implemented Memristor Convolutional Neural Network. Nature 577 (7792), 641–646. doi:10.1038/s41586-020-1942-4
Yoon, J. H., Wang, Z., Kim, K. M., Wu, H., Ravichandran, V., Xia, Q., et al. (2018). An Artificial Nociceptor Based on a Diffusive Memristor. Nat. Commun. 9, 417. doi:10.1038/s41467-017-02572-3
Yu, A., Chen, X., Wang, R., Liu, J., Luo, J., Chen, L., et al. (2016). Triboelectric Nanogenerator as a Self-Powered Communication Unit for Processing and Transmitting Information. ACS Nano 10 (4), 3944–3950. doi:10.1021/acsnano.5b07407
Yu, H., Wei, H., Gong, J., Han, H., Ma, M., Wang, Y., et al. (2021). Evolution of Bio-Inspired Artificial Synapses: Materials, Structures, and Mechanisms. Small 17 (9), 2000041. doi:10.1002/smll.202000041
Zang, Y., Shen, H., Huang, D., Di, C.-A., and Zhu, D. (2017). A Dual-Organic-Transistor-Based Tactile-Perception System with Signal-Processing Functionality. Adv. Mat. 29 (18), 1606088. doi:10.1002/adma.201606088
Zeng, M., He, Y., Zhang, C., and Wan, Q. (2021). Neuromorphic Devices for Bionic Sensing and Perception. Front. Neurosci. 15. doi:10.3389/fnins.2021.690950
Zhang, C., Ye, W. B., Zhou, K., Chen, H. Y., Yang, J. Q., Ding, G., et al. (2019). Bioinspired Artificial Sensory Nerve Based on Nafion Memristor. Adv. Funct. Mat. 29 (20), 1808783. doi:10.1002/adfm.201808783
Zhang, X., Cheng, J., Wu, L., Mei, Y., Jaffrezic-Renault, N., and Guo, Z. (2018). An Overview of an Artificial Nose System. Talanta 184, 93–102. doi:10.1016/j.talanta.2018.02.113
Zhang, X., Zhuo, Y., Luo, Q., Wu, Z., Midya, R., Wang, Z., et al. (2020). An Artificial Spiking Afferent Nerve Based on Mott Memristors for Neurorobotics. Nat. Commun. 11 (1), 51. doi:10.1038/s41467-019-13827-6
Zhang, Z.-C., Li, Y., Wang, J.-J., Qi, D.-H., Yao, B.-W., Yu, M.-X., et al. (2021). Synthesis of Wafer-Scale Graphdiyne/graphene Heterostructure for Scalable Neuromorphic Computing and Artificial Visual Systems. Nano Res. 14 (12), 4591–4600. doi:10.1007/s12274-021-3381-4
Zhou, J., Wan, C., Zhu, L., Shi, Y., and Wan, Q. (2013). Synaptic Behaviors Mimicked in Flexible Oxide-Based Transistors on Plastic Substrates. IEEE Electron Device Lett. 34 (11), 1433–1435. doi:10.1109/led.2013.2280663
Zhu, B., Wang, H., Liu, Y., Qi, D., Liu, Z., Wang, H., et al. (2016). Skin-Inspired Haptic Memory Arrays with an Electrically Reconfigurable Architecture. Adv. Mat. 28 (8), 1559–1566. doi:10.1002/adma.201504754
Zhu, J., Zhang, T., Yang, Y., and Huang, R. (2020). A Comprehensive Review on Emerging Artificial Neuromorphic Devices. Appl. Phys. Rev. 7 (1), 011312. doi:10.1063/1.5118217
Zhu, Y., Zhu, Y., Mao, H., He, Y., Jiang, S., Zhu, L., et al. (2022). Recent Advances in Emerging Neuromorphic Computing and Perception Devices. J. Phys. D. Appl. Phys. 55 (5), 053002. doi:10.1088/1361-6463/ac2868
Zidan, M. A., Strachan, J. P., and Lu, W. D. (2018). The Future of Electronics Based on Memristive Systems. Nat. Electron. 1 (1), 22–29. doi:10.1038/s41928-017-0006-8
Keywords: transistor-based bionic perceptual devices, visual perception, tactile perception, auditory perception, olfactory perception, nociceptor perception
Citation: Yu H, Zhu Y, Zhu L, Lin X and Wan Q (2022) Recent Advances in Transistor-Based Bionic Perceptual Devices for Artificial Sensory Systems. Front. Nanotechnol. 4:954165. doi: 10.3389/fnano.2022.954165
Received: 27 May 2022; Accepted: 21 June 2022;
Published: 11 July 2022.
Edited by:
Zhongrui Wang, The University of Hong Kong, Hong Kong SAR, ChinaCopyright © 2022 Yu, Zhu, Zhu, Lin and Wan. This is an open-access article distributed under the terms of the Creative Commons Attribution License (CC BY). The use, distribution or reproduction in other forums is permitted, provided the original author(s) and the copyright owner(s) are credited and that the original publication in this journal is cited, in accordance with accepted academic practice. No use, distribution or reproduction is permitted which does not comply with these terms.
*Correspondence: Qing Wan, d2FucWluZ0BuanUuZWR1LmNu