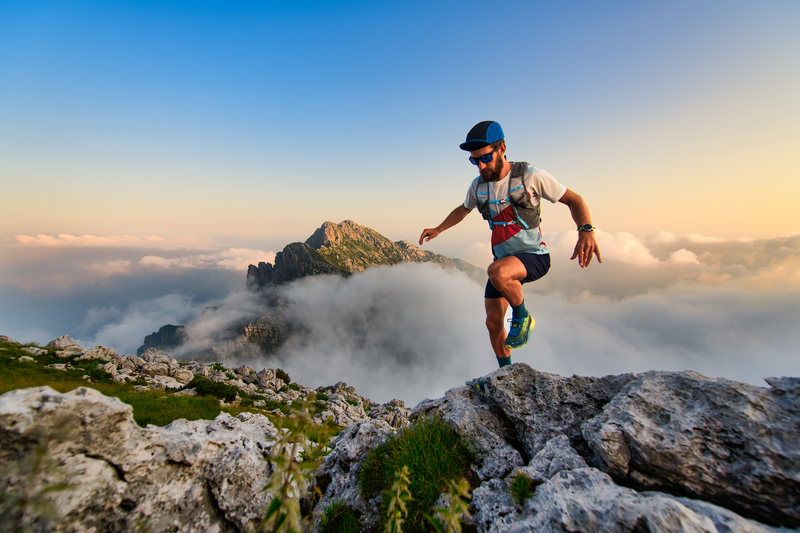
94% of researchers rate our articles as excellent or good
Learn more about the work of our research integrity team to safeguard the quality of each article we publish.
Find out more
EDITORIAL article
Front. Nanotechnol. , 21 November 2022
Sec. Nanodevices
Volume 4 - 2022 | https://doi.org/10.3389/fnano.2022.1073863
This article is part of the Research Topic In-memory Sensing and Computing: New Materials and Devices meet New Challenges View all 5 articles
Editorial on the Research Topic
In-memory sensing and computing: New materials and devices meet new challenges
With the convergence of Artificial Intelligence (AI) and Internet of Things (IoT) redefining the way industries, business, and economies function, the demand for energy-efficient and high-performance computing at the edge is exponentially increasing. Inspired by the low power and parallel processing capabilities of the biological brain, Neuromorphic Computing is an emerging computing paradigm that overcomes many limitations of the conventional computer architecture. Most importantly, by performing computations in-memory, Neuromorphic Computing overcomes the von Neuman bottleneck, thus improving the computational capability along with additional area and power savings. While several stand-alone neuromorphic chips have been developed with excellent energy efficiency for running specific AI algorithms, such digital systems still suffer when interfaced with edge sensors. This is because the sensory inputs are nonstructural, non-normalized, and fragmented, which incur large energy, time and wiring overheads on digital systems with separated sensing and processing units. This calls for in-memory sensing technologies, with fused sensing, memory, and processing capabilities, to unleash the full potential of highly sophisticated sensor and actuator systems used in bioelectronics and robotics.
Despite its infancy, the concepts of in-memory sensing and computing has already made significant inroads in specialized areas like e-skin and bionic eye. However, these are majorly software implementations and the hardware challenges to complement these have not been addressed yet. To take full advantage of the bioinspired edge processing capabilities, there are still fundamental challenges at the hardware level (materials and devices) that need to be addressed. Therefore, “In-memory Sensing and Computing: New Materials and Devices meet New Challenges” was launched last year, initiating the discussions on the recent developments as well as perspectives. Researchers from multidisciplinary backgrounds, like microelectronics, materials, and computer science, and different regions have posted their opinions and/or original works pertinent to this Research Topic, providing a comprehensive overview of the state-of-the-art and inspiring future development.
In “In-Memory Computation Based Mapping of Keccak-f Hash Function,” Kingra et al. from the Indian Institute of Technology Delhi propose a mapping strategy for implementing a hardware-based secure hash algorithm for addressing in-memory cybersecurity challenges. In this work, nonvolatile memory (NVM) is used to map the state, functioning as logic-in-memory (LIM) units. Four emerging NVM devices, including OxRAM, CBRAM, PCM, and FeRAM, are simulated for the implementation of Keccak-f. The proposed strategy shows 300 folds improvement in energy delay product (EDP) than conventional software-based strategies, which verifies the advantages of hardware-based secure hash algorithms and reveals the great potential of NVM for cryptographic hash applications.
In “Flexible and stretchable memristive arrays for in-memory computing,” Liu et al. from Fudan University (China) have summarized the recent advances on in-memory computing and shared their perspective, highlighting emerging flexible and/or stretchable systems. Insights into the development of flexible and stretchable memristive arrays for in-memory computing are provided by summarizing the recent progress at the material and device levels. Materials-wise, inorganic, organic and two-dimensional (2D) material systems are systematically surveyed, and the strategies for fabricating stretchable memristive arrays are also reviewed.
In “Emerging memristive devices for brain-inspired computing and perception,” Wang et al. from Nanjing University (China) reviews the recent developments of two-terminal memristive devices in terms of neuromorphic computing and perception applications. In “Recent Advances in Transistor-based Bionic Perceptual Devices for Artificial Sensory Systems,” Yu et al. from Nanjing University (China) covers the latest progress of transistor-based bionic perceptual devices for artificial sensory systems. These two device technologies are widely regarded as the most promising contenders for the next generation of artificial synapses, thanks to their analog memory capability and the ability to fuse sensing, memory and processing capabilities like biological systems. Both reviews have provided comprehensive literature surveys on material and device configurations for building artificial sensory neurons which is considered to be the building block for the NPS. In general, the two-terminal memristors feature a smaller footprint in comparison with transistor-type devices and can potentially achieve higher memory densities. On the other hand, the transistor-type devices have the advantage of parallel sensory processing, thanks to their multi-terminal configuration. In short, the hardware implementation of such technologies is a new area of research that has potential applications in interdisciplinary areas such as bio-integrated electronics, human machine interfaces and robotics.
The aforementioned research works are just examples of the recent advances in bio-plausible in-sensor/memory systems that can parallel the efficiency and compactness of their biological counterparts. We hope this Research Topic of research articles will foster the interaction of researchers from different disciplines including but not limited to, material science, circuit design, algorithms, and neuroscience. Further device and system engineering of in-sensor/memory systems may eventually lead to a breakthrough in neuromorphic electronics in the coming decade, bringing transformative impact on the way we compute.
CW, ZW, and RJ wrote the editorial.
The authors declare that the research was conducted in the absence of any commercial or financial relationships that could be construed as a potential conflict of interest.
All claims expressed in this article are solely those of the authors and do not necessarily represent those of their affiliated organizations, or those of the publisher, the editors and the reviewers. Any product that may be evaluated in this article, or claim that may be made by its manufacturer, is not guaranteed or endorsed by the publisher.
Keywords: neuromorphic, memristor, memtransistor, computing, security
Citation: Wan C, Wang Z and John RA (2022) Editorial: In-memory sensing and computing: New materials and devices meet new challenges. Front. Nanotechnol. 4:1073863. doi: 10.3389/fnano.2022.1073863
Received: 19 October 2022; Accepted: 11 November 2022;
Published: 21 November 2022.
Edited and reviewed by:
Dimitra G. Georgiadou, University of Southampton, United KingdomCopyright © 2022 Wan, Wang and John. This is an open-access article distributed under the terms of the Creative Commons Attribution License (CC BY). The use, distribution or reproduction in other forums is permitted, provided the original author(s) and the copyright owner(s) are credited and that the original publication in this journal is cited, in accordance with accepted academic practice. No use, distribution or reproduction is permitted which does not comply with these terms.
*Correspondence: Changjin Wan, Y2p3YW5Abmp1LmVkdS5jbg==; Zhongrui Wang, enJ3YW5nQGVlZS5oa3UuaGs=; Rohit Abraham John, cm9oam9obkBldGh6LmNo
Disclaimer: All claims expressed in this article are solely those of the authors and do not necessarily represent those of their affiliated organizations, or those of the publisher, the editors and the reviewers. Any product that may be evaluated in this article or claim that may be made by its manufacturer is not guaranteed or endorsed by the publisher.
Research integrity at Frontiers
Learn more about the work of our research integrity team to safeguard the quality of each article we publish.