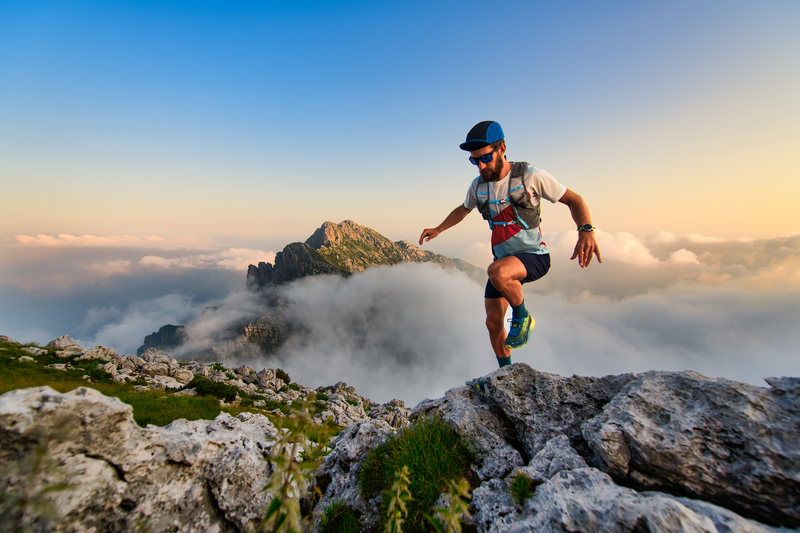
94% of researchers rate our articles as excellent or good
Learn more about the work of our research integrity team to safeguard the quality of each article we publish.
Find out more
REVIEW article
Front. Mol. Neurosci. , 29 August 2024
Sec. Molecular Signalling and Pathways
Volume 17 - 2024 | https://doi.org/10.3389/fnmol.2024.1429316
This article is part of the Research Topic Mechanisms of Cholinergic Transmission in Motivation and Cognition View all 6 articles
Attention and reward are functions that are critical for the control of behavior, and massive multi-region neural systems have evolved to support the discrete computations associated with each. Previous research has also identified that attention and reward interact, though our understanding of the neural mechanisms that mediate this interplay is incomplete. Here, we review the basic neuroanatomy of attention, reward, and cholinergic systems. We then examine specific contexts in which attention and reward computations interact. Building on this work, we propose two discrete neural circuits whereby acetylcholine, released from cell groups located in different parts of the brain, mediates the impact of stimulus-reward associations as well as motivation on attentional control. We conclude by examining these circuits as a potential shared loci of dysfunction across diseases states associated with deficits in attention and reward.
Our brains are faced with an enormously difficult task; to steer us through a dynamic environment imbued with possibility. To guide behavior effectively, brains have evolved circuitries to determine what information is allowed to dominate ongoing cognitive processing (attention) as well as the attractiveness and value of internal states and external stimuli (reward). Functionally, attention and reward are inextricably linked, and while many studies have explored the brain circuits that underlie attention and reward independently, relatively less is known regarding the systems that stand at the interface between these functions. Given their capacity to modulate activity across wide expanses of brain circuits known to mediate attention and reward processing, ascending neuromodulator systems (e.g., those responsible for producing the neurotransmitters dopamine, serotonin, norepinephrine, and acetylcholine) are uniquely situated to mediate this interaction. Here, we bring together anatomical, physiological, and behavioral evidence to propose that central cholinergic systems are particularly important for controlling these interactions between attention and reward. We first review evidence concerning the circuit-level organization of brain reward and attention systems, and current hypotheses of physiological and behavioral functions specifically controlled by forebrain cholinergic signaling. We then build upon previous work to propose a model whereby cholinergic systems mediate the influence of central representations of stimulus-reward associations and motivation on attentional control. Given that many neuropsychiatric and neurodegenerative disorders are associated with impairments in attention and reward processing, we conclude by exploring this perspective in the context of the pathophysiology of behavioral and cognitive impairments common to both neuropsychiatric and neurodegenerative diseases.
The construct of attention has long been a focus of both psychological and neuroscientific research. The pioneering psychologist William James famously said “Everyone knows what attention is. It is the taking possession by the mind, in clear and vivid form, of one out of what seem several simultaneously possible objects or trains of thought. Focalization, concentration, of consciousness are of its essence. It implies withdrawal from some things to deal effectively with others and is a condition which has a real opposite in the confused, dazed, scatterbrained state…” (James, 1890). As described by James, ‘attention’ is fundamentally a blanket term, used to apply to several complimentary operations that collectively organize perception and behavior. Early work by Broadbent (1954) envisioned attention as a filter; a collection of cognitive processes that enabled an individual to select certain stimuli from the environment and grant privileged access to associative learning and memory systems. Work by Posner and colleagues (Posner and Boies, 1971) created a more formalized framework for deployment of attention which included maintenance of general alertness and anticipatory monitoring of an environment for the appearance of instructive stimuli over time, as well as stimulus detection, which in Posner’s terms was an explicitly cognitive process that selects a stimulus from the environment to guide behavior. This conceptualization of attention assumes that its efficacy is bounded by the central processing capacity of a given individual (i.e., multitasking; Posner and Boies, 1971), and thus individual variation therein could seed both perceptual and cognitive abnormalities. Further refinements of this theory more formally operationalized the three main components of attention described above; alertness, orienting, and stimulus detection (Posner and Petersen, 1990). Alertness refers to a global and fundamentally neurobiological state that facilitates the relay of an input to primary sensory cortices and the decoding of its physical properties. Orienting refers to the process of either covertly or overtly biasing sensory processing to the predicted source of an instructive stimulus. Detection is the vehicle for conscious awareness of that stimulus and includes activation of learned associations regarding the predictive value of that stimulus that in turn guide behavior. Theories of attention have also long attempted to reconcile the multiple ways in which the construct can be activated or “recruited.” Voluntary or “top-down” attention refers to situations in which an individual’s goals and previous experience are used to guide attentional selection. In contrast, reflexive or “bottom-up” refers to scenarios in which the properties of a sensory stimulus (e.g., brightness, volume, and surprise) capture attentional resources (Posner et al., 1980; Jonides and Irwin, 1981; Desimone and Duncan, 1995; Corbetta and Shulman, 2002). This top-down/bottom-up framework has been incredibly influential and seeded the discovery of dedicated brain circuits that are differentially engaged in these contexts (Corbetta and Shulman, 2002). However, more recent work has highlighted situations in which this dichotomy falls apart, particularly in contexts where reward-associated stimuli are presented in tandem (Awh et al., 2012). Finally, a key component of all theories of attention is the capacity to focus on instructive stimuli while willfully ignoring task-irrelevant (distracting) sensory inputs (e.g., reading this manuscript while someone nearby is having a conversation), commonly referred to as “distracter filtering”. Current models suggest that both stimulus properties and cognitive demand can influence susceptibility to distraction. Specifically, when presented with complex visual and auditory stimuli subjects are less susceptible to the influence of distracting stimuli, but more susceptible when engaged in tasks with high cognitive demand (Lavie et al., 2004; Lavie, 2005, 2010).
Given the multi-faceted nature of attention, it is perhaps unsurprising that research aimed at determining the neurobiological mechanisms that underlie it have identified a sprawling system encompassing structures from the brainstem to the frontal lobe. While the complete neuroanatomy of attention-related brain areas is outside the scope of this review, it should be noted that the parietal lobe has been shown to be critical for directing attention and enabling shifts of attention between stimulus locations and modalities (Yin and Mountcastle, 1977, Posner and Petersen, 1990). Indeed, patients with lesions in the parietal lobes exhibit a phenomenon referred to as spatial neglect and are seemingly incapable of focusing on stimuli within the receptive field of the affected hemisphere (Brain, 1941). Popular theories based on data from humans and primate (e.g., Corbetta and Shulman, 2002) as well as rodents (Bucci et al., 1998) suggest that such parietal areas are particularly important for bottom-up or surprise-induced changes in attention, perhaps due in part to their connectivity with limbic structures like the dorsal posterior cingulate cortex, which has been implicated in orienting toward a stimulus (Vogt et al., 2006). Areas of the prefrontal cortex (PFC), in contrast, are typically described as important for ‘top-down’ or goal directed attention (Nagahama et al., 2001; Corbetta and Shulman, 2002; Buschman and Miller, 2007; Li et al., 2010), although it is important to note that in the literature a number of functionally and anatomically distinct subregions are often labeled as “PFC”, making it difficult to get a clear picture of the precise functions supported by discrete areas within the frontal lobe. A major barrier to higher granularity in the causal role of different subregions of the PFC to attentional control stems from anatomical differences between humans and the model systems typically used to test the necessity and sufficiency of brain areas for different functions. Inconsistencies in PFC nomenclature applied to model systems research further complicates efforts to integrate data across species. These issues have led to several recent revisions of rodent PFC anatomy and terminology (e.g., Laubach et al., 2018). For the purposes of this review, we note that in primates the term PFC is often used to refer to granular cortex that is largely absent in rodents (Preuss and Wise, 2022), whereas anterior cingulate (ACC) is a term typically used in reference to agranular subregions of the primate frontal lobe (Laubach et al., 2018). Within the rodent literature, studies commonly refer to the prelimbic (PrL) and infralimbic (IL) subregions of the medial frontal wall as “PFC” or “mPFC,” and more dorsal and posterior frontal cortical regions are referred to as cingulate cortex (e.g., Cg1/2). Further confounding the matter, some authors refer to the whole of the rodent frontal cortex as the anterior cingulate cortex (ACC), in reference to the largely agranular composition of the rodent frontal lobe (Vogt and Gabriel, 1993; Vogt et al., 2013). Recently researchers have made efforts towards establishing a more useful cross-species terminology that focuses on the major subdivisions of the rodent PFC and their potential homologies with the primate cortex (e.g., Laubach et al., 2018). In this schema, rodent PrL is roughly equivalent to pregenual ACC in the primate, IL with subgenual ACC, and the more dorsal and posterior cingulate cortex (e.g., Cg1 and Cg2) as synonymous with anterior midcingulate cortex (aMCC; Fillinger et al., 2017; Laubach et al., 2018; van Heukelum et al., 2020). Importantly, all these frontal cortex subregions have all been linked to the control of attention in primates and rodents. For example, studies in rodents have shown that lesions encompassing the PrL and IL selectively impair stimulus-guided attentional performance and not working memory (Kahn et al., 2012), and suggest these structures might be particularly critical when tasks require the ability to shift attention between perceptual domains (Birrell and Brown, 2000). The rodent aMCC in contrast, appears necessary for the acquisition of stimulus-response contingencies in attention tasks (Bussey et al., 1997), and interestingly, may act in concert with the PrL to maintain attentional focus in anticipation of instructive task cues (Totah et al., 2013). Research from primates and humans also suggests the posterior MCC may be key for orienting toward the location of a stimulus and shifting of attention based on cognitive demand, arousal, and awareness (Kulkarni et al., 2005; Leech and Sharp, 2014; Vogt, 2016). Together, studies from rodents, non-human primates, and humans all highlight the importance of parietal and frontal lobe structures for the control of different aspects of attention, though clearly more work is needed to understand the unique computations supported by the subregions within each.
Outside of the cortex, multiple thalamic nuclei appear to be important for attentional control. The pulvinar nuclei, which share reciprocal connections with the superior colliculus, visual cortex, and provide input to multiple frontal cortical areas, have been suggested to be key for both attentional orienting and the filtering of distracting stimuli (Posner and Petersen, 1990; Fischer and Whitney, 2012). In addition, projections from the medio-dorsal thalamus have been suggested to be key for relaying stimulus information to the cortex so that associative information imparted by a predictive stimulus may be used to guide behavior (Parikh and Sarter, 2008; Parikh et al., 2010; Hasselmo and Sarter, 2011; Saalmann and Kastner, 2015). Notably, the thalamic reticular complex or nucleus, a band of GABAergic cells that encapsulates the thalamus, has also been proposed to serve as an “attentional searchlight,” effectively focusing such thalamic input to the cortex via targeted inhibition (Crick, 1984; McAlonan et al., 2000; Pinault, 2004).
The term ‘reward’ is one that is commonly used by both the scientific and non-scientific community. Its commonality has created a certain amount of historical confusion around the way the term is being operationalized in different contexts. Like attention, in the scientific literature ‘reward’ is shorthand for several related functions that serve to gate the probability of behaviors proximal to the presentation of a stimulus. Berridge and Robinson (2003) proposed that reward can be broken into 3 separable, but connected, functional domains which we will focus upon here: affect/hedonics, reinforcement/associative learning, and motivation. The domain of hedonics chiefly concerns the affective experience of pleasure (positive) or disgust (negative). These hedonic signals are typically associated with the subjective experience of a primary reward like food or sex (Berridge and Kringelbach, 2011). These subjective effects can be enormous, such as the pleasure associated with the taste of a favorite food when hungry, or conversely visceral malaise following food poisoning (Cabanac, 1992; Rolls, 2005). Certain drugs of abuse, in particular opiates, are also capable of generating intense subjective experiences of pleasure (Doyle et al., 1993; Berridge et al., 2009). In humans, such hedonic encoding can also be applied to more abstract rewards, like art or social interactions (Berridge and Kringelbach, 2011; Goller et al., 2019), highlighting the interplay between learning and affective representations. In addition to interacting with affect, associative learning processes comprise their own unique domain of reward. Reinforcement learning refers to a particular class of associative learning focused on optimizing the outcomes of behavior and guided by representations of the relationship between predictive stimuli, actions, and value of a primary reward. Importantly, such learning is distinct from the hedonic impact (e.g., pleasure or disgust) associated with the unconditioned stimulus (or “reward”), though the two information streams likely interact. These learned and reinforced associations are often described as either model-free or model-based. The most straightforward way of conceptualizing the distinction between these two models is to first consider a primary reward (food or sex) as an unconditioned stimulus (something with innate value), and a neutral cue that predicts its future availability. In model-free learning, a learned association is an inevitable consequence of repeated pairing of the neutral cue with subsequent reward. While the association between cue and reward is shaped by the magnitude or “value” of the unconditioned stimulus, a conscious representation of a reward’s value, or the pleasure associated with receiving it, is not necessary to establish this relationship. In contrast, model-based learning assumes there is an internal, necessarily neurobiological, representation of need, probability, or magnitude of the predicted reward that influences the strength of the association. A key feature of such model-based associations is that these internal representations of reward value or contingency can be engaged to economize the decision-making process (Kahneman, 2003; Daw et al., 2005, 2011; Dayan and Berridge, 2014). Importantly, both forms of learning are accepted as playing major roles in guiding reward-related behavior, and while historically considered as separable functions likely controlled by dissociable brain networks, more recent work suggests that the two work in concert via overlapping circuitry (e.g., O’Doherty et al., 2017). The third domain of reward concerns the construct of motivation. Motivation is typically used to refer to a process or force that controls the vigor of ongoing behavior. It has alternatively been labeled as the desire, or “wanting”, for a particular reward or reward-paired stimulus (Berridge et al., 1989; Robinson and Berridge, 1993). Theories of motivation as a construct can be traced back to the work of Clark Hull, who posited that internal states drive an agent to satisfy physiological needs like access to food, water, and mates (Hull, 1943). Later work expanded upon these ideas to more fully incorporate the capacity of cues that have been paired with primary rewards to become potent activators of appetitive behaviors. Such ‘incentive stimuli’ take on reward-like qualities and can serve as reinforcers in and of themselves (Bindra, 1969; Bolles, 1972; Toates, 1986; Robinson and Berridge, 1993). Modern theories of motivation place varying amounts of emphasis on these two sources of drive, though each shares the capacity to trigger, attract, and guide appetitive behavior.
Like attention, the ability of behavior and cognition to be shaped by reward represents a major adaptive advantage, and our brains have evolved brain circuits to facilitate their encoding and representation (Nesse, 2002; Berridge and Kringelbach, 2008). The first set of experiments looking into the idea of localized reward centers was conducted in 1954 by Olds and Milner (1954), and demonstrated that stimulating the medial forebrain bundle, which is comprised of fibers connecting the hindbrain, midbrain, and forebrain, produced a range of reinforcement behaviors (Olds and Milner, 1954). In the years since this pioneering work, our understanding of how each of the domains of reward function are represented in the brain has become more in-depth, implicating a vast network of cortical and subcortical structures. Interestingly, while different studies have highlighted support for a variety of subcircuits in different domains of reward function, it is clear certain structures are key for each; the striatum (namely dorsal medial striatum (DS) and nucleus accumbens (NAc) core and shell, the orbitofrontal cortex (OFC), and the amygdala (basolateral and centro-medial). Manipulation of activity in the striatum, particularly the shell of the NAc, is key for modifying the experience of sensory pleasure (Castro and Berridge, 2014a). Work in rodents, primates, and humans has also demonstrated strong support for the OFC as a hub for neural encoding of pleasure associated with primary tastes (Castro and Berridge, 2017), smell (de Araujo et al., 2003), and sex (Buchel et al., 2018). The amygdala, which receives input from ascending and descending gustatory systems, provides strong input to the shell of the nucleus accumbens, and has long been suggested to represent a region where sensory inputs are ascribed with hedonic reward qualities (Aggleton, 1986; Zahm, 1999). Though unlike the striatum and OFC, evidence is less clear, and several studies have noted a lack of modification of sensory pleasure following amygdala manipulations (e.g., Castro and Berridge, 2014a). These same regions have also been identified by studies probing the neurobiological underpinnings of associative reward learning. OFC lesions reduce the capacity for rats to discriminate between good and bad predictors of reward (Ostlund and Balleine, 2007). The OFC is essential for encoding food-motivated associative learning (Aou et al., 1983; Ross et al., 2005), and shifting actions following a change in outcome value (Gremel et al., 2016), supporting a role for the OFC in specifically model-based learning (Huang et al., 2020). Similarly, lesions of the dorsal medial striatum (DMS) (Yin et al., 2005), NAc core and shell (Noguer-Calabús et al., 2022), and amygdala (Málková et al., 1997; Balleine et al., 2003) all impair the ability of animals to update their behavior based on changes in reward value and contingency. The amygdala shares connections with the OFC and is a major source of excitatory input to the striatum, and manipulations of OFC to basolateral amygdala (BLA) connectivity can differentially modulate value updating and retrieval, highlighting the importance of inter-connectivity within this circuitry (Malvaez et al., 2019). It is also worth noting that the importance of the OFC, amygdala, and striatum in both the encoding and retrieval of reward values (e.g., Balleine et al., 2003; Yin et al., 2005; Ostlund and Balleine, 2007; Malvaez et al., 2019; Lichtenberg et al., 2021; Sias et al., 2021) highlights the dynamic interplay between reward learning and memory systems that future studies should interrogate more explicitly. Striatal and amygdala circuitries have also been shown to be critical for both global motivational drive as well as the attribution of motivational value to reward paired cues (e.g., Holland and Gallagher, 2003; Corbit and Balleine, 2005; Talmi et al., 2008; Winstanley et al., 2010; Saunders et al., 2013, 2018). The contribution of OFC to motivation, independent of behavioral modifications stemming from updated representations of reward value, is less clear, though again multiple studies have noted correlations between OFC function and motivated behavior (Gallagher et al., 1999; Arana et al., 2003; Cetin et al., 2004).
An important question that emerges from this work highlighting shared neural circuitry across reward domains is how discrete functions are encoded within them. One possible explanation is that different domains of reward are encoded by unique patterns of neuromodulation that shift the activity state of local microcircuitries within these structures. Harkening back to the original experiments by Olds and Milner, midbrain dopamine (DA) systems are known to modulate multiple reward functions and as such, are a key part of the larger neuroanatomy of reward. Midbrain DA systems can be roughly segregated into two separate projection systems. The nigro-striatal system begins in the substantia nigra pars compacta (SNc) and largely projects to the DS as well as the central amygdala (Poulin et al., 2018), pallidum (Lavoie et al., 1989), subthalamic nucleus (Cragg et al., 2004), and motor thalamus (Antal et al., 2014). The meso-cortico-limbic pathway stems from the ventral tegmental area (VTA) and is the primary source of DA innervation of the NAc, frontal association cortex, BLA, and other components of brain limbic systems (Beier et al., 2015). Early work on DA modulation of food reward led to the hypothesis that these systems in the brain encode sensory pleasure associated with a food reward (Wise et al., 1978). Direct tests of this hypothesis suggested that DA is neither necessary nor sufficient for the experience of sensory pleasure (Berridge et al., 1989; Berridge and Valenstein, 1991). Pioneering work by Montague and colleagues provided the first accounts of a shift in DA neuron activity from the receipt of an unexpected reward to the time of a cue that predicts a reward, as a mechanism of associative learning (Montague et al., 1996; Schultz et al., 1997). This demonstration seeded the hypothesis that midbrain DA systems encode a reward prediction error, or the difference in value between received and expected rewards (Montague and Berns, 2002; Schultz, 2016; Lerner et al., 2021). More recent studies have suggested that SNc and VTA DA populations uniquely contribute to different aspects of reward learning, perhaps via changes in the patterns and timescales of on-going release (Hamid et al., 2016, 2021; Saunders et al., 2018; Mohebi et al., 2019), though overlapping and distinct functions of each are still debated. Hypotheses regarding the importance of DA for reward learning are supported by historical and recent studies (e.g., Amo et al., 2022). That said, there is also a substantial body of work suggesting that DA systems are keenly involved in motivated behaviors as well. Hyper-dopaminergic mice show increased willingness to work for food rewards, supporting a role in general appetitive drive (Pecina et al., 2003). More specifically, meso-limbic DA projections to the NAc are necessary for a predictive stimulus to become a driver of behavior, suggesting that VTA to NAc DA systems are particularly important for the attribution of incentive value to reward cues (e.g., Cannon and Palmiter, 2003; Robinson et al., 2006; Palmiter, 2008; Flagel et al., 2011; Saunders and Robinson, 2012; Saunders et al., 2013; Gallardo et al., 2014). Importantly, work in rodents demonstrates that PrL, IL, and amygdala receive inputs from, and project back to, these mesolimbic reward circuitries, setting the stage for dynamic feedback loops that are engaged to support reward-based decision making (Carr and Sesack, 2000; Geisler and Wise, 2008; Jo et al., 2013; Steinberg et al., 2020). This combined work importantly highlights a key role for neuromodulation in the coding of reward, and as we move forward, we will see that like DA, ascending cholinergic systems also likely contribute to specific reward states via circuit-defined patterns of activity.
Acetylcholine (ACh) was discovered in a series of experiments by Loewi (1921), providing the first demonstration of chemical signaling in the nervous system. ACh is ubiquitous in the peripheral and central nervous systems. When released, ACh exerts its effects via actions on two major receptor subtypes, muscarinic (mAChR) and nicotinic (nAChR) receptors. There are five different isoforms of mAChRs which are broadly separated into two groups: M1-like (M1, M3, and M5) which are generally post-synaptic and excitatory, and M2-like (M2, M4) which can be pre- or post-synaptic and are typically inhibitory (Kruse et al., 2014). nAChRs, in contrast, are ligand-gated cation channels that depolarize cells when opened. These pentameric receptors are composed of combinations of α- (α2–10) and β (β2–4)-subunits, the most common configurations found in the brain being the α4β2 and α7 subtypes (Leonard and Bertrand, 2001; Perry et al., 2002; Gotti and Clementi, 2004). In the brain, ACh is produced and released by a small number of neurons located in the brainstem, basal forebrain (BF), and striatum. The brainstem cholinergic system comprises two separate nuclei in the pons: the pedunculopontine nucleus (PPN) and the lateral dorsal tegmentum (LDT). These cells are important for regulating general behavioral sleep and arousal, their major projection target being the thalamus. The PPN/LDT is interconnected with another cholinergic nuclei, the basal forebrain (BF; Mesulam et al., 1983; Satoh and Fibiger, 1986; Woolf and Butcher, 1986, 1991), which in turn sends projections back to the PPN/LDT (Jourdain, 1988). Brainstem cholinergic systems also target midbrain DA cell populations of the VTA and SNc (Oakman et al., 1995; Forster and Blaha, 2000; Xiao et al., 2016), suggesting these cells may contribute to several reward and motor-related functions. Cholinergic neurons of the BF are the predominant source of acetylcholine in the cortex in rodents (Johnston et al., 1979; Wenk et al., 1980), non-human primates (Kitt et al., 1987), and humans (Mesulam and Van Hoesen, 1976; Mesulam, 1996). These cells are also the major source of ACh in the hippocampus and amygdala (Divac, 1975; Lehmann et al., 1980; Mesulam et al., 1983; Halliwell, 1989; Woolf and Butcher, 1991). The nucleus basalis of Mynert (nBM), a band of neurons within the BF, receives input from many monoaminergic systems including DA from the VTA (Fallon and Moore, 1978; Beckstead et al., 1979), serotonin from the dorsal raphe nuclei (Jones and Cuello, 1989), and norepinephrine from the locus coeruleus (Zaborszky, 1989). The BF also shares reciprocal connections with multiple areas of associative cortex important for attention and reward processing, notably the PrL and IL cortices in rodents and the OFC and temporal lobe in primates (Mesulam and Mufson, 1984; Gaykema et al., 1991; Zaborszky et al., 1997). Given this pattern of connectivity, along with the demonstration of profuse loss in the number and density of nBM neurons in brain samples from Alzheimer’s disease patients (Whitehouse et al., 1982), the BF has long been a target for research into brain systems underlying cognitive functions (Whishaw et al., 1985; Jacob Huff et al., 1988; Mandel et al., 1989). At the level of the striatum, the major source of ACh is a small population of local cholinergic interneurons (CINs; Bolam, 1984; Calabresi et al., 2000). The striatum has among the highest levels of markers for cholinergic signaling in the brain though it receives no input from the BF, and only a minor input from the PPN/LDT (Macintosh, 1941; Hebb and Silver, 1961; Woolf et al., 1984; Dautan et al., 2016). The striatum is largely a GABAergic nucleus; 95% of its neurons are GABAergic medium spiny (MSN) projection cells, and another 4% are local GABAergic interneurons. The remaining 1–2% of cells are CINs which are believed to produce a majority of striatal ACh (Woolf and Butcher, 1981; Oldenburg and Ding, 2011). First identified in 1896 by Kölliker (1896), these extensively arborized cells can directly modulate the activity state of striatal microcircuitries via muscarinic acetylcholine receptors expressed by both D1 and D2 populations of MSNs (Yan et al., 2001; Surmeier et al., 2007). CINs have large dendritic trees and because of this branching structure, they can integrate synaptic inputs over a large region (Wilson et al., 1990). Given their location in the striatum, it is perhaps not surprising that existing evidence suggests these cells may be key for modulating motor output and reward function (Pisani et al., 2007; Gritton et al., 2019; Mohebi et al., 2023). There is also a sparse population of interneurons in the cortex that are positive for choline acetyltransferase, an enzyme critical for the synthesis of ACh. These cells have been suggested to represent an additional source of cholinergic modulation in the cortex, though it should be noted that their expression varies between model organisms, and there is mixed evidence regarding their presence in humans (Hedreen et al., 1983; Mesulam et al., 1983; Avendaño et al., 1996; Raghanti et al., 2008). Research in rodent models suggests this cell population may independently contribute to attentional function, in addition to input from the basal forebrain (e.g., Obermayer et al., 2019). These interneurons are enriched in cortical layers 2/3, have a bipolar morphology, and receive excitatory and inhibitory input from nearby pyramidal cells. Their activation has an excitatory effect on surrounding cells, mediated in large part by nAChRs (e.g., Von Engelhardt et al., 2007; Obermayer et al., 2017, 2019).
Some of the first experiments into the functions of ACh in the brain provided strong evidence that cholinergic signaling can potently modulate the impact of sensory inputs on activity in primary sensory cortex (Metherate and Weinberger, 1990; Metherate and Ashe, 1991). Indeed, ACh appears to enhance the representation of thalamic input across multiple sensory cortical subregions, while simultaneously suppressing the impact of intracortical input on local activity (Hasselmo and Bower, 1992; Hasselmo and Cekic, 1996). These observations supported the general hypothesis that cholinergic inputs determine the strength of the representation of an external stimulus in sensory cortex (Donoghue, 1987; Ma et al., 1989; Tremblay et al., 1990a,b), perhaps through the modulation of local synaptic plasticity (Cole and Nicoll, 1984; Krnjević, 1993). Following the development of toxins used to selectively lesion cholinergic cells in animal models, evidence began to accumulate that suggested cortical ACh may play a more specific role in the modulation of attention. Specifically, lesions of basal forebrain via ACh-immunotoxin 192 IgG-saporin impaired performance on a sustained attention task designed for rodents, selectively reducing the capacity of rats to report the presence of instructive cues, while having no effect on their ability to report the absence of these cues (McGaughy et al., 1996). Similarly, selective cholinergic deafferentation of the entire cortical mantle was shown to impact the performance of attention tasks where animals must flexibly switch between stimulus modalities to guide behavioral selection (Turchi and Sarter, 1997). Additional work utilizing microdialysis in rats demonstrated that extra-synaptic ACh concentrations measured with a probe covering both the aMCC and parts of posterior parietal cortex greatly increase during tasks that explicitly tax attention compared to control tasks that include similar motor output and reward delivery (Arnold et al., 2002). In conjunction with primate studies demonstrating the necessity of ACh signaling for attentional modulation of neural activity in the visual cortex (Fries et al., 2001; Herrero et al., 2008), by the late 2000s the consensus in the field was that the BF-cortical cholinergic system is a chief component of brain attention networks. Further insight into the way cortical ACh contributes to attention came on the heels of the development of biosensors capable of monitoring sub-second ACh release dynamics is discrete parts of the cortex. Utilizing this technology, fast increases in ACh release were observed in the rodent PrL, but not the motor cortex, when animals successfully detected reward-predictive cues and used them to guide their subsequent behavior (Parikh et al., 2007). These data provided the first-ever demonstration of fast (e.g., seconds long) and cortex-area specific patterns of ACh release in task-performing animals, and suggested that regional patterns of ACh release are dynamic and key to the control of attention and related functions Further, such cue-evoked ACh release events develop only after animals learn the predictive value of cues, are not present when animals ignore these cues, and not observed when animals are aske to similiary indicate the absence of predictive cues (Parikh and Sarter, 2008; Howe et al., 2013). Follow up studies in rats and transgenic mice demonstrated that cue-triggered ACh release in PrL can amplify glutamate released by thalamic inputs (Parikh and Sarter, 2008; Parikh et al., 2008), increase synchrony in gamma-frequency oscillations in local cell populations via binding at both nAChRs and mAChRs (Bailey et al., 2010; Guillem et al., 2011; Howe et al., 2017; Záborszky et al., 2018; Lu et al., 2020; Yang et al., 2021), and is ultimately causal in the detection process (Gritton et al., 2016). Collapsing across data accumulated over the last four decades, it seems that BF cholinergic systems, particularly their projections to areas like the rodent PrL, are a central feature of the neuroanatomy of attention, and contribute by generating rapid bursts of release that bias activity in local cell populations such that predictive cues can gain control of ongoing decision-making machinery and guide behavior (Hasselmo and Sarter, 2011). Whether this effect of ACh on post-synaptic cells populations is ‘transient’ or can additionally influence attentional performance by supporting local synaptic remodeling as demonstrated in sensory cortex, is currently unknown, though it is interesting to speculate that ACh might be operating over multiple timescales to support both on-going cue-based decision making and facilitating future attentional performance by re-wiring cortical micro-circuitries.
Each of the major brain cholinergic systems have been linked to reward-related computations as well. Selective recordings of calcium dynamics in brainstem cholinergic neurons of mice have demonstrated robust modulation of neuronal activity around the time of receiving a food reward (Ruan et al., 2022). Similarly, studies in humans have shown that PPN activity is modulated by reward receipt, and further, electrical stimulation of the PPN selectively enhances reward (but not punishment)-based learning (Skvortsova et al., 2021). Interestingly, single unit recordings from presumptive cholinergic cells in primate PPN identified neurons that were modulated by reward expectation and others that were responsive reward receipt, suggestive of distinctly tuned cholinergic populations in the brainstem (Kobayashi and Okada, 2007). Physiological correlates of reward-related computations have been described in CINs of the striatum as well. In Pavlovian cue-reward paradigms, CINs respond to a predictive stimulus only when it is followed by reward (Apicella et al., 1991; Graybiel et al., 1994). CINs specifically exhibit a pause in firing following salient stimuli (Aosaki et al., 1994), which may be linked to the encoding of reward presentation (Shimo and Hikosaka, 2001) and facilitate information provided by coincident DA signals (Cragg, 2006). Selective inhibition of CINs in the NAc core increases, and optogenetic stimulation reduces, reward-seeking behavior (Collins et al., 2019). Conversely, blockade of muscarinic receptors in the NAc shell with non-selective antagonist scopolamine reduces both the pleasure associated with a food reward, as well as the motivation to seek it (Perry et al., 2009; Castro et al., 2016). This disparity could indicate region-specific encoding of reward by striatal CINs, though it should be noted that local scopolamine application has been shown to increase ACh release (Parikh et al., 2004). Striatal ACh has also been linked to the maintenance of flexibility in reward-guided behavior; the disruption of which could contribute to habitual reward-seeking phenotypes that have been associated disorders like drug abuse (Ragozzino, 2003; Ragozzino et al., 2009; Bradfield et al., 2013; Matamales et al., 2016; Favier et al., 2020). Neurophysiological evidence also suggests that BF cholinergic neurons signal reward and punishment through changes in their spiking activity as well. Single unit recordings with the BF revealed that populations of cholinergic neurons respond to both appetitive rewards and aversive stimuli, and moreover, the magnitude of these responses scale with predicted outcome values, similar to the activity of DA neurons in cued-reward tasks (Hangya et al., 2015). ACh from BF terminals is released in the BLA in response to reward predictive cues, and such release facilitates cue-reward learning (Crouse et al., 2020). Conversely, BLA ACh release, in interaction with local norepinephrine signaling, also appears to contribute to the modulation of behaviors associated with negative affective states (Mineur et al., 2016, 2018, 2022; Sizer et al., 2022).
The literature linking the activity of ACh systems to the control of specific reward behaviors is growing. Notably, brain cholinergic systems are anatomically well positioned to directly modulate the activity of midbrain DA systems, and thereby the multiple reward functions linked to DA release. DA neurons express both mAChRs and nAChRs, and nAChR modulation of DA is believed to be a key feature in pathological reward seeking in addiction (de Kloet et al., 2015; Grasing, 2016). As stated above, output from brainstem cholinergic systems directly targets DA neurons in the VTA and SNc (Dautan et al., 2014; Mena-Segovia and Bolam, 2017). Input from brainstem cholinergic neurons to SNc DA neurons can cause these cells to burst-fire, suggesting they can directly support the capacity of phasic DA to encode reward (Hong and Hikosaka, 2014). A similar result has been reported at the level of brainstem inputs to the VTA (Xiao et al., 2016), and further, activation of LDT-NAc pathway independently increases motivation, induces place preference, and drives positive reinforcement (Coimbra et al., 2019). Regarding CINs, their stimulation in the nucleus accumbens can directly drive DA release (Cachope et al., 2012). Interestingly, reward-related pauses in CIN activity are dependent upon DA receptor binding on CINs, suggesting that reward functions are likely dependent on a delicate balance of ACh and DA release in the striatum (Zhang et al., 2018; Gallo et al., 2022; Mohebi et al., 2023). Unlike brainstem and striatal cholinergic systems, evidence for direct control of VTA DA neurons by BF cholinergic neurons is lacking, though interestingly both GABAergic and glutamatergic projection neurons of the BF do target the midbrain, modulate DA neuron activity, and subsequent reward-related behaviors (Cai, 2020; Wang et al., 2021). Furthermore, the VTA sends projections back to the BF (Swanson, 1982; Gaykema and Zaborszky, 1996, 1997; Hu et al., 2016; Gielow and Zaborszky, 2017), again highlighting the structural and functional intermingling of these two systems.
A robust literature supports the general notion that internal representations of reward influence our decision of what to pay attention to. For example, the ability of a stimulus to capture attention is determined by the magnitude of reward it has been paired with, or its validity as a predictor of reward (Poh et al., 2019; Kaskan et al., 2022). High value rewards are particularly potent attractors of attention, and stimuli associated with them can become a source of distraction if presented in the context of other goal directed actions (Anderson et al., 2011; Watson et al., 2020). For example, Anderson et al. (2011) trained human participants to associate a color with a monetary reward. Subjects then completed a separate visual search task, and even though contextually irrelevant, presentation of that color increased the amount of time it took subjects to identify the task-relevant stimulus. Similarly, Watson et al. (2020) showed that there is a relationship between reward magnitude and time it takes one to avert their attention away from the stimulus that produced that reward previously. Together, studies from human subjects support the notion that stored representations of reward recruited by such cues can modulate the deployment of attention across multiple contexts (Anderson et al., 2011; Watson et al., 2020). Evidence from animal models also supports this relationship and provides insights into the neural mechanisms that mediate interactions between attention and reward. When trained on a Pavlovian cue-reward association where the extension of lever into an operant chamber predicts a food reward, rodents develop a conditioned response to lever extension, though this response varies between subjects. Some animals begin to approach the reward-predictive lever when it is extended into the chamber, interacting with it as though it was a food item (Sign trackers, ST). Such ST rats appear to place greater incentive value to reward-paired cues, are more sensitive to the effects of drug-paired cues on drug seeking behavior, and even show differences in the profile of DA release in the NAc and PrL in response to reward cues (Saunders and Robinson, 2010; Flagel et al., 2011; Meyer et al., 2012; Sarter and Phillips, 2018). Other animals instead approach the location where food rewards will eventually be presented (Goal trackers, GT) (Meyer et al., 2012). Follow-up studies in ST and GT rats revealed that ST are uniquely impaired in attentional control, have lower levels of attention-task-related acetylcholine release, show alterations in the cellular tracking of the high-affinity choline transporter that ultimately reduce their capacity to sustain PrL ACh release, and are more responsive to the sensory characteristics of salient external stimuli (Paolone et al., 2010; Cherian et al., 2017; Phillips and Sarter, 2020). These rodent studies provide a compelling demonstration that the cognitive and neural systems that control reward evaluation do not occur in a vacuum, but necessarily impact attentional function and associated brain systems (e.g., forebrain cholinergic).
Above we addressed the processes that contribute to attention and reward, described their associated neuroanatomy, and presented evidence suggesting that cholinergic systems support each through actions within these brain circuits. Next, we will specifically examine evidence regarding neurobiological scaffolds that enable interactions between attention and reward. We conclude by proposing separable circuitries linking the frontal cortex with mesolimbic systems whereby cholinergic systems act to mediate the interactions between attention, representations of reward value, and motivation.
Our primary hypothesis is that representations of the value of primary rewards and reward-paired cues are a major source of bias for the online control of attention, and this process depends on both dopamine and acetylcholine release (Hasselmo, 2006; Huang and Li, 2022). However, it is important to note that early learning of cue-reward associations is also likely to be dependent upon dopamine and acetylcholine release. We propose that each of these attention-reward interactions is differentially dependent upon ACh release from cholinergic cell groups in the basal forebrain and brainstem, respectively. Beginning with early cue-reward learning, brainstem cholinergic neurons of the PPN provide direct input to midbrain DA cells, which are enriched in nAChRs (de Kloet et al., 2015). Electrophysiological recordings of PPN neurons during reward conditioning tasks reveals that separable populations of PPN neurons respond phasically to auditory and visual stimuli (tones and rewards; Pan and Hyland, 2005). Importantly, these responses are present prior to these cues being paired with rewards and precede the DA neuron responses that these cues evoke after pairing. Thus, PPN input to the VTA may relay sensory modality-specific “bottom-up” information that alerts or primes DA neurons to the presence of a sensory stimulus, and thereby facilitate the establishment of a cue-reward association (Pan and Hyland, 2005). While this PPN input may facilitate the early stages of DA encoding of reward, the activity profile of these cells doesn’t appear to change dramatically across learning (Pan and Hyland, 2005). It therefore seems unlikely that they are a major substrate of the brain circuits that allow learned cue-reward associations to modulate attention. For this interaction, we draw attention to excitatory inputs to the dopaminergic midbrain from the frontal cortex, including the PrL and IL cortices of rats (Carr and Sesack, 2000; Geisler and Wise, 2008) and ACC and OFC of primates (Frankle et al., 2006). The PrL area in rodents, and its functional homologues in the primate cortex, have demonstrated to be key for the control of behaviors guided by representations of reward value, expectation, and error (Corbit and Balleine, 2003; Amiez et al., 2005; Rudebeck and Murray, 2014; Alexander and Brown, 2019). This region shares connections with nearly every node within the reward system, as well as other frontal cortical areas including aMCC, OFC, and IL (Carr and Sesack, 2000; Briand et al., 2007; Geisler and Wise, 2008; Wallis and Kennerley, 2010; Jo et al., 2013; Ferenczi et al., 2016). Indeed, previous rodent studies have also demonstrated that PrL input to the VTA can potently modulate the activity of local GABAergic neurons that in turn control DA neuron excitability (Jo et al., 2013), affording the PrL with the capacity to gate DA release and associated reward computations. As described above, these same frontal cortical regions (e.g., PrL/IL in rats) are critical components of the brain systems that enable attentional control and are reciprocally connected with the basal forebrain (Gaykema et al., 1991; Bloem et al., 2014). This combined pattern of connectivity suggests that these circuits connecting areas like PrL to ascending DA and ACh projection systems are uniquely situated to serve as a nexus of attention and reward. Building off these findings, we propose a circuit model whereby mesolimbic reward computations can directly impact cue-detection and attentional control. To conceptualize the functional interaction supported by this circuitry, we imagine an individual on a long car trip, constantly monitoring the roadside for the sign that indicates the exit they need to take to ultimately arrive at their desired destination. In the first scenario (Figure 1A), the individual successfully detects the exit sign. This detection event is supported by a transient increase in ACh release evoked in frontal cue detection networks (‘FDN’) as described above (e.g., PrL of rodents, analogous structures in primates; Parikh et al., 2007; Howe et al., 2013). This cue-evoked increase in ACh synchronizes oscillatory activity within the gamma frequency band through activation of local nAChRs and mAChRs, located on local interneurons and pyramidal cells within the FDN (Poorthuis et al., 2013; de Kloet et al., 2015; Howe et al., 2017). The induction of this high-frequency synchrony by ACh in turn modifies the strength of output from the FDN to the VTA. Importantly, VTA-projecting FDN neurons of deep layers 5/6 are enriched in the α5 nAChR subunit, which increases the responsivity of pyramidal cells to nAChR stimulation (Bailey et al., 2010; Poorthuis et al., 2013; Howe et al., 2018). Thus, detection-evoked ACh release in the FDN may recruit a descending pathway to the midbrain that allows attentional networks to shape the activity of DA projection neurons, and the cue-reward associations they encode. In an alternative scenario (Figure 1B), we imagine that while the individual is driving and scanning for the exit sign, they suddenly encounter an advertisement from a purveyor of their favorite treat: hot, delicious donuts. Drawn in by the promise of this potent primary reward signaled by the advertisement, the individual misses the sign that indicates their intended exit. Functionally, the presentation of the donut sign, a conditioned stimulus associated with an alternative source of reward, decreased the ability of the task-relevant cue (the exit sign) to capture their attention. Mechanistically, this interference could stem from direct interactions between the VTA/SNc and basal forebrain. Neurons of the VTA/SNc send efferent projections to the basal forebrain (Zaborszky and Cullinan, 1996; Gaykema and Zaborszky, 1997). This projection appears to be largely GABAergic (Gaykema and Zaborszky, 1997), though modern tracing studies suggest the possible existence of a minor projection from Th+ cells in the VTA to the BF (Gielow and Zaborszky, 2017). GABAergic neurons of the VTA are particularly responsive to reward cues (Wakabayashi et al., 2019), and gate value signals generated by DA cells (Eshel et al., 2015). We suggest that the unexpected presentation of this secondary reward cue (donut sign) activates a subset of midbrain GABA neurons that then project to cholinergic cells of the basal forebrain. Activation of this pathway could then hyperpolarize FDN-projecting BF cholinergic neurons, thereby reducing both the ACh release evoked by the exit sign (Gritton et al., 2016; Howe et al., 2017) and the probability that it can capture attention and guide subsequent behavior.
Figure 1. Proposed circuitry underlying the capacity of value representations to modulate cue-detection during attention-task performance. (A) Scenario 1. An individual is driving down the road monitoring for a road sign (cue) that indicates their intended exit. Detection of the road sign cue evokes an increase in ACh within a frontal cue detection network (or “FDN,” encompassing rodent PrL or functionally homologous structures in primates), synchronizing gamma oscillations that in turn enable the road sign cue to be used to guide behavior. FDN projection cells in layers 5/6 enriched in the α5 nAChR in turn project downstream to the VTA, which may shape activity in midbrain ensembles encoding this cue-reward association. (B) Scenario 2. A second cue, previously associated with a high value reward (a donut), is unexpectedly encountered. Midbrain GABAergic projections to the BF are recruited, reducing FDN ACh release and interfering with the ability of the individual to detect the exit sign cue relevant for their current goal. Created with BioRender.com.
Although the above discussion largely focuses on how learned cue-reward associations may interfere with ongoing attentional performance, it’s important to note that the brain most likely evolved circuitry to allow for such reward-based interference because it serves an adaptive advantage. Rewards are attributed with high value when they satisfy some biological or cognitive need, and thus an attention system that is oblivious to cues that signal the sudden availability of high value rewards could impair fitness. We propose that the circuitry described above represents an evolutionarily conserved mechanism for disengaging attentional focus from a current goal and enabling the subject to re-orient behavior towards the pursuit of a new one.
The notion that attentional processing can be modulated by motivation seems almost implicit; if one is hungry, they are likely engaging a significant amount of their attentional resources to scanning the environment for signs or cues that direct them to food. Similarly, a student with the goal of receiving high marks is likely to be more engaged and focused on lecture material. A significant amount of research has thus been devoted to understanding this crucial interplay between internal drive states and attentional control, although little consensus exists regarding the precise neurobiological mechanisms that mediate it. A major reason for this lack of consensus stems from the complexity of the constructs of motivation and attention. As reviewed in the preceding text, motivation on its own is a term used to refer to the influence of homeostatic drive states and cognitive representations of desired outcomes on the direction and vigor of ongoing behavior. Similarly, attention may refer to the process of maintaining focus over time, the selection of cues for integration into online decision-making processes (as described above and in Figure 1), or even the filtering out of distracting, task-irrelevant stimuli (e.g., “distractors”). In an influential review, Sarter et al. (2006) conceptualized the specific interactions between attention and motivation as driven by “cognitive incentives”, or internal representations of a goal that drive a subject to maintain attentional control when faced with performance challenges like distractors (Berridge and Robinson, 2003; Sarter et al., 2006). Here, we also focus our discussion on such goal-mediated attentional control mechanisms by first highlighting the major circuitry proposed by Sarter and colleagues. We then extend this model to integrate additional information regarding specific mechanisms revealed by recent studies and propose a shared pathway whereby cognitive and homeostatic drive systems can modulate both cortical cholinergic activity and attentional control.
To begin, we imagine an individual playing a video game at home. The goal of the game is simple; navigate a race car around a track at high speed. Each player-driver is accompanied by a co-pilot, who signals the direction of upcoming turns by flashing an arrow on a heads-up display. The faster the driver responds to the prompt from the co-pilot, the more efficient their path around the track, and the more likely they are to win the race. In our scenario, the driver is competing against their friends, and we assume that they have a desire to win. The race begins, when suddenly our driver’s sibling enters the room and turns on a television in the background. Curious about the sounds coming from the TV, the driver’s focus begins to drift from the screen. The driver then fails to detect the co-pilot’s subsequent cues, causing them to miss turns and fall behind in the race. As in the scenario above (Figure 1) describing the influence of competing value representations on attentional cue detection, here we also have a competing stimulus that is interfering with the individual’s ability to detect the task events that could be used to guide their ongoing behavior. For the present example, however, we want to highlight situations in which the individual’s ability to detect cues is not being modified by a competing representation of a reward value, or activation of an alternative drive state, per se. Rather, a situation in which an abundance of task-irrelevant environmental noise, or distractors, interfere with the driver’s ability to stay on-task. Within this context, we focus on the process whereby the individual recognizes a decline in performance in a game or task that is ongoing, and motivated by their will to win, they volitionally re-double their effort to maintain focus (Figure 2). The model of the brain circuitry that enables the individual to re-engage attentional focus proposed by Sarter et al. begins with this awareness of the impaired performance at the level of the aMCC. The aMCC is an aforementioned part of associative cortex that has long been proposed to be key in the interaction between motivation and attention (e.g., Mesulam, 1981). Animal and human studies provide support that the aMCC is key for the detection of negative events, errors, and more generally calculating cost-benefit ratios during goal-directed task performance (Carter et al., 1998; Walton et al., 2002; Brown and Braver, 2005; Totah et al., 2009; Hillman and Bilkey, 2010; Hayden et al., 2011; Brockett and Roesch, 2021). This information about performance decrements is then relayed from aMCC to the mesolimbic system, specifically the shell of the NAc (NAcS). NAcS is a subregion of the mesolimbic system with a unique pattern of inputs, enriched in markers of cholinergic signaling and neuropeptides, and is generally believed to play distinct roles in reward-guided behaviors relative to other parts of the striatum (e.g., Di Chiara, 2002; Saddoris et al., 2015; Shin et al., 2017; Castro and Bruchas, 2019). Neurons of the NAcS project to the cholinergic BF, and in interaction with local DA signaling have been shown to modulate BF output and ACh release in the PrL of rats (e.g., Moore et al., 1999; Brooks et al., 2007). This pathway represents a critical link between cortical and mesolimbic circuits whereby goal-directed motivation can modify attention. For example, the inclusion of a distracting stimulus decreases performance accuracy in attention tasks, and local infusion of NMDA into the NAcS is sufficient to increase ACh release in the FDN and reverse distracter-induced impairments in performance (Peters et al., 2011). In the model provided by Sarter and colleagues, this capacity of NAcS projections to increase ACh within the FDN, as well as other cortical areas that contribute to attention like posterior parietal cortex, is fundamental in the recovery of attentional performance (Peters et al., 2011). To simplify and summarize the major components of the model by Sarter and colleagues, aMCC error detection neurons recruit NAcS projections to the BF, which in turn amplify FDN (and posterior parietal) ACh release through some unknown mechanism to combat challenges to attentional control (Sarter et al., 2006). Data gathered in the years since this original work supports the translational relevance of this proposed circuitry (e.g., Berry et al., 2017), and has added further granularity to the circuit mechanisms underlying it. Cell-type specific tracing studies indicate that a major target of aMCC input to the NAcS are D1-receptor expressing MSNs (Li et al., 2018). These D1 MSNs of the NAcS project directly to the VTA, and negatively modulate appetitive behaviors typically linked to mesolimbic activity (Bond et al., 2020). These findings imply that output from D1 MSNs in the NAcS hyperpolarizes DA cell bodies of the VTA, an effect that should reduce action potential-dependent DA release and general behavioral impulsivity (Pine et al., 2010; Kim and Lee, 2011; Simon et al., 2013; Weiland et al., 2014). Thus, aMCC input to D1 MSNs of the NAcS may facilitate the recovery of attentional performance in part by first inhibiting VTA output and making behavior more intentional (Flores-Dourojeanni et al., 2021). Improvement in attentional performance, however, should also depend on NAcS modulation of BF cholinergic input to the cortex. NAcS modulation of the BF is not completely understood but it has been shown that local antagonism of D2 receptors in the NAcS is sufficient to increase ACh levels in the FDN (e.g., PrL), likely through actions on local MSNs, but also potentially via D2 receptors located pre-synaptically on glutamatergic inputs (Brooks et al., 2007; Howe et al., 2016). Thus, aMCC input to D1 MSNs, and the inhibition of the VTA, should also reduce D2-mediated activity in the NAcS, and in turn, amplify ACh release in the FDN. Another important target of aMCC projections to the striatum is the local populations of CINs (e.g., Guo et al., 2015). CIN activity in the striatum has generally been linked to the control of goal-directed behavior, including the maintenance of behavioral flexibility and updating behavior based on changing response-feedback contingencies (Ragozzino et al., 2009; Brown et al., 2010; Bradfield et al., 2013). Interestingly, NAcS shows particularly high levels of aceytlcholinesterase, the enzyme that determines the temporal and spatial extent of cholinergic modulation, suggesting this circuit is uniquely tuned to changes in local ACh concentrations (Jongen-Rêlo et al., 1994; Voorn et al., 1994; Shin et al., 2015). The behavioral effects of CINs are due in part to their direct actions on local populations of MSNs (Gritton et al., 2019) which express high levels of mAChRs (Santiago and Potter, 2001), but also through their capacity to modulate terminal DA release via presynaptic nAChRs (Threlfell et al., 2012; Cachope and Cheer, 2014; Kosillo et al., 2016; Brimblecombe et al., 2018). Key to our discussion of the motivated control of attentional performance is the distinction between DA release triggered by changes in bursting activity within DA soma of the midbrain which are typically associated with encoding cue-reward associations (Montague et al., 1996; Schultz et al., 1997; Day et al., 2007; Mohebi et al., 2019), and DA release that is triggered by local presynaptic modulation of DA terminals by nAChRs and correlated with the behavioral pursuit of a goal (e.g., Threlfell et al., 2012; Howe et al., 2013; Cachope and Cheer, 2014; Hamid et al., 2016; Kosillo et al., 2016; Brimblecombe et al., 2018; Mohebi et al., 2019). Recent work has highlighted that CINs exhibit changes in activity during goal pursuit like that described for DA (Mohebi et al., 2023). Combined, we propose that in addition to directly boosting cortical ACh to recover attentional function, and inhibiting action potential-dependent DA release from the VTA, aMCC inputs to the NAcS also recruit local CIN populations, which in turn directly modulate terminal DA release to amplify goal-directed behavior (summarized in Figure 2).
Figure 2. Proposed pathways by which attentional control and frontal cholinergic activity are mediated by cognitive drives. Anterior midcingulate cortex (aMCC) relays information about the loss of task rewards to D1 MSNs in the shell of the NAc. These D1 MSNs in turn project to the VTA and inhibit DA cell bodies. The resulting reduction in DA release also reduces D2 mediated inhibitory drive from the NAcS to the BF, ultimately enhancing ACh release within the FDN and helping to recover detection performance. aMCC projection neurons also target CINs of the NAcS. Recruitment of this descending pathway can increase the activity of CINs, which in turn increase action potential- independent DA release via stimulation of pre-synaptic nAChRs located on DA terminals withing the NAcS. These combined effects on CIN activity and DA release may also help boost the goal-directed motivation to recover task performance. Created with BioRender.com.
Finally, the discussion above focused explicitly on cognitive, goal-directed recruitment of mesolimbic motivational circuitry to enhance attentional control. However, goal-directed pursuit is not the only context in which motivational drive systems might interact with attentional control mechanisms to optimize performance. Returning to our initial example, consider the context in which an organism is searching for food while hungry. It seems plausible that the brain may also have developed mechanisms to enhance attention in response to such a homeostatic drive, to increase the probability that one detects and can gain access to objects that enhance their likelihood of survival. Within this context, it is important to note that the NAcS also receives abundant input from structures like the lateral hypothalamus, including the population of orexin neurons that are known to encode appetitive drive (e.g., Anand and Brobeck, 1951; Sakurai et al., 1998; Castro and Berridge, 2014a; Castro and Bruchas, 2019; Liu et al., 2020). Lateral hypothalamic orexin neurons also project to the BF, where they can directly modulate cortical ACh release and attentional performance (Arrigoni et al., 2010; Fadel and Burk, 2010; Villano et al., 2017). Thus, we propose that the NAcS may represent a key nucleus in a larger circuitry that allows hypothalamic-homeostatic drives, and frontal-cognitive goal representations, to modulate the BF output to the cortex and enhance attentional control.
Impairments in reward processing and attention are co-morbid across neuropsychiatric and neurodegenerative disorders, as well as drug addiction. We propose that efforts to develop more effective treatments for these symptoms should begin by first defining the specific disruptions in attention and reward that characterize a particular disease state. As an example, we will focus on the impact of a cue that has been paired with the receipt of drugs on attentional control in someone afflicted by addiction. Addiction is associated with increased sensitivity to drug-associated cues (Carter and Tiffany, 1999), and exposure to such drug-associated stimuli would be predicted to simultaneously impair the capacity of task-relevant cues to capture attention and guide behavior, as well as bias motivational control of behavior towards drug seeking over the task at hand (Robinson and Berridge, 1993; Lubman et al., 2000; Paolone et al., 2013). Building off the framework described above, we propose that different circuitries would underlie these two behavioral consequences of exposure to a drug cue. With respect to the capacity of a drug cue to interfere with the detection of task-relevant stimuli, we note that relative to local DA neurons, GABAergic neurons of the VTA seem to be particularly reactive to task-irrelevant stimuli (e.g., Root et al., 2020). We propose that a subset of these neurons project to the BF, are activated by drug cues when encountered during the performance of another goal directed behavior, reduce cue-evoked ACh in the FDN and ultimately interfere with cue detection. It is also worth noting that single-nucleotide polymorphisms in the α5 nAChR is associated with an increased addiction vulnerability for multiple drugs (Saccone et al., 2007; Joslyn et al., 2008). Given the positioning of these subunits across the frontal cortex, including structures within FDN, (Bailey et al., 2010), compounds that selectively boost the function of nAChRs expressing the α5 may enhance the post-synaptic effects of ACh release and simultaneously boost FDN control of the VTA to allow task-relevant cues to maintain control of behavior. With respect to the capacity of these drug cues to re-direct motivated control of behavior, we first draw attention to the importance of CIN modulation of terminal DA release that has been linked to pursuit of rewards (e.g., Hamid et al., 2016; Mohebi et al., 2023). Aside from the aMCC, the OFC is the major prefrontal projection to CINs of the striatum (Schilman et al., 2008; Klug et al., 2018). As described above, the OFC has been linked to a number of reward-related behaviors, and previous studies have shown that repeated exposure to drugs of abuse creates enduring changes in task-related OFC activity (Stalnaker et al., 2006). Interestingly, it appears that different populations of OFC neurons encode drug and non-drug rewards and increases in both the size of the neuronal population as well as the firing rate of those cells seem underlie the decision to seek drugs (Guillem and Ahmed, 2018). Thus, the increase in motivation to seek drugs triggered by drug cues may stem from an abnormally strong input from OFC to CINs and subsequent increase striatal DA release. To combat this drug cue-triggered motivational state, one could imagine that compounds capable of selectively modifying NAcS input to the BF and increases in cortical ACh concentrations could preserve cognitive control of motivation. While targets specific to the BF-projecting population of NAcS neurons are not known, it is worth acknowledging that endogenous opioid signaling mechanisms play an incredibly important role in shaping NAcS contributions to behavior (Castro and Berridge, 2014b; Castro and Bruchas, 2019). As these very mechanisms may also be altered by drugs of abuse, and contribute to the modulation of post-synaptic effects of DA and ACh in the NAcS (e.g., Fiserová et al., 1999; Laurent et al., 2014), future studies should focus on identifying peptide-specific output pathways linking the NAcS to the BF as potential sites of intervention.
It has long been proposed that the cholinergic system is at the interface between attention and reward. Ours is certainly not the first attempt at accounting for how this ubiquitous neurochemical messenger contributes to each process alone, or in interaction. However, we have attempted to make clear distinctions at both functional and circuit levels, to provide further clarity on the unique processes being mediated by ACh release. Indeed, as mounting evidence continues to suggest, the function of ACh in the brain has for too long been cast as a canonical neuromodulator system; globally rising and falling on relatively slow time scales to support ill-defined global constructs like wakefulness or arousal (Briand et al., 2007; Sarter et al., 2009). This long held idea of ACh function biases interpretations of its relevance to specific functions, which in turn spills over into the design of pharmacological therapies for disorders associated with cholinergic impairments. The clearest example of the impact of this bias is the continued prevalence of acetylcholinesterase inhibitors despite their limited efficacy (Marucci et al., 2021), which likely stems from their non-selective elevation of extra-synaptic concentrations of ACh across the brain. As we have described here, ACh, originating from different cell groups, released in different brain regions, and acting on different receptors, has the capacity to contribute uniquely to a host of different neural computations spanning sensory pleasure to the activation of previously learned action sequences. Similarly, ACh, acting within discrete circuitries that enable reward and attention to interact, can differentially contribute to the control of cue detection and cognitive control of motivation. As always, further understanding of the functions supported by ACh will require further studies. However, true progress in our understanding of its contribution to the neural basis of conscious experience and potential as a target for the treatment of disease necessitates that we as a field acknowledge the functional and regional complexities of this evolutionarily ancient ascending system.
KR: Writing – original draft, Writing – review and editing. TB: Writing – original draft, Writing – review and editing. SM: Writing – original draft, Writing – review and editing. AH: Writing – original draft, Writing – review and editing. KM: Writing – original draft, Writing – review and editing. WH: Writing – original draft, Writing – review and editing.
The author(s) declare financial support was received for the research, authorship, and/or publication of the article. This study was funded by the NIH, NIDDK, and DK133823.
The authors declare that the research was conducted in the absence of any commercial or financial relationships that could be construed as a potential conflict of interest.
All claims expressed in this article are solely those of the authors and do not necessarily represent those of their affiliated organizations, or those of the publisher, the editors and the reviewers. Any product that may be evaluated in this article, or claim that may be made by its manufacturer, is not guaranteed or endorsed by the publisher.
Aggleton, J. P. (1986). A description of the amygdalo-hippocampal interconnections in the macaque monkey. Exp. Brain Res. 64, 515–526. doi: 10.1007/BF00340489
Alexander, W. H., and Brown, J. W. (2019). The role of the anterior cingulate cortex in prediction error and signaling surprise. Top. Cogn. Sci. 11, 119–135.
Amiez, C., Joseph, J. P., and Procyk, E. (2005). Anterior cingulate error-related activity is modulated by predicted reward. European J. Neurosci. 21, 3447–3452.
Amo, R., Matias, S., Yamanaka, A., Tanaka, K. F., Uchida, N., and Watabe-Uchida, M. (2022). A gradual temporal shift of dopamine responses mirrors the progression of temporal difference error in machine learning. Nat. Neurosci. 25, 1082–1092. doi: 10.1038/s41593-022-01109-2
Anand, B. K., and Brobeck, J. R. (1951). Localization of a “feeding center” in the hypothalamus of the rat. Proc. Soc. Exp. Biol. Med. 77, 323–325.
Anderson, B. A., Laurent, A., and Yantis, S. (2011). Value-driven attentional capture. Proc. Natl. Acad. Sci. U.S.A. 108, 10367–10371.
Antal, M., Beneduce, B. M., and Regehr, W. G. (2014). The Substantia nigra conveys target-dependent excitatory and inhibitory outputs from the basal ganglia to the thalamus. J. Neurosci. 34, 8032–8042. doi: 10.1523/JNEUROSCI.0236-14.2014
Aosaki, T., Graybiel, A. M., and Kimura, M. (1994). Effect of the nigrostriatal dopamine system on acquired neural responses in the striatum of behaving monkeys. Science 265, 412–415. doi: 10.1126/science.8023166
Aou, S., Oomura, Y., and Nishino, H. (1983). Influence of acetylcholine on neuronal activity in monkey orbitofrontal cortex during bar press feeding task. Brain Res. 275, 178–182. doi: 10.1016/0006-8993(83)90433-x
Apicella, P., Ljungberg, T., Scarnati, E., and Schultz, W. (1991). Responses to reward in monkey dorsal and ventral striatum. Exp. Brain Res. 85, 491–500.
Arana, F. S., Parkinson, J. A., Hinton, E., Holland, A. J., Owen, A. M., and Roberts, A. C. (2003). Dissociable contributions of the human amygdala and orbitofrontal cortex to incentive motivation and goal selection. J. Neurosci. 23, 9632–9638. doi: 10.1523/JNEUROSCI.23-29-09632.2003
Arnold, H. M., Burk, J. A., Hodgson, E. M., Sarter, M., and Bruno, J. P. (2002). Differential cortical acetylcholine release in rats performing a sustained attention task versus behavioral control tasks that do not explicitly tax attention. Neuroscience 114, 451–460. doi: 10.1016/s0306-4522(02)00292-0
Arrigoni, E., Mochizuki, T., and Scammell, T. E. (2010). Activation of the basal forebrain by the orexin/hypocretin neurones. Acta Physiol. 198, 223–235.
Avendaño, C., Umbriaco, D., Dykes, R. W., and Descarries, L. (1996). Acetylcholine innervation of sensory and motor neocortical areas in adult cat: A choline acetyltransferase immunohistochemical study. J. Chem. Neuroanat. 11, 113–130. doi: 10.1016/0891-0618(96)00132-9
Awh, E., Belopolsky, A. V., and Theeuwes, J. (2012). Top-down versus bottom-up attentional control: A failed theoretical dichotomy. Trends Cogn. Sci. 16, 437–443. doi: 10.1016/j.tics.2012.06.010
Bailey, C. D., De Biasi, M., Fletcher, J., and Lambe, E. K. (2010). The nicotinic acetylcholine receptor α5 subunit plays a key role in attention circuitry and accuracy. J. Neurosci. 30, 9241–9252.
Balleine, B. W., Killcross, A. S., and Dickinson, A. (2003). The effect of lesions of the basolateral amygdala on instrumental conditioning. J. Neurosci. 23, 666–675.
Beckstead, R. M., Domesick, V. B., and Nauta, W. J. (1979). Efferent connections of the Substantia nigra and ventral tegmental area in the rat. Brain Res. 175, 191–217. doi: 10.1016/0006-8993(79)91001-1
Beier, K. T., Steinberg, E. E., DeLoach, K. E., Xie, S., Miyamichi, K., Schwarz, L., et al. (2015). Circuit architecture of VTA dopamine neurons revealed by systematic input-output mapping. Cell 162, 622–634.
Berridge, K. C., and Kringelbach, M. L. (2008). Affective neuroscience of pleasure: Reward in humans and animals. Psychopharmacology 199, 457–480.
Berridge, K. C., and Kringelbach, M. L. (2011). Building a neuroscience of pleasure and well-being. Psychol. Well Being Theory Res. Pract. 1, 1–26.
Berridge, K. C., and Valenstein, E. S. (1991). What psychological process mediates feeding evoked by electrical stimulation of the lateral hypothalamus? Behav. Neurosci. 105:3. doi: 10.1037//0735-7044.105.1.3
Berridge, K. C., Robinson, T. E., and Aldridge, J. W. (2009). Dissecting components of reward: ‘Liking’, ‘wanting’, and learning. Curr. Opin. Pharmacol. 9, 65–73. doi: 10.1016/j.coph.2008.12.014
Berridge, K. C., Venier, I. L., and Robinson, T. E. (1989). Taste reactivity analysis of 6-hydroxydopamine-induced aphagia: Implications for arousal and anhedonia hypotheses of dopamine function. Behav. Neurosci. 103:36. doi: 10.1037//0735-7044.103.1.36
Berry, A. S., Sarter, M., and Lustig, C. (2017). Distinct frontoparietal networks underlying attentional effort and cognitive control. J. Cogn. Neurosci. 29, 1212–1225.
Bindra, D. (1969). A unified interpretation of emotion and motivation. Ann. N. Y. Acad. Sci. 159, 1071–1083.
Birrell, J. M., and Brown, V. J. (2000). Medial frontal cortex mediates perceptual attentional set shifting in the rat. J. Neurosci. 20, 4320–4324.
Bloem, B., Schoppink, L., Rotaru, D. C., Faiz, A., Hendriks, Mansvelder, H. D., et al. (2014). Topographic mapping between basal forebrain cholinergic neurons and the medial prefrontal cortex in mice. J. Neurosci. 34, 16234–16246. doi: 10.1523/JNEUROSCI.3011-14.2014
Bolam, J. P. (1984). Synapses of identified neurons in the neostriatum. Ciba Found. Symp. 107, 30–47.
Bond, C. W., Trinko, R., Foscue, E., Furman, K., Groman, S. M., Taylor, J. R., et al. (2020). Medial nucleus accumbens projections to the ventral tegmental area control food consumption. J. Neurosci. 40, 4727–4738.
Bradfield, L. A., Bertran-Gonzalez, J., Chieng, B., and Balleine, B. W. (2013). The thalamostriatal pathway and cholinergic control of goal-directed action: Interlacing new with existing learning in the striatum. Neuron 79, 153–166. doi: 10.1016/j.neuron.2013.04.039
Brain, W. R. (1941). Visual orientation with special reference to lesions of the right cerebral hemisphere. Brain J. Neurol. 64, 244–272.
Briand, L. A., Gritton, H., Howe, W. M., Young, D. A., and Sarter, M. (2007). Modulators in concert for cognition: Modulator interactions in the prefrontal cortex. Prog. Neurobiol. 83, 69–91.
Brimblecombe, K. R., Threlfell, S., Dautan, D., Kosillo, Mena-Segovia, J., and Cragg, S. J. (2018). Targeted activation of cholinergic interneurons accounts for the modulation of dopamine by striatal nicotinic receptors. Eneuro 5. doi: 10.1523/ENEURO.0397-17.2018
Broadbent, D. E. (1954). The role of auditory localization in attention and memory span. J. exp. Psychol. 47:191. doi: 10.1037/h0054182
Brockett, A. T., and Roesch, M. R. (2021). Anterior cingulate cortex and adaptive control of brain and behavior. Int. Rev. Neurobiol. 158, 283–309.
Brooks, J. M., Sarter, M., and Bruno, J. P. (2007). D2-like receptors in nucleus accumbens negatively modulate acetylcholine release in prefrontal cortex. Neuropharmacology 53, 455–463. doi: 10.1016/j.neuropharm.2007.06.006
Brown, H. D., Baker, M., and Ragozzino, M. E. (2010). The parafascicular thalamic nucleus concomitantly influences behavioral flexibility and dorsomedial striatal acetylcholine output in rats. J. Neurosci. 30, 14390–14398. doi: 10.1523/JNEUROSCI.2167-10.2010
Brown, J. W., and Braver, T. S. (2005). Learned predictions of error likelihood in the anterior cingulate cortex. Science 307, 1118–1121.
Bucci, D. J., Holland, C., and Gallagher, M. (1998). Removal of cholinergic input to rat posterior parietal cortex disrupts incremental processing of conditioned stimuli. J. Neurosci. 18, 8038–8046. doi: 10.1523/JNEUROSCI.18-19-08038.1998
Buchel, C., Miedl, S., and Sprenger, C. (2018). Hedonic processing in humans is mediated by an opioidergic mechanism in a mesocorticolimbic system. Elife 7:e39648. doi: 10.7554/eLife.39648
Buschman, T. J., and Miller, E. K. (2007). Top-down versus bottom-up control of attention in the prefrontal and posterior parietal cortices. Science 315, 1860–1862.
Bussey, T. J., Everitt, B. J., and Robbins, T. W. (1997). Dissociable effects of cingulate and medial frontal cortex lesions on stimulus-reward learning using a novel Pavlovian autoshaping procedure for the rat: Implications for the neurobiology of emotion. Behav. Neurosci. 111:908.
Cachope, R., and Cheer, J. F. (2014). Local control of striatal dopamine release. Front. Behav. Neurosci. 8:188. doi: 10.3389/fnbeh.2014.00188
Cachope, R., Mateo, Y., Mathur, B. N., Irving, J., Wang, H. L., Morales, M., et al. (2012). Selective activation of cholinergic interneurons enhances accumbal phasic dopamine release: Setting the tone for reward processing. Cell Rep. 2, 33–41. doi: 10.1016/j.celrep.2012.05.011
Cai, Y. (2020). Striatal acetylcholine-dopamine interactions in physiology and pathophysiology. Cleveland, OH: Case Western Reserve University.
Calabresi, P., Centonze, D., Gubellini, Pisani, A., and Bernardi, G. (2000). Acetylcholine-mediated modulation of striatal function. Trends Neurosci. 23, 120–126.
Carr, D. B., and Sesack, S. R. (2000). Dopamine terminals synapse on callosal projection neurons in the rat prefrontal cortex. J. Comp. Neurol. 425, 275–283. doi: 10.1002/1096-9861(20000918)425:2<275::aid-cne9>3.0.co;2-z
Carter, B. L., and Tiffany, S. T. (1999). Meta-analysis of cue-reactivity in addiction research. Addiction 94, 327–340.
Carter, C. S., Braver, T. S., Barch, D. M., Botvinick, M. M., Noll, D., and Cohen, J. D. (1998). Anterior cingulate cortex, error detection, and the online monitoring of performance. Science 280, 747–749.
Castro, D. C., and Berridge, K. C. (2014a). Advances in the neurobiological bases for food ‘liking’ versus ‘wanting’. Physiol. Behav. 136, 22–30. doi: 10.1016/j.physbeh.2014.05.022
Castro, D. C., and Berridge, K. C. (2014b). Opioid hedonic hotspot in nucleus accumbens shell: Mu, delta, and kappa maps for enhancement of sweetness “liking” and “wanting”. J. Neurosci. 34, 4239–4250. doi: 10.1523/JNEUROSCI.4458-13.2014
Castro, D. C., and Berridge, K. C. (2017). Opioid and orexin hedonic hotspots in rat orbitofrontal cortex and insula. Proc. Natl. Acad. Sci. U.S.A. 114, E9125–E9134. doi: 10.1073/pnas.1705753114
Castro, D. C., and Bruchas, M. R. (2019). A motivational and neuropeptidergic hub: Anatomical and functional diversity within the nucleus accumbens shell. Neuron 102, 529–552. doi: 10.1016/j.neuron.2019.03.003
Castro, D. C., Terry, R. A., and Berridge, K. C. (2016). Orexin in rostral hotspot of nucleus accumbens enhances sucrose ‘liking’and intake but scopolamine in caudal shell shifts ‘liking’ toward ‘disgust’ and ‘fear’. Neuropsychopharmacology 41, 2101–2111. doi: 10.1038/npp.2016.10
Cetin, T., Freudenberg, F., Füchtemeier, M., and Koch, M. (2004). Dopamine in the orbitofrontal cortex regulates operant responding under a progressive ratio of reinforcement in rats. Neurosci. Lett. 370, 114–117. doi: 10.1016/j.neulet.2004.08.002
Cherian, A. K., Parikh, V., Wu, Q., Mao-Draayer, Y., Wang, Q., Blakely, R. D., et al. (2017). Hemicholinium-3 sensitive choline transport in human T lymphocytes: Evidence for use as a proxy for brain choline transporter (CHT) capacity. Neurochem. Int. 108, 410–416. doi: 10.1016/j.neuint.2017.05.022
Coimbra, B., Soares-Cunha, C., Vasconcelos, N. A., Domingues, A. V., Borges, S., Sousa, N., et al. (2019). Role of laterodorsal tegmentum projections to nucleus accumbens in reward-related behaviors. Nat. Commun. 10:4138.
Cole, A. E., and Nicoll, R. A. (1984). The pharmacology of cholinergic excitatory responses in hippocampal pyramidal cells. Brain Res. 305, 283–290.
Collins, A. L., Aitken, T. J., Huang, I. W., Shieh, C., Greenfield, V. Y., Monbouquette, H. G., et al. (2019). Nucleus accumbens cholinergic interneurons oppose cue-motivated behavior. Biol. Psychiatry 86, 388–396. doi: 10.1016/j.biopsych.2019.02.014
Corbetta, M., and Shulman, G. L. (2002). Control of goal-directed and stimulus-driven attention in the brain. Nat. Rev. Neurosci. 3, 201–215.
Corbit, L. H., and Balleine, B. W. (2003). The role of prelimbic cortex in instrumental conditioning. Behav. Brain Res. 146, 145–157.
Corbit, L. H., and Balleine, B. W. (2005). Double dissociation of basolateral and central amygdala lesions on the general and outcome-specific forms of pavlovian-instrumental transfer. J. Neurosci. 25, 962–970. doi: 10.1523/JNEUROSCI.4507-04.2005
Cragg, S. J. (2006). Meaningful silences: How dopamine listens to the ACh pause. Trends Neurosci. 29, 125–131. doi: 10.1016/j.tins.2006.01.003
Cragg, S. J., Baufreton, J., Xue, Y., Bolam, J. P., and Bevan, M. D. (2004). Synaptic release of dopamine in the subthalamic nucleus. Eur. J. Neurosci. 20, 1788–1802.
Crick, F. (1984). Function of the thalamic reticular complex: The searchlight hypothesis. Proc. Natl. Acad. Sci. U.S.A. 81, 4586–4590.
Crouse, R. B., Kim, K., Batchelor, H. M., Girardi, E. M., Kamaletdinova, R., Chan, J., et al. (2020). Acetylcholine is released in the basolateral amygdala in response to predictors of reward and enhances the learning of cue-reward contingency. Elife 9:e57335. doi: 10.7554/eLife.57335
Dautan, D., Hacioğlu Bay, H., Bolam, J. P., Gerdjikov, T. V., and Mena-Segovia, J. (2016). Extrinsic sources of cholinergic innervation of the striatal complex: A whole-brain mapping analysis. Front. Neuroanat. 10:1. doi: 10.3389/fnana.2016.00001
Dautan, D., Huerta-Ocampo, I., Witten, I. B., Deisseroth, K., Bolam, J. P., Gerdjikov, T., et al. (2014). A major external source of cholinergic innervation of the striatum and nucleus accumbens originates in the brainstem. J. Neurosci. 34, 4509–4518. doi: 10.1523/JNEUROSCI.5071-13.2014
Daw, N. D., Gershman, S. J., Seymour, B., Dayan, and Dolan, R. J. (2011). Model-based influences on humans’ choices and striatal prediction errors. Neuron 69, 1204–1215. doi: 10.1016/j.neuron.2011.02.027
Daw, N. D., Niv, Y., and Dayan, P. (2005). Uncertainty-based competition between prefrontal and dorsolateral striatal systems for behavioral control. Nat. Neurosci. 8, 1704–1711. doi: 10.1038/nn1560
Day, J. J., Roitman, M. F., Wightman, R. M., and Carelli, R. M. (2007). Associative learning mediates dynamic shifts in dopamine signaling in the nucleus accumbens. Nat. Neurosci. 10, 1020–1028.
Dayan, P., and Berridge, K. C. (2014). Model-based and model-free Pavlovian reward learning: Revaluation, revision, and revelation. Cogn. Affect. Behav. Neurosci. 14, 473–492. doi: 10.3758/s13415-014-0277-8
de Araujo, I. E., Rolls, E. T., Kringelbach, M. L., McGlone, F., and Phillips, N. (2003). Taste-olfactory convergence, and the representation of the pleasantness of flavour, in the human brain. Eur. J. Neurosci. 18, 2059–2068. doi: 10.1046/j.1460-9568.2003.02915.x
de Kloet, S. F., Mansvelder, H. D., and De Vries, T. J. (2015). Cholinergic modulation of dopamine pathways through nicotinic acetylcholine receptors. Biochem. Pharmacol. 97, 425–438.
Desimone, R., and Duncan, J. (1995). Neural mechanisms of selective visual attention. Annu. Rev. Neurosci. 18, 193–222.
Di Chiara, G. (2002). Nucleus accumbens shell and core dopamine: Differential role in behavior and addiction. Behav. Brain Res. 137, 75–114.
Divac, I. (1975). Magnocellular nuclei of the basal forebrain project to neocortex, brain stem, and olfactory bulb. Review of some functional correlates. Brain Res. 93, 385–398. doi: 10.1016/0006-8993(75)90178-x
Donoghue, J. P. (1987). Cholinergic modulation of sensory responses in cerebral cortex. The role of neuroplasticity in the response to drugs. NIDA Res. Monogr. 78, 169–188.
Doyle, T. G., Berridge, K. C., and Gosnell, B. A. (1993). Morphine enhances hedonic taste palatability in rats. Pharmacol. Biochem. Behav. 46, 745–749.
Eshel, N., Bukwich, M., Rao, V., Hemmelder, V., Tian, J., and Uchida, N. (2015). Arithmetic and local circuitry underlying dopamine prediction errors. Nature 525, 243–246.
Fadel, J., and Burk, J. A. (2010). Orexin/hypocretin modulation of the basal forebrain cholinergic system: Role in attention. Brain Res. 1314, 112–123.
Fallon, J. H., and Moore, R. Y. (1978). Catecholamine innervation of the basal forebrain IV. Topography of the dopamine projection to the basal forebrain and neostriatum. J. Comp. Neurol. 180, 545–579. doi: 10.1002/cne.901800310
Favier, M., Janickova, H., Justo, D., Kljakic, O., Runtz, L., Natsheh, J. Y., et al. (2020). Cholinergic dysfunction in the dorsal striatum promotes habit formation and maladaptive eating. J. Clin. Invest. 130, 6616–6630. doi: 10.1172/JCI138532
Ferenczi, E. A., Zalocusky, K. A., Liston, C., Grosenick, L., Warden, M. R., Amatya, D., et al. (2016). Prefrontal cortical regulation of brainwide circuit dynamics and reward-related behavior. Science 351:aac9698. doi: 10.1126/science.aac9698
Fillinger, C., Yalcin, I., Barrot, M., and Veinante. (2017). Afferents to anterior cingulate areas 24a and 24b and midcingulate areas 24a′ and 24b′ in the mouse. Brain Struct. Funct. 222, 1509–1532.
Fischer, J., and Whitney, D. (2012). Attention gates visual coding in the human pulvinar. Nat. Commun. 3:1051. doi: 10.1038/ncomms2054
Fiserová, M., Consolo, S., and Krsiak, M. (1999). Chronic morphine induces long-lasting changes in acetylcholine release in rat nucleus accumbens core and shell: An in vivo microdialysis study. Psychopharmacology 142, 85–94. doi: 10.1007/s002130050866
Flagel, S. B., Clark, J. J., Robinson, T. E., Mayo, L., Czuj, A., Willuhn, I., et al. (2011). A selective role for dopamine in stimulus–reward learning. Nature 469, 53–57.
Flores-Dourojeanni, J. P., van Rijt, C., van den Munkhof, M. H., Boekhoudt, L., Luijendijk, M. C., Vanderschuren, L. J., et al. (2021). Temporally specific roles of ventral tegmental area projections to the nucleus accumbens and prefrontal cortex in attention and impulse control. J. Neurosci. 41, 4293–4304. doi: 10.1523/JNEUROSCI.0477-20.2020
Forster, G. L., and Blaha, C. D. (2000). Laterodorsal tegmental stimulation elicits dopamine efflux in the rat nucleus accumbens by activation of acetylcholine and glutamate receptors in the ventral tegmental area. Eur. J. Neurosci. 12, 3596–3604. doi: 10.1046/j.1460-9568.2000.00250.x
Frankle, W. G., Laruelle, M., and Haber, S. N. (2006). Prefrontal cortical projections to the midbrain in primates: Evidence for a sparse connection. Neuropsychopharmacology 31, 1627–1636. doi: 10.1038/sj.npp.1300990
Fries, P., Reynolds, J. H., Rorie, A. E., and Desimone, R. (2001). Modulation of oscillatory neuronal synchronization by selective visual attention. Science 291, 1560–1563.
Gallagher, M., McMahan, R. W., and Schoenbaum, G. (1999). Orbitofrontal cortex and representation of incentive value in associative learning. J. Neurosci. 19, 6610–6614.
Gallardo, C. M., Darvas, M., Oviatt, M., Chang, C. H., Michalik, M., Huddy, T. F., et al. (2014). Dopamine receptor 1 neurons in the dorsal striatum regulate food anticipatory circadian activity rhythms in mice. Elife 3:e03781. doi: 10.7554/eLife.03781
Gallo, E. F., Greenwald, J., Yeisley, J., Teboul, E., Martyniuk, K. M., Villarin, J. M., et al. (2022). Dopamine D2 receptors modulate the cholinergic pause and inhibitory learning. Mol. Psychiatry 27, 1502–1514.
Gaykema, R. P., and Zaborszky, L. (1996). Direct catecholaminergic-cholinergic interactions in the basal forebrain. II. Substantia nigra-ventral tegmental area projections to cholinergic neurons. J. Comp. Neurol. 374, 555–577. doi: 10.1002/(SICI)1096-9861(19961028)374:4<555::AID-CNE6>3.0.CO;2-0
Gaykema, R. P., and Zaborszky, L. (1997). Parvalbumin-containing neurons in the basal forebrain receive direct input from the substantia nigra-ventral tegmental area. Brain Res. 747, 173–179. doi: 10.1016/s0006-8993(96)01309-1
Gaykema, R. P., Van Weeghel, R., Hersh, L. B., and Luiten, G. (1991). Prefrontal cortical projections to the cholinergic neurons in the basal forebrain. J. Comp. Neurol. 303, 563–583. doi: 10.1002/cne.903030405
Geisler, S., and Wise, R. A. (2008). Functional implications of glutamatergic projections to the ventral tegmental area. Rev. Neurosci. 19, 227–244. doi: 10.1515/revneuro.2008.19.4-5.227
Gielow, M. R., and Zaborszky, L. (2017). The input-output relationship of the cholinergic basal forebrain. Cell Rep. 18, 1817–1830.
Goller, J., Mitrovic, A., and Leder, H. (2019). Effects of liking on visual attention in faces and paintings. Acta Psychol. 197, 115–123. doi: 10.1016/j.actpsy.2019.05.008
Gotti, C., and Clementi, F. (2004). Neuronal nicotinic receptors: From structure to pathology. Prog. Neurobiol. 74, 363–396.
Grasing, K. (2016). A threshold model for opposing actions of acetylcholine on reward behavior: Molecular mechanisms and implications for treatment of substance abuse disorders. Behav. Brain Res. 312, 148–162. doi: 10.1016/j.bbr.2016.06.022
Graybiel, A. M., Aosaki, T., Flaherty, A. W., and Kimura, M. (1994). The basal ganglia and adaptive motor control. Science 265, 1826–1831.
Gremel, C. M., Chancey, J. H., Atwood, B. K., Luo, G., Neve, R., Ramakrishnan, C., et al. (2016). Endocannabinoid modulation of orbitostriatal circuits gates habit formation. Neuron 90, 1312–1324. doi: 10.1016/j.neuron.2016.04.043
Gritton, H. J., Howe, W. M., Mallory, C. S., Hetrick, V. L., Berke, J. D., and Sarter, M. (2016). Cortical cholinergic signaling controls the detection of cues. Proc. Natl. Acad. Sci. U.S.A. 113, E1089–E1097.
Gritton, H. J., Howe, W. M., Romano, M. F., DiFeliceantonio, A. G., Kramer, M. A., Saligrama, V., et al. (2019). Unique contributions of parvalbumin and cholinergic interneurons in organizing striatal networks during movement. Nat. Neurosci. 22, 586–597. doi: 10.1038/s41593-019-0341-3
Guillem, K., and Ahmed, S. H. (2018). Preference for cocaine is represented in the orbitofrontal cortex by an increased proportion of cocaine use-coding neurons. Cereb. Cortex 28, 819–832. doi: 10.1093/cercor/bhw398
Guillem, K., Bloem, B., Poorthuis, R. B., Loos, M., Smit, A. B., Maskos, U., et al. (2011). Nicotinic acetylcholine receptor β2 subunits in the medial prefrontal cortex control attention. Science 333, 888–891.
Guo, Q., Wang, D., He, X., Feng, Q., Lin, R., Xu, F., et al. (2015). Whole-brain mapping of inputs to projection neurons and cholinergic interneurons in the dorsal striatum. PLoS One 10:e0123381. doi: 10.1371/journal.pone.0123381
Hamid, A. A., Frank, M. J., and Moore, C. I. (2021). Wave-like dopamine dynamics as a mechanism for spatiotemporal credit assignment. Cell 184, 2733–2749. doi: 10.1016/j.cell.2021.03.046
Hamid, A. A., Pettibone, J. R., Mabrouk, O. S., Hetrick, V. L., Schmidt, R., Vander Weele, C. M., et al. (2016). Mesolimbic dopamine signals the value of work. Nat. Neurosci. 19, 117–126.
Hangya, B., Ranade, S. P., Lorenc, M., and Kepecs, A. (2015). Central cholinergic neurons are rapidly recruited by reinforcement feedback. Cell 162, 1155–1168. doi: 10.1016/j.cell.2015.07.057
Hasselmo, M. E. (2006). The role of acetylcholine in learning and memory. Curr. Opin. Neurobiol. 16, 710–715.
Hasselmo, M. E., and Bower, J. M. (1992). Cholinergic suppression specific to intrinsic not afferent fiber synapses in rat piriform (olfactory) cortex. J. Neurophysiol. 67, 1222–1229.
Hasselmo, M. E., and Cekic, M. (1996). Suppression of synaptic transmission may allow combination of associative feedback and self-organizing feedforward connections in the neocortex. Behav. Brain Res. 79, 153–161. doi: 10.1016/0166-4328(96)00010-1
Hasselmo, M. E., and Sarter, M. (2011). Modes and models of forebrain cholinergic neuromodulation of cognition. Neuropsychopharmacology 36, 52–73.
Hayden, B. Y., Heilbronner, S. R., Pearson, J. M., and Platt, M. L. (2011). Surprise signals in anterior cingulate cortex: Neuronal encoding of unsigned reward prediction errors driving adjustment in behavior. J. Neurosci. 31, 4178–4187. doi: 10.1523/JNEUROSCI.4652-10.2011
Hebb, C. O., and Silver, A. (1961). Gradient of cholinesterase activity and of choline acetylase activity in nerve fibres: Gradient of choline acetylase activity. Nature 189, 123–125.
Hedreen, J. C., Bacon, S. J., Cork, L. C., Kitt, C. A., Crawford, G. D., Salvterra, M., et al. (1983). Immunocytochemical identification of cholinergic neurons in the monkey central nervous system using monoclonal antibodies against choline acetyltransferase. Neurosci. Lett. 43, 173–177. doi: 10.1016/0304-3940(83)90183-0
Herrero, J. L., Roberts, M. J., Delicato, L. S., Gieselmann, M. A., Dayan, and Thiele, A. (2008). Acetylcholine contributes through muscarinic receptors to attentional modulation in V1. Nature 454, 1110–1114.
Hillman, K. L., and Bilkey, D. K. (2010). Neurons in the rat anterior cingulate cortex dynamically encode cost–benefit in a spatial decision-making task. J. Neurosci. 30, 7705–7713. doi: 10.1523/JNEUROSCI.1273-10.2010
Holland, C., and Gallagher, M. (2003). Double dissociation of the effects of lesions of basolateral and central amygdala on conditioned stimulus-potentiated feeding and Pavlovian-instrumental transfer. Eur. J. Neurosci. 17, 1680–1694. doi: 10.1046/j.1460-9568.2003.02585.x
Hong, S., and Hikosaka, O. (2014). Pedunculopontine tegmental nucleus neurons provide reward, sensorimotor, and alerting signals to midbrain dopamine neurons. Neuroscience 282, 139–155. doi: 10.1016/j.neuroscience.2014.07.002
Howe, W. M., Berry, A. S., Francois, J., Gilmour, G., Carp, J. M., Tricklebank, M., et al. (2013). Prefrontal cholinergic mechanisms instigating shifts from monitoring for cues to cue-guided performance: Converging electrochemical and fMRI evidence from rats and humans. J. Neurosci. 33, 8742–8752. doi: 10.1523/JNEUROSCI.5809-12.2013
Howe, W. M., Brooks, J. L., Tierney, L., Pang, J., Rossi, A., Young, D., et al. (2018). α5 nAChR modulation of the prefrontal cortex makes attention resilient. Brain Struct. Funct. 223, 1035–1047.
Howe, W. M., Gritton, H. J., Lusk, N. A., Roberts, E. A., Hetrick, V. L., Berke, J. D., et al. (2017). Acetylcholine release in prefrontal cortex promotes gamma oscillations and theta–gamma coupling during cue detection. J. Neurosci. 37, 3215–3230. doi: 10.1523/JNEUROSCI.2737-16.2017
Howe, W. M., Young, D., Bekheet, G., and Kozak, R. (2016). Nicotinic receptor subtypes differentially modulate glutamate release in the dorsal medial striatum. Neurochem. Int. 100, 30–34. doi: 10.1016/j.neuint.2016.08.009
Hu, R., Jin, S., He, X., Xu, F., and Hu, J. (2016). Whole-brain monosynaptic afferent inputs to basal forebrain cholinergic system. Front. Neuroanat. 10:98. doi: 10.3389/fnana.2016.00098
Huang, Y., Yaple, Z. A., and Yu, R. (2020). Goal-oriented and habitual decisions: Neural signatures of model-based and model-free learning. Neuroimage 215:116834. doi: 10.1016/j.neuroimage.2020.116834
Huang, Z., and Li, S. (2022). Reactivation of learned reward association reduces retroactive interference from new reward learning. J. Exp. Psychol. Learn. Mem. Cogn. 48:213. doi: 10.1037/xlm0000987
Hull, C. L. (1943). Principles of behavior: An introduction to behavior theory. New York, NY: Appleton-Century-Crofts.
Jacob Huff, F., Mickel, S. F., Corkin, S., and Growdon, J. H. (1988). Cognitive functions affected by scopolamine in Alzheimer’s disease and normal aging. Drug Dev. Res. 12, 271–278. doi: 10.1159/000072883
Jo, Y. S., Lee, J., and Mizumori, S. J. (2013). Effects of prefrontal cortical inactivation on neural activity in the ventral tegmental area. J. Neurosci. 33, 8159–8171.
Johnston, M. V., McKinney, M., and Coyle, J. T. (1979). Evidence for a cholinergic projection to neocortex from neurons in basal forebrain. Proc. Natl. Acad. Sci. U.S.A. 76, 5392–5396.
Jones, B. E., and Cuello, A. C. (1989). Afferents to the basal forebrain cholinergic cell area from pontomesencephalic—catecholamine, serotonin, and acetylcholine—neurons. Neuroscience 31, 37–61. doi: 10.1016/0306-4522(89)90029-8
Jongen-Rêlo, A. L., Voorn, H., and Groenewegen, H. J. (1994). Immunohistochemical characterization of the shell and core territories of the nucleus accumbens in the rat. Eur. J. Neurosci. 6, 1255–1264.
Joslyn, G., Brush, G., Robertson, M., Smith, T. L., Kalmijn, J., Schuckit, M., et al. (2008). Chromosome 15q25. 1 genetic markers associated with level of response to alcohol in humans. Proc. Natl. Acad. Sci. U.S.A. 105, 20368–20373. doi: 10.1073/pnas.0810970105
Jourdain, A. (1988). Studies on the collateralization of some basal forebrain and mesopontine tegmental projection systems in the rat. Ph.D. thesis. Vancouver, BC: University of British Columbia.
Kahn, J. B., Ward, R. D., Kahn, L. W., Rudy, N. M., Kandel, E. R., Balsam, D., et al. (2012). Medial prefrontal lesions in mice impair sustained attention but spare maintenance of information in working memory. Learn. Mem. 19, 513–517. doi: 10.1101/lm.026302.112
Kahneman, D. (2003). Maps of bounded rationality: Psychology for behavioral economics. Am. Econ. Rev. 93, 1449–1475. doi: 10.3758/s13423-016-1198-z
Kaskan, M., Nicholas, M. A., Dean, A. M., and Murray, E. A. (2022). Attention to stimuli of learned versus innate biological value relies on separate neural systems. J. Neurosci. 42, 9242–9252. doi: 10.1523/JNEUROSCI.0925-22.2022
Kim, S., and Lee, D. (2011). Prefrontal cortex and impulsive decision making. Biol. Psychiatry 69, 1140–1146.
Kitt, C. A., Mitchell, S. J., DeLong, M. R., Wainer, B. H., and Price, D. L. (1987). Fiber pathways of basal forebrain cholinergic neurons in monkeys. Brain Res. 406, 192–206.
Klug, J. R., Engelhardt, M. D., Cadman, C. N., Li, H., Smith, J. B., Ayala, S., et al. (2018). Differential inputs to striatal cholinergic and parvalbumin interneurons imply functional distinctions. Elife 7:e35657. doi: 10.7554/eLife.35657
Kobayashi, Y., and Okada, K. I. (2007). Reward prediction error computation in the pedunculopontine tegmental nucleus neurons. Ann. N. Y. Acad. Sci. 1104, 310–323.
Kosillo, P., Zhang, Y. F., Threlfell, S., and Cragg, S. J. (2016). Cortical control of striatal dopamine transmission via striatal cholinergic interneurons. Cereb. Cortex 26, 4160–4169.
Kruse, A. C., Kobilka, B. K., Gautam, D., Sexton, M., Christopoulos, A., and Wess, J. (2014). Muscarinic acetylcholine receptors: Novel opportunities for drug development. Nat. Rev. Drug Discov. 13, 549–560.
Kulkarni, B., Bentley, D. E., Elliott, R., Youell, A., Watson, A., Derbyshire, S. W. G., et al. (2005). Attention to pain localization and unpleasantness discriminates the functions of the medial and lateral pain systems. Eur. J. Neurosci. 21, 3133–3142. doi: 10.1111/j.1460-9568.2005.04098.x
Laubach, M., Amarante, L. M., Swanson, K., and White, S. R. (2018). What, if anything, is rodent prefrontal cortex? Eneuro 5.
Laurent, V., Bertran-Gonzalez, J., Chieng, B. C., and Balleine, B. W. (2014). δ-opioid and dopaminergic processes in accumbens shell modulate the cholinergic control of predictive learning and choice. J. Neurosci. 34, 1358–1369. doi: 10.1523/JNEUROSCI.4592-13.2014
Lavie, N. (2005). Distracted and confused?: Selective attention under load. Trends cogn. Sci. 9, 75–82.
Lavie, N. (2010). Attention, distraction, and cognitive control under load. Curr. Dir. Psychol. Sci. 19, 143–148.
Lavie, N., Hirst, A., De Fockert, J. W., and Viding, E. (2004). Load theory of selective attention and cognitive control. J. Exp. Psychol. Gen. 133:339.
Lavoie, B., Smith, Y., and Parent, A. (1989). Dopaminergic innervation of the basal ganglia in the squirrel monkey as revealed by tyrosine hydroxylase immunohistochemistry. J. Comp. Neurol. 289, 36–52.
Leech, R., and Sharp, D. J. (2014). The role of the posterior cingulate cortex in cognition and disease. Brain 137, 12–32.
Lehmann, J., Nagy, J. I., Atmadja, S., and Fibiger, H. C. (1980). The nucleus Basalis magnocellularis: The origin of a cholinergic projection to the neocortex of the rat. Neuroscience 5, 1161–1174. doi: 10.1016/0306-4522(80)90195-5
Leonard, S., and Bertrand, D. (2001). Neuronal nicotinic receptors: From structure to function. Nicot. Tobacco Res. 3, 203–223.
Lerner, T. N., Holloway, A. L., and Seiler, J. L. (2021). Dopamine, updated: Reward prediction error and beyond. Curr. Opin. Neurobiol. 67, 123–130.
Li, L., Gratton, C., Yao, D., and Knight, R. T. (2010). Role of frontal and parietal cortices in the control of bottom-up and top-down attention in humans. Brain Res. 1344, 173–184.
Li, Z., Chen, Z., Fan, G., Li, A., Yuan, J., and Xu, T. (2018). Cell-type-specific afferent innervation of the nucleus accumbens core and shell. Front. Neuroanat. 12:84. doi: 10.3389/fnana.2018.00084
Lichtenberg, N. T., Sepe-Forrest, L., Pennington, Z. T., Lamparelli, A. C., Greenfield, V. Y., and Wassum, K. M. (2021). The medial orbitofrontal cortex–basolateral amygdala circuit regulates the influence of reward cues on adaptive behavior and choice. J. Neurosci. 41, 7267–7277. doi: 10.1523/JNEUROSCI.0901-21.2021
Liu, X., Gao, S., Zhang, N., Jin, T., Sun, X., Luan, X., et al. (2020). The orexinergic neural pathway from the lateral hypothalamus to the nucleus accumbens and its regulation of palatable food intake. Neuropeptides 80:102028. doi: 10.1016/j.npep.2020.102028
Loewi, O. (1921). Über humorale übertragbarkeit der Herznervenwirkung. Pflügers Arch. Eur. J. Physiol. 189, 239–242.
Lu, Y., Sarter, M., Zochowski, M., and Booth, V. (2020). Phasic cholinergic signaling promotes emergence of local gamma rhythms in excitatory–inhibitory networks. Eur. J. Neurosci. 52, 3545–3560. doi: 10.1111/ejn.14744
Lubman, D. I., Peters, L. A., Mogg, K., Bradley, B. P., and Deakin, J. F. W. (2000). Attentional bias for drug cues in opiate dependence. Psychol. Med. 30, 169–175.
Ma, W., Höhmann, C. F., Coyle, J. T., and Juliano, S. L. (1989). Lesions of the basal forebrain alter stimulus-evoked metabolic activity in mouse somatosensory cortex. J. Comp. Neurol. 288, 414–427. doi: 10.1002/cne.902880305
Macintosh, F. C. (1941). The distribution of acetylcholine in the peripheral and the central nervous system. J. Physiol. 99:436.
Málková, L., Gaffan, D., and Murray, E. A. (1997). Excitotoxic lesions of the amygdala fail to produce impairment in visual learning for auditory secondary reinforcement but interfere with reinforcer devaluation effects in rhesus monkeys. J. Neurosci. 17, 6011–6020. doi: 10.1523/JNEUROSCI.17-15-06011.1997
Malvaez, M., Shieh, C., Murphy, M. D., Greenfield, V. Y., and Wassum, K. M. (2019). Distinct cortical–amygdala projections drive reward value encoding and retrieval. Nat. Neurosci. 22, 762–769. doi: 10.1038/s41593-019-0374-7
Mandel, R. J., Gage, F. H., and Thal, L. J. (1989). Enhanced detection of nucleus basalis magnocellularis lesion-induced spatial learning deficit in rats by modification of training regimen. Behav. Brain Res. 31, 221–229. doi: 10.1016/0166-4328(89)90004-1
Marucci, G., Buccioni, M., Dal Ben, D., Lambertucci, C., Volpini, R., and Amenta, F. (2021). Efficacy of acetylcholinesterase inhibitors in Alzheimer’s disease. Neuropharmacology 190:108352.
Matamales, M., Götz, J., and Bertran-Gonzalez, J. (2016). Quantitative imaging of cholinergic interneurons reveals a distinctive spatial organization and a functional gradient across the mouse striatum. PLoS One 11:e0157682.
McAlonan, K., Brown, V. J., and Bowman, E. M. (2000). Thalamic reticular nucleus activation reflects attentional gating during classical conditioning. J. Neurosci. 20, 8897–8901. doi: 10.1523/JNEUROSCI.20-23-08897.2000
McGaughy, J., Kaiser, T., and Sarter, M. (1996). Behavioral vigilance following infusions of 192 IgG-saporin into the basal forebrain: Selectivity of the behavioral impairment and relation to cortical AChE-positive fiber density. Behav. Neurosci. 110:247. doi: 10.1037//0735-7044.110.2.247
Mena-Segovia, J., and Bolam, J. P. (2017). Rethinking the pedunculopontine nucleus: From cellular organization to function. Neuron 94, 7–18. doi: 10.1016/j.neuron.2017.02.027
Mesulam, M. M. (1981). A cortical network for directed attention and unilateral neglect. Ann. Neurol. 10, 309–325. doi: 10.1002/ana.410100402
Mesulam, M. M. (1996). The systems-level organization of cholinergic innervation in the human cerebral cortex and its alterations in Alzheimer’s disease. Prog. Brain Res. 109, 285–297. doi: 10.1016/s0079-6123(08)62112-3
Mesulam, M. M., and Mufson, E. J. (1984). Neural inputs into the nucleus basalis of the Substantia innominata (Ch4) in the rhesus monkey. Brain 107, 253–274. doi: 10.1093/brain/107.1.253
Mesulam, M. M., and Van Hoesen, G. W. (1976). Acetylcholinesterase-rich projections from the basal forebrain of the rhesus monkey to neocortex. Brain Res. 109, 152–157. doi: 10.1016/0006-8993(76)90385-1
Mesulam, M. M., Mufson, E. J., Levey, A. I., and Wainer, B. H. (1983). Cholinergic innervation of cortex by the basal forebrain: Cytochemistry and cortical connections of the septal area, diagonal band nuclei, nucleus basalis (Substantia innominata), and hypothalamus in the rhesus monkey. J. Comp. Neurol. 214, 170–197. doi: 10.1002/cne.902140206
Metherate, R., and Ashe, J. H. (1991). Basal forebrain stimulation modifies auditory cortex responsiveness by an action at muscarinic receptors. Brain Res. 559, 163–167. doi: 10.1016/0006-8993(91)90301-b
Metherate, R., and Weinberger, N. M. (1990). Cholinergic modulation of responses to single tones produces tone-specific receptive field alterations in cat auditory cortex. Synapse 6, 133–145. doi: 10.1002/syn.890060204
Meyer, J., Lovic, V., Saunders, B. T., Yager, L. M., Flagel, S. B., Morrow, J. D., et al. (2012). Quantifying individual variation in the propensity to attribute incentive salience to reward cues. PLoS One 7:e38987. doi: 10.1371/journal.pone.0038987
Mineur, Y. S., Cahuzac, E. L., Mose, T. N., Bentham, M. P., Plantenga, M. E., Thompson, D. C., et al. (2018). Interaction between noradrenergic and cholinergic signaling in amygdala regulates anxiety-and depression-related behaviors in mice. Neuropsychopharmacology 43, 2118–2125. doi: 10.1038/s41386-018-0024-x
Mineur, Y. S., Fote, G. M., Blakeman, S., Cahuzac, E. L., Newbold, S. A., and Picciotto, M. R. (2016). Multiple nicotinic acetylcholine receptor subtypes in the mouse amygdala regulate affective behaviors and response to social stress. Neuropsychopharmacology 41, 1579–1587. doi: 10.1038/npp.2015.316
Mineur, Y. S., Mose, T. N., Maibom, K. L., Pittenger, S. T., Soares, A. R., Wu, H., et al. (2022). ACh signaling modulates activity of the GABAergic signaling network in the basolateral amygdala and behavior in stress-relevant paradigms. Mol. Psychiatry 27, 4918–4927. doi: 10.1038/s41380-022-01749-7
Mohebi, A., Collins, V. L., and Berke, J. D. (2023). Accumbens cholinergic interneurons dynamically promote dopamine release and enable motivation. Elife 12:e85011.
Mohebi, A., Pettibone, J. R., Hamid, A. A., Wong, J. M. T., Vinson, L. T., Patriarchi, T., et al. (2019). Dissociable dopamine dynamics for learning and motivation. Nature 570, 65–70.
Montague, R., and Berns, G. S. (2002). Neural economics and the biological substrates of valuation. Neuron 36, 265–284.
Montague, R., Dayan, P., and Sejnowski, T. J. (1996). A framework for mesencephalic dopamine systems based on predictive Hebbian learning. J. Neurosci. 16, 1936–1947.
Moore, H., Fadel, J., Sarter, M., and Bruno, J. P. (1999). Role of accumbens and cortical dopamine receptors in the regulation of cortical acetylcholine release. Neuroscience 88, 811–822.
Nagahama, Y., Okada, T., Katsumi, Y., Hayashi, T., Yamauchi, H., Oyanagi, C., et al. (2001). Dissociable mechanisms of attentional control within the human prefrontal cortex. Cereb. Cortex 11, 85–92.
Noguer-Calabús, I., Schäble, S., and Kalenscher, T. (2022). Lesions of nucleus accumbens shell abolish socially transmitted food preferences. Eur. J. Neurosci. 56, 5795–5809. doi: 10.1111/ejn.15827
Oakman, S. A., Faris, L., Kerr, E., Cozzari, C., and Hartman, B. K. (1995). Distribution of pontomesencephalic cholinergic neurons projecting to Substantia nigra differs significantly from those projecting to ventral tegmental area. J. Neurosci. 15, 5859–5869. doi: 10.1523/JNEUROSCI.15-09-05859.1995
Obermayer, J., Luchicchi, A., Heistek, T. S., de Kloet, S. F., Terra, H., Bruinsma, B., et al. (2019). Prefrontal cortical ChAT-VIP interneurons provide local excitation by cholinergic synaptic transmission and control attention. Nat. Commun. 10:5280.
Obermayer, J., Verhoog, M. B., Luchicchi, A., and Mansvelder, H. D. (2017). Cholinergic modulation of cortical microcircuits is layer-specific: Evidence from rodent, monkey and human brain. Front. Neural Circuits 11:100. doi: 10.3389/fncir.2017.00100
O’Doherty, J. P., Cockburn, J., and Pauli, W. M. (2017). Learning, reward, and decision making. Annu. Rev. Psychol. 68, 73–100.
Oldenburg, I. A., and Ding, J. B. (2011). Cholinergic modulation of synaptic integration and dendritic excitability in the striatum. Curr. Opin. Neurobiol. 21, 425–432.
Olds, J., and Milner, P. (1954). Positive reinforcement produced by electrical stimulation of septal area and other regions of rat brain. J. Comp. Physiol. Psychol. 47:419. doi: 10.1037/h0058775
Ostlund, S. B., and Balleine, B. W. (2007). The contribution of orbitofrontal cortex to action selection. Ann. N. Y. Acad. Sci. 1121, 174–192.
Palmiter, R. D. (2008). Dopamine signaling in the dorsal striatum is essential for motivated behaviors: Lessons from dopamine-deficient mice. Ann. N. Y. Acad. Sci. 1129, 35–46. doi: 10.1196/annals.1417.003
Pan, W. X., and Hyland, B. I. (2005). Pedunculopontine tegmental nucleus controls conditioned responses of midbrain dopamine neurons in behaving rats. J. Neurosci. 25, 4725–4732. doi: 10.1523/JNEUROSCI.0277-05.2005
Paolone, G., Angelakos, C. C., Meyer, J., Robinson, T. E., and Sarter, M. (2013). Cholinergic control over attention in rats prone to attribute incentive salience to reward cues. J. Neurosci. 33, 8321–8335.
Paolone, G., Howe, W. M., Gopalakrishnan, M., Decker, M. W., and Sarter, M. (2010). “Regulation and function of the tonic component of cortical acetylcholine release,” in Monitoring molecules in neuroscience, eds Y. Michotte, B. Westerink, and S. Sarre (Brussels: Vrije Universiteit Brussels), 363–365.
Parikh, V., and Sarter, M. (2008). Cholinergic mediation of attention: Contributions of phasic and tonic increases in prefrontal cholinergic activity. Ann. N. Y. Acad. Sci. 1129, 225–235. doi: 10.1196/annals.1417.021
Parikh, V., Ji, J., Decker, M. W., and Sarter, M. (2010). Prefrontal β2 subunit-containing and α7 nicotinic acetylcholine receptors differentially control glutamatergic and cholinergic signaling. J. Neurosci. 30, 3518–3530.
Parikh, V., Kozak, R., Martinez, V., and Sarter, M. (2007). Prefrontal acetylcholine release controls cue detection on multiple timescales. Neuron 56, 141–154. doi: 10.1016/j.neuron.2007.08.025
Parikh, V., Man, K., Decker, M. W., and Sarter, M. (2008). Glutamatergic contributions to nicotinic acetylcholine receptor agonist-evoked cholinergic transients in the prefrontal cortex. J. Neurosci. 28, 3769–3780.
Parikh, V., Pomerleau, F., Huettl, Gerhardt, G. A., Sarter, M., and Bruno, J. P. (2004). Rapid assessment of in vivo cholinergic transmission by amperometric detection of changes in extracellular choline levels. Eur. J. Neurosci. 20, 1545–1554. doi: 10.1111/j.1460-9568.2004.03614.x
Pecina, S., Cagniard, B., Berridge, K. C., Aldridge, J. W., and Zhuang, X. (2003). Hyperdopaminergic mutant mice have higher “wanting” but not “liking” for sweet rewards. J. Neurosci. 23, 9395–9402. doi: 10.1523/JNEUROSCI.23-28-09395.2003
Perry, D. C., Xiao, Y., Nguyen, H. N., Musachio, J. L., Dávila-García, M. I., and Kellar, K. J. (2002). Measuring nicotinic receptors with characteristics of α4β2, α3β2 and α3β4 subtypes in rat tissues by autoradiography. J. Neurochem. 82, 468–481.
Perry, M. L., Baldo, B. A., Andrzejewski, M. E., and Kelley, A. E. (2009). Muscarinic receptor antagonism causes a functional alteration in nucleus accumbens μ-opiate-mediated feeding behavior. Behav. Brain Res. 197, 225–229. doi: 10.1016/j.bbr.2008.08.002
Peters, M. S., Demeter, E., Lustig, C., Bruno, J. P., and Sarter, M. (2011). Enhanced control of attention by stimulating mesolimbic–corticopetal cholinergic circuitry. J. Neurosci. 31, 9760–9771. doi: 10.1523/JNEUROSCI.1902-11.2011
Phillips, K. B., and Sarter, M. (2020). Addiction vulnerability and the processing of significant cues: Sign-, but not goal-, tracker perceptual sensitivity relies on cue salience. Behav. Neurosci. 134:133. doi: 10.1037/bne0000353
Pinault, D. (2004). The thalamic reticular nucleus: Structure, function and concept. Brain Res. Rev. 46, 1–31.
Pine, A., Shiner, T., Seymour, B., and Dolan, R. J. (2010). Dopamine, time, and impulsivity in humans. J. Neurosci. 30, 8888–8896.
Pisani, A., Bernardi, G., Ding, J., and Surmeier, D. J. (2007). Re-emergence of striatal cholinergic interneurons in movement disorders. Trends Neurosci. 30, 545–553. doi: 10.1016/j.tins.2007.07.008
Poh, J. H., Massar, S. A., Jamaluddin, S. A., and Chee, M. W. (2019). Reward supports flexible orienting of attention to category information and influences subsequent memory. Psychon. Bull. Rev. 26, 559–568. doi: 10.3758/s13423-019-01595-9
Poorthuis, R. B., Bloem, B., Schak, B., Wester, J., de Kock, C. P., and Mansvelder, H. D. (2013). Layer-specific modulation of the prefrontal cortex by nicotinic acetylcholine receptors. Cereb. Cortex 23, 148–161. doi: 10.1093/cercor/bhr390
Posner, M. I., and Petersen, S. E. (1990). The attention system of the human brain. Annu. Rev. Neurosci. 13, 25–42.
Posner, M. I., Snyder, C. R., and Davidson, B. J. (1980). Attention and the detection of signals. J. Exp. Psychol. Gen. 109:160.
Poulin, J. F., Caronia, G., Hofer, C., Cui, Q., Helm, B., Ramakrishnan, C., et al. (2018). Mapping projections of molecularly defined dopamine neuron subtypes using intersectional genetic approaches. Nat. Neurosci. 21, 1260–1271. doi: 10.1038/s41593-018-0203-4
Preuss, T. M., and Wise, S. P. (2022). Evolution of prefrontal cortex. Neuropsychopharmacology 47, 3–19.
Raghanti, M. A., Stimpson, C. D., Marcinkiewicz, J. L., Erwin, J. M., Hof, R., and Sherwood, C. C. (2008). Cholinergic innervation of the frontal cortex: Differences among humans, chimpanzees, and macaque monkeys. J. Comp. Neurol. 506, 409–424. doi: 10.1002/cne.21546
Ragozzino, M. E. (2003). Acetylcholine actions in the dorsomedial striatum support the flexible shifting of response patterns. Neurobiol. Learn. Mem. 80, 257–267. doi: 10.1016/s1074-7427(03)00077-7
Ragozzino, M. E., Mohler, E. G., Prior, M., Palencia, C. A., and Rozman, S. (2009). Acetylcholine activity in selective striatal regions supports behavioral flexibility. Neurobiol. Learn. Mem. 91, 13–22. doi: 10.1016/j.nlm.2008.09.008
Robinson, S., Sotak, B. N., During, M. J., and Palmiter, R. D. (2006). Local dopamine production in the dorsal striatum restores goal-directed behavior in dopamine-deficient mice. Behav. Neurosci. 120:196. doi: 10.1037/0735-7044.120.1.000
Robinson, T. E., and Berridge, K. C. (1993). The neural basis of drug craving: An incentive-sensitization theory of addiction. Brain Res. Rev. 18, 247–291.
Root, D. H., Barker, D. J., Estrin, D. J., Miranda-Barrientos, J. A., Liu, B., Zhang, S., et al. (2020). Distinct signaling by ventral tegmental area glutamate, GABA, and combinatorial glutamate-GABA neurons in motivated behavior. Cell Rep. 32:108094. doi: 10.1016/j.celrep.2020.108094
Ross, R. S., McGaughy, J., and Eichenbaum, H. (2005). Acetylcholine in the orbitofrontal cortex is necessary for the acquisition of a socially transmitted food preference. Learn. Mem. 12, 302–306.
Ruan, Y., Li, K. Y., Zhen, R., Yan, Y. Q., Wang, Z. X., Chen, Y., et al. (2022). Cholinergic neurons in the pedunculopontine nucleus guide reversal learning by signaling the changing reward contingency. Cell Rep. 38:110437. doi: 10.1016/j.celrep.2022.110437
Rudebeck, H., and Murray, E. A. (2014). The orbitofrontal oracle: Cortical mechanisms for the prediction and evaluation of specific behavioral outcomes. Neuron 84, 1143–1156. doi: 10.1016/j.neuron.2014.10.049
Saalmann, Y. B., and Kastner, S. (2015). The cognitive thalamus. Front. Syst. Neurosci. 9:39. doi: 10.3389/fnsys.2015.00039
Saccone, S. F., Hinrichs, A. L., Saccone, N. L., Chase, G. A., Konvicka, K., Madden, A., et al. (2007). Cholinergic nicotinic receptor genes implicated in a nicotine dependence association study targeting 348 candidate genes with 3713 SNPs. Hum. Mol. Genet. 16, 36–49. doi: 10.1093/hmg/ddl438
Saddoris, M. P., Cacciapaglia, F., Wightman, R. M., and Carelli, R. M. (2015). Differential dopamine release dynamics in the nucleus accumbens core and shell reveal complementary signals for error prediction and incentive motivation. J. Neurosci. 35, 11572–11582. doi: 10.1523/JNEUROSCI.2344-15.2015
Sakurai, T., Amemiya, A., Ishii, M., Matsuzaki, I., Chemelli, R. M., Tanaka, H., et al. (1998). Orexins and orexin receptors: A family of hypothalamic neuropeptides and G protein-coupled receptors that regulate feeding behavior. Cell 92, 573–585.
Santiago, M. P., and Potter, L. T. (2001). Biotinylated m4-toxin demonstrates more M4 muscarinic receptor protein on direct than indirect striatal projection neurons. Brain Res. 894, 12–20. doi: 10.1016/s0006-8993(00)03170-x
Sarter, M., and Phillips, K. B. (2018). The neuroscience of cognitive-motivational styles: Sign-and goal-trackers as animal models. Behav. Neurosci. 132:1. doi: 10.1037/bne0000226
Sarter, M., Gehring, W. J., and Kozak, R. (2006). More attention must be paid: The neurobiology of attentional effort. Brain Res. Rev. 51, 145–160.
Sarter, M., Parikh, V., and Howe, W. M. (2009). Phasic acetylcholine release and the volume transmission hypothesis: Time to move on. Nat. Rev. Neurosci. 10, 383–390. doi: 10.1038/nrn2635
Satoh, K., and Fibiger, H. C. (1986). Cholinergic neurons of the laterodorsal tegmental nucleus: Efferent and afferent connections. J. Comp. Neurol. 253, 277–302.
Saunders, B. T., and Robinson, T. E. (2010). A cocaine cue acts as an incentive stimulus in some but not others: Implications for addiction. Biol. Psychiatry 67, 730–736.
Saunders, B. T., and Robinson, T. E. (2012). The role of dopamine in the accumbens core in the expression of Pavlovian-conditioned responses. Eur. J. Neurosci. 36, 2521–2532.
Saunders, B. T., Richard, J. M., Margolis, E. B., and Janak, H. (2018). Dopamine neurons create Pavlovian conditioned stimuli with circuit-defined motivational properties. Nat. Neurosci. 21, 1072–1083. doi: 10.1038/s41593-018-0191-4
Saunders, B. T., Yager, L. M., and Robinson, T. E. (2013). Cue-evoked cocaine “craving”: Role of dopamine in the accumbens core. J. Neurosci. 33, 13989–14000.
Schilman, E. A., Uylings, H. B., Galis-de Graaf, Y., Joel, D., and Groenewegen, H. J. (2008). The orbital cortex in rats topographically projects to central parts of the caudate–putamen complex. Neurosci. Lett. 432, 40–45. doi: 10.1016/j.neulet.2007.12.024
Schultz, W., Dayan, P., and Montague, R. (1997). A neural substrate of prediction and reward. Science 275, 1593–1599.
Shimo, Y., and Hikosaka, O. (2001). Role of tonically active neurons in primate caudate in reward-oriented saccadic eye movement. J. Neurosci. 21, 7804–7814. doi: 10.1523/JNEUROSCI.21-19-07804.2001
Shin, J. H., Adrover, M. F., and Alvarez, V. A. (2017). Distinctive modulation of dopamine release in the nucleus accumbens shell mediated by dopamine and acetylcholine receptors. J. Neurosci. 37, 11166–11180. doi: 10.1523/JNEUROSCI.0596-17.2017
Shin, J. H., Adrover, M. F., Wess, J., and Alvarez, V. A. (2015). Muscarinic regulation of dopamine and glutamate transmission in the nucleus accumbens. Proc. Natl. Acad. Sci. U.S.A. 112, 8124–8129.
Sias, A. C., Morse, A. K., Wang, S., Greenfield, V. Y., Goodpaster, C. M., Wrenn, T. M., et al. (2021). A bidirectional corticoamygdala circuit for the encoding and retrieval of detailed reward memories. Elife 10:e68617. doi: 10.7554/eLife.68617
Simon, N. W., Beas, B. S., Montgomery, K. S., Haberman, R. P., Bizon, J. L., and Setlow, B. (2013). Prefrontal cortical–striatal dopamine receptor m RNA expression predicts distinct forms of impulsivity. Eur. J. Neurosci. 37, 1779–1788. doi: 10.1111/ejn.12191
Sizer, S. E., Price, M. E., Parrish, B. C., Barth, S. H., Heaney, C. F., Raab-Graham, K. F., et al. (2022). Chronic intermittent ethanol exposure dysregulates nucleus basalis magnocellularis afferents in the basolateral amygdala. Eneuro 9.
Skvortsova, V., Palminteri, S., Buot, A., Karachi, C., Welter, M. L., Grabli, D., et al. (2021). A causal role for the pedunculopontine nucleus in human instrumental learning. Curr. Biol. 31, 943–954. doi: 10.1016/j.cub.2020.11.042
Stalnaker, T. A., Roesch, M. R., Franz, T. M., Burke, K. A., and Schoenbaum, G. (2006). Abnormal associative encoding in orbitofrontal neurons in cocaine-experienced rats during decision-making. Eur. J. Neurosci. 24, 2643–2653. doi: 10.1111/j.1460-9568.2006.05128.x
Steinberg, E. E., Gore, F., Heifets, B. D., Taylor, M. D., Norville, Z. C., Beier, K. T., et al. (2020). Amygdala-midbrain connections modulate appetitive and aversive learning. Neuron 106, 1026–1043. doi: 10.1016/j.neuron.2020.03.016
Surmeier, D. J., Ding, J., Day, M., Wang, Z., and Shen, W. (2007). D1 and D2 dopamine-receptor modulation of striatal glutamatergic signaling in striatal medium spiny neurons. Trends Neurosci. 30, 228–235.
Swanson, L. W. N. (1982). The projections of the ventral tegmental area and adjacent regions: A combined fluorescent retrograde tracer and immunofluorescence study in the rat. Brain Res. Bull. 9, 321–353. doi: 10.1016/0361-9230(82)90145-9
Talmi, D., Seymour, B., Dayan, and Dolan, R. J. (2008). Human Pavlovian–instrumental transfer. J. Neurosci. 28, 360–368.
Threlfell, S., Lalic, T., Platt, N. J., Jennings, K. A., Deisseroth, K., and Cragg, S. J. (2012). Striatal dopamine release is triggered by synchronized activity in cholinergic interneurons. Neuron 75, 58–64.
Totah, N. K., Jackson, M. E., and Moghaddam, B. (2013). Preparatory attention relies on dynamic interactions between prelimbic cortex and anterior cingulate cortex. Cereb. Cortex 23, 729–738. doi: 10.1093/cercor/bhs057
Totah, N. K., Kim, Y. B., Homayoun, H., and Moghaddam, B. (2009). Anterior cingulate neurons represent errors and preparatory attention within the same behavioral sequence. J. Neurosci. 29, 6418–6426. doi: 10.1523/JNEUROSCI.1142-09.2009
Tremblay, N., Warren, R. A., and Dykes, R. W. (1990a). Electrophysiological studies of acetylcholine and the role of the basal forebrain in the somatosensory cortex of the cat. I. Cortical neurons excited by glutamate. J. Neurophysiol. 64, 1199–1211. doi: 10.1152/jn.1990.64.4.1199
Tremblay, N., Warren, R. A., and Dykes, R. W. (1990b). Electrophysiological studies of acetylcholine and the role of the basal forebrain in the somatosensory cortex of the cat. II. Cortical neurons excited by somatic stimuli. J. Neurophysiol. 64, 1212–1222.
Turchi, J., and Sarter, M. (1997). Cortical acetylcholine and processing capacity: Effects of cortical cholinergic deafferentation on crossmodal divided attention in rats. Cogn. Brain Res. 6, 147–158. doi: 10.1016/s0926-6410(97)00027-x
van Heukelum, S., Mars, R. B., Guthrie, M., Buitelaar, J. K., Beckmann, C. F., Tiesinga, H., et al. (2020). Where is cingulate cortex? A cross-species view. Trends Neurosci. 43, 285–299. doi: 10.1016/j.tins.2020.03.007
Villano, I., Messina, A., Valenzano, A., Moscatelli, F., Esposito, T., Monda, V., et al. (2017). Basal forebrain cholinergic system and orexin neurons: Effects on attention. Front. Behav. Neurosci. 11:10. doi: 10.3389/fnbeh.2017.00010
Vogt, B. A. (2016). Midcingulate cortex: Structure, connections, homologies, functions and diseases. J. Chem. Neuroanat. 74, 28–46. doi: 10.1016/j.jchemneu.2016.01.010
Vogt, B. A., and Gabriel, M. (1993). Neurobiology of cingulate cortex and limbic thalamus: A comprehensive handbook. Boston, MA: Birkhäuser Boston, 249.
Vogt, B. A., Hof, R., Zilles, K., Vogt, L. J., Herold, C., and Palomero-Gallagher, N. (2013). Cingulate area 32 homologies in mouse, rat, macaque and human: Cytoarchitecture and receptor architecture. J. Comp. Neurol. 521, 4189–4204. doi: 10.1002/cne.23409
Vogt, B. A., Vogt, L., and Laureys, S. (2006). Cytology and functionally correlated circuits of human posterior cingulate areas. Neuroimage 29, 452–466. doi: 10.1016/j.neuroimage.2005.07.048
Von Engelhardt, J., Eliava, M., Meyer, A. H., Rozov, A., and Monyer, H. (2007). Functional characterization of intrinsic cholinergic interneurons in the cortex. J. Neurosci. 27, 5633–5642.
Voorn, P., Docter, G. J., Jongen-Rêlo, A. L., and Jonker, A. J. (1994). Rostrocaudal subregional differences in the response of enkephalin, dynorphin and substance P synthesis in rat nucleus accumbens to dopamine depletion. Eur. J. Neurosci. 6, 486–496. doi: 10.1111/j.1460-9568.1994.tb00291.x
Wakabayashi, K. T., Feja, M., Baindur, A. N., Bruno, M. J., Bhimani, R. V., Park, J., et al. (2019). Chemogenetic activation of ventral tegmental area GABA neurons, but not mesoaccumbal GABA terminals, disrupts responding to reward-predictive cues. Neuropsychopharmacology 44, 372–380.
Wallis, J. D., and Kennerley, S. W. (2010). Heterogeneous reward signals in prefrontal cortex. Curr. Opin. Neurobiol. 20, 191–198.
Walton, M. E., Bannerman, D. M., and Rushworth, M. F. (2002). The role of rat medial frontal cortex in effort-based decision making. J. Neurosci. 22, 10996–11003.
Wang, J., Li, J., Yang, Q., Xie, Y. K., Wen, Y. L., Xu, Z. Z., et al. (2021). Basal forebrain mediates prosocial behavior via disinhibition of midbrain dopamine neurons. Proc. Natl. Acad. Sci. U.S.A. 118:e2019295118. doi: 10.1073/pnas.2019295118
Watson, P., Pearson, D., Theeuwes, J., Most, S. B., and Le Pelley, M. E. (2020). Delayed disengagement of attention from distractors signalling reward. Cognition 195:104125. doi: 10.1016/j.cognition.2019.104125
Weiland, B. J., Heitzeg, M. M., Zald, D., Cummiford, C., Love, T., Zucker, R. A., et al. (2014). Relationship between impulsivity, prefrontal anticipatory activation, and striatal dopamine release during rewarded task performance. Psychiatry Res. Neuroimaging 223, 244–252. doi: 10.1016/j.pscychresns.2014.05.015
Wenk, H., Bigl, V., and Meyer, U. (1980). Cholinergic projections from magnocellular nuclei of the basal forebrain to cortical areas in rats. Brain Res. Rev. 2, 295–316.
Whishaw, I. Q., O’connor, W. T., and Dunnett, S. B. (1985). Disruption of central cholinergic systems in the rat by basal forebrain lesions or atropine: Effects on feeding, sensorimotor behaviour, locomotor activity and spatial navigation. Behav. Brain Res. 17, 103–115.
Whitehouse, J., Price, D. L., Struble, R. G., Clark, A. W., Coyle, J. T., and DeLong, M. R. (1982). Alzheimer’s disease and senile dementia: Loss of neurons in the basal forebrain. Science 215, 1237–1239.
Wilson, C. J., Chang, H. T., and Kitai, S. T. (1990). Firing patterns and synaptic potentials of identified giant aspiny interneurons in the rat neostriatum. J. Neurosci. 10, 508–519. doi: 10.1523/JNEUROSCI.10-02-00508.1990
Winstanley, C. A., Olausson, Taylor, J. R., and Jentsch, J. D. (2010). Insight into the relationship between impulsivity and substance abuse from studies using animal models. Alcoholism Clin. Exp. Res. 34, 1306–1318. doi: 10.1111/j.1530-0277.2010.01215.x
Wise, R. A., Spindler, J., and Legault, L. (1978). Major attenuation of food reward with performance-sparing doses of pimozide in the rat. Can. J. Psychol. 32:77. doi: 10.1037/h0081678
Woolf, N. J., and Butcher, L. L. (1981). Cholinergic neurons in the caudate-putamen complex proper are intrinsically organized: A combined Evans blue and acetylcholinesterase analysis. Brain Res. Bull. 7, 487–507. doi: 10.1016/0361-9230(81)90004-6
Woolf, N. J., and Butcher, L. L. (1986). Cholinergic systems in the rat brain: III. Projections from the Pontomesencephalic tegmentum to the thalamus, tectum, basal ganglia, and basal forebrain. Brain Res. Bull. 16, 603–637. doi: 10.1016/0361-9230(86)90134-6
Woolf, N. J., and Butcher, L. L. (1991). “The cholinergic basal forebrain as a cognitive machine,” in Activation to acquisition: Functional aspects of the basal forebrain cholinergic system, ed. R. T. Richardson (Boston, MA: Birkhäuser Boston), 347–380.
Woolf, N. J., Eckenstein, F., and Butcher, L. L. (1984). Cholinergic systems in the rat brain: I. Projections to the limbic telencephalon. Brain Res. Bull. 13, 751–784. doi: 10.1016/0361-9230(84)90236-3
Xiao, C., Cho, J. R., Zhou, C., Treweek, J. B., Chan, K., McKinney, S. L., et al. (2016). Cholinergic mesopontine signals govern locomotion and reward through dissociable midbrain pathways. Neuron 90, 333–347. doi: 10.1016/j.neuron.2016.03.028
Yan, Z., Flores-Hernandez, J., and Surmeier, D. J. (2001). Coordinated expression of muscarinic receptor messenger RNAs in striatal medium spiny neurons. Neuroscience 103, 1017–1024. doi: 10.1016/s0306-4522(01)00039-2
Yang, T., Wang, D., Chen, X., Liang, Y., Guo, F., Wu, C., et al. (2021). 18F-ASEM imaging for evaluating atherosclerotic plaques linked to α7-nicotinic acetylcholine receptor. Front. Bioeng. Biotechnol. 9:684221. doi: 10.3389/fbioe.2021.684221
Yin, H. H., Ostlund, S. B., Knowlton, B. J., and Balleine, B. W. (2005). The role of the dorsomedial striatum in instrumental conditioning. Eur. J. Neurosci. 22, 513–523.
Yin, T. C. T., and Mountcastle, V. B. (1977). Visual input to the visuomotor mechanisms of the monkey’s parietal lobe. Science 197, 1381–1383. doi: 10.1126/science.408924
Zaborszky, L. (1989). Afferent connections of the forebrain cholinergic projection neurons, with special reference to monoaminergic and peptidergic fibers. EXS 57, 12–32. doi: 10.1007/978-3-0348-9138-7_2
Zaborszky, L., and Cullinan, W. E. (1996). Direct catecholaminergic-cholinergic interactions in the basal forebrain. I. Dopamine−β−hydroxylase-and tyrosine hydroxylase input to cholinergic neurons. J. Comp. Neurol. 374, 535–554.
Zaborszky, L., Gaykema, R. P., Swanson, D. J., and Cullinan, W. E. (1997). Cortical input to the basal forebrain. Neuroscience 79, 1051–1078. doi: 10.1016/s0306-4522(97)00049-3
Záborszky, L., Gombkoto, Varsanyi, Gielow, M. R., Poe, G., Role, L. W., et al. (2018). Specific basal forebrain–cortical cholinergic circuits coordinate cognitive operations. J. Neurosci. 38, 9446–9458. doi: 10.1523/JNEUROSCI.1676-18.2018
Zahm, D. S. (1999). Functional-anatomical implications of the nucleus accumbens core and shell subterritories. Ann. N. Y. Acad. Sci. 877, 113–128.
Keywords: acetylcholine, attention, reward, striatum, midbrain, dopamine, frontal cortex
Citation: Runyon K, Bui T, Mazanek S, Hartle A, Marschalko K and Howe WM (2024) Distinct cholinergic circuits underlie discrete effects of reward on attention. Front. Mol. Neurosci. 17:1429316. doi: 10.3389/fnmol.2024.1429316
Received: 07 May 2024; Accepted: 01 August 2024;
Published: 29 August 2024.
Edited by:
Anna Mathia Klawonn, Danish Research Institute of Translational Neuroscience (DANDRITE), DenmarkReviewed by:
David Krolewski, University of Michigan, United StatesCopyright © 2024 Runyon, Bui, Mazanek, Hartle, Marschalko and Howe. This is an open-access article distributed under the terms of the Creative Commons Attribution License (CC BY). The use, distribution or reproduction in other forums is permitted, provided the original author(s) and the copyright owner(s) are credited and that the original publication in this journal is cited, in accordance with accepted academic practice. No use, distribution or reproduction is permitted which does not comply with these terms.
*Correspondence: William Matthew Howe, d21ob3dlQHZ0LmVkdQ==
Disclaimer: All claims expressed in this article are solely those of the authors and do not necessarily represent those of their affiliated organizations, or those of the publisher, the editors and the reviewers. Any product that may be evaluated in this article or claim that may be made by its manufacturer is not guaranteed or endorsed by the publisher.
Research integrity at Frontiers
Learn more about the work of our research integrity team to safeguard the quality of each article we publish.