- 1Neurological Intensive Unit, Department of Neurology, The First Affiliated Hospital, Sun Yat-sen University, Guangzhou, China
- 2Department of Neurology, Guangzhou Woman and Children’s Medical Centre, Guangzhou, China
Objectives: To determine risk factors for the occurrence of adverse outcomes in patients with new-onset refractory status epilepsy (NORSE) and to construct a concomitant nomogram.
Methods: Seventy-six adult patients with NORSE who were admitted to the Department of Neurology, First Affiliated Hospital of Sun Yat-sen University between January 2016 and December 2022 were enrolled for the study. Participants were divided into two—those with good and poor functional outcomes—and their pertinent data was obtained from the hospital medical recording system. Univariate analysis was used to identify potential causes of poor outcomes in both groups and a multivariate logistic regression model was used to identify risk factors for the occurrence of poor outcomes. Using the R programming language RMS package, a nomogram was created to predict the occurrence of poor outcomes.
Results: The NORSE risk of adverse outcome nomogram model included four predictors, namely duration of mechanical ventilation (OR = 4.370, 95% CI 1.221–15.640, p = 0.023), antiviral therapy (OR = 0.045, 95% CI 0.005–0.399, p = 0.005), number of anesthetics (OR = 13.428, 95% CI 2.16–83.48, p = 0.005) and neutrophil count/lymphocyte count ratio (NLR) (OR = 5.248, 95% CI 1.509–18.252, p = 0.009). The nomogram had good consistency and discrimination in predicting risk and can thus assist clinical care providers to assess outcomes for NORSE patients. Through ordinary bootstrap analyses, the results of the original set prediction were confirmed as consistent with those of the test set.
Conclusion: The nomogram model of risk of adverse outcomes in NORSE adult patients developed in this study can facilitate clinicians to predict the risk of adverse outcomes in NORSE patients and make timely and reasonable interventions for patients at high risk of adverse outcomes.
1 Introduction
Epilepsy is a chronic brain disorder that, although with many underlying causes, is generally characterized by recurring, episodic and temporary abnormalities of the central nervous system due to excessive neuronal discharges. An epileptic condition is status epilepticus (SE) (Trinka et al., 2015) where a seizure either lasts for more than 5 min or the patient does not regain consciousness between seizures—this is the second most frequent neurological emergency (Hesdorffer et al., 1998). Approximately 40% of SE patients are unresponsive to first- and second-line treatments (Holtkamp et al., 2005). An uncommon but challenging form of SE is new-onset refractory status epilepticus (NORSE), which is characterized by not only persistent refractory epilepticus in people with no prior history of epilepsy but also unresponsiveness to standard treatment (Hirsch et al., 2018). Its etiology remains unknown.
While some treatable causes, such as autoimmune encephalitis, have been identified in a subset of patients with New-Onset Refractory Status Epilepticus (NORSE), leading to significant research interest, the majority of patients remain cryptogenic (52%) (Sculier and Gaspard, 2019). Furthermore, there is limited knowledge regarding the potential pathophysiological mechanisms underlying NORSE, and the outcomes of treatments are often poor. Research indicates that adverse outcomes in NORSE patients are associated with several factors, including the duration of seizures, mechanical ventilation, and the utilization of antiepileptic drugs, with the prognosis frequently being extremely poor (Towne et al., 1994; Holtkamp et al., 2005; Hocker et al., 2013; Sutter et al., 2013; Chen et al., 2021). Given that NORSE is a rare clinical presentation, there are no literature statistics available on its incidence rate; however, the incidence rate of Refractory Status Epilepticus (RSE) is reported to be between 3.4 and 7.2 per 100,000 individuals annually, with NORSE accounting for 20% of these cases (Mantoan Ritter and Nashef, 2021). A meta-analysis of 193 RSE patients from 1980 to 2001 reported a mortality rate of 48% (Claassen et al., 2002). It is therefore critical to rapidly and objectively assess the condition and mortality risk in NORSE patients.
Currently, there are three scoring systems for Status Epilepticus internationally: the Status Epilepticus Severity Score (STESS), the Epidemiology-based Mortality Score in Status Epilepticus (EMSE), and the END-IT score. However, a meta-analysis published by Yuan et al. on the predictive scoring of Status Epilepticus suggests that the effectiveness of the STESS, EMSE, and END-IT scores in clinical use remains questionable, highlighting the need for further research to develop more accurate scoring systems (Yuan et al., 2023). This underscores that predicting the functional outcomes of patients with Status Epilepticus remains a challenge, especially for the rarer type such as New-Onset Refractory Status Epilepticus (NORSE), for which no studies have yet proposed a related outcome prediction system.
Given the uncertain prognosis of NORSE, treatment decisions are contentious and challenging, particularly regarding the duration of maintenance therapy during prolonged seizures, which remains a subject of debate. Against this backdrop, we conducted this study to identify prognostic predictors for NORSE, with the aim that these findings will assist clinicians in formulating treatment strategies to improve the prognosis of NORSE, facilitating the enhancement of care quality and the efficient utilization of medical resources.
Nomograms integrate and visualize influencing factors so as to predict clinical events on an individual basis. Thus, to aid clinicians to identify NORSE patients who are at a high risk of experiencing negative outcomes, this study aimed to analyze the factors influencing the occurrence of adverse outcomes in NORSE patients, by creating a nomogram model.
2 Methods
2.1 Study participants
Seventy-six NORSE adult patients who were admitted to the Department of Neurology, First Hospital of Sun Yat-sen University from January 2016 to December 2022 were enrolled for the study. All met the diagnostic criteria for NORSE (Gaspard et al., 2018) as defined at the 1st International Workshop on New-Onset Refractory Persistent Epilepsy (NORSE) and Febrile Infection-Related Epilepsy Syndrome (FIRES) Symposium. These were: an exhibition of multiple clinical syndromes; no previous history of epilepsy nor related neurological conditions; first-time presentation; attainment of refractory status epilepticus; and no identified structural, toxic nor metabolic etiology. Patient functional outcome at discharge was assessed by the modified Rankin Scale (mRS) score (Balu et al., 2019)—good functional outcome was defined as an mRS score ≤ 2, ranging from no disability (mRS = 0) to a slight disability but able to independently care for themselves (mRS = 2) whereas poor functional outcome (defined as mRS >2) represented a range from moderate disability that requires assistance for daily living (mRS = 3) to severe disability that requires ongoing care (mRS = 5) and possibly causes death (mRS = 6). This was used to divide patients into poor (mRS >2, n = 52) and good (mRS ≤2, n = 24) functional outcome groups.
NORSE patients who met the following criteria were enrolled in the study: new patients with refractory persistent epilepsy; age of onset ≥18 -year-old; and admission to the Department of Neurology, First Affiliated Hospital of Sun Yat-sen University. Exclusion criteria were: a previous history of seizures; identified structural, toxic, or metabolic etiology; lack of medical records due to failure to either continue treatment at the hospital or automatic abandonment (Figure 1).
The study protocol was reviewed and approved by the Ethics Committee of the First Affiliated Hospital of Sun Yat-sen University. It was exempted from obligatory signed informed consent forms from participants as it posed little risk to them.
2.2 Data collection
Patient information was obtained from the medical record database of the First Affiliated Hospital of Sun Yat-sen University. This included gender, age, etiology, prodromal stage, presence of infection, intubation, STESS score, END-IT score, GCS (glasglow coma scale), duration on a mechanical ventilator, immunotherapy regimens (one round of hormone shock = 1 g of methylprednisolone for 3 days; one round of IVIg = 0.4 g/kg/d immunoglobulin for 5 days), antiepilepsy regimens, presence of MR lesions, QAlb (Yu et al., 2021) (albumin of CSF/albumin of serum), NLR (Zeng et al., 2019) (neutrophil-to-lymphocyte ratio), CSF pressure of first time, albumin on admission, ICU stay, total hospital stay and financial cost, etc. Indicators were converted to categorical variables using relevant reference ranges.
2.3 Statistical analysis
The data were analyzed using SPSS 26.0. The Shapiro-Wilk test was used to perform normality tests. For quantitative data conforming to a normal distribution, comparisons between groups were conducted using independent samples t-tests; for quantitative data with a skewed distribution, comparisons between groups were performed using the Mann–Whitney U test. To ensure consistency throughout the document, quantitative data are presented as (x ± s), while count data are presented as case numbers and percentages [n (%)], with comparisons between groups conducted using the chi-square (χ2) test. For univariate analysis, an alpha level of 0.10 was used. Multivariate logistic regression was used to analyze factors influencing the occurrence of adverse outcomes in NORSE patients. For multivariate analysis, an alpha level of 0.05 was used. After identifying predictive risk factors with high values for adverse outcomes in NORSE patients, a nomogram for predicting adverse outcomes in NORSE patients was created using the R software RMS package.
2.4 Development and validation of the nomogram
To evaluate the predictive ability of the model, the receiver operator characteristic (ROC) curve and the consistency index (C-index) were used. To assess the accuracy of the predictive model, a calibration curve was plotted. The clinical decision curve analysis (DCA) was used to evaluate the clinical validity of the model. Internal validation was performed by bootstrap analysis.
3 Results
3.1 Patient characteristics
The average age of the 76 NORSE patients was 35.6 ± 19.9 years (median 28.5 years), with 40 (52.6%) males and 36 (47.4%) females and the average length of hospitalization was 41.4 ± 31.6 days (median 31.5 days). The average ICU stay was 24.3 ± 27.8 days and the average cost of hospitalization was ¥256,385.691. At discharge, 52 patients had a poor functional outcome, with a 68.4% incidence of adverse outcomes and 6 in-hospital deaths (7.9%). NCSE was detected by EEG monitoring in 5 patients (6.6%).
Mechanical ventilation was required in 49 of 76 patients (64.5%) with 39 (75%) in the poor outcome group and 10 (41.7%) in the good outcome group. The average duration of mechanical ventilation was 23.2 ± 26.3 days (median 10.5 days) with an average duration of 31.0 ± 28.8 days for mechanical ventilation in the poor outcome group and 6.0 ± 10.1 days for mechanical ventilation in the good outcome group.
All 76 patients underwent third-line coma induction therapy with 37 (48.7%) requiring 2 or more anesthetic agents. The most commonly used anesthetic drug was midazolam (n = 52, 68.4%), followed by propofol (n = 23, 30.3%), and phenobarbital (n = 21, 27.6%).
Of the 76 patients with NORSE enrolled, 39 (51.3%) had autoimmune etiology and 15 (19.7%) had infectious etiology, of which 13 (86.7%) had a diagnosis of viral encephalitis which was mainly diagnosed by combining clinical symptoms, cerebrospinal fluid indices and imaging manifestations. Antiviral therapy was administered to 39 (51.3%) of the 76 patients. It was used in 17 (70.8%) cases in the good outcome group and 22 (42.3%) cases in the poor outcome group.
3.2 Risk factors for adverse outcomes
To identify predictors of adverse outcomes, univariate logistic regression was performed for each variable in the development dataset. Variables with p < 0.05 included etiology, infection, GCS score, NLR, intubation, mechanical ventilation, days on a ventilator, antiviral therapy, rounds of intravenous immunoglobulin (IVIg), number of anesthetics, number of antiepileptic drugs (AEDs) (Table 1). Statistically significant indicators were included in a multifactorial logistic regression model. The results showed that antiviral therapy, number of anesthetics, mechanical ventilation, and NLR were independent risk factors for adverse outcomes in NORSE patients (p < 0.05) (Table 2).
3.3 Nomogram model development and validation
The variables identified from the multivariate logistic regression were used as predictor variables and the total score for each factor—the score range was 0–220 and the higher the score, the greater the risk of adverse outcome occurrence—was calculated using the R software RMS package for nomogram analysis (Figure 2). Using the factors of mechanically ventilation, the NLR value, antiviral therapy, the rounds of IVIg, and the number of anesthetics, the corresponding risk of adverse outcomes was calculated by obtaining the corresponding scores of values from the vertical lines on the score line at the top of the column plot, and subsequently adding the scores of all variables to obtain the total score.
The dynamic nomogram: https://luoqiuyan.shinyapps.io/NORSE/.
The ROC curve was used to determine the ability of the model to predict the occurrence of adverse outcomes in patients with NORSE. The area under the ROC curve (AUC) for this model was 0.912 (95% CI: 0.844–0.980), with a sensitivity of 80.8% and specificity of 95.8% (Figure 3).
For internal validation, an analysis of 1,000 bootstrap resamples with replacement was done. Then, the calibration curves for predicted adverse outcomes were plotted against the actual adverse outcomes, with the dashed line indicating the uncalibrated portion and the realization indicating the calibrated portion, both of which were close to the theoretical curve (Figure 4).
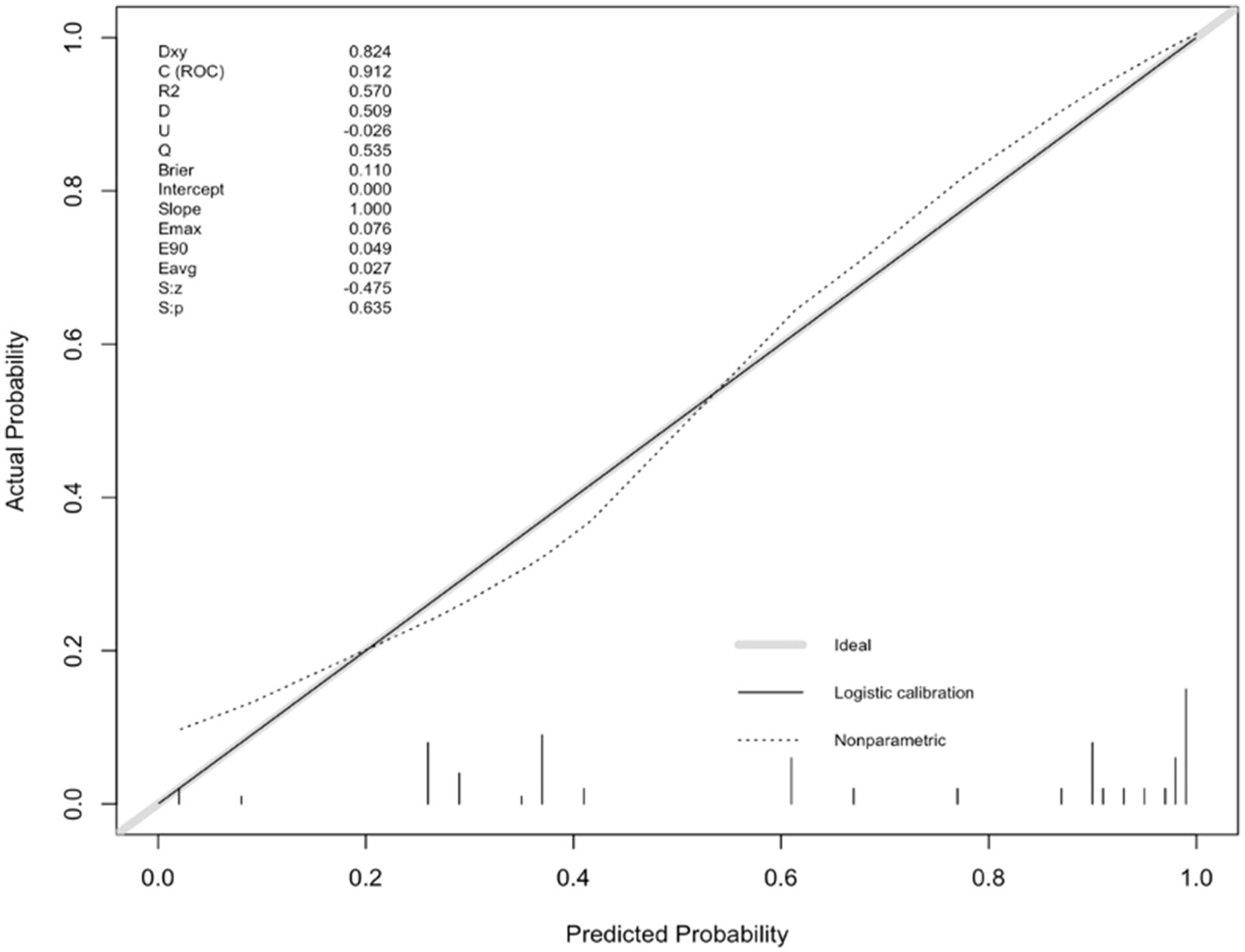
Figure 4. Calibration plot of the nomogram predicting the risk of adverse outcomes versus the actual risk of adverse outcomes.
For the graph on clinical validity, the thick solid line represented no clinical benefit for patients who do not experience an adverse outcome whereas the thin solid line—an inverse slope with a negative gradient—represented the net clinical benefit for patients who experienced an adverse outcome. The red line above the two extreme curves represented clinical validity. The threshold probability was 25% when the net benefit of patients was higher than the two extreme curves (Figure 5).
Final internal validation was performed via ordinary bootstrapping. The Root Mean Square Error (RMSE) value of the model was calculated to be 0.41.The model fit was moderately high (Alba et al., 2017).
4 Discussion
New onset refractory status epilepticus (NORSE) is a neurological emergency that not only occurs in patients with no previous history of neurological disease but also often has unknown etiology (Hirsch et al., 2018). The prognosis is extremely poor, as 62% of the 130 patients had poor outcomes with a 22% mortality rate (Gaspard et al., 2015). The pathophysiological mechanisms underlying NORSE are currently unknown and there are no standard international guidelines for treatment. Thus, a current research focus is the identification of variables that affect outcomes in NORSE patients.
Antiviral treatment was one of the predictive factors possibly due to the effectiveness of specific therapies for validated etiologies. Although autoimmune disorders are the most common etiological diagnoses (37%) for NORSE, little is known on other etiologies apart from infectious agents (8%) which are mainly viral (Yu et al., 2021). The diagnosis of NORSE does not exclude any particular virus to prevent varying disease definitions due to the capacities for viral identification (Gaspard et al., 2015). Moreover, the causal role of testing for certain viruses is unclear. NORSE patients often have an unclear etiology upon admission, which leads to a differential diagnosis of autoimmune encephalitis or viral encephalitis. As a results, attempted antiviral therapy, primarily acyclovir, is provided to most patients. Our study diagnosed patients with viral encephalitis mainly based on clinical manifestations and ancillary tests with negative cerebrospinal fluid polymerase chain reaction (PCR). Literatures suggest that initially PCR-negative status epilepsy can become PCR-positive after a period of illness, so antiviral therapy may still be effective (Buerger et al., 2015; Roberts et al., 2021). Case reports also propose that NORSE may be a precursor manifestation of herpes simplex viral encephalitis, even in PCR-negative cases and seizures may only cease after treating the etiology (Verma et al., 2013). However, lumbar puncture is often not repeated to confirm the etiologic agent due to the disease’s rapid progression. Our study found an independent effect of antiviral therapy on outcome after first univariate and then multifactorial analyses, ruling out the effect of other interfering or confounding factors. As the causal role of viral positivity is currently unknown, it is necessary to design prospective studies with periodic repeat lumbar puncture data to determine the role of antiviral therapy.
Current treatment of NORSE can be divided into two axis: disease-modifying treatments through immunotherapy and administration of appropriate antiepileptic drugs (AEDs) to terminate seizures (Jang et al., 2020). Current management of status epilepsy varies with region, and case studies are often on the selection of the best AEDs. Yet AEDs treatment is often disappointing with many patients requiring multiple anesthetics to regain control—status epilepticus often reoccurs upon discontinuation of the drug (Kramer et al., 2011; Gaspard et al., 2015; van Baalen et al., 2017). In this study, almost all patients were on one or more anesthetic drugs. The use of anesthetic agents is often associated with adverse outcomes, whose risk increases significantly with the number of anesthetic agents used, either sequentially or concurrently (Gaspard et al., 2015) and this corroborated our findings. Relatedly, adult SE patients under intravenous anesthetic therapies had high rates of infections and an increased risk of death (Hocker et al., 2013; Sutter et al., 2014; Marchi et al., 2015; Hocker, 2019), casting doubt on the efficacy of anesthetics for the treatment of persistent epilepsy. The severity and duration of SE is hypothesized as the main proponent for the use of anesthetic drugs and resultant high complications and mortality (Towne et al., 1994). Patients with refractory status epilepsy (RSE) who were under high doses of anesthetics had better outcomes than those under low doses further supporting the hypothesis that the severity of RSE itself, and not the use of anesthetics (Fernandez et al., 2014), is the main determinant of adverse outcomes. In the initial phase of our study, we attempted to conduct a subgroup analysis based on the types of anesthetics used. However, due to the limited size of our sample, this endeavor did not yield effective statistical analysis. Therefore, future research will require a larger sample size to thoroughly investigate the impact of different anesthetics on the prognosis of patients with NORSE.
Mechanical ventilation was a risk factor for adverse outcomes in NORSE patients, corroborating mechanical ventilation during hospitalization as an independent risk factor for adverse outcomes in RSE and the development of post-autoimmune encephalitis epilepsy (PAEE) (Lowenstein, 1999; Li et al., 2009; Chen et al., 2021). Over 90% of RSE cases required mechanical ventilation, a third of which eventually required tracheotomies. The longer the duration of mechanical ventilation, the higher the resultant mortality rate (Hocker et al., 2013). In a coma, tracheal intubation is used to protect the airwaves of patients with refractory persistent epilepsy (Zeidan et al., 2021). Yet patients who present this degree of impaired consciousness often have poor outcomes.
The peripheral blood neutrophil-to-lymphocyte ratio (NLR) is an increasingly recognized biomarker of systemic inflammation for a variety of disease states (Celikbilek et al., 2013; Demirci et al., 2016; Mercan et al., 2016; Qin et al., 2016). In NORSE autoimmune pathogenesis is more common than viral infections (Jang et al., 2020). Therefore, NORSE is a potential autoimmune epilepsy requiring aggressive immunotherapy following assessment of the infection (Jang et al., 2020). Based on relationships between autoimmune encephalitis and NLR, the best threshold for predicting severe disability (mRS > 2) was NLR > 4.82 (AUC 0.875) (Zeng et al., 2019). Here, using univariate and multivariate analyses, we also identified NLR > 4.82 as an independent risk factor. For autoimmune encephalitis patients, each 1-unit increase in NLR was associated with a 1.3-fold increase in the odds of treatment failure (Broadley et al., 2020). The similarity in clinical features and laboratory results between NORSE patients with an autoimmune etiology and those without a known etiology suggests that we can identify more autoimmune etiologies, especially with the discovery of autoantibodies (Gaspard et al., 2015).
To accurately predict the risk of adverse outcomes in NORSE patients, we constructed a nomogram based on the aforementioned factors and internally validated it. The calibration curve was close to the ideal curve and there was no statistically significant difference between the predicted and actual values. From ROC curve analysis, the area under the curve for the model, which predicted the occurrence of adverse outcomes in NORSE patients was 0.912 (95% CI: 0.844–0.980), indicating that the model had good consistency and discrimination in predicting risk.
This study, utilizing a nomogram predictive model based on clinical data from NORSE patients, effectively aids clinicians in assessing the prognosis of NORSE patients and provides reference for further interventions. However, there are several limitations to our research. Firstly, our study was based on clinical data from a single center to develop the nomogram model and only underwent internal validation, lacking verification against external clinical data. Therefore, we need to collect a broader dataset to further validate its applicability in different clinical settings. Secondly, the limited sample size of clinical data in our study resulted in high variability, increasing the uncertainty in risk prediction for individual patients and diminishing the ability to generalize the study results to a broader population. This limitation also restricted the depth and breadth of subgroup analyses, such as those involving different types of anesthetic drugs. Expanding the sample size for more extensive validation would be beneficial. If future research could increase the sample size and design prospective studies or subgroup analyses to further validate and confirm the model’s effectiveness and reliability, it would provide better clinical application and promotional value. Additionally, considering the potential fluctuations in the condition of NORSE patients, incorporating follow-up data and further survival analysis, if feasible, could be more meaningful for accurately assessing patient outcomes.
5 Conclusion
In conclusion, independent risk factors for adverse outcomes in NORSE are antiviral therapy, the number of rounds of IVIg, the number of anesthetics, mechanical ventilation and high NLR score. The nomogram for predicting the risk of poor outcomes had good consistency and discrimination in predicting risk and can thus assist clinical care providers to assess outcomes for NORSE patients. However, this single-center study had a small sample size and the model was only validated internally. Therefore, the discrimination and accuracy of this model should be further confirmed by analyzing data from multiple centers, increasing the sample size and adopting a combination of internal and external validations.
Data availability statement
The raw data supporting the conclusions of this article will be made available by the authors, without undue reservation.
Ethics statement
The studies involving humans were approved by the Ethics Committee of the First Affiliated Hospital of Sun Yat-sen University. The studies were conducted in accordance with the local legislation and institutional requirements. Written informed consent for participation was not required from the participants or the participants’ legal guardians/next of kin in accordance with the national legislation and institutional requirements.
Author contributions
QL: Data curation, Formal analysis, Investigation, Methodology, Project administration, Resources, Software, Writing – original draft. RL: Funding acquisition, Investigation, Methodology, Writing – original draft. MS: Methodology, Project administration, Resources, Writing – original draft. ZW: Project administration, Resources, Software, Writing – original draft. HF: Funding acquisition, Supervision, Validation, Visualization, Writing – review & editing. HZ: Conceptualization, Data curation, Formal analysis, Supervision, Validation, Visualization, Writing – review & editing.
Funding
The author(s) declare that no financial support was received for the research, authorship, and/or publication of this article.
Conflict of interest
The authors declare that the research was conducted in the absence of any commercial or financial relationships that could be construed as a potential conflict of interest.
Publisher’s note
All claims expressed in this article are solely those of the authors and do not necessarily represent those of their affiliated organizations, or those of the publisher, the editors and the reviewers. Any product that may be evaluated in this article, or claim that may be made by its manufacturer, is not guaranteed or endorsed by the publisher.
References
Alba, A. C., Agoritsas, T., Walsh, M., Hanna, S., Iorio, A., Devereaux, P. J., et al. (2017). Discrimination and calibration of clinical prediction models: users’ guides to the medical literature. JAMA 318, 1377–1384. doi: 10.1001/jama.2017.12126
Balu, R., McCracken, L., Lancaster, E., Graus, F., Dalmau, J., and Titulaer, M. J. (2019). A score that predicts 1-year functional status in patients with anti-NMDA receptor encephalitis. Neurology 92, e244–e252. doi: 10.1212/WNL.0000000000006783
Broadley, J., Wesselingh, R., Seneviratne, U., Kyndt, C., Beech, P., Buzzard, K., et al. (2020). Peripheral immune cell ratios and clinical outcomes in seropositive autoimmune encephalitis: a study by the Australian autoimmune encephalitis consortium. Front. Immunol. 11:597858. doi: 10.3389/fimmu.2020.597858
Buerger, K. J., Zerr, K., and Salazar, R. (2015). An unusual presentation of herpes simplex encephalitis with negative PCR. BMJ Case Rep. 2015:bcr2015210522. doi: 10.1136/bcr-2015-210522
Celikbilek, M., Dogan, S., Ozbakır, O., Zararsız, G., Kücük, H., Gürsoy, S., et al. (2013). Neutrophil-lymphocyte ratio as a predictor of disease severity in ulcerative colitis. J. Clin. Lab. Anal. 27, 72–76. doi: 10.1002/jcla.21564
Chen, S.-S., Zhang, Y. F., di, Q., Shi, J. P., Wang, L. L., Lin, X. J., et al. (2021). Predictors and prognoses of epilepsy after anti-neuronal antibody-positive autoimmune encephalitis. Seizure 92, 189–194. doi: 10.1016/j.seizure.2021.09.007
Claassen, J., Hirsch, L. J., Emerson, R. G., and Mayer, S. A. (2002). Treatment of refractory status epilepticus with pentobarbital, propofol, or midazolam: a systematic review. Epilepsia 43, 146–153. doi: 10.1046/j.1528-1157.2002.28501.x
Demirci, S., Demirci, S., Kutluhan, S., Koyuncuoglu, H. R., and Yurekli, V. A. (2016). The clinical significance of the neutrophil-to-lymphocyte ratio in multiple sclerosis. Int. J. Neurosci. 126, 1–7. doi: 10.3109/00207454.2015.1050492
Fernandez, A., Lantigua, H., Lesch, C., Shao, B., Foreman, B., Schmidt, J. M., et al. (2014). High-dose midazolam infusion for refractory status epilepticus. Neurology 82, 359–365. doi: 10.1212/WNL.0000000000000054
Gaspard, N., Foreman, B. P., Alvarez, V., Cabrera Kang, C., Probasco, J. C., Jongeling, A. C., et al. (2015). New-onset refractory status epilepticus: etiology, clinical features, and outcome. Neurology 85, 1604–1613. doi: 10.1212/WNL.0000000000001940
Gaspard, N., Hirsch, L. J., Sculier, C., Loddenkemper, T., van Baalen, A., Lancrenon, J., et al. (2018). New-onset refractory status epilepticus (NORSE) and febrile infection-related epilepsy syndrome (FIRES): state of the art and perspectives. Epilepsia 59, 745–752. doi: 10.1111/epi.14022
Hesdorffer, D. C., Logroscino, G., Cascino, G., Annegers, J. F., and Hauser, W. A. (1998). Incidence of status epilepticus in Rochester, Minnesota, 1965-1984. Neurology 50, 735–741. doi: 10.1212/wnl.50.3.735
Hirsch, L. J., Gaspard, N., van Baalen, A., Nabbout, R., Demeret, S., Loddenkemper, T., et al. (2018). Proposed consensus definitions for new-onset refractory status epilepticus (NORSE), febrile infection-related epilepsy syndrome (FIRES), and related conditions. Epilepsia 59, 739–744. doi: 10.1111/epi.14016
Hocker, S. (2019). Why do patients die after status epilepticus? Epilepsy Behav. 101:106567. doi: 10.1016/j.yebeh.2019.106567
Hocker, S. E., Britton, J. W., Mandrekar, J. N., Wijdicks, E. F. M., and Rabinstein, A. A. (2013). Predictors of outcome in refractory status epilepticus. JAMA Neurol. 70, 72–77. doi: 10.1001/jamaneurol.2013.578
Holtkamp, M., Othman, J., Buchheim, K., and Meierkord, H. (2005). Predictors and prognosis of refractory status epilepticus treated in a neurological intensive care unit. J. Neurol. Neurosurg. Psychiatry 76, 534–539. doi: 10.1136/jnnp.2004.041947
Jang, Y., Kim, D. W., Yang, K. I., Byun, J. I., Seo, J. G., No, Y. J., et al. (2020). Clinical approach to autoimmune epilepsy. J. Clin. Neurol. 16, 519–529. doi: 10.3988/jcn.2020.16.4.519
Kramer, U., Chi, C. S., Lin, K. L., Specchio, N., Sahin, M., Olson, H., et al. (2011). Febrile infection-related epilepsy syndrome (FIRES): pathogenesis, treatment, and outcome: a multicenter study on 77 children. Epilepsia 52, 1956–1965. doi: 10.1111/j.1528-1167.2011.03250.x
Li, J.-M., Chen, L., Zhou, B., Zhu, Y., and Zhou, D. (2009). Convulsive status epilepticus in adults and adolescents of Southwest China: mortality, etiology, and predictors of death. Epilepsy Behav. 14, 146–149. doi: 10.1016/j.yebeh.2008.09.005
Lowenstein, D. H. (1999). Status epilepticus: an overview of the clinical problem. Epilepsia 40, S3–S8. discussion S21–S22. doi: 10.1111/j.1528-1157.1999.tb00872.x
Mantoan Ritter, L., and Nashef, L. (2021). New-onset refractory status epilepticus (NORSE). Pract. Neurol. 21, 119–127. doi: 10.1136/practneurol-2020-002534
Marchi, N. A., Novy, J., Faouzi, M., Stähli, C., Burnand, B., and Rossetti, A. O. (2015). Status epilepticus: impact of therapeutic coma on outcome. Crit. Care Med. 43, 1003–1009. doi: 10.1097/CCM.0000000000000881
Mercan, R., Bitik, B., Tufan, A., Bozbulut, U. B., Atas, N., Ozturk, M. A., et al. (2016). The association between neutrophil/lymphocyte ratio and disease activity in rheumatoid arthritis and ankylosing spondylitis. J. Clin. Lab. Anal. 30, 597–601. doi: 10.1002/jcla.21908
Qin, B., Ma, N., Tang, Q., Wei, T., Yang, M., Fu, H., et al. (2016). Neutrophil to lymphocyte ratio (NLR) and platelet to lymphocyte ratio (PLR) were useful markers in assessment of inflammatory response and disease activity in SLE patients. Mod. Rheumatol. 26, 372–376. doi: 10.3109/14397595.2015.1091136
Roberts, J. I., Jewett, G. A. E., Tellier, R., Couillard, P., and Peters, S. (2021). Twice negative PCR in a patient with herpes simplex virus type 1 (HSV-1) encephalitis. Neurohospitalist 11, 66–70. doi: 10.1177/1941874420943031
Sculier, C., and Gaspard, N. (2019). New onset refractory status epilepticus (NORSE). Seizure 68, 72–78. doi: 10.1016/j.seizure.2018.09.018
Sutter, R., Kaplan, P. W., and Rüegg, S. (2013). Outcome predictors for status epilepticus--what really counts. Nat. Rev. Neurol. 9, 525–534. doi: 10.1038/nrneurol.2013.154
Sutter, R., Marsch, S., Fuhr, P., Kaplan, P. W., and Rüegg, S. (2014). Anesthetic drugs in status epilepticus: risk or rescue? A 6-year cohort study. Neurology 82, 656–664. doi: 10.1212/WNL.0000000000000009
Towne, A. R., Pellock, J. M., Ko, D., and DeLorenzo, R. J. (1994). Determinants of mortality in status epilepticus. Epilepsia 35, 27–34. doi: 10.1111/j.1528-1157.1994.tb02908.x
Trinka, E., Cock, H., Hesdorffer, D., Rossetti, A. O., Scheffer, I. E., Shinnar, S., et al. (2015). A definition and classification of status epilepticus--report of the ILAE task force on classification of status epilepticus. Epilepsia 56, 1515–1523. doi: 10.1111/epi.13121
van Baalen, A., Vezzani, A., Häusler, M., and Kluger, G. (2017). Febrile infection-related epilepsy syndrome: clinical review and hypotheses of Epileptogenesis. Neuropediatrics 48, 5–18. doi: 10.1055/s-0036-1597271
Verma, R., Raut, T. P., Giri, P., and Praharaj, H. N. (2013). New onset refractory status epilepticus (NORSE) as the heralding manifestation of herpes simplex encephalitis. BMJ Case Rep. 2013:bcr2013009466. doi: 10.1136/bcr-2013-009466
Yu, Y., Wu, Y., Cao, X., Li, J., Liao, X., Wei, J., et al. (2021). The clinical features and prognosis of anti-NMDAR encephalitis depends on blood brain barrier integrity. Mult. Scler. Relat. Disord. 47:102604. doi: 10.1016/j.msard.2020.102604
Yuan, F., Damien, C., and Gaspard, N. (2023). Prognostic scores in status epilepticus: a systematic review and meta-analysis. Epilepsia 64, 17–28. doi: 10.1111/epi.17442
Zeidan, S., Rohaut, B., Outin, H., Bolgert, F., Houot, M., Demoule, A., et al. (2021). Not all patients with convulsive status epilepticus intubated in pre-hospital settings meet the criteria for refractory status epilepticus. Seizure 88, 29–35. doi: 10.1016/j.seizure.2021.03.015
Keywords: new-onset refractory status epilepsy, neurocritical illness, predictive factor, nomogram, autoimmune mechanisms
Citation: Luo Q, Lai R, Su M, Wu Z, Feng H and Zhou H (2024) Risk factors and a predictive model for the occurrence of adverse outcomes in patients with new-onset refractory status epilepsy. Front. Mol. Neurosci. 17:1360949. doi: 10.3389/fnmol.2024.1360949
Edited by:
Saak V. Ovsepian, University of Greenwich, United KingdomReviewed by:
Amanda Morato Do Canto, State University of Campinas, BrazilJavier Franco-Pérez, Manuel Velasco Suárez National Institute of Neurology and Neurosurgery, Mexico
Copyright © 2024 Luo, Lai, Su, Wu, Feng and Zhou. This is an open-access article distributed under the terms of the Creative Commons Attribution License (CC BY). The use, distribution or reproduction in other forums is permitted, provided the original author(s) and the copyright owner(s) are credited and that the original publication in this journal is cited, in accordance with accepted academic practice. No use, distribution or reproduction is permitted which does not comply with these terms.
*Correspondence: Hongyan Zhou, zhouhy7@mail.sysu.edu.cn