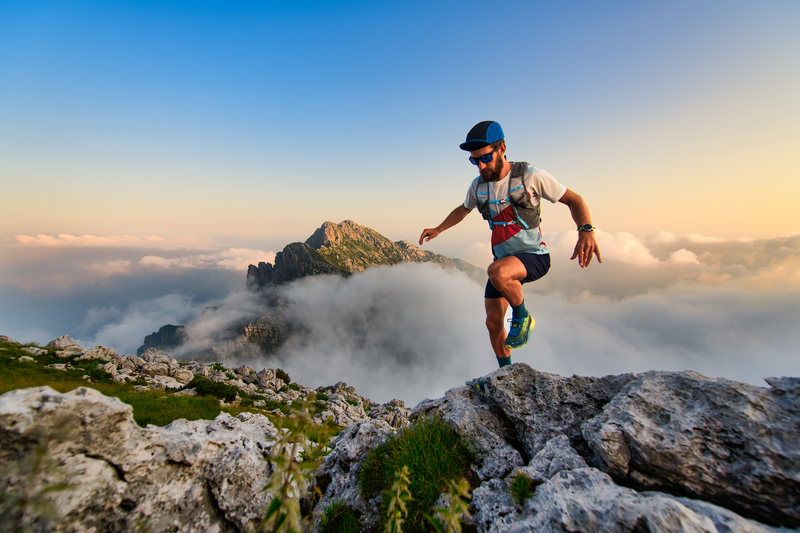
95% of researchers rate our articles as excellent or good
Learn more about the work of our research integrity team to safeguard the quality of each article we publish.
Find out more
MINI REVIEW article
Front. Mol. Neurosci. , 26 October 2023
Sec. Neuroplasticity and Development
Volume 16 - 2023 | https://doi.org/10.3389/fnmol.2023.1292685
Motor learning is crucial for the survival of many animals. Acquiring a new motor skill involves complex alterations in both local neural circuits in many brain regions and long-range connections between them. Such changes can be observed anatomically and functionally. The primary motor cortex (M1) integrates information from diverse brain regions and plays a pivotal role in the acquisition and refinement of new motor skills. In this review, we discuss how motor learning affects the M1 at synaptic, cellular, and circuit levels. Wherever applicable, we attempt to relate and compare findings in humans, non-human primates, and rodents. Understanding the underlying principles shared by different species will deepen our understanding of the neurobiological and computational basis of motor learning.
Muscle-based motor systems are evolutionarily very ancient, present in all metazoans except sponges (Seipel and Schmid, 2005; Wang et al., 2022). In an ever-changing, unpredictable environment, it is advantageous to have a variety of motor functions and to be able to generate motor outputs adaptively. Mammals arguably possess the richest motor repertoire, and have the capability to change or improve motor performances through practice, a process generally referred to as motor learning (Shmuelof and Krakauer, 2011). Mammalian motor behaviors engage a complex network of brain regions, including the neocortex, the basal ganglia, the motor thalamus, the cerebellum, and brainstem and midbrain areas such as the ventral tegmental area (VTA), the substantia nigra pars compacta (SNc), the periaqueductal gray area (PAG), the vestibular nuclei, and the pedunculopontine nucleus (PPN) (Suryanarayana et al., 2022). Particularly, placental mammals possess at least one separate cortical motor area (Krubitzer, 2007). The primary motor cortex (M1) serves as a control hub of this network, sending commands that enable the flexible recruitment of lower motor neurons in the spinal cord, which underlies the ability to learn and to perform novel tasks (Shmuelof and Krakauer, 2011). Its functions are subserved by the local circuit as well as the long-range connections with other brain regions. M1 has a laminar structure, containing many of the same neuronal elements of the canonical microcircuit as in other neocortical areas (Douglas and Martin, 2004). Traditionally, it is believed that M1 is cytoarchitectonically distinct by the lack of layer (L) 4 (Geyer et al., 2000), which mainly consists of granular cells and is the principal target of thalamic inputs in sensory cortices. Some recent studies (Garcia-Cabezas and Barbas, 2014; Yamawaki et al., 2014; Bopp et al., 2017), however, suggest the existence of a bona fide L4 in M1 of mice and rhesus monkeys, which echoes Ramón y Cajal’s description of a L4 in the human motor cortex (Ramón y Cajal, 1899).
M1 receives inputs from several thalamic nuclei (thalamocortical or TC inputs) and other cortical areas (corticocortical or CC inputs). TC inputs exhibit a sub-region- and layer-specific distribution. The ventral medial nucleus (VM) and the anterior medial nucleus (AM) of the thalamus project to L1, the ventral anterior/ventral lateral nucleus (VA/VL) projects to L1 and L2/3, and the posterior nucleus (PO) innervates L2/3 and L5 (Hooks et al., 2013; Kuramoto et al., 2015; Tanaka et al., 2018; Hasegawa et al., 2020; Munoz-Castaneda et al., 2021). CC inputs also exhibit sub-region- and layer-specificity. For example, L5 neurons in the mouse rostral forelimb area (RFA) of the motor cortex receive inputs from L2/3 and L5A, but not L5B, neurons in the caudal forelimb area (CFA); conversely, CFA L5 neurons receive inputs from RFA L5B neurons (Hira et al., 2013). In the rat brain, it has been reported (Ueta et al., 2014) that projections from the secondary motor cortex (M2) to M1 mostly arise from pyramidal neurons (PyrNs) in lower L2/3 and L5A. Among them, M2 L5A neurons predominantly send their axons to L1 of M1, whereas M2 L2/3 neurons also innervate L2/3 of M1. Furthermore, in the mouse vibrissal M1 (vM1), axons from the vibrissal primary somatosensory cortex (vS1) preferentially innervate L2/3 and L5A neurons, providing only weak inputs to L5B and L6 neurons (Mao et al., 2011). A later study by the same group (Hooks et al., 2015) showed with dual-channel optogenetic stimulation that individual L2/3 neurons in the mouse vM1 receive inputs from both vS1 and the posteromedial nucleus of the thalamus (POm). Taken together, such anatomical specificity suggests that neurons in different layers of M1 integrate different information streams for motor functions.
M1 sends outputs to many brain regions. Among the main projection neurons in M1, pyramidal tract (PT) neurons send axons down the white matter tract in the brainstem and innervate a variety of regions within and outside the telencephalon, including the spinal cord, pons, striatum, brainstem, and thalamus; intratelencephalic (IT) neurons, in contrast, project exclusively within the telencephalon to the cortex and striatum (Baker et al., 2018). A special sub-class of PT neurons are the corticomotoneuronal (CM) cells, which directly synapse onto spinal cord alpha motor neurons controlling hand and finger muscles. They are unique to dexterous primates, which they work synergistically with evolutionarily more ancient descending pathways to generate highly dexterous movements (Lemon, 2008; Yoshida and Isa, 2018; Strick et al., 2021). In rodents CM connections are present only transiently and are eliminated shortly after birth (Gu et al., 2017; Murabe et al., 2018). Besides projecting to different targets, one recent study also suggests that corticostriatal projections from M1 IT neurons exhibit a higher degree of topographic stereotypy than those from PT neurons (Hooks et al., 2018). A schematic of the organization of major M1 inputs and outputs is given in Figure 1. For a more comprehensive description of M1 input and output patterns, (see Hooks et al., 2013; Baker et al., 2018; BRAIN Initiative Cell Census Network (BICCN), 2021; Munoz-Castaneda et al., 2021); for details of cortical local circuitry, (see Harris and Shepherd, 2015).
Figure 1. A schematic of M1 input–output organization. Left: the laminar distribution of inputs from major sources to M1, shown as relative input strength. Right: targets of major types of M1 output neurons. ET, extratelencephalic neurons (traditionally termed pyramidal tract or PT neurons); IT, intratelencephalic neurons; CT, corticothalamic neurons. Adapted from Baker et al. (2018, licensed under CC-BY 4.0), Hooks et al. (2013, licensed under CC-BY-NC-SA 3.0), and BRAIN Initiative Cell Census Network (BICCN) (2021, licensed under CC-BY 4.0).
Previous studies across species have demonstrated that M1 plays an essential role in motor learning. An early human study (Schlaug et al., 1994) used positron emission tomography (PET), which uses radioactive tracers to measure physiological processes such as metabolism and blood flow (Raichle, 1983), to identify cerebral structures activated in a task in which participants carried out a complex sequence of finger tapping. It showed that the contralateral sensorimotor area had the highest activation, with other cortical and subcortical areas variably implicated. Another study trained human subjects to move fingers in specific orders paired with specific rhythms and found through functional magnetic resonance imaging (fMRI) that contralateral M1 encodes the learned finger movement sequences and rhythms as an integrated unit (Kornysheva and Diedrichsen, 2014). Later, it was discovered that bilateral transcranial direct current stimulation (tDCS) to the motor cortex substantially improved the human subject’s learning in a unimanual sequence movement task compared to unilateral or sham stimulation (Waters et al., 2017). Upregulation of M1 excitability by transcranial magnetic stimulation (TMS) (Hallett, 2000) also improved learning in a non-dominant hand digit sequence task (Narayana et al., 2014) and during a serial reaction time task (SRTT, commonly used to investigate motor learning in humans) (Nitsche et al., 2003). On the other hand, inactivating M1 interferes with motor learning. For example, Muellbacher et al. trained humans to perform fast finger movement and measured movement acceleration and muscle force generation. They found that low-frequency, repetitive TMS to M1 acutely disrupted the retention of learned behavior, without affecting basal motor behaviors or subsequent motor learning, suggesting that M1 is specifically engaged in the early phase of motor skill consolidation (Muellbacher et al., 2002). Another study found that inhibitory TMS through continuous theta burst stimulation to M1 impaired motor learning in SRTT. This effect is believed to result from a decreased functional connectivity between M1 and other brain regions relevant to the task (Steel et al., 2016).
In rodent models, researchers have tried to identify brain regions active during motor learning by immunostaining Fos, the protein product of the immediate-early gene cFOS, as a proxy for neuronal activation. An early study (Kleim et al., 1996) found that Fos expression was significantly higher in M1 L2/3 of rats trained on an acrobatic task (traversing a series of 10 obstacles including a rotating cylinder, a suspended chain, wooden blocks, dowel rods of varying diameters, etc.), which requires a wide variety of fine motor skills, compared to control animals. Furthermore, Fos signal was greater in brains collected during skill acquisition than in those collected during task performance, suggesting that M1 is the most active during the early phase of learning, consistent with findings in human experiments (Muellbacher et al., 2002). Notably, training on complex motor behaviors (running on a wheel with an irregular rung pattern) activates M1 stronger than simple motor training (running on an accelerating rotating rod or “rotarod”), as shown by Fos immunostaining (Nagai et al., 2017).
Interestingly, while accumulating evidence suggests that M1 is crucial for motor learning and dexterity, it seems more or less dispensable for simpler, “innate” movements. Early studies have shown that lesioning the motor cortex (Castro, 1972; Whishaw, 2000) impairs dexterous movements in rats. Unilateral partial or complete pyramidal sections, which disrupt the corticospinal tract (CST, the major neural pathway connecting M1 to the spinal cord), likewise impair skilled reaching in rats (Whishaw et al., 1993; Piecharka et al., 2005). Similarly, interrupting the CST in monkeys by bilateral lesioning at the upper medullary level impairs dexterity (Lawrence and Kuypers, 1968). However, simpler movements such as running in a maze or cage are generally not impaired by cortical lesions encompassing M1 (Franz and Lashley, 1917; Maier, 1935). A study on rhesus monkeys also showed that after complete removal of sensorimotor areas including M1, walking and jumping recovered over time but dexterity did not (Passingham et al., 1983). To avoid the confounding effect of M1 lesion on dexterity during motor learning, Kawai et al. created a motor sequence task, in which the rat learns to press a lever twice in a prescribed temporal sequence to obtain a water reward (Kawai et al., 2015). As dexterity is not explicitly required, this task dissociates the learning and execution of motor sequences from the generation of dexterous movements. Strikingly, although pre-training M1 lesions prevented rats from acquiring the motor sequence, post-training M1 lesions did not affect the execution of the acquired motor sequence. Consistent with this, M1 inactivation through optogenetic activation of inhibitory interneurons impairs the mouse’s motor performance in early and mid, but not late, stages of learning a joystick-press task (Hwang et al., 2019). Moreover, a recent study (Serradj et al., 2023) showed that learning a “precise” motor task (isometric pull with a specific force) remodeled the activity pattern of relevant M1 corticospinal neurons in mice, whereas learning an “imprecise” task (pull without the need for precise force adjustment) did not. Bilateral pyramidotomy or optogenetic silencing of corticospinal neurons disrupted the performance in the precise task but largely spared the imprecise task. Taken together, these studies highlight M1’s role in learning new, complex motor skills rather than performing routine motor tasks.
Both human and animal studies have revealed that motor learning induces macroscopic anatomical changes in M1. Several works have compared the brains of musicians (professional and amateur keyboard players) and non-musicians, as playing a musical instrument requires one to learn complex fine movement patterns and to rehearse them over time. Using voxel-based morphometry (Ashburner and Friston, 2000), an automated technique to identify brain anatomy differences by statistical analysis of high-resolution structural MRI data, Gaser and Schlaug found a significant positive correlation between musician status and the volume of gray matter in several brain regions including M1, with professional musicians having the largest volume (Gaser and Schlaug, 2003). Analogously, world-class gymnasts have a larger volume of gray matter in the precentral gyrus (M1) than non-gymnast controls, and among gymnasts, larger gray matter volume correlates with higher average scores in routines they competed (Fukuo et al., 2020). In concert pianists, diffusion tensor imaging (DTI) discovered a significant correlation between the practice time and the fractional anisotropy (FA) (Bengtsson et al., 2005). FA is a widely-used measure that reflects axonal fiber density, diameter, and myelination in the white matter (Le Bihan, 2003). Interestingly, practice time during different age periods–childhood, adolescence, or adulthood–correlates with FA in different sets of brain regions. In particular, childhood practice time correlates with FA in the posterior limbs of the internal capsule, which contain CST fibers that are critically important for independent finger movements in humans and other primates (Bengtsson et al., 2005). Furthermore, learning a new motor skill can modify the motor cortex on a shorter time scale. Human subjects practicing left hand finger-thumb opposition movement for 4 weeks showed an increase in cortical thickness in the right post-central gyrus, consistent with the motor representation of trained fingers (Sale et al., 2017). Diffusion MRI tractography has further revealed a significantly increased FA in the right CST, with no change in the left CST, in subjects undergoing such training (Reid et al., 2017). A recent study comparing DTI results with myelin water imaging (MWI) suggests that increased myelination plays a central role in motor learning-induced white matter plasticity (Kirby et al., 2022). Similar macroscopic reorganizations in response to motor learning have also been observed in the rodent M1. An early study reports that consistent use of one forelimb in a reaching task increases the thickness of the contralateral M1 in rats (Diaz et al., 1994). The increase in motor cortical thickness has also been observed in rats subjected to motor learning on an obstacle course, but not in those voluntarily running on a wheel or in inactive controls (Anderson et al., 2002). Recently, it was found that learning a dexterous reaching task induces dynamic retraction and formation of myelin sheaths around learning-activated axons in mouse M1 (Bacmeister et al., 2022). Together, these studies suggest that both gray and white matter undergo structural alterations in motor learning.
Interestingly, humans learning a three-ball juggling task for 3 months show a transient gray matter expansion, which decreases over the following 3 months of non-practice (Draganski et al., 2004). Another study on right-handed subjects practicing left-hand writing found that the gray matter volume increased over the first 4 weeks, but then returned to the baseline level either completely or partially (Wenger et al., 2017b). These results have led to the expansion-renormalization model of plastic changes in the human brain accompanying skill acquisition (Wenger et al., 2017a). The model postulates an initial increase of gray matter structure, such as synaptic growth, followed by a selective elimination of “unwanted” surplus structures, which renormalizes the total volume. It resolves a paradox already recognized by Ramón y Cajal: “[How] can the volume of the brain be maintained if there is a multiplication and a new formation of small terminal branches of dendrites and axonal collaterals?” (Ramón y Cajal, 1894). It also broadly agrees with the observation of learning-associated structural dynamics of synapses in rodents in vivo (see below).
Many studies have attempted to determine how motor commands are encoded at the level of individual neurons or neuronal populations. Evidence abounds that single neurons in primate M1 may encode a variety of kinematic parameters related to movement, such as position (Georgopoulos et al., 1984; Paninski et al., 2004), direction of movement (Georgopoulos et al., 1982), amplitude (Messier and Kalaska, 2000), and acceleration (Ashe and Georgopoulos, 1994). Indeed, a large variable set of finger movements may be decoded from the activity patterns of a relatively small number of neurons in monkey M1 (Ben Hamed et al., 2007). However, as the activity of single M1 neurons may correlate with a wide range of movement parameters and change across behaviors, it leads some researchers to speculate that the apparent “tuning” of individual M1 neuron’s activity to specific movement variables is but coincidental; that it would be more natural to view M1 as a dynamical system that generates and controls movements, in which the activity of individual neurons evolves as part of the system-wide dynamics (Scott, 2008; Churchland et al., 2012). As succinctly put in a Perspective article, “the ultimate role of M1 is to generate movement, not to represent it” (Gallego et al., 2017). An emerging idea is that the parameters to generate movements are encoded in the activity pattern of populations of neurons, which may lie on a low-dimensional manifold (Shenoy et al., 2013; Gallego et al., 2017; Vyas et al., 2020). According to this idea, motor learning would involve a gradual shift in the population activity patterns that leads to the optimization of the motor output.
Previous studies have shown that motor learning leads to functional reorganization of M1, i.e., changes in the topography of motor representations. An fMRI study (Karni et al., 1995) on human subjects learning a motor sequence (finger opposition movement) showed that the activated region in M1 grew as learning proceeded, and after weeks of practice, the active area remained large compared to areas activated upon learning a new task. In squirrel monkeys, learning two tasks–small object retrieval, which requires digit movement, and eyebolt-turning, which requires supination and pronation of the hand–differentially alters the topographic map in M1, as determined by intracortical microstimulation (Nudo et al., 1996). This is corroborated by the finding that skilled reaching (pellet retrieval) elicits an increase in the area of wrist and digit representation in rat M1, whereas unskilled reaching (bar pressing) does not (Kleim et al., 1998). The expansion of distal forelimb representation occurs only after 10 days of training, following significant synaptogenesis (Kleim et al., 2004). On the other hand, as learning progresses, M1 displays the same or even reduced global activation level. A human fMRI study (Wiestler and Diedrichsen, 2013) found that highly distinguishable activation patterns emerged in M1 for trained finger movement sequences without an increase in average activity.
To determine how M1 population activity pattern evolves during motor learning with single neuron resolution, Peters et al. performed in vivo two-photon (2P) calcium imaging of L2/3 neurons in the mouse M1 during a lever-press task over 2 weeks (Peters et al., 2014). They found that, during the initial phase of learning, the population of active excitatory neurons expanded and exhibited a variety of activity patterns even when executing similar movements; as learning proceeded, this was refined to a smaller population with more reproducible activity patterns. A later study by the same group (Hwang et al., 2019) confirmed that M1 L2/3 population activity became more consistent across trials from early- to mid-stage of learning a joystick-press task, as kinematic stereotypy rapidly increased. However, over prolonged training (2 months), M1 population activity became again variable despite expert-level performance, which is consistent with the disengagement of M1 from controlling well-established movements. Recently, Hwang et al. further tested this idea by training mice on two tasks requiring distinct sets of muscles (forward vs. downward joystick press) over long term. They found that of the two tasks, the one in which the mouse achieved higher trial-to-trial consistency activated fewer M1 L2/3 neurons, producing weaker and less consistent population activity, and was less affected by optogenetic inactivation of M1 (Hwang et al., 2021). Apparently, M1 relinquishes the control over movements that have achieved perfection through practice.
It is widely accepted that the activity pattern of neurons is fundamentally determined by their synaptic connectivity. A corollary of this idea is that changes in synaptic connections underlie the evolution of neural activity patterns throughout the learning process. Indeed, Ramón y Cajal suggested this possibility as early as in 1894. In the Croonian Lecture delivered before the Royal Society of London, he perspicaciously remarked that, in a use-dependent manner, “associations already established among certain groups of cells would be notably reinforced by means of the multiplication of the small terminal branches of the dendritic appendages and axonal collaterals; but, in addition, completely new intercellular connections could be established thanks to the new formation of [axonal] collaterals and dendrites” (Ramón y Cajal, 1894). Essentially, he envisages that structural plasticity in the synaptic circuit serves as the anatomical substrate of learning.
In the context of motor learning, evidence supporting this idea in M1 emerged almost a century later. In 1996, Greenough et al. used serial electron microscopy (EM) to examine M1 L2/3 neurons in rats trained on acrobatics, and found that their synapse density increased from skill acquisition to the maintenance phase of learning (Kleim et al., 1996). Consistent with this, in rats trained on a pellet reaching task, synapse number per M1 neuron significantly increased at day 7 and day 10 of learning compared to the pre-learning level (Kleim et al., 2004). While such fixed-tissue studies have yielded invaluable information about learning-associated synaptic changes, they cannot follow the dynamic process. The advent of two-photon microscopy (Denk et al., 1990) and transgenic mice with cortical neurons sparsely labeled by fluorescent proteins (Feng et al., 2000) enable researchers to interrogate the structural dynamics of synapse changes in the living brain. Focusing on dendritic spines – tiny protrusions that host the postsynaptic sites of most excitatory synapses in the mammalian cortex – on apical dendrites of L5 PyrNs in sensory cortices, several pioneering works (Grutzendler et al., 2002; Trachtenberg et al., 2002; Zuo et al., 2005; Holtmaat et al., 2006) discovered a rich dynamics of spine formation and elimination under baseline conditions and in response to sensory manipulations. Leveraging the same imaging technique, Xu et al. found a rapid emergence of new spines on M1 L5 PyrNs in mice trained on a pellet reaching task; continued training preferentially stabilized such learning-induced spines, which persisted long after the cessation of training (Xu et al., 2009). A similar phenomenon was reported in mice receiving rotarod training (Yang et al., 2009).
Moreover, spine formation and stabilization are correlated with the acquisition (Xu et al., 2009) and retention (Yang et al., 2009) of learned motor skills. Subsequent studies further demonstrated the positive correlation between spine dynamics and motor learning by molecular or genetic tools. For example, intrathecal application of antibodies against Nogo-A (a membrane protein best known as an inhibitor of axonal outgrowth and regeneration in the central nervous system) increases spine formation and improves learning of single-pellet reaching (Zemmar et al., 2014). Mice over-expressing KCC2 (a neuronal K+-Cl− co-transporter) in forebrain excitatory neurons show increased spine formation rate during rotarod training, which is correlated with faster learning and better performance outcome (Nakamura et al., 2019). Similarly, paired immunoglobulin receptor B knockout (PirB−/−) mice have increased spine formation rate as well as lower spine elimination rate, and learn skilled reaching faster. Notably, acute blockage of PirB signaling by infusion of a recombinant soluble PirB decoy receptor in wild-type mice recapitulates the spine dynamics phenotype and learning improvement observed in PirB−/− mice, suggesting that the effect is not due to a developmental alteration in the neural circuit (Albarran et al., 2021). To move beyond correlation and establish the causal relationship between the structural potentiation of spines (formation and enlargement) and motor learning, Hayashi-Takagi et al. devised a novel “optoprobe” called AS-PaRac1, which is targeted to potentiated spines and can induce spine shrinkage when activated by light. They expressed AS-PaRac1 in M1 and trained the mice to run on the rotarod. Light activation of AS-PaRac1 after learning caused the potentiated spines to shrink and impaired the animal’s performance on the rotarod. The effect is task-specific, as AS-PaRac1 activation of spines potentiated during beam walking or spontaneously during other movements did not interfere with rotarod performance. Thus, this study provides the first direct evidence that structural changes in M1 synaptic connections are necessary for motor learning (Hayashi-Takagi et al., 2015).
Furthermore, new spines associated with motor learning do not form randomly along the dendrite, but exhibit distinct spatial patterns. On L5 PyrNs, new spines form in clusters in response to motor learning, and the emergence of the second spine in the cluster is associated with the enlargement of the first spine (Fu et al., 2012). Recently, an elegant study (Hedrick et al., 2022) used in vivo glutamate imaging to reveal the potentiation of clustered spines exhibiting task-related activity during motor learning. Correlative EM reconstruction suggests that these functional clusters are hotspots for filopodia outgrowth to establish new connections with axons. The survival of the new spines depends on their co-activity with task-related spines in the vicinity, consistent with an earlier report that in the developing visual cortex, synapses “out of sync” with their neighbors are weakened over time (Winnubst et al., 2015). Theoretical studies have suggested that such clusters of synapses sharing similar activity patterns would enable the neuron to fully exploit the information processing capacity offered by dendritic nonlinearities (Kastellakis et al., 2023).
While the majority of in vivo imaging studies have focused on dendritic spines on M1 PyrNs, some researchers have endeavored to examine their inputs. Hasegawa et al. imaged the structural dynamics of axonal boutons in mouse M1 L1 during rotarod learning. They found an increased rate of bouton formation on axons from M2 from training day 2 to 4, and a decreased rate of bouton elimination on axons from the motor thalamus from training day 4 to 7 (Hasegawa et al., 2020). Combining in vivo 2P imaging with post hoc immunohistochemical validation, Sohn et al. found that motor learning-induced new spines receiving CC inputs tend to be transient, whereas those receiving TC inputs tend to enlarge and persist (Sohn et al., 2022). Together these works suggest that the transient CC connections facilitate motor learning, while the persistent TC connections store the established motor memory.
The importance of inhibitory circuits in motor learning has been recognized for a long time. As astutely observed by Sir Charles S. Sherrington, “to refrain from an act is no less an act than to commit one, because inhibition is coequally with excitation a nervous activity” (Sherrington, 1933). In the mammalian brain, gamma-amino butyric acid (GABA) is the primary inhibitory neurotransmitter. To probe the role of inhibitory neurotransmission in motor learning, human studies have used Magnetic Resonance Spectroscopy (MRS) to measure local GABA level. One such study showed that anodal tDCS delivered to M1 induces a significant reduction in local GABA concentration, which is correlated with better performance in learning a force adaptation task (Kim et al., 2014). Other studies have probed inhibitory circuits by paired-pulse TMS (Kujirai et al., 1993; Ljubisavljevic, 2006). When a subthreshold conditioning stimulus delivered to M1 is followed by a suprathreshold test stimulus with a 1–6 ms inter-stimulus interval, the electromyography (EMG) response evoked by the test stimulus is inhibited. This phenomenon is known as short interval intracortical inhibition (SICI) and is considered a measure of GABAA receptor-mediated inhibition (Ziemann et al., 1996a,b; Di Lazzaro et al., 2000, 2006, 2007). Several studies have reported a decrease in SICI associated with motor learning (Liepert et al., 1998; Perez et al., 2004; Smyth et al., 2010; Dupont-Hadwen et al., 2019), indicating a transient reduction in GABAergic inhibition putatively mediated through the GABAA receptor. Taken together, these studies suggest that modulation of the inhibitory tone in M1 is crucial for human motor learning.
Rodent models enable researchers to dissect the contribution of inhibitory circuits to motor learning with cellular specificity. There are three major classes of inhibitory interneurons (INs) in the cerebral cortex: those expressing parvalbumin (PV+), somatostatin (SST+), and the serotonin receptor 5HT3aR. Among the 5HT3aR group, the most abundant are those expressing vasoactive intestinal peptide (VIP+) (Lim et al., 2018). Distinct subtypes of INs selectively synapse onto different domains of excitatory neurons as well as each other. For example, PV+ INs provide inhibition to the peri-somatic region of PyrNs, whereas SST+ INs inhibit distal dendrites of PyrNs (Fishell and Kepecs, 2020). A recent study combining rabies virus-based monosynaptic tracing and channelrhodopsin-assisted circuit mapping further shows that different types of M1 INs also exhibit distinct laminar profiles of inputs (Okoro et al., 2022). Using transgenic mouse lines that express Cre recombinase in specific subtypes of INs, Chen et al. found a subtype-specific plasticity of M1 inhibitory circuits during motor learning: axonal boutons of SST+ INs undergo elimination upon motor training initiation, while the number of axonal boutons of PV+ INs gradually increases through training (Chen et al., 2015).
The importance of SST+ INs in learning-associated synaptic reorganization is highlighted in other studies. While acute ablation or chemogenetic silencing of SST+ INs disrupts the branch-specificity of dendritic Ca2+ spikes and the associated synaptic potentiation on L5 PyrNs (Cichon and Gan, 2015), activating SST+ INs during motor training prevents learning-induced sequential activities of M1 L2/3 PyrNs and behavioral improvement (Adler et al., 2019). Notably, two recent studies suggest a transient reduction in SST+ IN activity level during the early phase of motor learning (Ren et al., 2022; Yang et al., 2022). This is reminiscent of an earlier study (Donato et al., 2013) that with environmental enrichment, a larger fraction of PV+ INs in the adult hippocampus show low PV expression; this state is related to enhanced structural synaptic plasticity, memory consolidation and retrieval. Together with human research, these studies suggest that a reduction in the inhibitory tone is prerequisite to the acquisition of a new motor skill.
Motor learning is a ubiquitous capability of mammals. While motor learning induces a plethora of concerted changes from the molecular to the systems level not only in M1 but also in other movement-related areas such as the anterior lateral motor cortex (ALM), M2, the striatum, and the cerebellum, structural and functional plasticity of the synaptic circuit is emerging as the leitmotif. At the same time, different species exhibit specific anatomical and physiological features dictated by their unique ethology and evolutionary history. Elucidating such variations on the common theme will greatly deepen our understanding of the neurobiological and computational basis of motor learning. To promote such cross-species studies, it is highly desirable to devise behavioral paradigms that are applicable to different species. Comparative studies on the transcriptomics, epigenetics, neuronal morphology, electrophysiology, and connectomics (Beaulieu-Laroche et al., 2018, 2021; Bakken et al., 2021; BRAIN Initiative Cell Census Network (BICCN), 2021; Loomba et al., 2022) will help distinguish conserved core features from species-specific innovations. Finally, there is a conspicuous gap in the accessible spatiotemporal scales in human vs. animal experiments. Many invasive techniques for probing and modifying the neural circuit at the molecular and cellular levels are not applicable to human studies for ethical reasons. Thus, it is important to develop novel non-invasive methods to study the human brain with synaptic and cellular resolution in vivo. Conversely, research on rodents and non-human primates very often focuses on one small subset of cells in one brain region at a time; optical and electrophysiological methods that retain the high resolution but cover larger volumes will be highly informative. Achieving these goals will require dialogues between neuroscientists working on humans and animal models, as well as interdisciplinary collaborations with experts in other branches of physical, biological, and applied sciences.
EK: Conceptualization, Writing – original draft. JL: Conceptualization, Writing – original draft, Writing – review & editing. YZ: Conceptualization, Funding acquisition, Supervision, Writing – review & editing.
The author(s) declare financial support was received for the research, authorship, and/or publication of this article. This work was supported by grants from the National Institute of Mental Health (R01MH127737), the National Institute on Aging (R01AG071787), and a Max Planck Fellowship at the Max Planck Florida Institute for Neuroscience to YZ.
We thank Euiseok Kim and Shaorong Ma for critical comments on this manuscript.
The authors declare that the research was conducted in the absence of any commercial or financial relationships that could be construed as a potential conflict of interest.
All claims expressed in this article are solely those of the authors and do not necessarily represent those of their affiliated organizations, or those of the publisher, the editors and the reviewers. Any product that may be evaluated in this article, or claim that may be made by its manufacturer, is not guaranteed or endorsed by the publisher.
Adler, A., Zhao, R., Shin, M. E., Yasuda, R., and Gan, W. B. (2019). Somatostatin-expressing interneurons enable and maintain learning-dependent sequential activation of pyramidal neurons. Neuron 102, 202–216.e7. doi: 10.1016/j.neuron.2019.01.036
Albarran, E., Raissi, A., Jaidar, O., Shatz, C. J., and Ding, J. B. (2021). Enhancing motor learning by increasing the stability of newly formed dendritic spines in the motor cortex. Neuron 109, 3298–3311.e4. doi: 10.1016/j.neuron.2021.07.030
Anderson, B. J., Eckburg, P. B., and Relucio, K. I. (2002). Alterations in the thickness of motor cortical subregions after motor-skill learning and exercise. Learn. Mem. 9, 1–9. doi: 10.1101/lm.43402
Ashburner, J., and Friston, K. J. (2000). Voxel-based morphometry—the methods. NeuroImage 11, 805–821. doi: 10.1006/nimg.2000.0582
Ashe, J., and Georgopoulos, A. P. (1994). Movement parameters and neural activity in motor cortex and area 5. Cereb. Cortex 4, 590–600. doi: 10.1093/cercor/4.6.590
Bacmeister, C. M., Huang, R., Osso, L. A., Thornton, M. A., Conant, L., Chavez, A. R., et al. (2022). Motor learning drives dynamic patterns of intermittent myelination on learning-activated axons. Nat. Neurosci. 25, 1300–1313. doi: 10.1038/s41593-022-01169-4
Baker, A., Kalmbach, B., Morishima, M., Kim, J., Juavinett, A., Li, N., et al. (2018). Specialized subpopulations of deep-layer pyramidal neurons in the neocortex: bridging cellular properties to functional consequences. J. Neurosci. 38, 5441–5455. doi: 10.1523/JNEUROSCI.0150-18.2018
Bakken, T. E., Jorstad, N. L., Hu, Q., Lake, B. B., Tian, W., Kalmbach, B. E., et al. (2021). Comparative cellular analysis of motor cortex in human, marmoset and mouse. Nature 598, 111–119. doi: 10.1038/s41586-021-03465-8
Beaulieu-Laroche, L., Brown, N. J., Hansen, M., Toloza, E. H. S., Sharma, J., Williams, Z. M., et al. (2021). Allometric rules for mammalian cortical layer 5 neuron biophysics. Nature 600, 274–278. doi: 10.1038/s41586-021-04072-3
Beaulieu-Laroche, L., Toloza, E. H. S., van der Goes, M. S., Lafourcade, M., Barnagian, D., Williams, Z. M., et al. (2018). Enhanced dendritic compartmentalization in human cortical neurons. Cells 175, 643–651.e14. doi: 10.1016/j.cell.2018.08.045
Ben Hamed, S., Schieber, M. H., and Pouget, A. (2007). Decoding M1 neurons during multiple finger movements. J. Neurophysiol. 98, 327–333. doi: 10.1152/jn.00760.2006
Bengtsson, S. L., Nagy, Z., Skare, S., Forsman, L., Forssberg, H., and Ullen, F. (2005). Extensive piano practicing has regionally specific effects on white matter development. Nat. Neurosci. 8, 1148–1150. doi: 10.1038/nn1516
BRAIN Initiative Cell Census Network (BICCN) (2021). A multimodal cell census and atlas of the mammalian primary motor cortex. Nature 598, 86–102. doi: 10.1038/s41586-021-03950-0
Bopp, R., Holler-Rickauer, S., Martin, K. A., and Schuhknecht, G. F. (2017). An ultrastructural study of the thalamic input to layer 4 of primary motor and primary somatosensory cortex in the mouse. J. Neurosci. 37, 2435–2448. doi: 10.1523/JNEUROSCI.2557-16.2017
Castro, A. J. (1972). The effects of cortical ablations on digital usage in the rat. Brain Res. 37, 173–185. doi: 10.1016/0006-8993(72)90665-8
Chen, S. X., Kim, A. N., Peters, A. J., and Komiyama, T. (2015). Subtype-specific plasticity of inhibitory circuits in motor cortex during motor learning. Nat. Neurosci. 18, 1109–1115. doi: 10.1038/nn.4049
Churchland, M. M., Cunningham, J. P., Kaufman, M. T., Foster, J. D., Nuyujukian, P., Ryu, S. I., et al. (2012). Neural population dynamics during reaching. Nature 487, 51–56. doi: 10.1038/nature11129
Cichon, J., and Gan, W. B. (2015). Branch-specific dendritic ca(2+) spikes cause persistent synaptic plasticity. Nature 520, 180–185. doi: 10.1038/nature14251
Denk, W., Strickler, J. H., and Webb, W. W. (1990). Two-photon laser scanning fluorescence microscopy. Science 248, 73–76. doi: 10.1126/science.2321027
Di Lazzaro, V., Oliviero, A., Meglio, M., Cioni, B., Tamburrini, G., Tonali, P., et al. (2000). Direct demonstration of the effect of lorazepam on the excitability of the human motor cortex. Clin. Neurophysiol. 111, 794–799. doi: 10.1016/S1388-2457(99)00314-4
Di Lazzaro, V., Pilato, F., Dileone, M., Profice, P., Ranieri, F., Ricci, V., et al. (2007). Segregating two inhibitory circuits in human motor cortex at the level of GABAA receptor subtypes: a TMS study. Clin. Neurophysiol. 118, 2207–2214. doi: 10.1016/j.clinph.2007.07.005
Di Lazzaro, V., Pilato, F., Dileone, M., Ranieri, F., Ricci, V., Profice, P., et al. (2006). GABAA receptor subtype specific enhancement of inhibition in human motor cortex. J. Physiol. 575, 721–726. doi: 10.1113/jphysiol.2006.114694
Diaz, E., Pinto-Hamuy, T., and Fernandez, V. (1994). Interhemispheric structural asymmetry induced by a lateralized reaching task in the rat motor cortex. Eur. J. Neurosci. 6, 1235–1238. doi: 10.1111/j.1460-9568.1994.tb00622.x
Donato, F., Rompani, S. B., and Caroni, P. (2013). Parvalbumin-expressing basket-cell network plasticity induced by experience regulates adult learning. Nature 504, 272–276. doi: 10.1038/nature12866
Douglas, R. J., and Martin, K. A. (2004). Neuronal circuits of the neocortex. Annu. Rev. Neurosci. 27, 419–451. doi: 10.1146/annurev.neuro.27.070203.144152
Draganski, B., Gaser, C., Busch, V., Schuierer, G., Bogdahn, U., and May, A. (2004). Neuroplasticity: changes in grey matter induced by training. Nature 427, 311–312. doi: 10.1038/427311a
Dupont-Hadwen, J., Bestmann, S., and Stagg, C. J. (2019). Motor training modulates intracortical inhibitory dynamics in motor cortex during movement preparation. Brain Stimul. 12, 300–308. doi: 10.1016/j.brs.2018.11.002
Feng, G., Mellor, R. H., Bernstein, M., Keller-Peck, C., Nguyen, Q. T., Wallace, M., et al. (2000). Imaging neuronal subsets in transgenic mice expressing multiple spectral variants of GFP. Neuron 28, 41–51. doi: 10.1016/S0896-6273(00)00084-2
Fishell, G., and Kepecs, A. (2020). Interneuron types as attractors and controllers. Annu. Rev. Neurosci. 43, 1–30. doi: 10.1146/annurev-neuro-070918-050421
Franz, S. I., and Lashley, K. S. (1917). The retention of habits by the rat after destruction of the frontal portion of the cerebrum. Psychobiology 1, 3–18. doi: 10.1037/h0074177
Fu, M., Yu, X., Lu, J., and Zuo, Y. (2012). Repetitive motor learning induces coordinated formation of clustered dendritic spines in vivo. Nature 483, 92–95. doi: 10.1038/nature10844
Fukuo, M., Kamagata, K., Kuramochi, M., Andica, C., Tomita, H., Waki, H., et al. (2020). Regional brain gray matter volume in world-class artistic gymnasts. J. Physiol. Sci. 70:43. doi: 10.1186/s12576-020-00767-w
Gallego, J. A., Perich, M. G., Miller, L. E., and Solla, S. A. (2017). Neural manifolds for the control of movement. Neuron 94, 978–984. doi: 10.1016/j.neuron.2017.05.025
Garcia-Cabezas, M. A., and Barbas, H. (2014). Area 4 has layer IV in adult primates. Eur. J. Neurosci. 39, 1824–1834. doi: 10.1111/ejn.12585
Gaser, C., and Schlaug, G. (2003). Brain structures differ between musicians and non-musicians. J. Neurosci. 23, 9240–9245. doi: 10.1523/JNEUROSCI.23-27-09240.2003
Georgopoulos, A. P., Caminiti, R., and Kalaska, J. F. (1984). Static spatial effects in motor cortex and area 5: quantitative relations in a two-dimensional space. Exp. Brain Res. 54, 446–454.
Georgopoulos, A. P., Kalaska, J. F., Caminiti, R., and Massey, J. T. (1982). On the relations between the direction of two-dimensional arm movements and cell discharge in primate motor cortex. J. Neurosci. 2, 1527–1537. doi: 10.1523/JNEUROSCI.02-11-01527.1982
Geyer, S., Matelli, M., Luppino, G., and Zilles, K. (2000). Functional neuroanatomy of the primate isocortical motor system. Anat. Embryol. (Berl) 202, 443–474. doi: 10.1007/s004290000127
Grutzendler, J., Kasthuri, N., and Gan, W. B. (2002). Long-term dendritic spine stability in the adult cortex. Nature 420, 812–816. doi: 10.1038/nature01276
Gu, Z., Kalambogias, J., Yoshioka, S., Han, W., Li, Z., Kawasawa, Y. I., et al. (2017). Control of species-dependent cortico-motoneuronal connections underlying manual dexterity. Science 357, 400–404. doi: 10.1126/science.aan3721
Hallett, M. (2000). Transcranial magnetic stimulation and the human brain. Nature 406, 147–150. doi: 10.1038/35018000
Harris, K. D., and Shepherd, G. M. (2015). The neocortical circuit: themes and variations. Nat. Neurosci. 18, 170–181. doi: 10.1038/nn.3917
Hasegawa, R., Ebina, T., Tanaka, Y. R., Kobayashi, K., and Matsuzaki, M. (2020). Structural dynamics and stability of corticocortical and thalamocortical axon terminals during motor learning. PLoS One 15:e0234930. doi: 10.1371/journal.pone.0234930
Hayashi-Takagi, A., Yagishita, S., Nakamura, M., Shirai, F., Wu, Y. I., Loshbaugh, A. L., et al. (2015). Labelling and optical erasure of synaptic memory traces in the motor cortex. Nature 525, 333–338. doi: 10.1038/nature15257
Hedrick, N. G., Lu, Z., Bushong, E., Singhi, S., Nguyen, P., Magana, Y., et al. (2022). Learning binds new inputs into functional synaptic clusters via spinogenesis. Nat. Neurosci. 25, 726–737. doi: 10.1038/s41593-022-01086-6
Hira, R., Ohkubo, F., Tanaka, Y. R., Masamizu, Y., Augustine, G. J., Kasai, H., et al. (2013). In vivo optogenetic tracing of functional corticocortical connections between motor forelimb areas. Front. Neural Circuits 7:55. doi: 10.3389/fncir.2013.00055
Holtmaat, A., Wilbrecht, L., Knott, G. W., Welker, E., and Svoboda, K. (2006). Experience-dependent and cell-type-specific spine growth in the neocortex. Nature 441, 979–983. doi: 10.1038/nature04783
Hooks, B. M., Lin, J. Y., Guo, C., and Svoboda, K. (2015). Dual-channel circuit mapping reveals sensorimotor convergence in the primary motor cortex. J. Neurosci. 35, 4418–4426. doi: 10.1523/JNEUROSCI.3741-14.2015
Hooks, B. M., Mao, T., Gutnisky, D. A., Yamawaki, N., Svoboda, K., and Shepherd, G. M. G. (2013). Organization of cortical and thalamic input to pyramidal neurons in mouse motor cortex. J. Neurosci. 33, 748–760. doi: 10.1523/JNEUROSCI.4338-12.2013
Hooks, B. M., Papale, A. E., Paletzki, R. F., Feroze, M. W., Eastwood, B. S., Couey, J. J., et al. (2018). Topographic precision in sensory and motor corticostriatal projections varies across cell type and cortical area. Nat. Commun. 9:3549. doi: 10.1038/s41467-018-05780-7
Hwang, E. J., Dahlen, J. E., Hu, Y. Y., Aguilar, K., Yu, B., Mukundan, M., et al. (2019). Disengagement of motor cortex from movement control during long-term learning. Sci. Adv. 5:eaay0001. doi: 10.1126/sciadv.aay0001
Hwang, E. J., Dahlen, J. E., Mukundan, M., and Komiyama, T. (2021). Disengagement of motor cortex during long-term learning tracks the performance level of learned movements. J. Neurosci. 41, 7029–7047. doi: 10.1523/JNEUROSCI.3049-20.2021
Karni, A., Meyer, G., Jezzard, P., Adams, M. M., Turner, R., and Ungerleider, L. G. (1995). Functional MRI evidence for adult motor cortex plasticity during motor skill learning. Nature 377, 155–158.
Kastellakis, G., Tasciotti, S., Pandi, I., and Poirazi, P. (2023). The dendritic engram. Front. Behav. Neurosci. 17:1212139. doi: 10.3389/fnbeh.2023.1212139
Kawai, R., Markman, T., Poddar, R., Ko, R., Fantana, A. L., Dhawale, A. K., et al. (2015). Motor cortex is required for learning but not for executing a motor skill. Neuron 86, 800–812. doi: 10.1016/j.neuron.2015.03.024
Kim, S., Stephenson, M. C., Morris, P. G., and Jackson, S. R. (2014). tDCS-induced alterations in GABA concentration within primary motor cortex predict motor learning and motor memory: a 7 T magnetic resonance spectroscopy study. NeuroImage 99, 237–243. doi: 10.1016/j.neuroimage.2014.05.070
Kirby, E. D., Frizzell, T. O., Grajauskas, L. A., Song, X., Gawryluk, J. R., Lakhani, B., et al. (2022). Increased myelination plays a central role in white matter neuroplasticity. NeuroImage 263:119644. doi: 10.1016/j.neuroimage.2022.119644
Kleim, J. A., Barbay, S., and Nudo, R. J. (1998). Functional reorganization of the rat motor cortex following motor skill learning. J. Neurophysiol. 80, 3321–3325. doi: 10.1152/jn.1998.80.6.3321
Kleim, J. A., Hogg, T. M., VandenBerg, P. M., Cooper, N. R., Bruneau, R., and Remple, M. (2004). Cortical synaptogenesis and motor map reorganization occur during late, but not early, phase of motor skill learning. J. Neurosci. 24, 628–633. doi: 10.1523/JNEUROSCI.3440-03.2004
Kleim, J. A., Lussnig, E., Schwarz, E. R., Comery, T. A., and Greenough, W. T. (1996). Synaptogenesis and Fos expression in the motor cortex of the adult rat after motor skill learning. J. Neurosci. 16, 4529–4535. doi: 10.1523/JNEUROSCI.16-14-04529.1996
Kornysheva, K., and Diedrichsen, J. (2014). Human premotor areas parse sequences into their spatial and temporal features. elife 3:e03043. doi: 10.7554/eLife.03043
Krubitzer, L. (2007). The magnificent compromise: cortical field evolution in mammals. Neuron 56, 201–208. doi: 10.1016/j.neuron.2007.10.002
Kujirai, T., Caramia, M. D., Rothwell, J. C., Day, B. L., Thompson, P. D., Ferbert, A., et al. (1993). Corticocortical inhibition in human motor cortex. J. Physiol. 471, 501–519. doi: 10.1113/jphysiol.1993.sp019912
Kuramoto, E., Ohno, S., Furuta, T., Unzai, T., Tanaka, Y. R., Hioki, H., et al. (2015). Ventral medial nucleus neurons send thalamocortical afferents more widely and more preferentially to layer 1 than neurons of the ventral anterior-ventral lateral nuclear complex in the rat. Cereb. Cortex 25, 221–235. doi: 10.1093/cercor/bht216
Lawrence, D. G., and Kuypers, H. G. (1968). The functional organization of the motor system in the monkey. I. The effects of bilateral pyramidal lesions. Brain 91, 1–14. doi: 10.1093/brain/91.1.1
Le Bihan, D. (2003). Looking into the functional architecture of the brain with diffusion MRI. Nat. Rev. Neurosci. 4, 469–480. doi: 10.1038/nrn1119
Lemon, R. N. (2008). Descending pathways in motor control. Annu. Rev. Neurosci. 31, 195–218. doi: 10.1146/annurev.neuro.31.060407.125547
Liepert, J., Classen, J., Cohen, L. G., and Hallett, M. (1998). Task-dependent changes of intracortical inhibition. Exp. Brain Res. 118, 421–426. doi: 10.1007/s002210050296
Lim, L., Mi, D., Llorca, A., and Marin, O. (2018). Development and functional diversification of cortical interneurons. Neuron 100, 294–313. doi: 10.1016/j.neuron.2018.10.009
Ljubisavljevic, M. (2006). Transcranial magnetic stimulation and the motor learning-associated cortical plasticity. Exp. Brain Res. 173, 215–222. doi: 10.1007/s00221-006-0538-z
Loomba, S., Straehle, J., Gangadharan, V., Heike, N., Khalifa, A., Motta, A., et al. (2022). Connectomic comparison of mouse and human cortex. Science 377:eabo0924. doi: 10.1126/science.abo0924
Maier, N. R. F. (1935). The cortical area concerned with coordinated walking in the rat. J. Comp. Neurol. 61, 395–405. doi: 10.1002/cne.900610209
Mao, T., Kusefoglu, D., Hooks, B. M., Huber, D., Petreanu, L., and Svoboda, K. (2011). Long-range neuronal circuits underlying the interaction between sensory and motor cortex. Neuron 72, 111–123. doi: 10.1016/j.neuron.2011.07.029
Messier, J., and Kalaska, J. F. (2000). Covariation of primate dorsal premotor cell activity with direction and amplitude during a memorized-delay reaching task. J. Neurophysiol. 84, 152–165. doi: 10.1152/jn.2000.84.1.152
Muellbacher, W., Ziemann, U., Wissel, J., Dang, N., Kofler, M., Facchini, S., et al. (2002). Early consolidation in human primary motor cortex. Nature 415, 640–644. doi: 10.1038/nature712
Munoz-Castaneda, R., Zingg, B., Matho, K. S., Chen, X., Wang, Q., Foster, N. N., et al. (2021). Cellular anatomy of the mouse primary motor cortex. Nature 598, 159–166. doi: 10.1038/s41586-021-03970-w
Murabe, N., Mori, T., Fukuda, S., Isoo, N., Ohno, T., Mizukami, H., et al. (2018). Higher primate-like direct corticomotoneuronal connections are transiently formed in a juvenile subprimate mammal. Sci. Rep. 8:16536. doi: 10.1038/s41598-018-34961-z
Nagai, H., de Vivo, L., Bellesi, M., Ghilardi, M. F., Tononi, G., and Cirelli, C. (2017). Sleep consolidates motor learning of complex movement sequences in mice. Sleep 40:zsw059. doi: 10.1093/sleep/zsw059
Nakamura, K., Moorhouse, A. J., Cheung, D. L., Eto, K., Takeda, I., Rozenbroek, P. W., et al. (2019). Overexpression of neuronal K(+)-Cl(−) co-transporter enhances dendritic spine plasticity and motor learning. J. Physiol. Sci. 69, 453–463. doi: 10.1007/s12576-018-00654-5
Narayana, S., Zhang, W., Rogers, W., Strickland, C., Franklin, C., Lancaster, J. L., et al. (2014). Concurrent TMS to the primary motor cortex augments slow motor learning. NeuroImage 85, 971–984. doi: 10.1016/j.neuroimage.2013.07.024
Nitsche, M. A., Schauenburg, A., Lang, N., Liebetanz, D., Exner, C., Paulus, W., et al. (2003). Facilitation of implicit motor learning by weak transcranial direct current stimulation of the primary motor cortex in the human. J. Cogn. Neurosci. 15, 619–626. doi: 10.1162/089892903321662994
Nudo, R. J., Milliken, G. W., Jenkins, W. M., and Merzenich, M. M. (1996). Use-dependent alterations of movement representations in primary motor cortex of adult squirrel monkeys. J. Neurosci. 16, 785–807. doi: 10.1523/JNEUROSCI.16-02-00785.1996
Okoro, S. U., Goz, R. U., Njeri, B. W., Harish, M., Ruff, C. F., Ross, S. E., et al. (2022). Organization of cortical and thalamic input to inhibitory neurons in mouse motor cortex. J. Neurosci. 42, 8095–8112. doi: 10.1523/JNEUROSCI.0950-22.2022
Paninski, L., Fellows, M. R., Hatsopoulos, N. G., and Donoghue, J. P. (2004). Spatiotemporal tuning of motor cortical neurons for hand position and velocity. J. Neurophysiol. 91, 515–532. doi: 10.1152/jn.00587.2002
Passingham, R. E., Perry, V. H., and Wilkinson, F. (1983). The long-term effects of removal of sensorimotor cortex in infant and adult rhesus monkeys. Brain 106, 675–705. doi: 10.1093/brain/106.3.675
Perez, M. A., Lungholt, B. K., Nyborg, K., and Nielsen, J. B. (2004). Motor skill training induces changes in the excitability of the leg cortical area in healthy humans. Exp. Brain Res. 159, 197–205. doi: 10.1007/s00221-004-1947-5
Peters, A. J., Chen, S. X., and Komiyama, T. (2014). Emergence of reproducible spatiotemporal activity during motor learning. Nature 510, 263–267. doi: 10.1038/nature13235
Piecharka, D. M., Kleim, J. A., and Whishaw, I. Q. (2005). Limits on recovery in the corticospinal tract of the rat: partial lesions impair skilled reaching and the topographic representation of the forelimb in motor cortex. Brain Res. Bull. 66, 203–211. doi: 10.1016/j.brainresbull.2005.04.013
Raichle, M. E. (1983). Positron emission tomography. Annu. Rev. Neurosci. 6, 249–267. doi: 10.1146/annurev.ne.06.030183.001341
Ramón y Cajal, S. (1894). The Croonian Lecture - La fine Structure des Centres Nerveux. Proc. R. Soc. Lond. 55, 444–468.
Ramón y Cajal, S. (1899). Estudios sobre la corteza cerebral humana. II Estructura de la corteza motriz del hombre y mamíferos superiores. Rev. Trim Microg. 4, 117–200.
Reid, L. B., Sale, M. V., Cunnington, R., Mattingley, J. B., and Rose, S. E. (2017). Brain changes following four weeks of unimanual motor training: evidence from fMRI-guided diffusion MRI tractography. Hum. Brain Mapp. 38, 4302–4312. doi: 10.1002/hbm.23514
Ren, C., Peng, K., Yang, R., Liu, W., Liu, C., and Komiyama, T. (2022). Global and subtype-specific modulation of cortical inhibitory neurons regulated by acetylcholine during motor learning. Neuron 110, 2334–2350.e8. doi: 10.1016/j.neuron.2022.04.031
Sale, M. V., Reid, L. B., Cocchi, L., Pagnozzi, A. M., Rose, S. E., and Mattingley, J. B. (2017). Brain changes following four weeks of unimanual motor training: evidence from behavior, neural stimulation, cortical thickness, and functional MRI. Hum. Brain Mapp. 38, 4773–4787. doi: 10.1002/hbm.23710
Schlaug, G., Knorr, U., and Seitz, R. (1994). Inter-subject variability of cerebral activations in acquiring a motor skill: a study with positron emission tomography. Exp. Brain Res. 98, 523–534.
Scott, S. H. (2008). Inconvenient truths about neural processing in primary motor cortex. J. Physiol. 586, 1217–1224. doi: 10.1113/jphysiol.2007.146068
Seipel, K., and Schmid, V. (2005). Evolution of striated muscle: jellyfish and the origin of triploblasty. Dev. Biol. 282, 14–26. doi: 10.1016/j.ydbio.2005.03.032
Serradj, N., Marino, F., Moreno-Lopez, Y., Bernstein, A., Agger, S., Soliman, M., et al. (2023). Task-specific modulation of corticospinal neuron activity during motor learning in mice. Nat. Commun. 14:2708. doi: 10.1038/s41467-023-38418-4
Shenoy, K. V., Sahani, M., and Churchland, M. M. (2013). Cortical control of arm movements: a dynamical systems perspective. Annu. Rev. Neurosci. 36, 337–359. doi: 10.1146/annurev-neuro-062111-150509
Sherrington, C. S. (1933). The brain and its mechanism. The Rede Lecture delivered before the University of Cambridge, 5 December 1933, Cambridge, Cambridge University Press.
Shmuelof, L., and Krakauer, J. W. (2011). Are we ready for a natural history of motor learning? Neuron 72, 469–476. doi: 10.1016/j.neuron.2011.10.017
Smyth, C., Summers, J. J., and Garry, M. I. (2010). Differences in motor learning success are associated with differences in M1 excitability. Hum. Mov. Sci. 29, 618–630. doi: 10.1016/j.humov.2010.02.006
Sohn, J., Suzuki, M., Youssef, M., Hatada, S., Larkum, M. E., Kawaguchi, Y., et al. (2022). Presynaptic supervision of cortical spine dynamics in motor learning. Sci. Adv. 8:eabm0531. doi: 10.1126/sciadv.abm0531
Steel, A., Song, S., Bageac, D., Knutson, K. M., Keisler, A., Saad, Z. S., et al. (2016). Shifts in connectivity during procedural learning after motor cortex stimulation: a combined transcranial magnetic stimulation/functional magnetic resonance imaging study. Cortex 74, 134–148. doi: 10.1016/j.cortex.2015.10.004
Strick, P. L., Dum, R. P., and Rathelot, J. A. (2021). The cortical motor areas and the emergence of motor skills: a neuroanatomical perspective. Annu. Rev. Neurosci. 44, 425–447. doi: 10.1146/annurev-neuro-070918-050216
Suryanarayana, S. M., Robertson, B., and Grillner, S. (2022). The neural bases of vertebrate motor behaviour through the lens of evolution. Philos. Trans. R. Soc. Lond. Ser. B Biol. Sci. 377:20200521. doi: 10.1098/rstb.2020.0521
Tanaka, Y. H., Tanaka, Y. R., Kondo, M., Terada, S. I., Kawaguchi, Y., and Matsuzaki, M. (2018). Thalamocortical axonal activity in motor cortex exhibits layer-specific dynamics during motor learning. Neuron 100, 244–258.e12. doi: 10.1016/j.neuron.2018.08.016
Trachtenberg, J. T., Chen, B. E., Knott, G. W., Feng, G., Sanes, J. R., Welker, E., et al. (2002). Long-term in vivo imaging of experience-dependent synaptic plasticity in adult cortex. Nature 420, 788–794. doi: 10.1038/nature01273
Ueta, Y., Otsuka, T., Morishima, M., Ushimaru, M., and Kawaguchi, Y. (2014). Multiple layer 5 pyramidal cell subtypes relay cortical feedback from secondary to primary motor areas in rats. Cereb. Cortex 24, 2362–2376. doi: 10.1093/cercor/bht088
Vyas, S., Golub, M. D., Sussillo, D., and Shenoy, K. V. (2020). Computation through neural population dynamics. Annu. Rev. Neurosci. 43, 249–275. doi: 10.1146/annurev-neuro-092619-094115
Wang, X., Vannier, J., Yang, X., Leclere, L., Ou, Q., Song, X., et al. (2022). Muscle systems and motility of early animals highlighted by cnidarians from the basal Cambrian. elife 11:e74716. doi: 10.7554/eLife.74716
Waters, S., Wiestler, T., and Diedrichsen, J. (2017). Cooperation not competition: Bihemispheric tDCS and fMRI show role for ipsilateral hemisphere in motor learning. J. Neurosci. 37, 7500–7512. doi: 10.1523/JNEUROSCI.3414-16.2017
Wenger, E., Brozzoli, C., Lindenberger, U., and Lovden, M. (2017a). Expansion and renormalization of human brain structure during skill acquisition. Trends Cogn. Sci. 21, 930–939. doi: 10.1016/j.tics.2017.09.008
Wenger, E., Kuhn, S., Verrel, J., Martensson, J., Bodammer, N. C., Lindenberger, U., et al. (2017b). Repeated structural imaging reveals nonlinear progression of experience-dependent volume changes in human motor cortex. Cereb. Cortex 27, 2911–2925. doi: 10.1093/cercor/bhw141
Whishaw, I. Q. (2000). Loss of the innate cortical engram for action patterns used in skilled reaching and the development of behavioral compensation following motor cortex lesions in the rat. Neuropharmacology 39, 788–805. doi: 10.1016/S0028-3908(99)00259-2
Whishaw, I. Q., Pellis, S. M., Gorny, B., Kolb, B., and Tetzlaff, W. (1993). Proximal and distal impairments in rat forelimb use in reaching follow unilateral pyramidal tract lesions. Behav. Brain Res. 56, 59–76. doi: 10.1016/0166-4328(93)90022-I
Wiestler, T., and Diedrichsen, J. (2013). Skill learning strengthens cortical representations of motor sequences. elife 2:e00801. doi: 10.7554/eLife.00801
Winnubst, J., Cheyne, J. E., Niculescu, D., and Lohmann, C. (2015). Spontaneous activity drives local synaptic plasticity in Vivo. Neuron 87, 399–410. doi: 10.1016/j.neuron.2015.06.029
Xu, T., Yu, X., Perlik, A. J., Tobin, W. F., Zweig, J. A., Tennant, K., et al. (2009). Rapid formation and selective stabilization of synapses for enduring motor memories. Nature 462, 915–919. doi: 10.1038/nature08389
Yamawaki, N., Borges, K., Suter, B. A., Harris, K. D., and Shepherd, G. M. (2014). A genuine layer 4 in motor cortex with prototypical synaptic circuit connectivity. elife 3:e05422. doi: 10.7554/eLife.05422
Yang, G., Pan, F., and Gan, W. B. (2009). Stably maintained dendritic spines are associated with lifelong memories. Nature 462, 920–924. doi: 10.1038/nature08577
Yang, J., Serrano, P., Yin, X., Sun, X., Lin, Y., and Chen, S. X. (2022). Functionally distinct NPAS4-expressing somatostatin interneuron ensembles critical for motor skill learning. Neuron 110, 3339–3355.e8. doi: 10.1016/j.neuron.2022.08.018
Yoshida, Y., and Isa, T. (2018). Neural and genetic basis of dexterous hand movements. Curr. Opin. Neurobiol. 52, 25–32. doi: 10.1016/j.conb.2018.04.005
Zemmar, A., Weinmann, O., Kellner, Y., Yu, X., Vicente, R., Gullo, M., et al. (2014). Neutralization of Nogo-a enhances synaptic plasticity in the rodent motor cortex and improves motor learning in vivo. J. Neurosci. 34, 8685–8698. doi: 10.1523/JNEUROSCI.3817-13.2014
Ziemann, U., Lonnecker, S., Steinhoff, B. J., and Paulus, W. (1996a). The effect of lorazepam on the motor cortical excitability in man. Exp. Brain Res. 109, 127–135.
Ziemann, U., Rothwell, J. C., and Ridding, M. C. (1996b). Interaction between intracortical inhibition and facilitation in human motor cortex. J. Physiol. 496, 873–881. doi: 10.1113/jphysiol.1996.sp021734
Keywords: motor learning, cross-species, primary motor cortex, neuron, inhibitory interneuron, synapse, dendritic spine
Citation: Kogan E, Lu J and Zuo Y (2023) Cortical circuit dynamics underlying motor skill learning: from rodents to humans. Front. Mol. Neurosci. 16:1292685. doi: 10.3389/fnmol.2023.1292685
Received: 11 September 2023; Accepted: 11 October 2023;
Published: 26 October 2023.
Edited by:
Gaia Olivo, University of Gothenburg, SwedenReviewed by:
Gerardo Rojas-Piloni, Universidad Nacional Autónoma de México, MexicoCopyright © 2023 Kogan, Lu and Zuo. This is an open-access article distributed under the terms of the Creative Commons Attribution License (CC BY). The use, distribution or reproduction in other forums is permitted, provided the original author(s) and the copyright owner(s) are credited and that the original publication in this journal is cited, in accordance with accepted academic practice. No use, distribution or reproduction is permitted which does not comply with these terms.
*Correspondence: Ju Lu, amx1MzlAdWNzYy5lZHU=; Yi Zuo, eWl6dW9AdWNzYy5lZHU=
†These authors have contributed equally to this work
Disclaimer: All claims expressed in this article are solely those of the authors and do not necessarily represent those of their affiliated organizations, or those of the publisher, the editors and the reviewers. Any product that may be evaluated in this article or claim that may be made by its manufacturer is not guaranteed or endorsed by the publisher.
Research integrity at Frontiers
Learn more about the work of our research integrity team to safeguard the quality of each article we publish.