- 1Molecular Biology and Genetics Laboratory, Department of Biological Sciences, Birla Institute of Technology and Science, Pilani – Hyderabad Campus, Hyderabad, India
- 2Centralized Core Research Facility, All India Institute of Medical Sciences, New Delhi, India
- 3Department of Zoology, Thiagarajar College, Madurai, India
- 4Asha Hospital Institute of Medical Psychology, Hyderabad, India
- 5ESIC Medical College and Hospital, Hyderabad, India
- 6Department of Computer Engineering, National Institute of Technology, Kurukshetra, India
- 7Department of Psychiatry, M.S. Chellamuthu Trust and Research Foundation, Madurai, India
- 8Centre for Human Disease Research, Birla Institute of Technology and Science, Pilani – Hyderabad Campus, Hyderabad, India
Copy number variants (CNVs) are among the main genetic factors identified in schizophrenia (SZ) through genome-scale studies conducted mostly in Caucasian populations. However, to date, there have been no genome-scale CNV reports on patients from India. To address this shortcoming, we generated, for the first time, genome-scale CNV data for 168 SZ patients and 168 controls from South India. In total, 63 different CNVs were identified in 56 patients and 46 controls with a significantly higher proportion of medium-sized deletions (100 kb–1 Mb) after multiple testing (FDR = 2.7E-4) in patients. Of these, 13 CNVs were previously reported; however, when searched against GWAS, transcriptome, exome, and DNA methylation studies, another 17 CNVs with candidate genes were identified. Of the total 30 CNVs, 28 were present in 38 patients and 12 in 27 controls, indicating a significantly higher representation in the former (p = 1.87E-5). Only 4q35.1-q35.2 duplications were significant (p = 0.020) and observed in 11 controls and 2 patients. Among the others that are not significant, a few examples of patient-specific and previously reported CNVs include deletions of 11q14.1 (DLG2), 22q11.21, and 14q21.1 (LRFN5). 16p13.3 deletion (RBFOX1), 3p14.2 duplication (CADPS), and 7p11.2 duplication (CCT6A) were some of the novel CNVs containing candidate genes. However, these observations need to be replicated in a larger sample size. In conclusion, this report constitutes an important foundation for future CNV studies in a relatively unexplored population. In addition, the data indicate that there are advantages in using an integrated approach for better identification of candidate CNVs for SZ and other mental health disorders.
Introduction
Schizophrenia is a complex neuropsychiatric disorder with symptoms such as delusions, hallucinations, cognitive impairment, and lack of social interest. Although the exact cause is not established, both genetic and environmental factors are well recognized as playing a role in its occurrence (Wahbeh and Avramopoulos, 2021). In the case of genetic evidence, there is a 6-fold risk in families with an affected first-degree relative, whereas in monozygotic and dizygotic twins, the risk increases by 48- and 17-fold, respectively (Gottesman, 1994).
Multiple large-scale genome-wide association studies (GWAS) involving cases and controls identified three kinds of risk variants: single nucleotide polymorphisms (SNPs), copy number variants (CNVs), and de novo mutations (DNMs) (Kirov et al., 2012; Rees et al., 2014; Ripke et al., 2014; Marshall et al., 2017). Of these, CNVs involve segmental deletion or duplication of a DNA segment ranging in size from 1 kb to several Mb, resulting in a dosage imbalance of genes equivalent to haplo-insufficiency in cases that involve deletions and triplosenstivity in cases of duplications (Rees and Kirov, 2021).
To date, most genome-scale CNV studies have been conducted in Caucasian populations and a few in Asian populations but none have been undertaken for populations in India (Ikeda et al., 2010; Rees et al., 2014; Li et al., 2016; Kushima et al., 2017; Marshall et al., 2017). Since India is the most populated country in the world, this lack of genome-scale studies impedes understanding of the contribution of CNVs to SZ in this region. In this study, we first identified CNVs in 168 SZ patients and 168 age- and sex-matched controls from South India using PsychArrays. The CNVs identified in the sample set were searched against databases that contain previously reported CNVs. To detect unreported CNVs with candidate genes, GWAS, de novo mutation, methylation, and expression data from the SZDB V2.0 were used (Wu et al., 2017).
Materials and methods
Generation of genotyping data, quality control filtering, and relatedness testing
A total of 384 samples containing 194 schizophrenia cases (mean age ± SD: 36.4 ± 12.4; women = 48%) and 190 age-and sex-matched controls (mean age ± SD: 36.7 ± 10.2; women = 46%) of South Indian origin were identified based on the Diagnostic Statistic Manual-5 (DSM-V) criteria by an experienced psychiatrist. Written consent for peripheral blood sampling was obtained from all the participants or their legally authorized relatives. Genomic DNAs from the blood samples were genotyped using Illumina’s Infinum™ PsychArray v 1.3 (Illumina, San Diego, California, USA) at Sandor Life Sciences (Hyderabad, India). The intensity data obtained were used for sample and SNP-level quality control. GenomeStudio was used for data pre-processing using the clustering algorithm with a quality cutoff score of 0.15 (Bacchelli et al., 2020), and samples with low GenCall (GC) scores, having call rates below 0.98, and those of unknown sex were excluded from the analysis. These QC-passed data were subjected to further quality control using PLINK1.9 (Purcell et al., 2007), and SNPs with >5% missing call rates, those with a minor allele frequency of less than 1%, and those that failed the Hardy–Weinberg equilibrium (HWE; case value of p < 1 × 10−6, control value of p: <1 × 10−10) were removed from analyses (Rodríguez-López et al., 2018; Behera et al., 2023). Samples with 5% missing calls, sex discrepancy, and those deviating by three standard deviations from the heterozygosity rate were then excluded. Cryptic relatedness between individuals was detected based on pairwise identity-by-descent analysis, wherein any member of each pair of individuals with PIHAT >0.2 was removed (Marees et al., 2018). Using the remaining samples, MDS-based clustering was performed to study the population structure using PLINK1.9, and data were plotted using R-program to verify an appropriate overlap of the cases and controls and, to identify any outliers.
CNV calling
CNV calling was performed using the PennCNV algorithm to identify regions with copy number variations following standard pipelines that mainly focus on autosomes (Wang et al., 2007; Fang and Wang, 2018). First, the intensity files were used to generate PennCNV-compatible log R ratio (LRR) and B-allele frequency (BAF) input files for each sample. Then, a custom population B-allele frequency file (PFB) was generated using the “compile_pfb.pl” script followed by a GC model signal file specific for PsychArray using “cal_gc_snp.pl” to adjust the genomic waves and reduce false-positive CNV calls. CNVs were detected using the “detect_cnv.pl” script, and the adjacent calls were merged using “clean_cnv.pl” if two successive CNVs provided a gap of less than 20% of the total length. For CNV filtering based on the sample level, individuals with a standard deviation of log R ratio ≥ 0.3 were excluded. Furthermore, CNVs based on call-level filtration (≥10 kb with ≥30 consecutive probes) were identified using the “filter_cnv.pl” script (Vega-Sevey et al., 2020). The centromeric and telomeric regions were compiled from UCSC (hg19 assembly) and CNV calls overlapping with ≥50% of these chromosomal regions were removed by running the “scan_region.pl” script (Karolchik et al., 2004; Fang and Wang, 2018) using PennCNV. For annotating the identified CNVs, we used the hg19 reference gene annotation file from the PennCNV package using script “scan_region.pl.” The coordinates of the shortlisted CNVs were viewed in the UCSC browser to determine cytogenetic band information. CNVs were classified as non-recurrent or recurrent based on their occurrence in one or more individuals, respectively.
Statistical analyses
Significant differences between cases and controls were identified using the two-tailed Fisher’s exact test.1 Odds ratios were calculated using MedCalc Software Ltd. version 22.007.2 For multiple testing of the association of CNVs based on size, we used the Benjamini–Hochberg method of false discovery rate (FDR). Briefly, the p-values obtained by the Fisher’s test were ranked, and FDRs were calculated based on the formula: FDR = , where n = number of different CNV types tested (see Table 1; Thissen et al., 2002). Post-hoc statistical power was calculated using the frequencies of CNVs observed in patients and controls, the sample sizes used, and the type I error rate of 0.05 using the ClinCalc tool.3
Identification of CNVs with potential association with SZ
We first filtered CNVs as reported or unreported based on their presence and association with SZ in the SZDB2.0 database. Unreported CNVs were further queried against the genes identified by GWAS (11,260 patients and 24,542 controls), exome sequencing (14,598 patients and 11,515 controls), and DNA methylation analyses (191 patients and 335 controls) reported in the same database. Genome-wide transcriptome studies reported by the CommonMind and PsychEncode Consortia (817 patients and 1,115 controls) were used to identify a subset of the CNVs containing the candidate genes (Gandal et al., 2018; Hoffman et al., 2019). CNVs that were not present in the SZDB2.0 and that did not contain the candidate genes were considered to not be associated with SZ. Wherever possible, the frequencies of the observed CNVs in the present population were compared with those reported for other populations.
Calculation of penetrance
The number of controls and patients containing 22q11.2 deletions and the total number of samples tested were obtained from the SZDB 2.0. Using an incidence of 0.7%, penetrance and its critical intervals were calculated using CalPen software (Addepalli et al., 2020).
Results
Of the 194 patients and 190 controls used, 373 samples passed filtering for low GenCall (GC) scores, call rates <0.98, and unknown sex. A further 11 samples were excluded due to ambiguous sex calls. SNPs that did not conform to HWE or showed >5% missing call rates or MAF < 1.0% were removed from further analysis. This resulted in 273,175 SNPs with which heterozygosity analysis was used to eliminate one outlier, resulting in 361 samples. Cryptic relatedness testing using PIHAT further identified another 13 patients and 12 controls with scores ≥0.2 that were removed to finally obtain 336 analyzable samples (168 controls and 168 patients). MDS clustering to study population stratification confirmed an appropriate overlap of the controls and patients with no outliers and were suitable for CNV detection (Figure 1A).
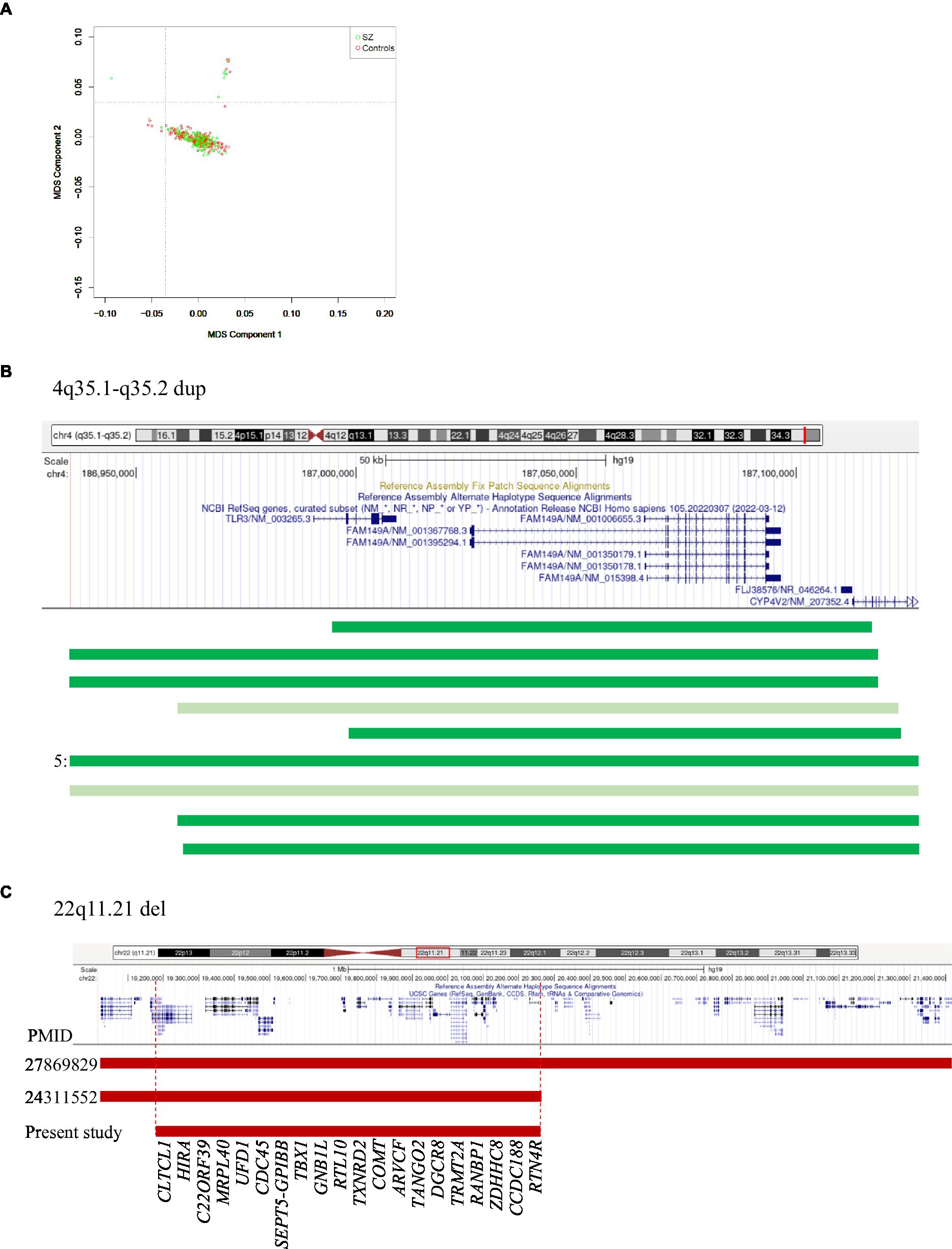
Figure 1. (A) MDS plot of controls and patients. (B) 4q35.1-q35.2 region showing duplications in 11 controls (dark green) and 2 patients (light green). 5 indicates that five controls had identical duplications. (C) 22q11.21 region showing reported and observed deletions. Vertical dotted lines indicate the minimal region. The numbers on the left indicate PMIDs corresponding to Marshall et al. (2017; 27869829) and Rees et al. (2014; 24311552).
All the 336 samples had standard deviations of log R ratios <0.3 and gave an initial list of 3,110 CNVs which when filtered based on size (≥10 kb), the presence of ≥30 consecutive probes gave 122 variants, of which 106 were genic. Four of them were further removed because of their overlaps with centromeric and telomeric regions. Of the remaining 102 CNVs, 39 were represented more than once, leaving 63 unique CNVs. The size distribution of these 63 CNVs is summarized in Table 1. The medium-sized CNVs (0.1–1.0 Mb) were higher in patients than in controls (p = 0.1975), but the difference was significant in case of deletions (20 vs. 8; p = 4.5E-5; FDR = 2.7E-4). Of the 63 different CNVs, 45 were present in patients and 30 in controls, including 12 that were shared between the two groups (Supplementary Table 1). These data suggest ~1.5-fold representation in patients (p = 0.0107) over controls.
In total, 13 of the 63 different CNVs observed in 17 patients and 7 controls were previously reported (Table 2). Of these, seven were present only in patients and two in only controls, whereas the remaining four were shared. When the remaining 50 unreported CNVs were tested for the presence of candidate genes (mentioned in the section Materials and Methods), an additional 17 were identified (Table 2). Of these, 11 CNVs were present exclusively in patients, and the remaining 6 were shared with controls. In total, of the 30 different CNVs (17 unreported with candidate genes and 13 reported), 28 were present in patients and 12 in controls, indicating a significant difference in their representation (p = 1.87E-5). This difference was mainly because of a higher number of medium-sized deletions in patients as mentioned above.
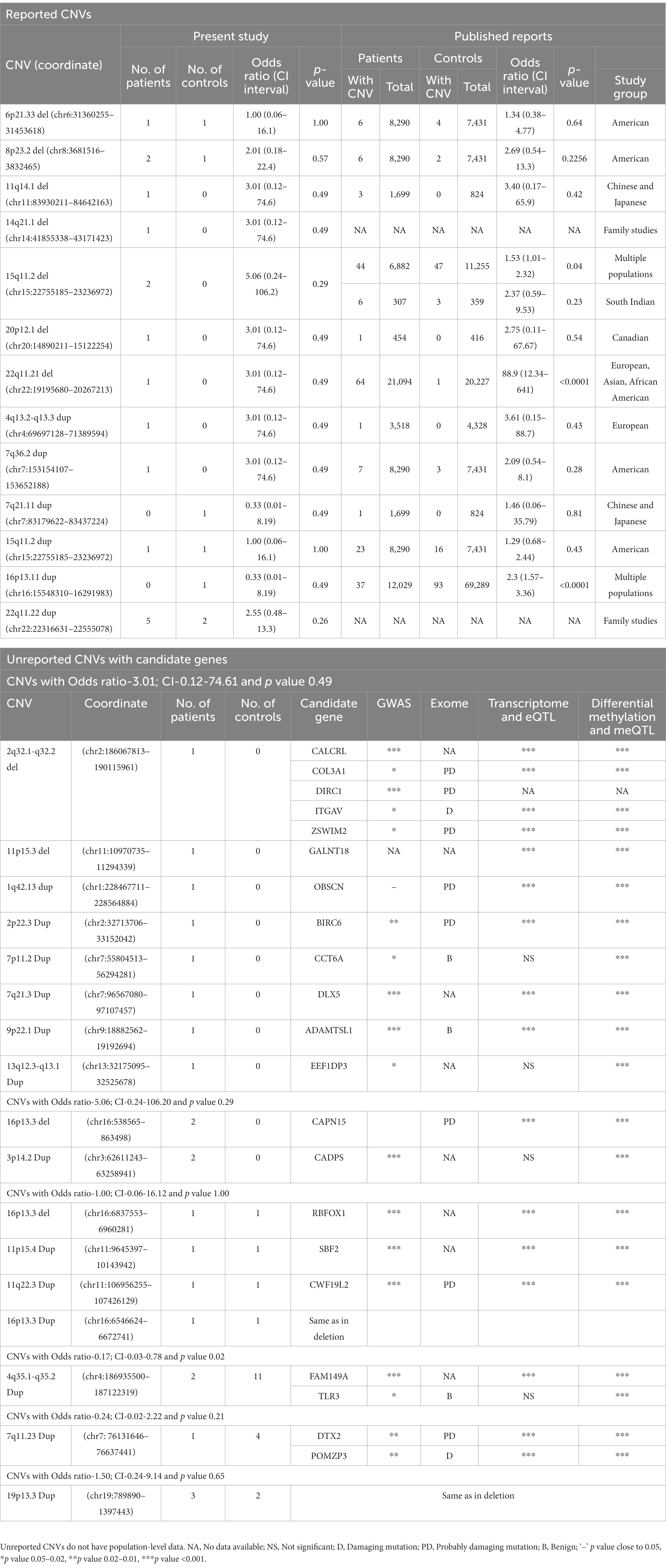
Table 2. List of reported and unreported CNVs with candidate genes identified in the patients and controls in the present study and their frequencies in other populations.
4q35.1-q35.2 duplication
This was the most common CNV in our dataset, measured ~0.19 Mb containing CYP4V2, FAM149A, FLJ38576, and TLR3 genes, and occurred at a significantly higher number in controls than patients (11 versus 2, respectively; p = 0.02). Two of the controls had partial duplications of TLR3 (Figure 1B), a member of the highly conserved toll-like receptors that play a role in innate immunity. Experimental data suggest that TLR3 negatively controls the expression of DISC1, resulting in impaired dendritic arborization. CNVs of this region have not been reported, but there was a nominal association of an intronic variant (rs3775294) in SZ (p = 0.046) (Pardiñas et al., 2018). Both TLR3 and FAM149A were implicated in GWAS, expression, and methylation studies on SZ patients (Wu et al., 2017).
None of the other CNVs have a significant difference in their occurrence between the patients and controls studied. A few that have been previously reported and the unreported ones with candidate genes are mentioned here: (i) Duplications of 22q11.22 (~0.24 Mb) involving PRAMENP and the first 13 exons of TOP3β were observed in five patients and two controls. This was the second most common CNV in this dataset. (ii) 15q11.2 deletions were found in two patients but not in controls, whereas duplications were present in one patient and one control. When combined with the data from Saxena et al. (2019), both deletions and duplications were not significantly different in a total of 540 controls and 499 patients. CNVs of this region were considered as variants of uncertain significance (VUS; Mohan et al., 2019). (iii) 11q14.1 deletion (~0.71 Mb) involving DLG2 was found in one patient. Deletions of this region were reported in autism spectrum disorders (Griesius et al., 2022) and SZ patients in Caucasian and Asian populations (Walsh et al., 2008; Kushima et al., 2017). (iv) 14q21.1 deletion (~1.3 Mb) involving LRFN5 was found in one patient and was previously reported in SZ, developmental delay, intellectual disability, and microcephaly (Xu et al., 2009; Lybaek et al., 2022). The observed deletion was larger, involving the entire gene as well as the 60 kb region implicated in its regulation with conformation differences between maternally and paternally transmitted chromosomes (Mikhail et al., 2011). (v) 22q11.21 deletion (~1.1 Mb) was observed in one patient and is smaller than the previously reported ones (~2.35 Mb and ~ 1.24 Mb; Rees et al., 2014; Marshall et al., 2017). The observed deletion did not include DGCR2, ESS2, TSSK2, GSC2, SLC25A1, and CLTCL1 (Figure 1C). Deletions of this region are considered to have higher penetrance for SZ (Addepalli et al., 2020). For example, the data given in Table 2 gave a penetrance value of 0.272 with critical intervals of 0.079 to 0.818. (vi) 3p14.2 duplations of ~0.65 Mb affecting the copy number of CADPS were observed in two patients. Duplications of this gene are not reported in the literature, but duplication of CADPS2, a member of the same gene family, was reported in autism spectrum disorders (Girirajan et al., 2013).
Statistical power considerations
As mentioned above, of the total of 30 CNVs identified, 28 were present in 38 patients (22.6%), whereas 12 were present in 27 controls (16.1%). These incidences, along with a Type I error rate of 0.05, the data on 168 patients and 168 controls yielded a post-hoc statistical power of 32.5%.
Discussion
The present study is the first to describe. CNVs in Indian SZ patients and controls, and compare the data with other ethnic groups. The data were in overall agreement with the increased CNV burden observed in SZ in other populations (Marshall et al., 2017). Specifically, there was a significant enrichment of medium-sized deletions that have been previously reported or those containing SZ-associated genes in the patients studied here. These data also suggest the utility of PsychArrays in cost-effectively validating previous findings and identifying novel CNVs in patients from India and such unexplored ethnic groups. It may be noted that this report on CNVs used 168 patients and 168 controls and thus had a relatively small sample size. In this context, 4q35.1-q35.2 duplication, which was the only CNV with a significantly higher occurrence in controls, requires replication on a larger sample. However, such sample sizes were also used in other similar initial studies (e.g., Vega-Sevey et al., 2020; Abumadini et al., 2023).
In this initial search, only 13 out of the 63 different identified CNVs were previously reported in SZ. However, we reasoned that more relevant CNVs could be found if genes identified through GWAS, methylation, exome, and transcriptome studies were also considered. This approach draws support from a previous study on German and Chinese patients, wherein the possibility of the occurrence of CNVs was successfully tested based on GABRB2 variants reported in other studies (Ullah et al., 2020). In agreement with these expectations, 17 additional CNVs were identified in our dataset. Thus, an integrated approach of a similar kind is worthwhile implementing for identifying unreported CNVs that are of potential interest from publicly available data and for confirmation through replication studies for SZ and other mental health disorders. As mentioned in the ‘Results’ section, a few CNVs, such as 3p14.2 duplication, occurred only in patients but not in controls. This occurrence is at present not significant, but replication studies are needed to confirm any association between the reported and unreported CNVs described here.
A general observation among case–control studies on other populations suggests that the frequencies of many of the CNVs involved are less than 1% (Rees et al., 2014; Marshall et al., 2017). For instance, 15q11.2 del, which is the most common CNV in SZDB2.0, has a frequency of 0.46%, whereas 16p11.2 duplications that were highest in the patients studied by Marshall et al. (2017), showed a frequency of 0.33%. It is noteworthy that a majority of the SZ-associated CNVs that were identified in this study and listed in SZDB2.0 also do not have significant p-values except for 15q11.2 del, 22q11.21 del, and 16p13.11 dup (p = 0.04, <0.0001 and < 0.0001, respectively). Thus, the data suggest that, in general, CNVs with candidate genes are expected to be less frequent. In this context, case–control studies with access to samples from family members (affected as well as unaffected) will prove valuable in determining the contribution of these CNVs to SZ.
Data availability statement
The data presented in the study are deposited in the NCBI GEO repository with accession number GSE242813.
Ethics statement
The studies involving humans were approved by Institutional Human Ethics Committees of Birla Institute of Technology and Science, Pilani. Hyderabad, and all collaborating institutions. The studies were conducted in accordance with the local legislation and institutional requirements. The participants provided their written informed consent to participate in this study.
Author contributions
MS: Writing – review & editing, Data curation, Formal analysis, Investigation, Methodology, Software, Validation, Writing – original draft. DP: Writing – review & editing, Data curation, Methodology, Formal analysis, Investigation, Software. PK: Investigation, Writing – review & editing. GP: Investigation, Writing – review & editing. ND: Investigation, Writing – review & editing. LK: Investigation, Writing – review & editing, Software. CR: Investigation, Writing – review & editing. SK: Investigation, Writing – review & editing. SS: Investigation, Writing – review & editing. KM: Investigation, Writing – review & editing, Conceptualization, Funding acquisition, Project administration, Resources, Supervision, Methodology.
Funding
The author(s) declare financial support was received for the research, authorship, and/or publication of this article. This study was partially supported by grants from Department of Science and Technology (DST-India), Indian Council for Medical Research (ICMR-India), and Centre for Human Disease Research (CHDR) BITS-Pilaniand BITS Pilani Hyderabad Campus. MS was supported by fellowships from BITS Pilani and Indian Council of Medical Research.
Acknowledgments
The authors acknowledge all the study participants and Shivkumar Sharma Irukuvajjula for extending his support in the analysis.
Conflict of interest
The authors declare that the research was conducted in the absence of any commercial or financial relationships that could be construed as a potential conflict of interest.
Publisher’s note
All claims expressed in this article are solely those of the authors and do not necessarily represent those of their affiliated organizations, or those of the publisher, the editors and the reviewers. Any product that may be evaluated in this article, or claim that may be made by its manufacturer, is not guaranteed or endorsed by the publisher.
Supplementary material
The Supplementary material for this article can be found online at: https://www.frontiersin.org/articles/10.3389/fnmol.2023.1268827/full#supplementary-material
Footnotes
References
Abumadini, M. S., Al Ghamdi, K. S., Alqahtani, A. H., Almedallah, D. K., Callans, L., Jarad, J. A., et al. (2023). Genome-wide copy number variant screening of Saudi schizophrenia patients reveals larger deletions in cases versus controls. Front. Mol. Neurosci. 16:1069375. doi: 10.3389/fnmol.2023.1069375
Addepalli, A., Kalyani, S., Singh, M., Bandyopadhyay, D., and Mohan, K. N. (2020). CalPen (calculator of penetrance), a web-based tool to estimate penetrance in complex genetic disorders. PLoS One 15:e0228156. doi: 10.1371/journal.pone.0228156
Bacchelli, E., Cameli, C., Viggiano, M., Igliozzi, R., Mancini, A., Tancredi, R., et al. (2020). An integrated analysis of rare CNV and exome variation in autism Spectrum disorder using the Infinium PsychArray. Sci. Rep. 10:3198. doi: 10.1038/s41598-020-59922-3
Behera, C., Kaushik, R., Bharti, D. R., Nayak, B., Bhardwaj, D. N., Pradhan, D., et al. (2023). PsychArray-based genome wide association study of suicidal deaths in India. Brain Sci. 13:136. doi: 10.3390/brainsci13010136
Fang, L., and Wang, K. (2018). Identification of copy number variants from SNP arrays using PennCNV. Methods Mol. Biol. 1833, 1–28. doi: 10.1007/978-1-4939-8666-8_1
Gandal, M. J., Zhang, P., Hadjimichael, E., Walker, R. L., Chen, C., Liu, S., et al. (2018). Transcriptome-wide isoform-level dysregulation in ASD, schizophrenia, and bipolar disorder. Science 362:eaat8127. doi: 10.1126/science.aat8127
Girirajan, S., Dennis, M. Y., Baker, C., Malig, M., Coe, B. P., Campbell, C. D., et al. (2013). Refinement and discovery of new hotspots of copy-number variation associated with autism spectrum disorder. Am. J. Hum. Genet. 92, 221–237. doi: 10.1016/j.ajhg.2012.12.016
Gottesman, I. I. (1994). Complications to the complex inheritance of schizophrenia. Clin. Genet. 46, 116–123. doi: 10.1111/j.1399-0004.1994.tb04213.x
Griesius, S., O’Donnell, C., Waldron, S., Thomas, K. L., Dwyer, D. M., Wilkinson, L. S., et al. (2022). Reduced expression of the psychiatric risk gene DLG2 (PSD93) impairs hippocampal synaptic integration and plasticity. Neuropsychopharmacology 47, 1367–1378. doi: 10.1038/s41386-022-01277-6
Hoffman, G. E., Bendl, J., Voloudakis, G., Montgomery, K. S., Sloofman, L., Wang, Y.-C., et al. (2019). CommonMind consortium provides transcriptomic and epigenomic data for schizophrenia and bipolar disorder. Sci. Data 6:180. doi: 10.1038/s41597-019-0183-6
Ikeda, M., Aleksic, B., Kirov, G., Kinoshita, Y., Yamanouchi, Y., Kitajima, T., et al. (2010). Copy number variation in schizophrenia in the Japanese population. Biol. Psychiatry 67, 283–286. doi: 10.1016/j.biopsych.2009.08.034
Karolchik, D., Hinrichs, A. S., Furey, T. S., Roskin, K. M., Sugnet, C. W., Haussler, D., et al. (2004). The UCSC table browser data retrieval tool. Nucleic Acids Res. 32, 493D–4496D. doi: 10.1093/nar/gkh103
Kirov, G., Pocklington, A. J., Holmans, P., Ivanov, D., Ikeda, M., Ruderfer, D., et al. (2012). De novo CNV analysis implicates specific abnormalities of postsynaptic signalling complexes in the pathogenesis of schizophrenia. Mol. Psychiatry 17, 142–153. doi: 10.1038/mp.2011.154
Kushima, I., Aleksic, B., Nakatochi, M., Shimamura, T., Shiino, T., Yoshimi, A., et al. (2017). High-resolution copy number variation analysis of schizophrenia in Japan. Mol. Psychiatry 22, 430–440. doi: 10.1038/mp.2016.88
Li, Z., Chen, J., Xu, Y., Yi, Q., Ji, W., Wang, P., et al. (2016). Genome-wide analysis of the role of copy number variation in schizophrenia risk in Chinese. Biol. Psychiatry 80, 331–337. doi: 10.1016/j.biopsych.2015.11.012
Lybaek, H., Robson, M., de Leeuw, N., Hehir-Kwa, J. Y., Jeffries, A., Haukanes, B. I., et al. (2022). LRFN5 locus structure is associated with autism and influenced by the sex of the individual and locus conversions. Autism Res. 15, 421–433. doi: 10.1002/aur.2677
Marees, A. T., de Kluiver, H., Stringer, S., Vorspan, F., Curis, E., Marie-Claire, C., et al. (2018). A tutorial on conducting genome-wide association studies: quality control and statistical analysis. Int. J. Methods Psychiatr. Res. 27:e1608. doi: 10.1002/mpr.1608
Marshall, C. R., Howrigan, D. P., Merico, D., Thiruvahindrapuram, B., Wu, W., Greer, D. S., et al. (2017). Contribution of copy number variants to schizophrenia from a genome-wide study of 41,321 subjects. Nat. Genet. 49, 27–35. doi: 10.1038/ng.3725
Mikhail, F. M., Lose, E. J., Robin, N. H., Descartes, M. D., Rutledge, K. D., Rutledge, S. L., et al. (2011). Clinically relevant single gene or intragenic deletions encompassing critical neurodevelopmental genes in patients with developmental delay, mental retardation, and/or autism spectrum disorders. Am. J. Med. Genet. A 155, 2386–2396. doi: 10.1002/ajmg.a.34177
Mohan, K. N., Cao, Y., Pham, J., Cheung, S. W., Hoffner, L., Ou, Z. Z., et al. (2019). Phenotypic association of 15q11.2 CNVs of the region of breakpoints 1-2 (BP1-BP2) in a large cohort of samples referred for genetic diagnosis. J. Hum. Genet. 64, 253–255. doi: 10.1038/s10038-018-0543-7
Pardiñas, A. F., Holmans, P., Pocklington, A. J., Escott-Price, V., Ripke, S., Carrera, N., et al. (2018). Common schizophrenia alleles are enriched in mutation-intolerant genes and in regions under strong background selection. Nat. Genet. 50, 381–389. doi: 10.1038/s41588-018-0059-2
Purcell, S., Neale, B., Todd-Brown, K., Thomas, L., Ferreira, M. A. R., Bender, D., et al. (2007). PLINK: a tool set for whole-genome association and population-based linkage analyses. Am. J. Hum. Genet. 81, 559–575. doi: 10.1086/519795
Rees, E., and Kirov, G. (2021). Copy number variation and neuropsychiatric illness. Curr. Opin. Genet. Dev. 68, 57–63. doi: 10.1016/j.gde.2021.02.014
Rees, E., Walters, J. T. R., Georgieva, L., Isles, A. R., Chambert, K. D., Richards, A. L., et al. (2014). Analysis of copy number variations at 15 schizophrenia-associated loci. Br. J. Psychiatry 204, 108–114. doi: 10.1192/bjp.bp.113.131052
Ripke, S., Neale, B. M., Corvin, A., Walters, J. T. R., Farh, K.-H., Holmans, P. A., et al. (2014). Biological insights from 108 schizophrenia-associated genetic loci. Nature 511, 421–427. doi: 10.1038/nature13595
Rodríguez-López, J., Flórez, G., Blanco, V., Pereiro, C., Fernández, J. M., Fariñas, E., et al. (2018). Genome wide analysis of rare copy number variations in alcohol abuse or dependence. J. Psychiatr. Res. 103, 212–218. doi: 10.1016/j.jpsychires.2018.06.001
Saxena, S., Kkani, P., Ramasubramanian, C., Kumar, S. G., Monisha, R., Prasad Rao, G., et al. (2019). Analysis of 15q11.2 CNVs in an Indian population with schizophrenia. Ann. Hum. Genet. 83, 187–191. doi: 10.1111/ahg.12300
Thissen, D., Steinberg, L., and Kuang, D. (2002). Quick and easy implementation of the Benjamini-Hochberg procedure for controlling the false positive rate in multiple comparisons. J. Educ. Behav. Stat. 27, 77–83. doi: 10.3102/10769986027001077
Ullah, A., Long, X., Mat, W.-K., Hu, T., Khan, M. I., Hui, L., et al. (2020). Highly recurrent copy number variations in GABRB2 associated with schizophrenia and premenstrual dysphoric disorder. Front. Psych. 11:572. doi: 10.3389/fpsyt.2020.00572
Vega-Sevey, J. G., Martínez-Magaña, J. J., Genis-Mendoza, A. D., Escamilla, M., Lanzagorta, N., Tovilla-Zarate, C. A., et al. (2020). Copy number variants in siblings of Mexican origin concordant for schizophrenia or bipolar disorder. Psychiatry Res. 291:113018. doi: 10.1016/j.psychres.2020.113018
Wahbeh, M. H., and Avramopoulos, D. (2021). Gene-environment interactions in schizophrenia: a literature review. Genes 12:1850. doi: 10.3390/genes12121850
Walsh, T., McClellan, J. M., McCarthy, S. E., Addington, A. M., Pierce, S. B., Cooper, G. M., et al. (2008). Rare structural variants disrupt multiple genes in neurodevelopmental pathways in schizophrenia. Science 320, 539–543. doi: 10.1126/science.1155174
Wang, K., Li, M., Hadley, D., Liu, R., Glessner, J., Grant, S. F. A., et al. (2007). PennCNV: an integrated hidden Markov model designed for high-resolution copy number variation detection in whole-genome SNP genotyping data. Genome Res. 17, 1665–1674. doi: 10.1101/gr.6861907
Wu, Y., Yao, Y.-G., and Luo, X.-J. (2017). SZDB: a database for schizophrenia genetic research. Schizophr. Bull. 43, 459–471. doi: 10.1093/schbul/sbw102
Xu, B., Woodroffe, A., Rodriguez-Murillo, L., Roos, J. L., van Rensburg, E. J., Abecasis, G. R., et al. (2009). Elucidating the genetic architecture of familial schizophrenia using rare copy number variant and linkage scans. Proc. Natl. Acad. Sci. U. S. A. 106, 16746–16751. doi: 10.1073/pnas.0908584106
Keywords: schizophrenia, India, CNVs, case–control studies, deletions, duplications, PsychArray
Citation: Singh M, Pradhan D, Kkani P, Prasad Rao G, Dhagudu NK, Kumar L, Ramasubramanian C, Kumar SG, Sonttineni S and Mohan KN (2023) Genome-scale copy number variant analysis in schizophrenia patients and controls from South India. Front. Mol. Neurosci. 16:1268827. doi: 10.3389/fnmol.2023.1268827
Edited by:
Peristera Paschou, Purdue University, United StatesReviewed by:
Bruce J. Kinon, Karuna Therapeutics, Inc., United StatesHong Xue, Hong Kong University of Science and Technology, Hong Kong SAR, China
Copyright © 2023 Singh, Pradhan, Kkani, Prasad Rao, Dhagudu, Kumar, Ramasubramanian, Kumar, Sonttineni and Mohan. This is an open-access article distributed under the terms of the Creative Commons Attribution License (CC BY). The use, distribution or reproduction in other forums is permitted, provided the original author(s) and the copyright owner(s) are credited and that the original publication in this journal is cited, in accordance with accepted academic practice. No use, distribution or reproduction is permitted which does not comply with these terms.
*Correspondence: Kommu Naga Mohan, a29tbXVtb2hhbkBnbWFpbC5jb20=; Minali Singh, bWluYWxpc2luZ2g3OEBnbWFpbC5jb20=