- 1Laboratory of Medical Genetics, University Medical Centre Maribor, Maribor, Slovenia
- 2Department of Molecular Biology, Faculty of Medicine, University of Maribor, Maribor, Slovenia
- 3Centre for Human Molecular Genetics, and Pharmacogenomics, Faculty of Medicine, University of Maribor, Maribor, Slovenia
- 4Department of Animal Science, Biotechnical Faculty, University of Ljubljana, Ljubljana, Slovenia
- 5Department of Pediatrics, University Medical Centre Maribor, Maribor, Slovenia
Autism spectrum disorders (ASD) represent a phenotypically heterogeneous group of patients that strongly intertwine with other neurodevelopmental disorders (NDDs), with genetics playing a significant role in their etiology. Whole exome sequencing (WES) has become predominant in molecular diagnostics for ASD by considerably increasing the diagnostic yield. However, the proportion of undiagnosed patients still remains high due to complex clinical presentation, reduced penetrance, and lack of segregation analysis or clinical information. Thus, reverse phenotyping, where we first identified a possible genetic cause and then determine its clinical relevance, has been shown to be a more efficient approach. WES was performed on 147 Slovenian pediatric patients with suspected ASD. Data analysis was focused on identifying ultrarare or “single event” variants in ASD-associated genes and further expanded to NDD-associated genes. Protein function and gene prioritization were performed on detected clinically relevant variants to determine their role in ASD etiology and phenotype. Reverse phenotyping revealed a pathogenic or likely pathogenic variant in ASD-associated genes in 20.4% of patients, with subsequent segregation analysis indicating that 14 were de novo variants and 1 was presumed compound heterozygous. The diagnostic yield was further increased by 2.7% by the analysis of ultrarare or “single event” variants in all NDD-associated genes. Protein function analysis established that genes in which variants of unknown significance (VUS) were detected were predominantly the cause of intellectual disability (ID), and in most cases, features of ASD as well. Using such an approach, variants in rarely described ASD-associated genes, such as SIN3B, NR4A2, and GRIA1, were detected. By expanding the analysis to include functionally similar NDD genes, variants in KCNK9, GNE, and other genes were identified. These would probably have been missed by classic genotype–phenotype analysis. Our study thus demonstrates that in patients with ASD, analysis of ultrarare or “single event” variants obtained using WES with the inclusion of functionally similar genes and reverse phenotyping obtained a higher diagnostic yield despite limited clinical data. The present study also demonstrates that most of the causative genes in our cohort were involved in the syndromic form of ASD and confirms their comorbidity with other developmental disorders.
Introduction
Autism spectrum disorders (ASD) combine phenotypically heterogeneous groups that often co-occur with other neurodevelopmental disorders (NDDs) (Arteche-López et al., 2021). Clinically, two main features must be met for the diagnosis of ASD; the patient must present with difficulties in each of the three social communication subdomains and two of the four different restricted, repetitive sensory–motor behaviors in the past or present for both features (Lord et al., 2018). Variations in phenotype and their severity indicate that genetics and environmental factors are key players in the etiology of ASD (Rylaarsdam and Guemez-Gamboa, 2019). The size of genetic variants and mutation types vary from large chromosomal aberrations to single nucleotide variants (SNVs). They may also be rare or common risk variants (Balicza et al., 2019). Current guidelines for the detection of copy number variants (CNVs) with chromosomal microarray (CMA) in patients with NDDs, including ASD, as first-tier tests are still valid, although they were published over a decade ago (Miller et al., 2010).
Implementing next-generation sequencing (NGS) methods in the molecular diagnosis of NDDs has considerably increased the diagnostic yield (Lindstrand et al., 2019; Arteche-López et al., 2021). Whole exome sequencing (WES) enables the detection of rare or common small deletions, duplications, indels, synonymous, nonsense, splice, 3′-UTR, and missense or frameshift variants in the coding regions of the genome. The use of WES analysis in conjunction with CNV analysis enables a molecular diagnosis to be made in about 30% of patients with ASD and intellectual disability (ID) (Valentino et al., 2021). NGS methods have also enabled us to pinpoint genes implicated in ASD phenotypes (Bernier et al., 2014; Satterstrom et al., 2020). Trio-based WES analysis has proven to be successful in identifying rare inherited and de novo variants in genes involved in the etiology of ASD and novel ASD-associated genes (Satterstrom et al., 2020; Lintas et al., 2021). However, the inability to determine the origin of the suspected variant, reduced penetrance, broad clinical characterization of patients with ASD, or lack of clinical information aggravates the process of making proper genotype–phenotype correlations. Therefore, in these cases, it makes sense to reverse the diagnostic process by looking for ultrarare variants in the ASD-associated genes in the same group of patients and only then defining their clinical significance. This approach reduces the probability of an inaccurate analysis due to a highly heterogeneous phenotype or limited descriptions of patients by using simple algorithms. Following this approach, variants in rarely described ASD-associated genes, such as the SIN3B, NR4A2, and GRIA1, were detected, and a broad clinical phenotype combining ID and neurodevelopmental abnormalities, including ASD, was described in these patients (Geisheker et al., 2017; Lévy et al., 2018; Latypova et al., 2021). More importantly, the diagnostic yield was further increased using this type of analysis for potential causative variants in genes involved in other NDDs (the KCNK9 and GNE genes). These genes were not linked to ASD according to ASD-associated genes listed in the Simons Foundation Autism Research Initiative (SFARI) database1 (Graham, Zadeh et al., 2016; Yang et al., 2020). Variants of unknown significance (VUS) also represent a challenging category for clinical interpretation. Therefore, for genes with VUS, variant protein function predictions and gene prioritizations were performed to identify causative genes outside of the ASD-associated pool of genes.
The present study further endorses the comorbidity of ASD and NDDs, indicating that genetic analysis should not be limited only to the wide range of ASD-associated genes. We also demonstrated how phenotype-driven analysis is not always the optimal approach, especially in cases with limited clinical data. The present study also demonstrates how the usage of the obtained genetic data in combination with simple step algorithms and bioinformatics could increase the yield of molecular diagnostics for patients with ASD.
Materials and Methods
Patient Enrollment
Patients with ASD from a large cohort of 439 Slovenian children with NDD were included (Krgovic et al., 2018). In this study, patients with ASD and their parents were re-invited to participate in the WES study. Written informed consent from their parents or legal guardians was obtained for all probands included in our cohort. This study is part of a larger study that was approved by the Commission of the Republic of Slovenia for Medical Ethics (KME No. 89/01/11). All experimental procedures were performed according to guidelines and regulations and abided by the tenets of the Declaration of Helsinki.
Patients
A WES analysis was carried out on DNA samples from 147 pediatric patients with a suspected diagnosis of ASD. The patient group consisted of 110 boys (74.8%) and 37 girls (25.2%) with a mean age of 8.2 ± 0.935 years (±SD) at molecular diagnostic referral. The main referral diagnosis was suspected ASD, usually with coexistent ID, development delay (DD), language impairment, attention deficit hyperactivity disorder (ADHD), and other disorders. The test group included 10 pairs of siblings, of which 2 sets were twins, and 2 families had more than 2 affected children.
Whole Exome Sequencing
Whole exome sequencing was performed on patients and, in some cases, their parents using DNA extracted from peripheral blood leukocytes with the QIAamp® DNA Blood Midi Kit (QIAGEN, Hilden, Germany). WES and library construction were performed by Novogene (Novogene Company Limited, Cambridge, United Kingdom) using the Agilent SureSelect Human All Exon Kit (Agilent Technologies, Santa Clara, CA, United States). The DNA libraries were then sequenced on an Illumina HiSeq 4000 platform (150-bp paired-end reads) (Illumina, San Diego, CA, United States) with an average depth coverage of 100× with an average 12 Gb output per sample.
Analysis Algorithm
Raw fastq reads were first assessed for quality control using FastQC v0.11.9 software (Wingett and Andrews, 2018). The Trimmomatic tool v0.39 was used to trim technical sequences and adapters from raw sequences (Bolger et al., 2014). The Burrows-Wheeler Aligner BWA v0.7.12-r1039 with the MEM algorithm was used for mapping the raw reads to the human reference genome (GRCh37/hg19) (Li and Durbin, 2010). PCR duplicates’ marking and mate information fixation were performed using PicardTools v2.20.1. Base quality scores were additionally recalibrated using the genome analysis toolkit (GATK) v4.0 (McKenna et al., 2010; DePristo et al., 2011; Van der Auwera et al., 2013). Variant calling was performed using the HaplotypeCaller algorithm in genomic mode with joint calling (Poplin et al., 2018). Variant quality score recalibration using a GATK-implemented adaptive error model, a machine learning method with known genomic sites, was also used to establish a method for enabling variant filtering based on the fine balance between sensitivity and specificity for true or false genetic variants. Normalization with left trimming was also performed using GATK to obtain the final unfiltered VCF files. Variant annotation was performed using the VarAFT tool v2.17-2 (Desvignes et al., 2018). Machine learning algorithms using conservation profiles, such as SIFT and PolyPhen-2 conservation profiles, were used for the prediction of consequences of missense mutations (Kumar et al., 2009; Adzhubei et al., 2010, 2013). Subsequently, stepwise variant filtering was performed for variants with a read-depth coverage of >10× and an altered variant frequency of at least 30%. Variants with a frequency of more than 1% in the population variant databases [1,000 genome projects, Exome Aggregation Consortium (ExAC), and Exome Variant Server (EVS)] were excluded. Intergenic or 3′-UTR/5′-UTR untranslated region variants and non-splice–related intronic and synonymous variants were also filtered out, except for those located at canonical splice sites (Figure 1).
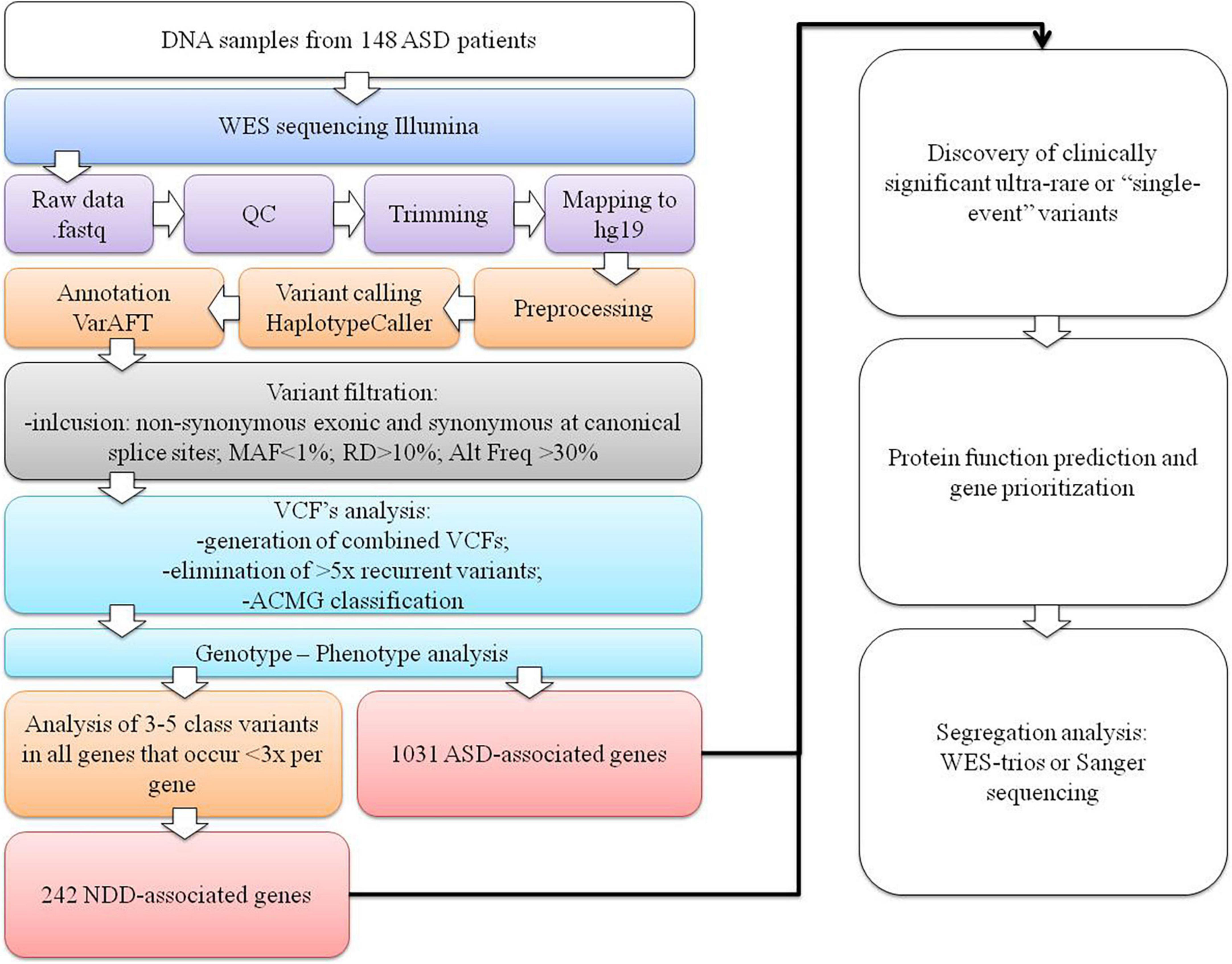
Figure 1. The WES data variant analysis step-by-step workflow used in our cohort. The first section represents the NGS pipeline for generating VCF files. In the second section, variant filtering, identifying ultrarare or “single event” variants process, and data interpretation are presented.
Variant Filtering and Classification
Filtered VCF files for all 147 patients were combined, generating a catalog of 39,668 variants (on average, 268 variants per patient), which were sorted by their canonical transcript and genomic position. All the recurrent variants that were present more than five times were eliminated as they were presumed to be sequence artifacts. We then obtained only ultrarare variants or “single event” variants that occurred only once per gene. Variants were then classified based on standards and guidelines of the American College of Medical Genetics and Genomics (ACMG) as pathogenic (class 5), likely pathogenic (class 4), variants of uncertain clinical significance (VUS) (class 3), likely benign (class 2), and benign (class 1) using the Franklin2 tool (Richards et al., 2015). For all variants classified as possible causative (classes 3–5) in 1,031 genes listed in the SFARI database (SFARI-Gene_genes_01-11-2022release_03-23-2022export) (Supplementary Table 1), genotype–phenotype analysis was performed based on the clinical description of patients and a literature search. If parents’ samples were available, segregation analysis with WES-trio for VUS or Sanger sequencing for likely pathogenic or pathogenic variants was carried out.
The analysis was further expanded for all class 3–5 variants in all genes, with the limitation that no more than three variants emerged per gene. This facilitated detection of the rare or “single event” variants in the gene that was more likely to be causal and reduced the scope of the analysis. For these genes, a subsequent extensive literature search was performed to determine whether the gene could be associated with NDDs, resulting in the inclusion of an additional 242 NDD-associated genes (Supplementary Table 1) in the genotype–phenotype analysis. The diagram of our analysis is presented in Figure 1.
Segregation Analysis
Segregation of the potential variant was performed in 32 trio-based WES analyses, 10 family WES analyses (siblings and parents), and Sanger sequencing in 14 families when pathogenic/likely pathogenic variants in ASD-associated genes were detected. All variants detected by WES analysis were then confirmed by Sanger sequencing using the Beckman Coulter CEQ 8000 Genetic Analysis System (Beckman Coulter Inc., Indianapolis, IN, United States).
Protein Function Predictions and Gene Prioritizations
The STRING3 tool was used for generating functionally similar genes based on genomic and proteomic data for the detected pathogenic, likely pathogenic, and VUS variants (Table 2; Szklarczyk et al., 2019). The genes were clustered with k-means clustering into four groups to identify possible correlations in the same biological pathways and/or involvement in specific phenotypes (diseases).
The same set of genes was also used in enrichment and Gene Ontology (GO) analysis, employing the g:Profiler4 tool to determine which Human Phenotype Ontology (HP) terms are most frequently linked with the analyzed genes, particularly for genes with VUS variants that are not listed in the SFARI database (Raudvere et al., 2019).
Results
Whole Exome Sequencing and Segregation Analysis
With WES analysis focusing on ultrarare variants in 1,031 ASD-associated genes, we were able to identify pathogenic or likely pathogenic variants, 14 of which were de novo, in 20.4% (N 30/147) of patients. In two patients, two variants were identified. One of these patients was presumed to be compound heterozygous with de novo and maternally inherited variants, while the other patient had a likely pathogenic and a VUS variant in two different genes. One patient has a maternally inherited variant that was also present in the younger brother with phenotype consistency. In one patient, a variant was excluded in the mother but not in the father. Segregation was not tested in 13 patients as parental samples were not available for further analysis. One of these 13 patients was the carrier of both the likely pathogenic and VUS variants in 2 different genes. The diagnostic yield was further increased by 2.7% (N 4/147) by adding the analysis of ultrarare variants detected in an additional 242 NDDs genes not listed in the SFARI database (Supplementary Table 1). Of these genes, one variant was de novo, one was maternally inherited, one variant was not from the mother, and segregation was not analyzed in one variant. The overall diagnostic yield was estimated to be 23.1% (N 34/147). VUS variants were detected in 10.2% (N 15/147) of patients, of which 4.1% (N 6/147) were detected by adding additional NDDs gene analysis. In 11 patients with VUS variants in all genes together, segregation was not tested, 1 was maternally and 2 were paternally inherited. In one patient, inheritance was excluded from the mother but not from the father, and one had a de novo variant. Our results are summarized in Table 1. Patient characteristics and identified pathogenic, likely pathogenic, and VUS variants are listed in Table 2.
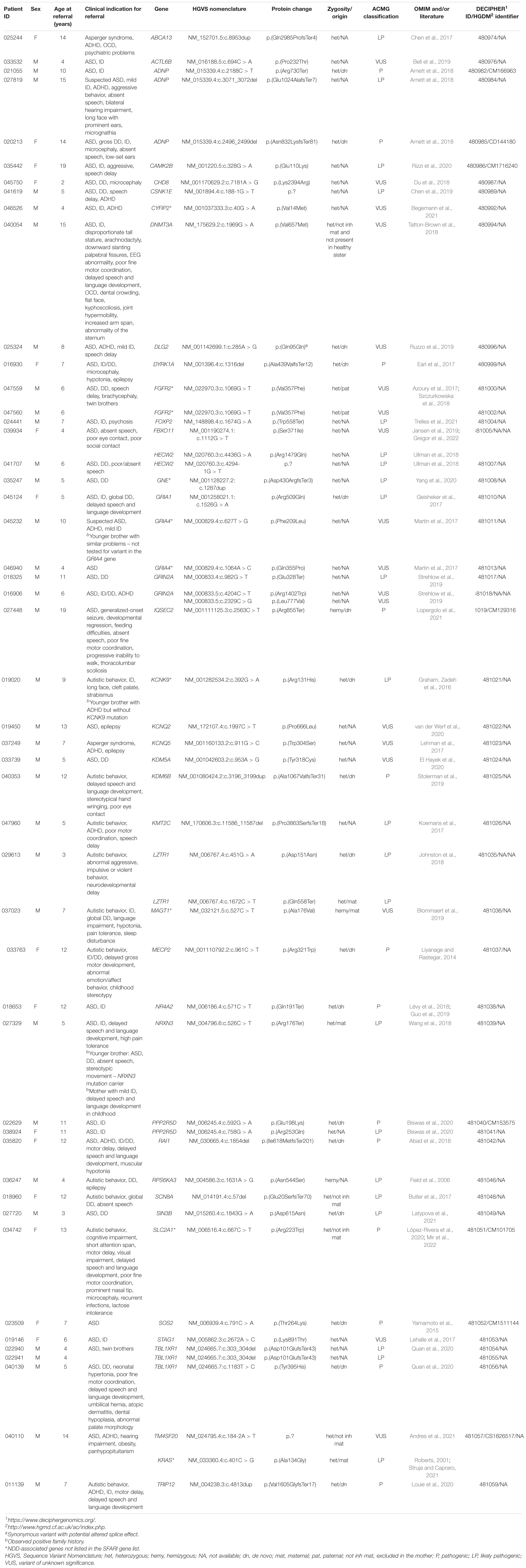
Table 2. Description of patients clinical characteristics for molecular diagnostic referral and identified as pathogenic, likely pathogenic, and VUS variants in the genes.
The occurrence of variants per gene for clinically significant variants analyzed in this cohort is presented in Figure 2. From the chart in the figure, it can be seen that in 18 out of 42 genes, the variant occurred as a “single event” (meaning we detected 1 variant per gene in all patients combined). Benign and likely benign variants were detected in the ABCA13, DNMT3A, KMT2C, and TRIP12 genes. In the other genes, only ACMG class 3–5 variants were found.
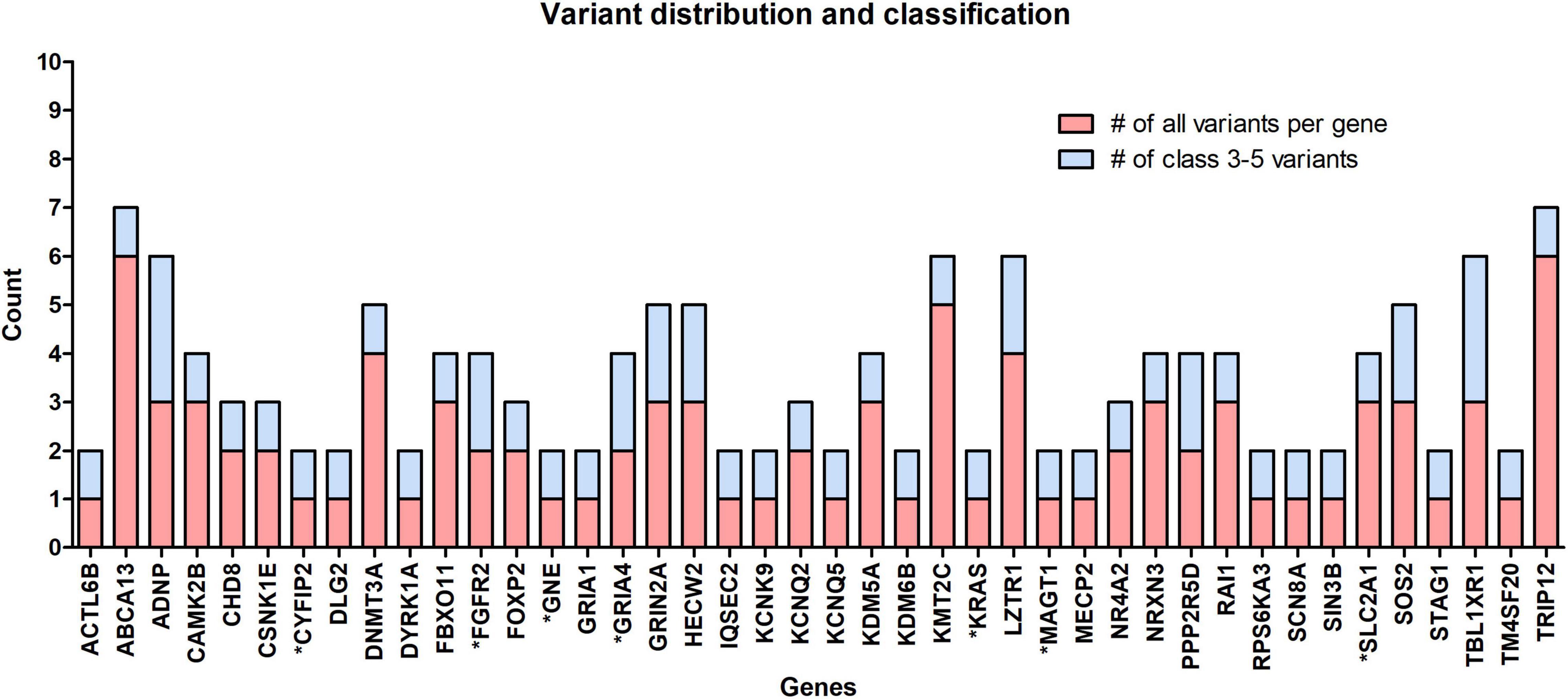
Figure 2. The occurrence of all rare variants per gene (red) and the number of those classified as clinically significant variants (blue). In 18 out of 42 identified genes, 1 variant per gene occurred, the so-called “single event” variant. Genes marked with an asterisk (*) belong to the group of NDD-associated genes.
Protein Function Predictions and Gene Prioritizations
Functional enrichment analysis of 42 genes showed that they were predominantly involved in the development of the nervous system with a p-value of 4.16e−06 (N 22/42). Genes can be ranked into three major groups according to their molecular function. The first group consists of chromatin-binding proteins (GO:0003682): ACTL6B, ADNP, CHD8, DNMT3A, KDM5A, KDM6B, MECP2, SIN3B, and STAG1. The second group consists of proteins with gate channel activity: GRIA1, GRIA4, GRIN2A, KCNK9, KCNQ2, KCNQ5, and SCN8A. The third group consists of proteins that share transcriptional regulatory function (GO:0140110) with ADNP, ACTL6B, KDM5A, MECP2, and SIN3B proteins, and they include DYRK1A, FOXP2, KMT2C, LZTR1, NR4A2, RAI1, and TBL1XR1. Other proteins are involved in processes, such as transmembrane transporter activity (GO:0022857) (ABCA13, MAGT1, and SLC2A1) and regulation of molecular functions (GO:0098772) (IQSEC2, PPP2R5D, RPS6KA3, and SOS2).
Disease-gene associations showed that 28 out of 42 genes analyzed were involved in mental health diseases (p-value of 4.97e−27), of which 22 genes were also linked to ID (p-value of 8.58e−23). Only four of these genes (ADNP, CHD8, DYRK1A, and MECP2) were linked to ASD (p-value of 7.9e−4). Clustering 42 genes using the STRING tool generated 4 gene clusters that were colored red (15 genes), yellow (11 genes), blue (8 genes), and green (8 genes). The largest red cluster consisted of three Noonan-causing genes KRAS, LZTR1, and SOS2 (Johnston et al., 2018). The KRAS gene interacted with other genes in this node, as well as the shared ID in disease-gene association with six other genes (colored red in the isolated figure form). The yellow-colored node encompassed the three Rett syndrome-causing genes MECP2, GRIN2A, and TBL1XR1 (colored red in Figure 3A), but mutations in other genes in this node were also involved in a Rett-like phenotype (Wang et al., 2019). The blue-colored node consists of the ASD-associated genes ADNP, CHD8, and DYRK1A (colored green in Figure 3C), but the majority of genes in this node are predominantly linked to ID (colored blue in Figure 3C). Functional enrichment analysis in this node also showed that five of the eight proteins (ACTL6B, ANDP, CHD8, KDM5A, and KDM6B) were chromatin binding (GO:0003682) (p-value of 3.6e−3). The green-colored node consists of eight genes, six of which code for proteins involved in transmembrane transporter activity (GO:0022857) (p-value of 3.6e−3) and were also linked to ID (colored red in the isolated figure form) with the exception of the ABCA13 gene.
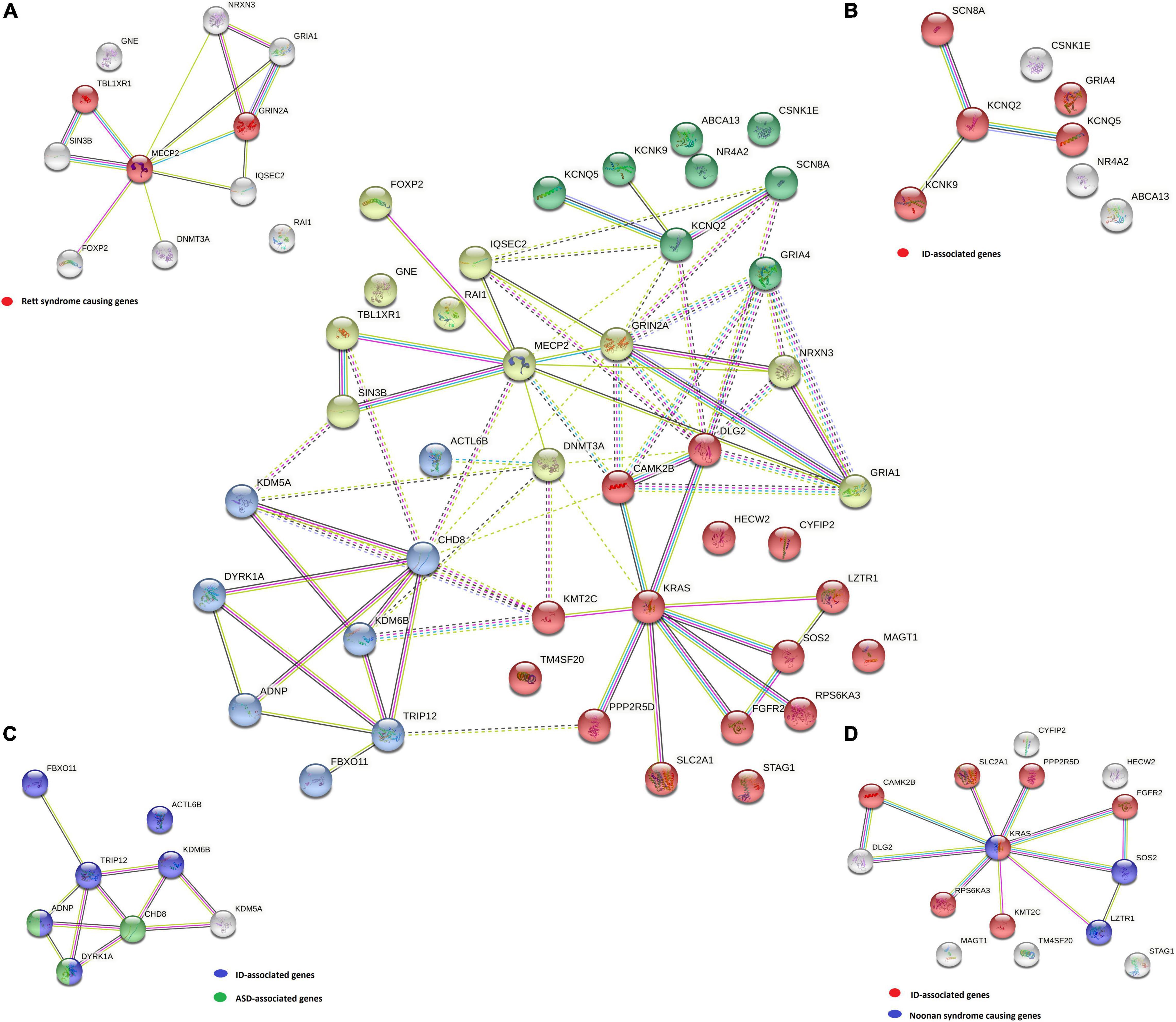
Figure 3. A STRING k-means clustering classified the genes into four groups: yellow (A), green (B), blue (C), and red (D) by possible correlation in the same biological pathways and/or involvement in specific phenotypes (diseases). For each group, specific disease-gene associations are marked on the isolated figure forms (A–D).
All four gene groups are presented in the center of Figure 3. Disease-gene associations are labeled for each group separately in Figures 3A–D.
On the contrary, using the g:Profiler tool for the same set of genes, it was clearly shown that ASD under HP:0000729 (purple bubble 2 in Figure 4) is the main feature (p-value of 2.457e−10) linked to the analyzed set of genes. Features, such as delayed speech and language, language impairment, global DD, neurodevelopmental delay, ID, and hyperactivity, and also the features observed in our patients, were significantly found less frequently than ASD (purple bubbles 3–8 in Figure 4). As observed in the STRING analysis, the GO biological process analysis showed that the analyzed genes were predominantly involved in the development of the nervous system (GO:0007399) (p-value of 1.878 × e−6). All the data from the g:Profiler analysis are available online.
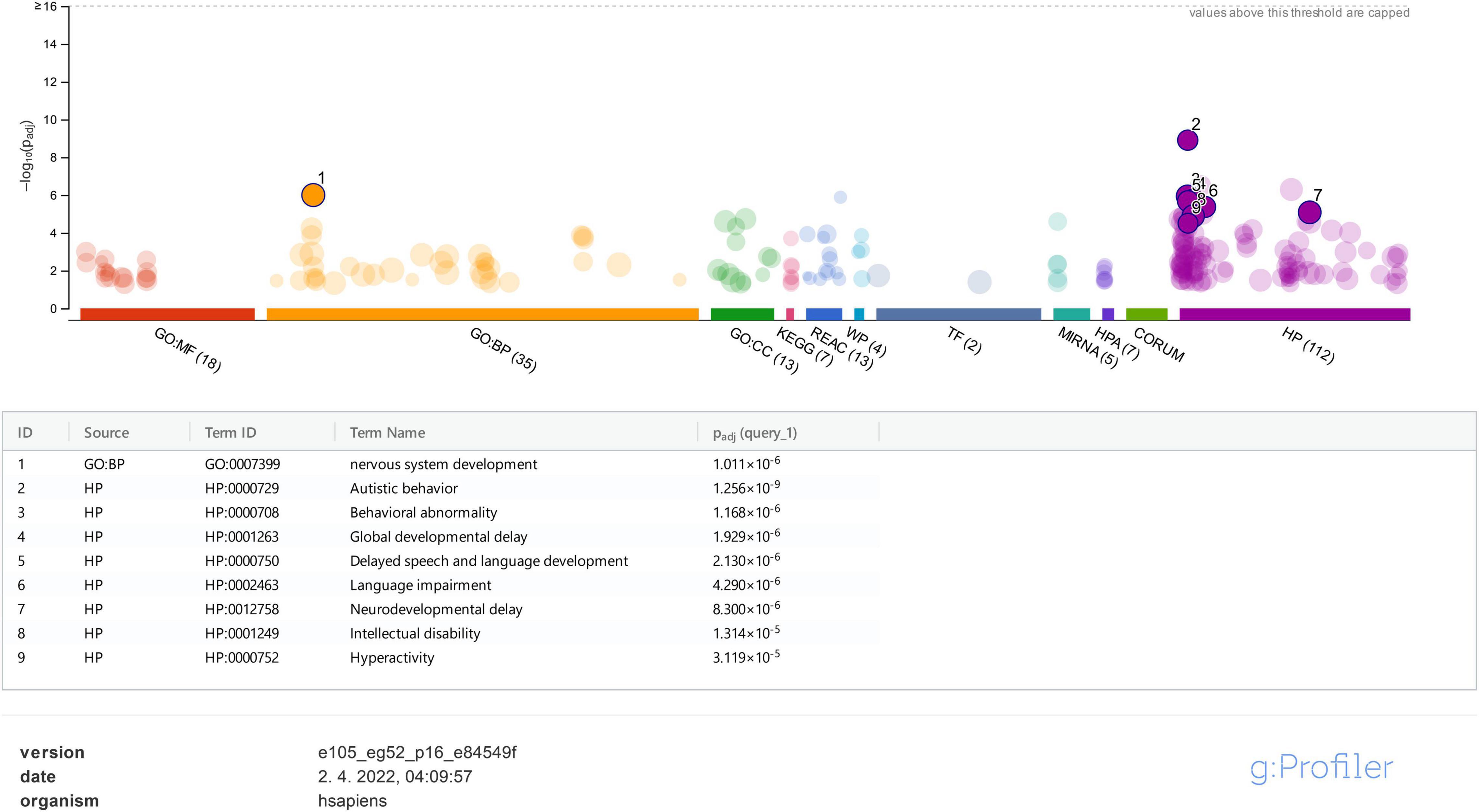
Figure 4. g:Profiler analysis for the studied genes based on GO is presented for molecular function (MF), biological process (BP), cellular component (CC), biological pathways (KEGG, Reactome, and WikiPathways), regulatory motifs in DNA (TF and MIRNA), protein databases (HPA and CORUM), and Human Phenotype Ontology (HP). Only data for BP and HP were analyzed and represented in the figure.
Discussion
The introduction of WES in the genetic diagnosis of ASD has significantly improved our knowledge of its etiology at the molecular level. The phenotype-driven analysis is often a limitation in the discovery of new disease-associated genes since the clinical presentation of patients with ASD is a key factor in genetic testing and data interpretation (Arteche-López et al., 2021; Lintas et al., 2021). The process of establishing an accurate diagnosis is further hindered by the lack of segregation analysis, reduced penetrance, limited data availability in the literature, as well as non-specific and limited clinical descriptions of patients. Therefore, reverse phenotyping and seeking the ultrarare variants in genes that are not only limited to ASD and then performing functional studies to obtain datasets should result in a higher diagnostic yield (Lintas et al., 2021).
In our study, a WES analysis was performed in 147 pediatric patients with suspected ASD. Genotype–phenotype analysis of 1,031 ASD-associated genes enabled the detection of pathogenic or likely pathogenic variants in 20.4% (N 30/147) of patients, of which almost half (N 14/30) were shown to be de novo in subsequent segregation analysis. The high incidence of de novo variants was expected in our study since it is estimated that de novo events contribute to autism in 30–39% of all patients with ASD (Yoon et al., 2021). In addition, one had a maternally inherited variant, and one patient was presumed to be a compound heterozygote, harboring one maternally inherited and one de novo variant. Adding 242 NDD-associated genes (Supplementary Table 1) to the genotype–phenotype analysis resulted in the detection of pathogenic/likely pathogenic variants in four more patients and increased the diagnostic yield to 23.1% (N 34/147). A VUS variant was detected in 10.2% (N 15/147) of patients. All the variants and patient clinical characteristics are listed in Tables 1, 2.
Protein function predictions and gene prioritization analyses were performed for genes identified in our cohort to establish possible correlations between already known ASD-associated genes and those not listed in the SFARI database. The disease-gene associations analysis (Figure 1) shows that the majority of detected variants are associated with an ID phenotype, and thus we presumed that our patients mostly have a syndromic ASD since autistic behavior (HP:0000729) is strongly associated with all of the analyzed genes (Figure 4). According to the literature, ASD is comorbidity with ID in 32% of patients (Miot et al., 2019). Our disease-gene associations showed that 22 out of 42 genes analyzed were also linked to ID (p-value of 8.58e−23), whereas only 4 genes (ADNP, CHD8, DYRK1A, and MECP2) were linked to ASD (p-value of 7.9e−4). As expected, functional enrichment analysis confirmed that genes involved in the etiology of ASD were predominantly involved in the development of the nervous system, and mutations in these genes, in our cases, altered their transcriptional regulatory functions or transmembrane transporter activity, which are known molecular mechanisms in the etiology of ASD (Rylaarsdam and Guemez-Gamboa, 2019).
Functional enrichment analysis for genes not listed in the SFARI database also demonstrated their association with the already known ASD-associated genes. For example, based on their functional similarity, the KRAS, SLC2A1, and FGFR2 genes were clustered in the red node. The KRAS gene, along with LZTR1 and SOS2, is one of the three Noonan syndrome-causing genes in this group. They cause dysregulation of the RAS-MaPK signaling pathway (Tartaglia et al., 2011) and are also linked to ID (Figure 3D). The maternally inherited pathogenic variant in the KRAS gene explains the panhypopituitarism observed in our patient (Patient 040110) (Struja and Capraro, 2021). Although the KRAS gene is not linked to ASD, ASD was observed in Patient 040110, presumed compound heterozygous variants in LZTR1 in Patient 029623, and a de novo variant in SOS2 in Patient 023509, are a probably causative in all three patients since ADHD and behavioral problems are reported in the Noonan syndrome phenotype (Roberts, 2001). For Patient 029623, additional analyses should be performed to establish whether the variants are truly biallelic since compound heterozygosity was presumed based only on the genotype–phenotype correlation. Patient 040110 is also a carrier of a VUS variant in the TM4SF20 gene with a predicted acceptor loss score of Δ0.99 using the SpliceAI tool,5 associated with language impairment (Andres et al., 2021). However, this feature was not reported in our patient (Table 2). This can be explained by the reduced penetrance observed for TM4SF20 truncation carriers (Wiszniewski et al., 2013). Our analysis showed that the KRAS gene is functionally linked to the SLC2A1 gene, which is also not reported as an ASD-associated gene. The abovementioned connection stems from tumor genetics since glucose deprivation has been shown to contribute to the development of KRAS pathway mutations in tumor cells (Yun et al., 2009). A presumed de novo pathogenic mutation in the SLC2A1 gene was detected in a 13-year-old girl (Patient 034742) with a severe phenotype, which reverse phenotyping has shown, is a cause of the GLUT1 deficiency syndrome (López-Rivera et al., 2020; Mir et al., 2022). Another gene, not reported to be associated with ASD, but which can be linked to KRAS, is the FGFR2 gene since they are both involved in the RTK/Ras/MAPK signaling pathway (Das et al., 2014). The paternally inherited VUS variant was detected by family-WES analyses in twin brothers with ASD, DD, speech delay, and brachycephaly. Mutations in the FGFR2 gene with incomplete penetrance have been reported to cause a syndromic form of craniosynostosis with ID/DD (Azoury et al., 2017). To the best of our knowledge, no clear association of this gene with ASD has been established, although a functional study in mice has shown that dysregulation of the Fgfr2 gene leads to impaired core behavior related to ASD (Szczurkowska et al., 2018). For all three abovementioned genes, the g:Profiler tool showed involvement with high significance in behavioral abnormalities (HP:0000708), DD (HP:0001263), ID (HP:0001249), and also in autistic behavior (HP:0000729) for the SLC2A1 gene (Figure 4 and data available online).
Similar correlations can be made for other non-SFARI genes in the other three clusters mentioned above. Two such genes are the KCNK9 and GRIA4 genes in the green cluster (Figure 3B). The pathogenic variant in the KCNK9 gene was identified as a “single event” variant in NDD-associated genes (Figure 2). Reverse phenotyping revealed that Patient 019020 has a clinical feature of the KCNK9 imprinting syndrome (Graham, Zadeh et al., 2016). Segregation analysis revealed a de novo variant, presumably on the maternal allele.
Two different VUS variants were detected in the GRIA4 gene in two male patients (Patients 045232 and 046940) who share ASD as a common clinical feature. Segregation analysis was not performed in either case. Although the GRIA4 gene is not listed in the SFARI database, de novo heterozygous pathogenic variants in the GRIA4 gene have already been reported in patients with an ASD-like phenotype (Martin et al., 2017). The KCNK9 and GRIA4 genes were also clustered together with KCNQ2, KCNQ5, and SCN8A (Figure 3B). Five of eight genes in this cluster share gate channel activity (GO:0022836) and are involved in the ASD/ID/DD phenotype according to g:Profiler analysis (data available online).
Additional gene correlations were also identified by gene clustering. In the yellow node, according to WikiPathways data (Martens et al., 2021), there are three Rett syndrome-causing genes (Figure 3A). Genes, such as IQSEC2 and even KCNQ2 from the green node, are linked to these genes since both genes are involved in the Rett-like syndrome phenotype (Wang et al., 2019).
Despite an attempt to establish a true protein function prediction analysis for some genes in our cohort, no connection could be made. Their role in a patient’s phenotype was set by focusing on “single event” variants. In almost half of the cases (18 out of 42 genes), the variant occurred as a “single event.” This means that we detected one variant per gene in all patients combined (Figure 2), which confirms that ultrarare variants are a key factor in the etiology of ASD, and new genes will emerge by adding new patient cohorts. Among non-SFARI listed genes, variants were detected in the CYFIP2, GNE, and MAGT1 genes. Using the g:Profiler tool, all three genes were found to be linked to NDD features (data available online), while the same three genes have been described in patients with an ASD-associated phenotype in the literature in recent years (Blommaert et al., 2019; Yang et al., 2020; Begemann et al., 2021). In the future, functional studies, variant segregation, and better genotype–phenotype analysis should be performed for this category of genes to establish their true roles in a patient’s phenotype.
These same studies should be performed for Patient 039934, a female with variants in both the FBXO11 and HECW2 genes, which are both associated with a complex NDD phenotype that includes autistic features, ID, and abnormalities in language development, features also present in our proband. A splice variant in the HECW2 gene with a predicted acceptor loss score Δ of 1.00 using the SpliceAI tool was detected in Patient 041707 with a similar phenotype consisting of ASD, DD, and poor/absent speech. Since segregation analysis was not performed in either of these patients, the true significance of the variants for the patients’ phenotype is not clear. For both genes, de novo variants in the genes were reported as causative, hence variant segregation and better genotype–phenotype analysis are essential (Ullman et al., 2018; Jansen et al., 2019). Therefore, despite both variants in the HECW2 gene being classified as likely pathogenic, at present, they should be treated as VUS.
By defining ultrarare and “single event” variants in the ASD-associated genes, variants in rarely reported patients with ASD in the literature were identified.
A pathogenic variant, later established as de novo after reverse phenotyping and segregation, was detected in a 12-year-old girl (Patient 018653) with ASD and ID. The NR4A2 gene encodes nuclear receptor 4A subfamily (Nr4a) of transcription factors involved in hippocampal synaptic plasticity and cognitive functions (Català-Solsona et al., 2021). NR4A2 haploinsufficiency caused by deletions or a frameshift mutation in this gene and associated with ID and ASD has been reported in the literature (Lévy et al., 2018; Guo et al., 2019). To the best of our knowledge, our patient has the first stop-gain mutation reported in the literature that confirms a de novo loss-of-function (LoF) mutation involved in the etiology of ASD. A different variant (Figure 2), also found in the NR4A2 gene in another patient, was marked as not causative since it was also present in the mother who had no health problems.
In a 5-year-old girl with ASD, ID/DD, and delayed speech and language development (Patient 045124), a “single event” variant is a likely pathogenic de novo variant in the GRIA1 gene. The GRIA1 gene encoding the α-amino-3-hydroxy-5-methyl-4-isoxazolepropionic acid (AMPA) receptor subunit GLUA1 has been reported in wide neurological and psychiatric disorders, including schizophrenia (Bygrave et al., 2019). In a large study of 17,688 patients with NDDs, 21 patients were carriers of a mutation causing a 636 amino acid change from alanine to threonine in the GRIA1 protein. These patients showed evidence of specific learning disabilities and autism (Geisheker et al., 2017). Hence, our patient represents a new mutational and phenotypic spectrum of patients with GRIA1 gene haploinsufficiency.
A missense variant in the SIN3B gene was also found as a “single event” variant in Patient 023509 with ASD and DD. This variant was classified as a VUS according to ACMG guidelines, but WES-trio showed that the variant occurred de novo. The SIN3B gene encodes a transcription corepressor which has an important role in histone deacetylation and transcriptional repression (Latypova et al., 2021). An animal model showed that Sin3b knockout mice and zebrafish sin3b mutants express skeletal and growth defects (David et al., 2008; Moravec et al., 2017). Sin3b knockout mice also showed defects in blood differentiation, whereas zebrafish sin3b mutants showed locomotor defects (Cantor and David, 2017; Moravec et al., 2017). Although STRING analysis did not show any correlation with a specific phenotype (data available online), both g:Profiler analysis and the literature have reported that haploinsufficiency of SIN3B causes an ID and ASD phenotypes (Latypova et al., 2021).
There are several limitations to our study. First and foremost, limitations are the limited sample size and the lack of segregation and functional studies, especially for VUS variants and variants in poorly researched genes. Second, we focused only on non-synonymous coding variants, and by using a filtering process, we probably eliminated variants that were poorly covered or were present with low allelic frequency. For genes where protein function predictions and gene prioritization analysis results were not obtained, functional enrichment analysis with all ASD-associated genes should be performed in the future.
The abovementioned cases demonstrate that by performing WES analysis for patients with ASD without focusing on ASD-associated genes but including gene lists for genes that are functionally similar or linked to them, we yielded a higher diagnostic output, despite the limited clinical data. Reverse phenotyping by a clinician can then be performed to determine whether the variant in the analyzed gene is clinically relevant for the patient. In our study, focusing on the ultrarare variants or “single event” variants per gene regardless of their previously known molecular function was crucial for patients with complex phenotypes. This is illustrated by the high proportion of de novo variants identified as causative. Our study also demonstrates that most of the causative genes are involved in a syndromic form of ASD. In most patients, this is not the main feature, but rather part of a more complicated phenotype seen in these patients. Thus, classical genotype–phenotype analysis of WES data would be time-consuming, and perhaps in some cases, proper genotype–phenotype correlation would not be made due to several factors: a variable phenotype in specific disorders, overlapping clinical features, the revolving phenotype in small children, or reduced penetrance.
Data Availability Statement
The datasets presented in this study can be found in online repositories. The names of the repositories and accession numbers can be found in the article. The datasets analyzed for this study can be found on the g:Profiler website (https://biit.cs.ut.ee/gplink/l/iGN_PmjmTv).
Ethics Statement
The studies involving human participants were reviewed and approved by the Commission of the Republic of Slovenia for Medical Ethics. Written informed consent was obtained from the individual(s), and minor(s)’ legal guardian/next of kin, for the publication of any potentially identifiable images or data included in this article.
Author Contributions
DK designed the study, analyzed the NGS data, performed the functional enrichment analysis and data interpretation, performed the validation by Sanger sequencing and the segregation analysis, and wrote the manuscript. MG and NR performed the NGS variant analysis workflow. MG participated in the chart and figure formation. IO and HG evaluated the patients. SS performed the validation by Sanger sequencing. MG, IO, SS, HG, PD, and NK revised the manuscript. All authors have read and approved the manuscript.
Funding
The authors acknowledge that the Advanced Genomic Analyses of Slovenian Children With Autistic Spectrum Disorders project [grant number Z3-9294 (B)] and the Comparative Genomics and Genome Biodiversity Research Program (grant number P4-0220) were financially supported by the Slovenian Research Agency.
Conflict of Interest
The authors declare that the research was conducted in the absence of any commercial or financial relationships that could be construed as a potential conflict of interest.
Publisher’s Note
All claims expressed in this article are solely those of the authors and do not necessarily represent those of their affiliated organizations, or those of the publisher, the editors and the reviewers. Any product that may be evaluated in this article, or claim that may be made by its manufacturer, is not guaranteed or endorsed by the publisher.
Acknowledgments
We are grateful to all the patients and their families for their cooperation. We also like to express gratitude to our student Tim Ravnjak for his substantial assistance with NGS data analysis, to Barbara Stojanov for participating in the evaluation of patients, and to our colleagues Lara Forstner, Leon Maric, and Spela Buneto for performing Sanger sequencing.
Supplementary Material
The Supplementary Material for this article can be found online at: https://www.frontiersin.org/articles/10.3389/fnmol.2022.912671/full#supplementary-material
Footnotes
- ^ https://gene.sfari.org/tools/
- ^ https://franklin.genoox.com/clinical-db/home
- ^ https://string-db.org/
- ^ https://biit.cs.ut.ee/gprofiler/gost
- ^ spliceailookup.broadinstitute.org
References
Abad, C., Cook, M. M., Cao, L., Jones, J. R., Rao, N. R., Dukes-Rimsky, L., et al. (2018). A rare de novo RAI1 gene mutation affecting BDNF-enhancer-driven transcription activity associated with autism and atypical smith-magenis syndrome presentation. Biology 7:31. doi: 10.3390/biology7020031
Adzhubei, I., Jordan, D. M., and Sunyaev, S. R. (2013). Predicting functional effect of human missense mutations using PolyPhen-2. Curr. Protoc. Hum. Genet. 7:20. doi: 10.1002/0471142905.hg0720s76
Adzhubei, I. A., Schmidt, S., Peshkin, L., Ramensky, V. E., Gerasimova, A., Bork, P., et al. (2010). A method and server for predicting damaging missense mutations. Nat. Methods 7, 248–249. doi: 10.1038/nmeth0410-248
Andres, E. M., Neely, H. L., Hafeez, H., Yasmin, T., Kausar, F., Basra, M. A. R., et al. (2021). Study of rare genetic variants in TM4SF20, NFXL1, CNTNAP2, and ATP2C2 in Pakistani probands and families with language impairment. Meta Gene 30:100966. doi: 10.1016/j.mgene.2021.100966
Arnett, A. B., Rhoads, C. L., Hoekzema, K., Turner, T. N., Gerdts, J., Wallace, A. S., et al. (2018). The autism spectrum phenotype in ADNP syndrome. Autism Res. 11, 1300–1310. doi: 10.1002/aur.1980
Arteche-López, A., Gómez Rodríguez, M. J., Sánchez Calvin, M. T., Quesada-Espinosa, J. F., Lezana Rosales, J. M., Palma Milla, C., et al. (2021). Towards a change in the diagnostic algorithm of autism spectrum disorders: evidence supporting whole exome sequencing as a first-tier test. Genes 12:560. doi: 10.3390/genes12040560
Azoury, S. C., Reddy, S., Shukla, V., and Deng, C. X. (2017). Fibroblast growth factor receptor 2 (FGFR2) mutation related syndromic craniosynostosis. Int. J. Biol. Sci. 13, 1479–1488. doi: 10.7150/ijbs.22373
Balicza, P., Varga, N. Á, Bolgár, B., Pentelényi, K., Bencsik, R., Gál, A., et al. (2019). Comprehensive analysis of rare variants of 101 autism-linked genes in a hungarian cohort of autism spectrum disorder patients. Front. Genet. 10:434. doi: 10.3389/fgene.2019.00434
Begemann, A., Sticht, H., Begtrup, A., Vitobello, A., Faivre, L., Banka, S., et al. (2021). New insights into the clinical and molecular spectrum of the novel CYFIP2-related neurodevelopmental disorder and impairment of the WRC-mediated actin dynamics. Genet. Med. 23, 543–554. doi: 10.1038/s41436-020-01011-x
Bell, S., Rousseau, J., Peng, H., Aouabed, Z., Priam, P., Theroux, J. F., et al. (2019). Mutations in ACTL6B cause neurodevelopmental deficits and epilepsy and lead to loss of dendrites in human neurons. Am. J. Hum. Genet. 104, 815–834. doi: 10.1016/j.ajhg.2019.03.022
Bernier, R., Golzio, C., Xiong, B., Stessman, H. A., Coe, B. P., Penn, O., et al. (2014). Disruptive CHD8 mutations define a subtype of autism early in development. Cell 158, 263–276. doi: 10.1016/j.cell.2014.06.017
Biswas, D., Cary, W., and Nolta, J. A. (2020). PPP2R5D-related intellectual disability and neurodevelopmental delay: a review of the current understanding of the genetics and biochemical basis of the disorder. Int. J. Mol. Sci. 21:1286. doi: 10.3390/ijms21041286
Blommaert, E., Péanne, R., Cherepanova, N. A., Rymen, D., Staels, F., Jaeken, J., et al. (2019). Mutations in MAGT1 lead to a glycosylation disorder with a variable phenotype. Proc. Natl. Acad. Sci. U.S.A. 116, 9865–9870. doi: 10.1073/pnas.1817815116
Bolger, A. M., Lohse, M., and Usadel, B. (2014). Trimmomatic: a flexible trimmer for Illumina sequence data. Bioinformatics 30, 2114–2120. doi: 10.1093/bioinformatics/btu170
Butler, K. M., da Silva, C., Shafir, Y., Weisfeld-Adams, J. D., Alexander, J. J., Hegde, M., et al. (2017). De novo and inherited SCN8A epilepsy mutations detected by gene panel analysis. Epilepsy Res. 129, 17–25. doi: 10.1016/j.eplepsyres.2016.11.002
Bygrave, A. M., Jahans-Price, T., Wolff, A. R., Sprengel, R., Kullmann, D. M., Bannerman, D. M., et al. (2019). Hippocampal-prefrontal coherence mediates working memory and selective attention at distinct frequency bands and provides a causal link between schizophrenia and its risk gene GRIA1. Transl. Psychiatry 9:142. doi: 10.1038/s41398-019-0471-0
Cantor, D. J., and David, G. (2017). The chromatin-associated Sin3B protein is required for hematopoietic stem cell functions in mice. Blood 129, 60–70. doi: 10.1182/blood-2016-06-721746
Català-Solsona, J., Miñano-Molina, A. J., and Rodríguez-Álvarez, J. (2021). Nr4a2 transcription factor in hippocampal synaptic plasticity, memory and cognitive dysfunction: a perspective review. Front. Mol. Neurosci. 14:786226. doi: 10.3389/fnmol.2021.786226
Chen, J., Khan, R. A. W., Wang, M., He, K., Wang, Q., Li, Z., et al. (2017). Association between the variability of the ABCA13 gene and the risk of major depressive disorder and schizophrenia in the Han Chinese population. World J. Biol. Psychiatry 18, 550–556. doi: 10.1080/15622975.2016.1245442
Chen, X., Jin, J., Wang, Q., Xue, H., Zhang, N., Du, Y., et al. (2019). A de novo pathogenic CSNK1E mutation identified by exome sequencing in family trios with epileptic encephalopathy. Hum. Mutat. 40, 281–287. doi: 10.1002/humu.23690
Das, K., Gunasegaran, B., Tan, I. B., Deng, N., Lim, K. H., and Tan, P. (2014). Mutually exclusive FGFR2, HER2, and KRAS gene amplifications in gastric cancer revealed by multicolour FISH. Cancer Lett. 353, 167–175. doi: 10.1016/j.canlet.2014.07.021
David, G., Grandinetti, K. B., Finnerty, P. M., Simpson, N., Chu, G. C., and Depinho, R. A. (2008). Specific requirement of the chromatin modifier mSin3B in cell cycle exit and cellular differentiation. Proc. Natl. Acad. Sci. U.S.A. 105, 4168–4172. doi: 10.1073/pnas.0710285105
DePristo, M. A., Banks, E., Poplin, R., Garimella, K. V., Maguire, J. R., Hartl, C., et al. (2011). A framework for variation discovery and genotyping using next-generation DNA sequencing data. Nat. Genet. 43, 491–498. doi: 10.1038/ng.806
Desvignes, J. P., Bartoli, M., Delague, V., Krahn, M., Miltgen, M., Béroud, C., et al. (2018). VarAFT: a variant annotation and filtration system for human next generation sequencing data. Nucleic Acids Res. 46, W545–W553. doi: 10.1093/nar/gky471
Du, X., Gao, X., Liu, X., Shen, L., Wang, K., Fan, Y., et al. (2018). Genetic diagnostic evaluation of trio-based whole exome sequencing among children with diagnosed or suspected autism spectrum disorder. Front. Genet. 9:594. doi: 10.3389/fgene.2018.00594
Earl, R. K., Turner, T. N., Mefford, H. C., Hudac, C. M., Gerdts, J., Eichler, E. E., et al. (2017). Clinical phenotype of ASD-associated DYRK1A haploinsufficiency. Mol. Autism. 8:54. doi: 10.1186/s13229-017-0173-5
El Hayek, L., Tuncay, I. O., Nijem, N., Russell, J., Ludwig, S., Kaur, K., et al. (2020). KDM5A mutations identified in autism spectrum disorder using forward genetics. eLife 9:e56883. doi: 10.7554/eLife.56883
Field, M., Tarpey, P., Boyle, J., Edkins, S., Goodship, J., Luo, Y., et al. (2006). Mutations in the RSK2(RPS6KA3) gene cause Coffin-Lowry syndrome and nonsyndromic X-linked mental retardation. Clin. Genet. 70, 509–515. doi: 10.1111/j.1399-0004.2006.00723.x
Geisheker, M. R., Heymann, G., Wang, T., Coe, B. P., Turner, T. N., Stessman, H. A. F., et al. (2017). Hotspots of missense mutation identify neurodevelopmental disorder genes and functional domains. Nat. Neurosci. 0, 1043–1051. doi: 10.1038/nn.4589
Graham, J. M., Zadeh, N., Kelley, M., Tan, E. S., Liew, W., Tan, V., et al. (2016). KCNK9 imprinting syndrome-further delineation of a possible treatable disorder. Am. J. Med. Genet. A 170, 2632–2637. doi: 10.1002/ajmg.a.37740
Gregor, A., Meerbrei, T., Gerstner, T., Toutain, A., Lynch, S. A., Stals, K., et al. (2022). De novo missense variants in FBXO11 alter its protein expression and subcellular localization. Hum. Mol. Genet. 31, 440–454. doi: 10.1093/hmg/ddab265
Guo, H., Duyzend, M. H., Coe, B. P., Baker, C., Hoekzema, K., Gerdts, J., et al. (2019). Genome sequencing identifies multiple deleterious variants in autism patients with more severe phenotypes. Genet. Med. 21, 1611–1620. doi: 10.1038/s41436-018-0380-2
Jansen, S., van der Werf, I. M., Innes, A. M., Afenjar, A., Agrawal, P. B., Anderson, I. J., et al. (2019). De novo variants in FBXO11 cause a syndromic form of intellectual disability with behavioral problems and dysmorphisms. Eur. J. Hum. Genet. 27, 738–746. doi: 10.1038/s41431-018-0292-2
Johnston, J. J., van der Smagt, J. J., Rosenfeld, J. A., Pagnamenta, A. T., Alswaid, A., Baker, E. H., et al. (2018). Autosomal recessive Noonan syndrome associated with biallelic LZTR1 variants. Genet. Med. 20, 1175–1185. doi: 10.1038/gim.2017.249
Koemans, T. S., Kleefstra, T., Chubak, M. C., Stone, M. H., Reijnders, M. R. F., de Munnik, S., et al. (2017). Functional convergence of histone methyltransferases EHMT1 and KMT2C involved in intellectual disability and autism spectrum disorder. PLoS Genet. 13:e1006864. doi: 10.1371/journal.pgen.1006864
Krgovic, D., Kokalj Vokac, N., Zagorac, A., and Gregoric Kumperscak, H. (2018). Rare structural variants in the DOCK8 gene identified in a cohort of 439 patients with neurodevelopmental disorders. Sci. Rep. 8:9449. doi: 10.1038/s41598-018-27824-0
Kumar, P., Henikoff, S., and Ng, P. C. (2009). Predicting the effects of coding non-synonymous variants on protein function using the SIFT algorithm. Nat. Protoc. 4, 1073–1081. doi: 10.1038/nprot.2009.86
Latypova, X., Vincent, M., Mollé, A., Adebambo, O. A., Fourgeux, C., Khan, T. N., et al. (2021). Haploinsufficiency of the Sin3/HDAC corepressor complex member SIN3B causes a syndromic intellectual disability/autism spectrum disorder. Am. J. Hum. Genet. 108, 929–941. doi: 10.1016/j.ajhg.2021.03.017
Lehalle, D., Mosca-Boidron, A. L., Begtrup, A., Boute-Benejean, O., Charles, P., Cho, M. T., et al. (2017). STAG1 mutations cause a novel cohesinopathy characterised by unspecific syndromic intellectual disability. J. Med. Genet. 54, 479–488. doi: 10.1136/jmedgenet-2016-104468
Lehman, A., Thouta, S., Mancini, G. M. S., Naidu, S., van Slegtenhorst, M., McWalter, K., et al. (2017). Loss-of-function and gain-of-function mutations in KCNQ5 cause intellectual disability or epileptic encephalopathy. Am. J. Hum. Genet. 101, 65–74. doi: 10.1016/j.ajhg.2017.05.016
Lévy, J., Grotto, S., Mignot, C., Maruani, A., Delahaye-Duriez, A., Benzacken, B., et al. (2018). NR4A2 haploinsufficiency is associated with intellectual disability and autism spectrum disorder. Clin. Genet. 94, 264–268. doi: 10.1111/cge.13383
Li, H., and Durbin, R. (2010). Fast and accurate long-read alignment with Burrows-Wheeler transform. Bioinformatics 26, 589–595. doi: 10.1093/bioinformatics/btp698
Lindstrand, A., Eisfeldt, J., Pettersson, M., Carvalho, C. M. B., Kvarnung, M., Grigelioniene, G., et al. (2019). From cytogenetics to cytogenomics: whole-genome sequencing as a first-line test comprehensively captures the diverse spectrum of disease-causing genetic variation underlying intellectual disability. Genome Med. 11:68. doi: 10.1186/s13073-019-0675-1
Lintas, C., Sacco, R., Azzarà, A., Cassano, I., and Gurrieri, F. (2021). Genotype-phenotype correlations in relation to newly emerging monogenic forms of autism spectrum disorder and associated neurodevelopmental disorders: the importance of phenotype reevaluation after pangenomic results. J. Clin. Med. 10:5060. doi: 10.3390/jcm10215060
Liyanage, V. R., and Rastegar, M. (2014). Rett syndrome and MeCP2. Neuromolecular Med. 16, 231–264. doi: 10.1007/s12017-014-8295-9
Lopergolo, D., Privitera, F., Castello, G., Lo Rizzo, C., Mencarelli, M. A., Pinto, A. M., et al. (2021). IQSEC2 disorder: a new disease entity or a Rett spectrum continuum? Clin. Genet. 99, 462–474. doi: 10.1111/cge.13908
López-Rivera, J. A., Pérez-Palma, E., Symonds, J., Lindy, A. S., McKnight, D. A., Leu, C., et al. (2020). A catalogue of new incidence estimates of monogenic neurodevelopmental disorders caused by de novo variants. Brain 143, 1099–1105. doi: 10.1093/brain/awaa051
Lord, C., Elsabbagh, M., Baird, G., and Veenstra-Vanderweele, J. (2018). Autism spectrum disorder. Lancet 392, 508–520. doi: 10.1016/S0140-6736(18)31129-2
Louie, R. J., Friez, M. J., Skinner, C., Baraitser, M., Clark, R. D., Schwartz, C. E., et al. (2020). Clark-Baraitser syndrome is associated with a nonsense alteration in the autosomal gene TRIP12. Am. J. Med. Genet. A 182, 595–596. doi: 10.1002/ajmg.a.61443
Martens, M., Ammar, A., Riutta, A., Waagmeester, A., Slenter, D. N., Hanspers, K., et al. (2021). WikiPathways: connecting communities. Nucleic Acids Res. 49, D613–D621. doi: 10.1093/nar/gkaa1024
Martin, S., Chamberlin, A., Shinde, D. N., Hempel, M., Strom, T. M., and Schreiber, A. (2017). De novo variants in GRIA4 lead to intellectual disability with or without seizures and gait abnormalities. Am. J. Hum. Genet. 101, 1013–1020. doi: 10.1016/j.ajhg.2017.11.004
McKenna, A., Hanna, M., Banks, E., Sivachenko, A., Cibulskis, K., Kernytsky, A., et al. (2010). The genome analysis toolkit: a MapReduce framework for analyzing next-generation DNA sequencing data. Genome Res. 20, 1297–1303. doi: 10.1101/gr.107524.110
Miller, D. T., Adam, M. P., Aradhya, S., Biesecker, L. G., Brothman, A. R., Carter, N. P., et al. (2010). Consensus statement: chromosomal microarray is a first-tier clinical diagnostic test for individuals with developmental disabilities or congenital anomalies. Am. J. Hum. Genet. 2010, 749–764. doi: 10.1016/j.ajhg.2010.04.006
Miot, S., Akbaraly, T., Michelon, C., Couderc, S., Crepiat, S., Loubersac, J., et al. (2019). Comorbidity burden in adults with autism spectrum disorders and intellectual disabilities-a report from the EFAAR (frailty assessment in ageing adults with autism spectrum and intellectual disabilities) study. Front. Psychiatry 10:617. doi: 10.3389/fpsyt.2019.00617
Mir, A., Almudhry, M., Alghamdi, F., Albaradie, R., Ibrahim, M., Aldurayhim, F., et al. (2022). SLC gene mutations and pediatric neurological disorders: diverse clinical phenotypes in a Saudi Arabian population. Hum. Genet. 141, 81–99. doi: 10.1007/s00439-021-02404-x
Moravec, C. E., Yousef, H., Kinney, B. A., Salerno-Eichenholz, R., Monestime, C. M., Martin, B. L., et al. (2017). Zebrafish sin3b mutants are viable but have size, skeletal, and locomotor defects. Dev. Dyn. 246, 946–955. doi: 10.1002/dvdy.24581
Poplin, R., Ruano-Rubio, V., DePristo, M. A., Fennell, T. J., Carneiro, M. O., Van der Auwera, G. A., et al. (2018). Scaling accurate genetic variant discovery to tens of thousands of samples. bioRXiv [Preprint]. doi: 10.1101/201178v3
Quan, Y., Zhang, Q., Chen, M., Wu, H., Ou, J., Shen, Y., et al. (2020). Genotype and phenotype correlations for TBL1XR1 in neurodevelopmental disorders. J. Mol. Neurosci. 70, 2085–2092. doi: 10.1007/s12031-020-01615-7
Raudvere, U., Kolberg, L., Kuzmin, I., Arak, T., Adler, P., Peterson, H., et al. (2019). g:Profiler: a web server for functional enrichment analysis and conversions of gene lists (2019 update). Nucleic Acids Res. 47, W191–W198. doi: 10.1093/nar/gkz369
Richards, S., Aziz, N., Bale, S., Bick, D., Das, S., Gastier-Foster, J., et al. (2015). Standards and guidelines for the interpretation of sequence variants: a joint consensus recommendation of the American College of Medical Genetics and Genomics and the Association for Molecular Pathology. Genet. Med. 17, 405–424. doi: 10.1038/gim.2015.30
Rizzi, S., Spagnoli, C., Salerno, G. G., Frattini, D., Caraffi, S. G., Trimarchi, G., et al. (2020). Severe intellectual disability, absence of language, epilepsy, microcephaly and progressive cerebellar atrophy related to the recurrent de novo variant p.(P139L) of the CAMK2B gene: a case report and brief review. Am. J. Med. Genet. A 182, 2675–2679. doi: 10.1002/ajmg.a.61803
Roberts, A. E. (2001). “Noonan syndrome. [updated 2022 Feb 17],” in GeneReviews§[Internet], eds M. P. Adam, H. H. Ardinger, R. A. Pagon, S. E. Wallace, L. J. H. Bean, K. W. Gripp, et al. (Seattle, WA: University of Washington), 1993–2022.
Ruzzo, E. K., Pérez-Cano, L., Jung, J. Y., Wang, L. K., Kashef-Haghighi, D., Hartl, C., et al. (2019). Inherited and de novo genetic risk for autism impacts shared networks. Cell 178, 850.e26–866.e26. doi: 10.1016/j.cell.2019.07.015
Rylaarsdam, L., and Guemez-Gamboa, A. (2019). Genetic causes and modifiers of autism spectrum disorder. Front. Cell Neurosci. 13:385. doi: 10.3389/fncel.2019.00385
Satterstrom, F. K., Kosmicki, J. A., Wang, J., Breen, M. S., De Rubeis, S., An, J. Y., et al. (2020). Large-scale exome sequencing study implicates both developmental and functional changes in the neurobiology of autism. Cell 180, 568.e23–584.e23. doi: 10.1016/j.cell.2019.12.036
Stolerman, E. S., Francisco, E., Stallworth, J. L., Jones, J. R., Monaghan, K. G., Keller-Ramey, J., et al. (2019). Genetic variants in the KDM6B gene are associated with neurodevelopmental delays and dysmorphic features. Am. J. Med. Genet. A 179, 1276–1286. doi: 10.1002/ajmg.a.61173
Strehlow, V., Heyne, H. O., Vlaskamp, D. R. M., Marwick, K. F. M., Rudolf, G., de Bellescize, J., et al. (2019). GRIN2A-related disorders: genotype and functional consequence predict phenotype. Brain 142, 80–92. doi: 10.1093/brain/awy304
Struja, T., and Capraro, J. (2021). Synchronous pituitary and pineal gland lesions presenting with panhypopituitarism in a patient with widespread colorectal cancer: a case report. Oxf. Med. Case Rep. 2021:omab030. doi: 10.1093/omcr/omab030
Szczurkowska, J., Pischedda, F., Pinto, B., Managò, F., Haas, C. A., Summa, M., et al. (2018). NEGR1 and FGFR2 cooperatively regulate cortical development and core behaviours related to autism disorders in mice. Brain 141, 2772–2794. doi: 10.1093/brain/awy190
Szklarczyk, D., Gable, A. L., Lyon, D., Junge, A., Wyder, S., Huerta-Cepas, J., et al. (2019). STRING v11: protein-protein association networks with increased coverage, supporting functional discovery in genome-wide experimental datasets. Nucleic Acids Res. 47, D607–D613. doi: 10.1093/nar/gky1131
Tartaglia, M., Gelb, B. D., and Zenker, M. (2011). Noonan syndrome and clinically related disorders. Best Pract. Res. Clin. Endocrinol. Metab. 25, 161–179. doi: 10.1016/j.beem.2010.09.002
Tatton-Brown, K., Zachariou, A., Loveday, C., Renwick, A., Mahamdallie, S., Aksglaede, L., et al. (2018). The tatton-brown-rahman syndrome: a clinical study of 55 individuals with de novo constitutive DNMT3A variants. Wellcome Open Res. 3:46. doi: 10.12688/wellcomeopenres.14430.1
Trelles, M. P., Levy, T., Lerman, B., Siper, P., Lozano, R., and Halpern, D. (2021). Individuals with FOXP1 syndrome present with a complex neurobehavioral profile with high rates of ADHD, anxiety, repetitive behaviors, and sensory symptoms. Mol. Autism. 12:61. doi: 10.1186/s13229-021-00469-z
Ullman, N. L., Smith-Hicks, C. L., Desai, S., and Stafstrom, C. E. (2018). De Novo HECW2 mutation associated with epilepsy, developmental decline, and intellectual disability: case report and review of literature. Pediatr. Neurol. 85, 76–78. doi: 10.1016/j.pediatrneurol.2018.03.005
Valentino, F., Bruno, L. P., Doddato, G., Giliberti, A., Tita, R., Resciniti, S., et al. (2021). Exome sequencing in 200 intellectual disability/autistic patients: new candidates and atypical presentations. Brain Sci. 11:936. doi: 10.3390/brainsci11070936
Van der Auwera, G. A., Carneiro, M. O., Hartl, C., Poplin, R., Del Angel, G., Levy-Moonshine, A., et al. (2013). From FastQ data to high-confidence variant calls: the genome analysis toolkit best practices pipeline. Curr. Protoc. Bioinform. 43, 11.10.1–11.10.33. doi: 10.1002/0471250953.bi1110s43
van der Werf, I. M., Jansen, S., de Vries, P. F., Gerstmans, A., van de Vorst, M., Van Dijck, A., et al. (2020). Overrepresentation of genetic variation in the AnkyrinG interactome is related to a range of neurodevelopmental disorders. Eur. J. Hum. Genet. 28, 1726–1733. doi: 10.1038/s41431-020-0682-0
Wang, J., Gong, J., Li, L., Chen, Y., Liu, L., Gu, H., et al. (2018). Neurexin gene family variants as risk factors for autism spectrum disorder. Autism Res. 11, 37–43. doi: 10.1002/aur.1881
Wang, J., Zhang, Q., Chen, Y., Yu, S., Wu, X., and Bao, X. (2019). Rett and Rett-like syndrome: expanding the genetic spectrum to KIF1A and GRIN1 gene. Mol. Genet. Genomic Med. 7:e968. doi: 10.1002/mgg3.968
Wingett, S. W., and Andrews, S. (2018). FastQ screen: a tool for multi-genome mapping and quality control. F1000Resarch 7:1338. doi: 10.12688/f1000research.15931.2
Wiszniewski, W., Hunter, J. V., Hanchard, N. A., Willer, J. R., Shaw, C., Tian, Q., et al. (2013). TM4SF20 ancestral deletion and susceptibility to a pediatric disorder of early language delay and cerebral white matter hyperintensities. Am. J. Hum. Genet. 93, 197–210. doi: 10.1016/j.ajhg.2013.05.027
Yamamoto, G. L., Aguena, M., Gos, M., Hung, C., Pilch, J., Fahiminiya, S., et al. (2015). Rare variants in SOS2 and LZTR1 are associated with Noonan syndrome. J. Med. Genet. 52, 413–421. doi: 10.1136/jmedgenet-2015-103018
Yang, X., Li, H., Ge, J., Chao, H., Li, G., Zhou, Z., et al. (2020). The level of GNE and its relationship with behavioral phenotypes in children with autism spectrum disorder. Medicine 99:e21013. doi: 10.1097/MD.0000000000021013
Yoon, S., Munoz, A., Yamrom, B., Lee, Y. H., Andrews, P., Marks, S., et al. (2021). Rates of contributory de novo mutation in high and low-risk autism families. Commun. Biol. 4:1026. doi: 10.1038/s42003-021-02533-z
Keywords: reverse phenotyping, single event variants, NDD-associated genes, GRIA1 gene, NR4A2 gene, SIN3B gene
Citation: Krgovic D, Gorenjak M, Rihar N, Opalic I, Stangler Herodez S, Gregoric Kumperscak H, Dovc P and Kokalj Vokac N (2022) Impaired Neurodevelopmental Genes in Slovenian Autistic Children Elucidate the Comorbidity of Autism With Other Developmental Disorders. Front. Mol. Neurosci. 15:912671. doi: 10.3389/fnmol.2022.912671
Received: 04 April 2022; Accepted: 11 May 2022;
Published: 23 June 2022.
Edited by:
Salam Salloum-Asfar, Qatar Biomedical Research Institute, QatarReviewed by:
María Eugenia De La Morena-Barrio, University of Murcia, SpainBranko Aleksic, Nagoya University, Japan
Copyright © 2022 Krgovic, Gorenjak, Rihar, Opalic, Stangler Herodez, Gregoric Kumperscak, Dovc and Kokalj Vokac. This is an open-access article distributed under the terms of the Creative Commons Attribution License (CC BY). The use, distribution or reproduction in other forums is permitted, provided the original author(s) and the copyright owner(s) are credited and that the original publication in this journal is cited, in accordance with accepted academic practice. No use, distribution or reproduction is permitted which does not comply with these terms.
*Correspondence: Danijela Krgovic, danijela.krgovic1@um.si